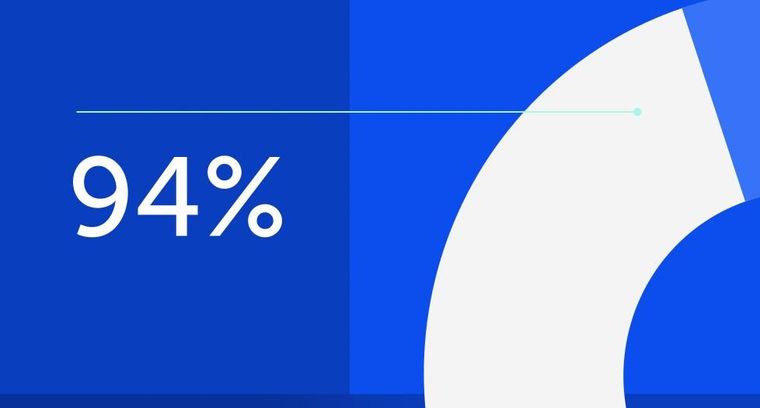
94% of researchers rate our articles as excellent or good
Learn more about the work of our research integrity team to safeguard the quality of each article we publish.
Find out more
REVIEW article
Front. Cardiovasc. Med., 03 November 2022
Sec. Cardiovascular Imaging
Volume 9 - 2022 | https://doi.org/10.3389/fcvm.2022.1043711
This article is part of the Research TopicCardiovascular Imaging in the Integrated Assessment of Metabolic HealthView all 5 articles
Diabetic heart disease is a major healthcare problem. Patients with diabetes show an excess of death from cardiovascular causes, twice as high as the general population and those with diabetes type 1 and longer duration of the disease present with more severe cardiovascular complications. Premature coronary artery disease and heart failure are leading causes of morbidity and reduced life expectancy. Multimodality cardiac imaging, including echocardiography, cardiac computed tomography, nuclear medicine, and cardiac magnetic resonance play crucial role in the diagnosis and management of different pathologies included in the definition of diabetic heart disease. In this review we summarise the utility of multi-modality cardiac imaging in characterising ischaemic and non-ischaemic causes of diabetic heart disease and give an overview of the current clinical practice. We also describe emerging imaging techniques enabling early detection of coronary artery inflammation and the non-invasive characterisation of the atherosclerotic plaque disease. Furthermore, we discuss the role of MRI-derived techniques in studying altered myocardial metabolism linking diabetes with the development of diabetic cardiomyopathy. Finally, we discuss recent data regarding the use of artificial intelligence applied to large imaging databases and how those efforts can be utilised in the future in screening of patients with diabetes for early signs of disease.
World Health Organisation estimates that there are 422 million people living with diabetes mellitus worldwide and 1.5 million deaths are attributed to diabetes annually (1). Patients with diabetes are 2–3 times more likely to have heart disease and 84% of people over 65 with diabetes die from heart disease and stroke. Diabetes is associated with a significant shortening of life. On average, a 50-year-old with diabetes but no history of vascular disease dies approximately 6 years younger than a counterpart without diabetes (2). Early detection of the effect of diabetes on the cardiovascular system could improve preventive measures and initiation of treatments with proven cardiovascular benefit. In this review, we describe the roles of multimodality cardiac imaging in detecting the impact of diabetes on the myocardium and its use for longitudinal follow up.
Global prevalence of diabetes mellitus has been increasing rapidly over the last decade. This has given rise to the worldwide epidemic of diabetic cardiomyopathy, a condition covering the spectrum of myocardial abnormalities linked to the underlying metabolic disturbances observed in patients with diabetes in the absence of coronary artery disease. Numerous studies have explored pathophysiological mechanisms underlying changes in the cardiac structure and resulting function leading to the development of heart failure because of diabetes. In type 1 diabetes as compared to type 2 diabetes, the cardiovascular mortality remains similar, however, the impact of hyperglycaemia on the risk of development of heart disease is more pronounced in type 1 diabetes (3). The aetiology of so-called diabetic cardiomyopathy remains unknown but is likely multifactorial and therefore more difficult to characterise by a single modality. Multi-modality imaging is particularly useful in monitoring disease progression and evaluating the effectiveness of medical interventions. It remains debatable if cardiac imaging could be also used to screen asymptomatic patients with diabetes for the presence for early signs of heart disease.
Patients with diabetes, however, often present with accelerated atherosclerosis, which may lead to the development of ischaemic heart disease and subsequently heart failure reduced ejection fraction. Thus, it is frequently not possible to decipher the contribution of ischaemic and non-ischaemic factors to the development of diabetic heart failure.
Diabetes is associated with at least 2-fold increased risk of coronary artery disease and for many years has been regarded as ‘coronary risk equivalent’ (4). More recently, in recognition of the heterogeneity of patients with diabetes, clinical guidelines have suggested that further risk stratification is warranted when treatment decisions are considered (5, 6). Patients with diabetes and cardiovascular disease (CVD) or diabetes with target organ damage and those with three or more risk factors, as well as with the duration of diabetes more than 20 years are at very high risk (6). Most other subgroups are at moderate risk of developing coronary artery disease (CAD). In a systemic review and meta-analysis patient with diabetes without previous myocardial infarction (MI) had 43% lower risk of CAD than those without diabetes with established history of CAD (7).
Multimodality cardiac imaging, including echocardiography, cardiac computed tomography (CCT), cardiac magnetic resonance (CMR) and nuclear cardiology, has advanced our understanding and treatment of different pathologies included in the definition of diabetic heart disease. Non-invasive assessment of coronary artery disease, structural heart disease, arrhythmias, and heart failure, guides clinical management and leads to a significant improvement in patient outcomes. Multiple imaging modalities are used to detect the signs of diabetic heart disease and the full assessment frequently involves more than one type of scan.
There has been also an increased interest in using hybrid and fusion modalities combining two imaging techniques within one scan and incorporating machine learning into the analysis of images with the goal to improve earlier detection of metabolic and structural abnormalities which could lead to an improvement in long-term clinical outcomes.
Echocardiography plays an important role in the detection of subclinical dysfunction. Left ventricular dysfunction in patients with diabetes can have various presentations including predominantly systolic, diastolic, or mixed phenotypes (Table 1). Therefore, cardiac imaging should not only detect those abnormalities but also characterise the pathogenesis and offer deep phenotyping.
Table 1. Summary of studies assessing the role of echocardiography in the diagnosis of diabetic cardiomyopathy.
Echocardiography is often the first-line evaluation of cardiac structure and function given its high temporal and spatial resolution, safety, availability, and cost-effectiveness. Left ventricular volumes and ejection fraction derived from 3D echocardiography has shown accuracy and reproducibility comparable to cardiac MRI (8). Advancements in image-based analysis of local myocardial deformation, including strain and Doppler tissue imaging, have allowed for the quantitative assessment of subclinical myocardial dysfunction and myocardial strain (9–11). Echocardiography plays central role in the assessment of diastolic dysfunction so prevalent among people with diabetes and obesity.
Key parameters assessing diastolic dysfunction and structural changes have been positively correlated with progression to clinical heart failure. Structural changes identified include larger end-systolic and end-diastolic dimension, greater left ventricular mass in the context of similar wall thickness and greater left atrial area (12). Several studies have assessed signs of pre-clinical diastolic dysfunction in patients with diabetes with consistent results (12–16). In the I-Preserve trial (Irbesartan in Heart Failure with Preserved Ejection Fraction) patients with diabetes were shown to have significant echocardiographic abnormalities associated with diastolic dysfunction comparing to non-diabetic counterparts (12). This suggests that the pathogenesis of diabetic cardiomyopathy at the early stages includes higher left atrial pressures, increased left ventricular stiffness with reduced myocardial relaxation and impaired left ventricular filling. Interestingly, prior to the onset of overt cardiac disease the degree of diabetic control, microvascular complication or insulin requirement is not associated with indices of heart function or reflectivity in type 2 diabetic patients (13). Noteworthy, the duration of diabetes has been associated with the progression to left ventricular diastolic dysfunction with early diastolic impairment noted in the first five years from diagnosis (13). Conversely, in type 1 diabetic patients, e’ mean has been identified to correlate with HbA1c values and duration of DM1 (14).
Hence those echocardiographic features of diastolic dysfunction as well as left ventricular hypertrophy are often regarded as early signs that diabetes affected the myocardium (17). Age, obesity, and hypertension can frequently affect those parameters of diastolic dysfunction. Obesity frequently coexists with type 2 diabetes and has an additive detrimental effect on diastolic function (18). Although diastolic dysfunction has been linked to such processes as myocardial fibrosis, myocardial triglyceride accumulation and insulin resistance, echocardiographic features of diastolic dysfunction have been also attributable to coronary microvascular dysfunction (19).
Patients with history of diabetes and significant coronary artery disease commonly present with heart failure reduced ejection fraction (HFrEF). Echocardiograms of those patients may detect regional wall motion abnormalities, alterations in strain and in the later stage also reduced ejection fraction. Abnormal global longitudinal strain (GLS) has been described as an early sign of developing HFrEF in patients with diabetes (13, 20). The clinical implication of these changes has been further examined with the endpoint of increased admissions secondary to cardiovascular disease or death related to cardiovascular disease. Jøgensen et al. found that when assessing a broader range of patients with diabetes and clinical heart failure, the structural and functional changes were associated with higher risk of progression to the cardiovascular disease (21). LVEF, GLS and GLS rate, E/e’ ratio was associated with the progression to heart failure (21). E/e’ ratio in combination with NT-proBNP levels have been shown to identify patients at highest risk of cardiovascular disease (22). Another study found that in the community-based patients with diabetes, screening of asymptomatic patients without previous history of coronary artery disease resulted in better long-term outcome although the result could not be explained by the revascularisation alone (23).
Stress echocardiography has been shown to be an accurate and reliable imaging modality to diagnose coronary artery disease and predict the long-term outcome in patients with diabetes (24). Exercise stress echocardiography is often used to confirm diminished systolic and diastolic functional reserve in this population (25). Although the negative predictive value of exercise stress echocardiography for myocardial ischaemia is reduced in patients with diabetes when compared to the non-diabetic population, recent analysis using dual-imaging dipyridamole stress echocardiography combining conventional wall motion analysis with Doppler-derived coronary flow velocity reserve (CFVR) of the left anterior descending coronary artery showed that abnormal findings were strong and independent predictors of major cardiovascular events in patients with DM (26).
It has been shown previously that patients with diabetes have higher burden of plaque disease than those without diabetes (27) and the extent of CAD in patients with diabetes is comparable to patients with previous MIs (28). Noteworthy, women with diabetes have similar extent of CAD to men, which is different than in the general population (28). Moreover, women with diabetes are at higher risk of MI then men despite low burden of CAD. High coronary artery calcium (CAC) score correlates with a higher number of cardiovascular events and an increased mortality (29–32). CAC is recognised as a better predictor of coronary artery events than the Framingham score and the UKPDS Risk Engine and has been shown to have much higher annual increase than in patients without diabetes. CAC has been also shown to be a good biomarker of CAD progression in people with diabetes (33–36) and therefore has been proposed for monitoring of asymptomatic patient with diabetes and CAD.
Advancements in the CT technology resulting in a reduction of the radiation doses increased the popularity of CT coronary angiography (CTA) in the assessment of CAD in patients with diabetes (Figure 1). In asymptomatic patients with diabetes, on average studies reported the prevalence of obstructive coronary artery disease in 25–30% of patients, any coronary atheroma in 76% and multivessel coronary atheroma in 55% (37–39). In a cohort of asymptomatic patients with diabetes approximately 17% had multivessel disease and significant lesions in the left main stem or proximal left anterior descending artery (40). Moreover, an increased severity of coronary artery disease was associated with poor prognosis implying an important prognostic role of CTA in the management of patients with diabetes. Those results highlighted the role of CTA in finding high-risk patients even among asymptomatic patients. The FACTOR-64 (For Asymptomatic Obstructive Coronary Artery Disease Among High-Risk Diabetic Patients Using CT Angiography) randomised controlled trial evaluated the utility of routine CTA screening in patients with diabetes (41). CTA showed no atheroma in 31%, mild stenosis in 46%, moderate in 12%, and severe in 11% of patients. Although coronary CTA screening prompted aggressive risk factor modifications in 70% of patients, there was no significant reduction in CAD events in the group randomised to the CTA screening (41). Thus, CTA is currently not recommended to risk stratify patients with diabetes (42). An ongoing Computed Tomography Coronary Angiography for the Prevention of Myocardial Infarction (SCOT-Heart 2) randomised controlled trial investigates the role of CTA in a broader primary prevention population including patients with diabetes.
Figure 1. Illustration of corresponding anatomical coronary CTA plaque analysis and functional assessment with FFR-CT in a patient with diabetes. Reconstructions confirm three vessel disease with diffuse mixed plaque and severe flow limiting stenoses in the distal LAD, distal RCA, and proximal intermediate branch as per FFR-CT functional analysis. (A) CTA curved multiplanar reconstruction of the left anterior descending artery (LAD) demonstrating diffuse calcific plaque disease in the proximal segment with mild stenosis and low attenuation plaque in the mid vessel with severe moderate flow limiting stenosis as per angiographic assessment. (B) CTA curved multiplanar reconstruction of the right coronary artery (RCA) demonstrating diffuse mixed plaque disease throughout the vessel with most significant stenosis in the mid segment. (C) CTA curved multiplanar reconstruction of the intermediate artery reconstruction demonstrating diffuse mixed plaque disease with severe stenosis at the ostium of the vessel. (D) Computational fractional flow reserve (FFR) CT by HeartFlow indicating haemodynamically moderate flow impairment (FFR-CT of 0.82) across the lesion in the mid segment and severe (FFR-CT of 0.67) in the distal segment of the LAD, severe stenoses in the proximal intermediate (FFR-CT of 0.65) and distal segment of RCA (FFR-CT of 0.65). (E) Three-dimensional model illustrating the reconstruction of coronary arteries (Syngovia Siemens software).
Myocardial computed tomography perfusion (CTP) imaging is an emerging CT-based method for detecting myocardial ischaemia with good accuracy (43) and correlated with nuclear myocardial perfusion imaging. The combination of anatomical information from CTA with functional assessment from CT adenosine-stress perfusion CT increases significantly the radiation exposure to the patients and therefore limits its wider use.
FFR-CT is an alternative functional technique, which combines anatomic and physiologic information in a single non-invasive test. Patients with diabetes have a high burden of coronary artery disease and it has been shown that every sixth asymptomatic patient at the time of the diagnosis of diabetes has haemodynamically significant plaque disease as evaluated by FFR-CT (44) (see an example of FFR-CT assessment in Figure 1). FFR-CT has improved specificity without impacting on the sensitivity of CTA in detecting haemodynamic significant coronary artery disease (45). Quality of image acquisition in CTA has long been established as important with multiple factors impacting calculation of FFR (46). Currently only HeartFlow technology is licenced by both the National Institute of Clinical Excellence and the US Food and Drug Administration for FFR-CT assessment (46, 47). Post-acquisition image processing occurs following images being sent to HeartFlow. Thus, producing FFR-CT results takes time with currently only >50% of images being processed in less than 5 h, limiting its use in the acute clinical setting. Local FFR-CT techniques will likely progress over time to improve streamlining, access, and timeliness of the technology.
European and American societies have recently introduced the use of CTA in patients with diabetes who present with a history of chest pain (6, 48). The SCOT-Heart trial, which recruited 444 patients with diabetes showed a significant reduction in the risk of cardiovascular mortality and myocardial infarction with CTA-guided management (49). The Prospective Multicentre Imaging Study for Evaluation of Chest Pain (PROMISE) randomised patients to CTA or stress test and showed that abnormal stress test was more specific for predicting primary outcome than CTA (50). Noteworthy, in the CTA arm 84% of events occurred in patients with calcium score above zero and only 43% of events in the functional test group. However, in the prespecified sub-analysis of 1908 recruited patients with diabetes, those who underwent CTA had a lower risk of cardiovascular event comparing to the stress test arm. The addition of computational fractional flow reserve may therefore improve even further identification of haemodynamically significant lesion (51). ISCHEMIA trial tested whether patients with moderate or severe ischaemia on stress test benefit from revascularisation in the setting of optimal medical therapy. There was no difference in the primary end point among participants with diabetes (41.8%) highlighting the importance of the optimal medical therapy (52).
Mapping of peri-coronary adipose tissue attenuation (PCAT) on CTA has been recently proposed as a non-invasive marker of coronary artery inflammation (53). Several clinical studies have confirmed that so called fat attenuation index (FAI) can improve the prediction of coronary events beyond traditional risk factors and CTA metrics (53, 54). FAI was first derived from studies demonstrating that inflammatory markers released from inflamed coronary arteries modify the composition of perivascular adipose tissue. Those changes can be detected using a CTA. Thus, using FAI assessment we can extract additional information from a standard CTA scan relating to coronary artery inflammation (55). Noteworthy, it has been demonstrated that lesion-specific FAI enhances the predictive ability of CTA plaque characterisation for ischaemia as assessed by invasive coronary angiography (56). When FAI was added to the CTA stenosis estimation the AUC of such model was comparable to the one achieved with the invasive assessment of stenosis severity. The value of PCAT has been now also assessed as a predictive tool in patients with diabetes (57). Adding PCAT along with findings of CTA improved the model fit for predicting cardiovascular events in type 2 diabetes patients and helped to identify patients at a higher risk independently of the plaque characteristics. PCAT is a dynamic marker reported to be markedly reduced in response to anti-inflammatory treatment (58) (Figure 2).
Figure 2. CT coronary angiography derived Fat Attenuation Index (FAI) derived from the analysis of Perivascular Adipose Tissue (PVAT) surrounding coronary arteries detects inflammation and may be used to guide the management of patients with diabetes and increased cardiovascular risk. Red-blue colour indicates inflammation and correlates with high risk of future cardiovascular events; yellow colour indicates low inflammation (55). FAI score can be provided for each of the main coronary arteries and considers FAI weighted for tube voltage, anatomical factors, and basic demographics. (A) Significantly increased coronary artery inflammation (FAI score 95th centile). (B) Follow-up scan after initiation of statin treatment shows significant reduction in inflammation (FAI score 58th centile). Panels (A,B) show CPR (top panel) and axial views (bottom panel).
Stress myocardial perfusion scintigraphy (MPS) is widely used in patients with and without diabetes to detect haemodynamically significant coronary artery disease. It has been reported that in 20–25% of asymptomatic patients with the diagnosis of diabetes MPS detects ischaemia (59, 60) and in those patients it is associated with cardiovascular events (61). Microvascular/endothelial dysfunction is frequently described in patients with diabetes and can be assessed by quantitative positron emission tomography (PET). Measurements with a blood flow radiotracer such as 82Rubidium, 13N-ammonia or 15O-water at rest and after vasodilator-stress enable calculation of coronary flow reserve (62). Moreover, PET has been also used to study utilisation of glucose and fatty acids in 31 young women and showed that insulin resistance correlated with utilisation and oxidation of fatty acids (63). Due to the complexity of its protocol and the high price, it remains to be a research tool rather than a technique which could be used to assess patients.
The cardiac consequences of diabetes on the myocardium ensue from metabolic and functional alterations. CMR is a versatile imaging modality (Figure 3) able to describe both ischaemic and non-ischaemic cardiomyopathies and detect haemodynamically significant coronary artery disease. Observational studies described abnormalities in structure and function in patients with diabetes (64, 65). Post mortem studies confirmed distinct ventricular hypertrophy with diffuse fibrotic strands observed between muscle fibres on histopathology and identified it as diabetic cardiomyopathy (66). The strong biological link between diabetes and hypertension and the phenotypic similarity of left ventricular hypertrophy caused by both conditions makes it difficult to distinguish (67). Diabetes was previously described to be associated with left ventricular concentric remodelling and a modestly increased mass (68).
Figure 3. Characterisation of diabetic heart disease with cardiac magnetic resonance including anatomical and functional assessment as well as tissue characterisation using CVI42 software. (A) Evaluation of LV and RV volumes and function using short axis stuck images. (B) Assessment of left atrial size. (C) Analysis of longitudinal and (D) circumferential strain. (E) Estimation of epi- and pericardial fat tissue and (F) 17 segments AHA model representation of average segmental native T1 mapping values. (G) Color map of native T1 mapping. (H) Late gadolinium images show no evidence of focal fibrosis.
Although cardiac magnetic resonance is mostly used to provide information about systolic function of the left ventricle, it can also characterise diastolic dysfunction and therefore can offer a holistic approach to the early detection of myocardial dysfunction associated with diabetes. Different MRI technique are currently available to evaluate diastolic function (phase contrast imaging for flow analysis, myocardial tagging for regional function analysis) allowing such measurements as longitudinal fractional shortening used when assessing patient presenting with heart failure preserved ejection fraction (HFpEF). Strain-encoded (SENC) (69), displacement encoding with simulated echoes (DENSE) (70) and MRI feature-tracking (MRI-FT) offer additional assessment of the regional function (71). Four-dimensional flow (4D-Flow) MRI is superior to Doppler echocardiography and offers evaluation of intracardiac velocity. Additionally, MRI-derived detection of parameters describing left atrial enlargement and dysfunction have been also shown to be predictive for identifying diastole dysfunction (72). Regrettably, those techniques are time-consuming and require complex post-processing and long image acquisition, what significantly limit its use.
Late gadolinium images confirmed silent myocardial infarctions in 4.3% of asymptomatic type 1 diabetes patients (65) and in 17% of older asymptomatic type 2 diabetes patients (73).
The community-based ICELAND-MI study, which showed that LGE diagnosis of unrecognised myocardial infarction was associated with a 45% increased mortality (74). Novel tissue characterisation techniques such as mapping techniques can demonstrate the degree of interstitial fibrosis observed in patients with diabetes. Extracellular volume (ECV) fraction, a measure of interstitial fibrosis linked to diastolic dysfunction, has been described to be increased in patients with diabetes (75, 76), however, other clinical studies reported inconsistent results regarding the presence of interstitial fibrosis in patients with diabetes (77). Those tissue characteristics by CMR can describe early signs of diabetic cardiomyopathy with the presence of interstitial fibrosis and subclinical abnormalities, which can be also described by tissue tracking images (78). It remains poorly understood if those changes could represent LV remodelling caused by the higher prevalence of hypertension in patients with diabetes.
MR spectroscopy techniques have been instrumental in describing an increased myocardial triglyceride content in patients with diabetes type 2 (79–81). Myocardial triglycerides can be quantified using hydrogen MRI spectroscopy (1H MRS) (82) and has been shown to be increased in ageing, and in patients with diabetes and obesity and is associated with myocardial dysfunction (79, 83). Noteworthy, weight loss was able to partially reverse myocardial triglyceride accumulation in patients with diabetes and therefore improve left ventricular function (81). Future studies will determine if novel glucose-lowering therapies will result in reducing myocardial triglyceride content. Altered myocardial metabolism has been considered among the potential mechanisms leading to diabetic heart disease. The energetic state of myocardium can be measured by phosphorus magnetic resonance spectroscopy (31P-MRS), which allows non-invasive assessment of relative concentration of PCr to ATP (PCr/ATP) as a sensitive index (84). Decreased PCr/ATP has been shown to be a predictor of mortality and left ventricle dysfunction (85). Such energetic deficit in diabetic cardiomyopathy is further exacerbated by exercise and is associated with coronary microvascular dysfunction (85). More recently, hyperpolarised 13C magnetic resonance spectroscopy has been shown to non-invasively assess physiological and pathological changes in cardiac metabolism in the human heart in health and disease (86). It demonstrated the emerging potential for hyperpolarised imaging in assessing mechanisms underpinning the development of heart failure in diabetes.
Increased oxidative stress, altered substrate use, and insufficient myocardial perfusion have been proposed as the mechanisms underlying myocardial structural and functional changes observed in the diabetic heart disease. Patients with type 2 diabetes have higher global myocardial perfusion at rest and lower maximal myocardial blood flow during vasodilator-induced stress than control subjects (87). Adenosine stress MRI has been shown to have a good ability to detect haemodynamically significant coronary artery disease in patients with diabetes (88, 89). CMR first-pass perfusion imaging during vasodilatory stress with adenosine or regadenoson has been now widely accepted as the first-choice test for cardiovascular risk stratification of patients with diabetes mellitus. The presence of inducible myocardial ischaemia, defined as at least one positive segment of >1 voxel thickness lasting for at least three heartbeats, was the strongest predictor of clinical outcome (90). However, this is usually evaluated with qualitative or semi-quantitative methods, and it can be inaccurate when myocardial blood flow is globally reduced (in 3-vessel disease). More recently, a dual-sequence protocol was developed to produce an in-line perfusion mapping allowing a pixel-wise quantification of myocardial blood flow (91). This technique can be used to detect physiologically significant CAD, microvascular disfunction and distinguish it from multivessel disease as defined by invasive measurements (92). In a larger cohort of patients with both suspected and known coronary artery disease this AI based approach was shown to be an independent predictor of adverse cardiovascular outcomes (93). Hence, CMR stress perfusion is used to detect highly prevalent multivessel CAD and microvascular angina in patients with diabetes, which is a result of endothelial dysfunction. Automated methods utilising deep learning have been shown to provide one-click analysis and reporting of cardiac perfusion mapping in a manner comparable to manual assessment (94).
Over the last decade we have also observed an increased interest in hybrid cardiac PET/MR imaging protocols, which have been incorporated into clinical workflows in many centres. PET is known for its role in quantification of myocardial perfusion and coronary flow reserve as well as visualisation and quantification of metabolic and inflammatory processes at the molecular level (95). Thus, when added to CMR, a technique offering a broad range of capabilities, it is regarded as a promising tool to diagnose and manage metabolic changes leading to myocardial remodelling in diabetic heart disease. A loss in metabolic flexibility reflected in an overdependence on fatty acids as the primary energy source limiting the hearts’ ability to alter substrate metabolism in response to varying physiological and metabolic conditions has been confirmed by preclinical and early human studies using PET/MRI (96). Moving forward a multi-modality imaging protocols interrelating hybrid imaging techniques may be required to improve our understanding of complex processes underlying development of diabetic cardiomyopathy.
Although asymptomatic cardiovascular disease is common in people with diabetes and is associated with adverse outcome, the role of multimodality cardiac imaging in screening remains debatable. Large randomised clinical trials testing the value of using imaging in early detection of diabetic heart disease are required to introduce such investigations into clinical practice. The success of novel therapies with proven cardiovascular benefit should motivate such investigations in future and lead to the development of clinical guidance utilising various cardiac imaging modalities in detecting early signs of heart disease in those patients. Patients with diabetes represent a heterogenous group and may require clinical assessment with several imaging modalities in the course of their disease. Figure 4 presents our proposed sequence of using various imaging modalities in screening patients with diabetes. However, several requirements should be met, before a test could be included in a widely accepted screening programme. Firstly, such a test should have superior sensitivity and specificity and be able to differentiate high and low risk patients. Secondly, given high costs of CT and MRI scans the cost-effectiveness of imaging modality should be considered against the benefit of detecting a particular outcome. Thirdly, if screening for early signs of diabetic cardiomyopathy would be considered, it may need to be undertaken in individuals with overall higher risk of HF as defined by other well recognised HF predictors such as more advanced age, hypertension, and microvascular complications of diabetes. This approach would emphasise the role of combining imaging biomarkers with established risk score calculators. We are observing an increasing use of such risk calculators allowing estimation of risk scores of cardiovascular outcomes in patients with diabetes based on their clinical characteristics. UKPDS Risk Engine (97), QDiabetes (98), WATCH-DM (99) and TRS-HFDM (100) risk scores are the most frequently cited examples. The advances in using AI algorithms in image interpretation is also very promising. For example, an application of AI to ECG, a routine, widely available, low-cost test has been proved to identify asymptomatic patients with heart failure (101). One could predict that AI- enhanced ECG, which is recorded for each patient with the diagnosis of diabetes could become the first screening tool. It would allow clustering patients into subgroups with differing cardiovascular risks and refining identification of patients requiring appropriate imaging modality to define the stage of their disease.
Figure 4. Suggested strategy of using various techniques and imaging modalities in screening patients with diabetes for signs of diabetic heart disease. Outline of currently available techniques and imaging modalities allowing characterisation of different stages of the disease with focus on processes leading to the development of diabetic heart failure.
From the cost-efficiency point of view, echocardiography with strain imaging has the highest chance to be considered as a modality of choice in screening for early signs of diabetic heart disease. Echocardiography remains the most accessible imaging modality and with the use of novel parameters can phenotype various subtypes of heart failure associated with the diagnosis of diabetes. It is also the most versatile technique able to examine and quantify the degree of systolic and diastolic function, provide evidence of regional wall motion abnormalities, assess signs of left ventricular hypertrophy, detect an increased left atrial pressure, and describe the presence of rarer types of inherited and acquired cardiomyopathies.
From the coronary artery point of view, calcium score remains to be a powerful tool for personalised risk assessment and decision making. Patients with diabetes and calcium score of zero have low event rate. Individuals with an increased calcium score may require further assessment with CTA and/or functional test to guide a wide range of preventive therapies and treatment strategies. In symptomatic patients, CTA has a well-established role and the addition of computational fraction flow reserve as well as novel imaging markers such as PCAT may strengthen its role even further. Given the complexity of mechanisms leading to myocardial involvement in patients with diabetes and all strengths and weaknesses of each imaging modality, the role of imaging in screening of asymptomatic patients with diabetes remains questionable.
The core value of cardiac magnetic resonance lies in the tissue characterisation, the detection of diffuse myocardial fibrosis, and an increase myocardial triglyceride accumulation. MR spectroscopy techniques including novel hyperpolarised 13C magnetic resonance spectroscopy allow assessment of altered myocardial metabolism in the diabetic heart and holds a promise to uncover the link between diabetes and heart diseases. Noteworthy, MRI provides multiple metrics assessing multi-organ health within one acquisition, which is particularly valuable in case of a systemic disease such as diabetes.
Another way of utilising multi-modality imaging techniques within clinical screening pathways is to focus on the highest risk of certain outcome and choose a test, which is most likely to identify that outcome. For example, in patients with diabetes who are at high risk of developing coronary artery disease, CTA will identify patients with obstructive coronary artery disease or left main stem disease. Depending on local availability, stress echocardiography and perfusion scintigraphy or perfusion CMR could also be considered as first line tests to detect haemodynamically significant coronary artery disease. On the other hand, in those patients who have higher risk of heart failure, ECG and echocardiography will be used as the first line investigations followed by CMR. Figure 4 outlines the strength of each imaging modality and proposes most appropriate use of various techniques.
The growing interest in the use of artificial intelligence in the field of imaging will certainly produce new insights into such complex pathophysiology. The rapid increase in the number of studies confirming superiority of AI algorithms in analysing imaging data is very encouraging. Most importantly, AI will also increase standardisation of interpretation and quantification in imaging. The role of AI has been also confirmed in deep phenotyping of highly heterogenous cohort of patients with diabetes, which on its own could personalise the choice of most appropriate imaging modality in various cohorts of patients. The myriad of applications of AI is a harbinger of a potential breakthrough in how we will come to view the utility of various imaging markers in the early detection of diabetic heart disease is the not-so-distant future.
MW wrote the first draft of the manuscript and finalised the last version after co-authors comments. MG prepared Table 1 and Figure 4. AR drafted section of the manuscript describing the role of echocardiography. AB prepared Figure 3. All authors contributed to manuscript revision, read, and approved the submitted version.
This work was supported by Mayo Clinic International.
The authors are grateful Andy Beale (Great Western Hospital NHS Trust in Swindon) for providing example of CTA images used in Figure 2.
The authors declare that the research was conducted in the absence of any commercial or financial relationships that could be construed as a potential conflict of interest.
All claims expressed in this article are solely those of the authors and do not necessarily represent those of their affiliated organizations, or those of the publisher, the editors and the reviewers. Any product that may be evaluated in this article, or claim that may be made by its manufacturer, is not guaranteed or endorsed by the publisher.
1. World Health Organization,. Diabetes. (2022). Available online at: https://www.who.int/health-topics/diabetes#tab=tab_1
2. Rao Kondapally Seshasai S, Kaptoge S, Thompson A, Di Angelantonio E, Gao P, Sarwar N, et al. Diabetes mellitus, fasting glucose, and risk of cause-specific death. N Engl J Med. (2011) 364:829–41. doi: 10.1056/NEJMoa1008862
3. Juutilainen A, Lehto S, Ronnemaa T, Pyorala K, Laakso M. Similarity of the impact of type 1 and type 2 diabetes on cardiovascular mortality in middle-aged subjects. Diabetes Care. (2008) 31:714–9. doi: 10.2337/dc07-2124
4. Haffner SM, Lehto S, Ronnemaa T, Pyorala K, Laakso M. Mortality from coronary heart disease in subjects with type 2 diabetes and in nondiabetic subjects with and without prior myocardial infarction. N Engl J Med. (1998) 339:229–34. doi: 10.1056/NEJM199807233390404
5. American Diabetes A. Standards of medical care in diabetes–2013. Diabetes Care. (2013) 36(Suppl 1):S11–66. doi: 10.2337/dc13-S011
6. Grant PJ, Cosentino F. The 2019 ESC Guidelines on diabetes, pre-diabetes, and cardiovascular diseases developed in collaboration with the EASD: New features and the ‘Ten Commandments’ of the 2019 Guidelines are discussed by Professor Peter J. Grant and Professor Francesco Cosentino, the Task Force chairmen. Eur Heart J. (2019) 40:3215–7. doi: 10.1093/eurheartj/ehz687
7. Bulugahapitiya U, Siyambalapitiya S, Sithole J, Idris I. Is diabetes a coronary risk equivalent? Systematic review and meta-analysis. Diabet Med. (2009) 26:142–8. doi: 10.1111/j.1464-5491.2008.02640.x
8. Dorosz JL, Lezotte DC, Weitzenkamp DA, Allen LA, Salcedo EE. Performance of 3-dimensional echocardiography in measuring left ventricular volumes and ejection fraction: a systematic review and meta-analysis. J Am Coll Cardiol. (2012) 59:1799–808. doi: 10.1016/j.jacc.2012.01.037
9. Mor-Avi V, Lang RM, Badano LP, Belohlavek M, Cardim NM, Derumeaux G, et al. Current and evolving echocardiographic techniques for the quantitative evaluation of cardiac mechanics: ASE/EAE consensus statement on methodology and indications endorsed by the Japanese Society of Echocardiography. J Am Soc Echocardiogr. (2011) 24:277–313. doi: 10.1016/j.echo.2011.01.015
10. Celentano A, Vaccaro O, Tammaro P, Galderisi M, Crivaro M, Oliviero M, et al. Early abnormalities of cardiac function in non-insulin-dependent diabetes mellitus and impaired glucose tolerance. Am J Cardiol. (1995) 76:1173–6. doi: 10.1016/S0002-9149(99)80330-0
11. Liu JE, Palmieri V, Roman MJ, Bella JN, Fabsitz R, Howard BV, et al. The impact of diabetes on left ventricular filling pattern in normotensive and hypertensive adults: the Strong Heart Study. J Am Coll Cardiol. (2001) 37:1943–9. doi: 10.1016/S0735-1097(01)01230-X
12. Kristensen SL, Mogensen UM, Jhund PS, Petrie MC, Preiss D, Win S, et al. Clinical and echocardiographic characteristics and cardiovascular outcomes according to diabetes status in patients with heart failure and preserved ejection fraction: A report from the i-preserve trial (Irbesartan in Heart Failure With Preserved Ejection Fraction). Circulation. (2017) 135:724–35. doi: 10.1161/CIRCULATIONAHA.116.024593
13. Fang ZY, Yuda S, Anderson V, Short L, Case C, Marwick TH. Echocardiographic detection of early diabetic myocardial disease. J Am Coll Cardiol. (2003) 41:611–7. doi: 10.1016/S0735-1097(02)02869-3
14. Weber TR, Silva RLD, Cossul S, Lofrano Alves MS, Lee SVS, Brum Marques JL. Echocardiographic evaluation in type 1 diabetes mellitus. Rev Port Cardiol (Engl Ed). (2021) 40:757–65. doi: 10.1016/j.repce.2021.08.003
15. Negishi K. Echocardiographic feature of diabetic cardiomyopathy: where are we now? Cardiovasc Diagn Ther. (2018) 8:47–56. doi: 10.21037/cdt.2018.01.03
16. Enomoto M, Ishizu T, Seo Y, Kameda Y, Suzuki H, Shimano H, et al. Myocardial dysfunction identified by three-dimensional speckle tracking echocardiography in type 2 diabetes patients relates to complications of microangiopathy. J Cardiol. (2016) 68:282–7. doi: 10.1016/j.jjcc.2016.03.007
17. Zarich SW, Arbuckle BE, Cohen LR, Roberts M, Nesto RW. Diastolic abnormalities in young asymptomatic diabetic patients assessed by pulsed Doppler echocardiography. J Am Coll Cardiol. (1988) 12:114–20. doi: 10.1016/0735-1097(88)90364-6
18. Ng ACT, Prevedello F, Dolci G, Roos CJ, Djaberi R, Bertini M, et al. Impact of diabetes and increasing body mass index category on left ventricular systolic and diastolic function. J Am Soc Echocardiogr. (2018) 31:916–25. doi: 10.1016/j.echo.2018.02.012
19. Halabi A, Nolan M, Potter E, Wright L, Asham A, Marwick TH. Role of microvascular dysfunction in left ventricular dysfunction in type 2 diabetes mellitus. J Diabetes Complicat. (2021) 35:107907. doi: 10.1016/j.jdiacomp.2021.107907
20. Moir S, Hanekom L, Fang ZY, Haluska B, Wong C, Burgess M, et al. Relationship between myocardial perfusion and dysfunction in diabetic cardiomyopathy: a study of quantitative contrast echocardiography and strain rate imaging. Heart. (2006) 92:1414–9. doi: 10.1136/hrt.2005.079350
21. Jorgensen PG, Biering-Sorensen T, Mogelvang R, Fritz-Hansen T, Vilsboll T, Rossing P, et al. Predictive value of echocardiography in Type 2 diabetes. Eur Heart J Cardiovasc Imaging. (2019) 20:687–93. doi: 10.1093/ehjci/jey164
22. Rorth R, Jorgensen PG, Andersen HU, Christoffersen C, Gotze JP, Kober L, et al. Cardiovascular prognostic value of echocardiography and N terminal pro B-type natriuretic peptide in type 1 diabetes: the Thousand & 1 Study. Eur J Endocrinol. (2020) 182:481–8. doi: 10.1530/EJE-19-1015
23. Bates RE, Omer M, Abdelmoneim SS, Arruda-Olson AM, Scott CG, Bailey KR, et al. Impact of stress testing for coronary artery disease screening in asymptomatic patients with diabetes mellitus: A community-based study in olmsted county, minnesota. Mayo Clin Proc. (2016) 91:1535–44. doi: 10.1016/j.mayocp.2016.07.013
24. Chaowalit N, Arruda AL, McCully RB, Bailey KR, Pellikka PA. Dobutamine stress echocardiography in patients with diabetes mellitus: enhanced prognostic prediction using a simple risk score. J Am Coll Cardiol. (2006) 47:1029–36. doi: 10.1016/j.jacc.2005.10.048
25. Sharma R, Pellerin D. Stress echocardiogaphy: a useful test for assessing cardiac risk in diabetes. Vasc Health Risk Manag. (2009) 5:1–7. doi: 10.2147/VHRM.S3165
26. Cortigiani L, Gherardi S, Faggioni M, Bovenzi F, Picano E, Petersen C, et al. Dual-Imaging stress echocardiography for prognostic assessment of high-risk asymptomatic patients with diabetes mellitus. J Am Soc Echocardiogr. (2017) 30:149–58. doi: 10.1016/j.echo.2016.10.003
27. Wong ND, Sciammarella MG, Polk D, Gallagher A, Miranda-Peats L, Whitcomb B, et al. The metabolic syndrome, diabetes, and subclinical atherosclerosis assessed by coronary calcium. J Am Coll Cardiol. (2003) 41:1547–53. doi: 10.1016/S0735-1097(03)82532-9
28. Schurgin S, Rich S, Mazzone T. Increased prevalence of significant coronary artery calcification in patients with diabetes. Diabetes Care. (2001) 24:335–8. doi: 10.2337/diacare.24.2.335
29. Malik S, Budoff MJ, Katz R, Blumenthal RS, Bertoni AG, Nasir K, et al. Impact of subclinical atherosclerosis on cardiovascular disease events in individuals with metabolic syndrome and diabetes: the multi-ethnic study of atherosclerosis. Diabetes Care. (2011) 34:2285–90. doi: 10.2337/dc11-0816
30. Anand DV, Lim E, Hopkins D, Corder R, Shaw LJ, Sharp P, et al. Risk stratification in uncomplicated type 2 diabetes: prospective evaluation of the combined use of coronary artery calcium imaging and selective myocardial perfusion scintigraphy. Eur Heart J. (2006) 27:713–21. doi: 10.1093/eurheartj/ehi808
31. Yeboah J, Erbel R, Delaney JC, Nance R, Guo M, Bertoni AG, et al. Development of a new diabetes risk prediction tool for incident coronary heart disease events: the Multi-Ethnic Study of Atherosclerosis and the Heinz Nixdorf Recall Study. Atherosclerosis. (2014) 236:411–7. doi: 10.1016/j.atherosclerosis.2014.07.035
32. Raggi P, Shaw LJ, Berman DS, Callister TQ. Prognostic value of coronary artery calcium screening in subjects with and without diabetes. J Am Coll Cardiol. (2004) 43:1663–9. doi: 10.1016/j.jacc.2003.09.068
33. Pandey AK, Blaha MJ, Sharma K, Rivera J, Budoff MJ, Blankstein R, et al. Family history of coronary heart disease and the incidence and progression of coronary artery calcification: Multi-Ethnic Study of Atherosclerosis (MESA). Atherosclerosis. (2014) 232:369–76. doi: 10.1016/j.atherosclerosis.2013.11.042
34. Wong ND, Nelson JC, Granston T, Bertoni AG, Blumenthal RS, Carr JJ, et al. Metabolic syndrome, diabetes, and incidence and progression of coronary calcium: the Multiethnic Study of Atherosclerosis study. JACC Cardiovasc Imaging. (2012) 5:358–66. doi: 10.14341/2071-8713-5059
35. Anand DV, Lim E, Darko D, Bassett P, Hopkins D, Lipkin D, et al. Determinants of progression of coronary artery calcification in type 2 diabetes role of glycemic control and inflammatory/vascular calcification markers. J Am Coll Cardiol. (2007) 50:2218–25. doi: 10.1016/j.jacc.2007.08.032
36. Budoff MJ, Hokanson JE, Nasir K, Shaw LJ, Kinney GL, Chow D, et al. Progression of coronary artery calcium predicts all-cause mortality. JACC Cardiovasc Imaging. (2010) 3:1229–36. doi: 10.1016/j.jcmg.2010.08.018
37. Kamimura M, Moroi M, Isobe M, Hiroe M. Role of coronary CT angiography in asymptomatic patients with type 2 diabetes mellitus. Int Heart J. (2012) 53:23–8. doi: 10.1536/ihj.53.23
38. Roos CJ, Kharagjitsingh AV, Jukema JW, Bax JJ, Scholte AJ. Comparison by computed tomographic angiography-the presence and extent of coronary arterial atherosclerosis in South Asians versus Caucasians with diabetes mellitus. Am J Cardiol. (2014) 113:1782–7. doi: 10.1016/j.amjcard.2014.03.005
39. Halon DA, Dobrecky-Mery I, Gaspar T, Azencot M, Yaniv N, Peled N, et al. Pulse pressure and coronary atherosclerosis in asymptomatic type 2 diabetes mellitus: a 64 channel cardiac computed tomography analysis. Int J Cardiol. (2010) 143:63–71. doi: 10.1016/j.ijcard.2009.01.066
40. Park GM, An H, Lee SW, Cho YR, Gil EH, Her SH, et al. Risk score model for the assessment of coronary artery disease in asymptomatic patients with type 2 diabetes. Medicine (Baltimore). (2015) 94:e508. doi: 10.1097/MD.0000000000000508
41. Muhlestein JB, Lappe DL, Lima JA, Rosen BD, May HT, Knight S, et al. Effect of screening for coronary artery disease using CT angiography on mortality and cardiac events in high-risk patients with diabetes: the FACTOR-64 randomized clinical trial. JAMA. (2014) 312:2234–43. doi: 10.1001/jama.2014.15825
42. Taylor AJ, Cerqueira M, Hodgson JM, Mark D, Min J, O’Gara P, et al. ACCF/SCCT/ACR/AHA/ASE/ASNC/NASCI/SCAI/SCMR 2010 Appropriate use criteria for cardiac computed tomography. A Report of the American college of cardiology foundation appropriate use criteria task force, the society of cardiovascular computed tomography, the American college of radiology, the American heart association, the american society of echocardiography, the american society of nuclear cardiology, the north american society for cardiovascular imaging, the society for cardiovascular angiography and interventions, and the society for cardiovascular magnetic resonance. J Cardiovasc Comput Tomogr. (2010) 4:407.
43. Sorgaard M, Linde JJ, Kofoed KF, Kuhl JT, Kelbaek H, Nielsen WB, et al. Diagnostic value of the updated diamond and forrester score to predict coronary artery disease in patients with acute-onset chest pain. Cardiology. (2016) 133:10–7.
44. Mrgan M, Norgaard BL, Dey D, Gram J, Olsen MH, Gram J, et al. Coronary flow impairment in asymptomatic patients with early stage type-2 diabetes: Detection by FFRCT. Diab Vasc Dis Res. (2020) 17:1479164120958422. doi: 10.1177/1479164120958422
45. Baumann S, Renker M, Hetjens S, Fuller SR, Becher T, Lossnitzer D, et al. Comparison of coronary computed tomography angiography-derived vs invasive fractional flow reserve assessment: meta-analysis with subgroup evaluation of intermediate stenosis. Acad Radiol. (2016) 23:1402–11. doi: 10.1016/j.acra.2016.07.007
46. Tesche C, De Cecco CN, Albrecht MH, Duguay TM, Bayer RR II, Litwin SE, et al. Coronary CT angiography-derived fractional flow reserve. Radiology. (2017) 285:17–33. doi: 10.1148/radiol.2017162641
47. Chen J, Wetzel LH, Pope KL, Meek LJ, Rosamond T, Walker CM. FFRCT: Current status. AJR Am J Roentgenol. (2021) 216:640–8. doi: 10.2214/AJR.20.23332
48. Arnold SV, Bhatt DL, Barsness GW, Beatty AL, Deedwania PC, Inzucchi SE, et al. Clinical management of stable coronary artery disease in patients with type 2 diabetes mellitus: A scientific statement from the american heart association. Circulation. (2020) 141:e779–806. doi: 10.1161/CIR.0000000000000766
49. Investigators S-H, Newby DE, Adamson PD, Berry C, Boon NA, Dweck MR, et al. Coronary CT Angiography and 5-Year Risk of Myocardial Infarction. N Engl J Med. (2018) 379:924–33. doi: 10.1056/NEJMoa1805971
50. Budoff MJ, Mayrhofer T, Ferencik M, Bittner D, Lee KL, Lu MT, et al. Prognostic value of coronary artery calcium in the PROMISE study (Prospective Multicenter Imaging Study for Evaluation of Chest Pain). Circulation. (2017) 136:1993–2005. doi: 10.1161/CIRCULATIONAHA.117.030578
51. Driessen RS, Danad I, Stuijfzand WJ, Raijmakers PG, Schumacher SP, van Diemen PA, et al. Comparison of coronary computed tomography angiography, fractional flow reserve, and perfusion imaging for ischemia diagnosis. J Am Coll Cardiol. (2019) 73:161–73. doi: 10.1016/j.jacc.2018.10.056
52. Maron DJ, Hochman JS, Reynolds HR, Bangalore S, O’Brien SM, Boden WE, et al. Initial invasive or conservative strategy for stable coronary disease. N Engl J Med. (2020) 382:1395–407.
53. Oikonomou EK, Antonopoulos AS, Schottlander D, Marwan M, Mathers C, Tomlins P, et al. Standardized measurement of coronary inflammation using cardiovascular computed tomography: integration in clinical care as a prognostic medical device. Cardiovasc Res. (2021) 117:2677–90. doi: 10.1093/cvr/cvab286
54. Oikonomou EK, Marwan M, Desai MY, Mancio J, Alashi A, Hutt Centeno E, et al. Non-invasive detection of coronary inflammation using computed tomography and prediction of residual cardiovascular risk (the CRISP CT study): a post-hoc analysis of prospective outcome data. Lancet. (2018) 392:929–39. doi: 10.1016/S0140-6736(18)31114-0
55. Oikonomou EK, Desai MY, Marwan M, Kotanidis CP, Antonopoulos AS, Schottlander D, et al. Perivascular fat attenuation index stratifies cardiac risk associated with high-risk plaques in the CRISP-CT Study. J Am Coll Cardiol. (2020) 76:755–7. doi: 10.1016/j.jacc.2020.05.078
56. Ma S, Chen X, Ma Y, Liu H, Zhang J, Xu L, et al. Lesion-Specific peri-coronary fat attenuation index is associated with functional myocardial ischemia defined by abnormal fractional flow reserve. Front Cardiovasc Med. (2021) 8:755295. doi: 10.3389/fcvm.2021.755295
57. Ichikawa K, Miyoshi T, Osawa K, Nakashima M, Miki T, Nishihara T, et al. High pericoronary adipose tissue attenuation on computed tomography angiography predicts cardiovascular events in patients with type 2 diabetes mellitus: post-hoc analysis from a prospective cohort study. Cardiovasc Diabetol. (2022) 21:44. doi: 10.1186/s12933-022-01478-9
58. Dai X, Yu L, Lu Z, Shen C, Tao X, Zhang J. Serial change of perivascular fat attenuation index after statin treatment: Insights from a coronary CT angiography follow-up study. Int J Cardiol. (2020) 319:144–9. doi: 10.1016/j.ijcard.2020.06.008
59. Scholte AJ, Schuijf JD, Kharagjitsingh AV, Dibbets-Schneider P, Stokkel MP, Jukema JW, et al. Different manifestations of coronary artery disease by stress SPECT myocardial perfusion imaging, coronary calcium scoring, and multislice CT coronary angiography in asymptomatic patients with type 2 diabetes mellitus. J Nucl Cardiol. (2008) 15:503–9. doi: 10.1016/j.nuclcard.2008.02.015
60. Scholte AJ, Schuijf JD, Kharagjitsingh AV, Dibbets-Schneider P, Stokkel MP, van der Wall EE, et al. Prevalence and predictors of an abnormal stress myocardial perfusion study in asymptomatic patients with type 2 diabetes mellitus. Eur J Nucl Med Mol Imaging. (2009) 36:567–75. doi: 10.1007/s00259-008-0967-y
61. Chiariello M, Indolfi C. Silent myocardial ischemia in patients with diabetes mellitus. Circulation. (1996) 93:2089–91. doi: 10.1161/01.CIR.93.12.2089
62. Taqueti VR, Di Carli MF. Clinical significance of noninvasive coronary flow reserve assessment in patients with ischemic heart disease. Curr Opin Cardiol. (2016) 31:662–9. doi: 10.1097/HCO.0000000000000339
63. Peterson LR, Herrero P, Schechtman KB, Racette SB, Waggoner AD, Kisrieva-Ware Z, et al. Effect of obesity and insulin resistance on myocardial substrate metabolism and efficiency in young women. Circulation. (2004) 109:2191–6. doi: 10.1161/01.CIR.0000127959.28627.F8
64. Velagaleti RS, Gona P, Sundstrom J, Larson MG, Siwik D, Colucci WS, et al. Relations of biomarkers of extracellular matrix remodeling to incident cardiovascular events and mortality. Arterioscler Thromb Vasc Biol. (2010) 30:2283–8. doi: 10.1161/ATVBAHA.110.208462
65. Turkbey EB, Backlund JY, Genuth S, Jain A, Miao C, Cleary PA, et al. Myocardial structure, function, and scar in patients with type 1 diabetes mellitus. Circulation. (2011) 124:1737–46. doi: 10.1161/CIRCULATIONAHA.111.022327
66. Rubler S, Dlugash J, Yuceoglu YZ, Kumral T, Branwood AW, Grishman A. New type of cardiomyopathy associated with diabetic glomerulosclerosis. Am J Cardiol. (1972) 30:595–602. doi: 10.1016/0002-9149(72)90595-4
67. Hansson L, Zanchetti A, Carruthers SG, Dahlof B, Elmfeldt D, Julius S, et al. Effects of intensive blood-pressure lowering and low-dose aspirin in patients with hypertension: principal results of the Hypertension Optimal Treatment (HOT) randomised trial. HOT Study Group. Lancet. (1998) 351:1755–62. doi: 10.1016/S0140-6736(98)04311-6
68. Bluemke DA, Kronmal RA, Lima JA, Liu K, Olson J, Burke GL, et al. The relationship of left ventricular mass and geometry to incident cardiovascular events: the MESA (Multi-Ethnic Study of Atherosclerosis) study. J Am Coll Cardiol. (2008) 52:2148–55. doi: 10.1016/j.jacc.2008.09.014
69. Korosoglou G, Humpert PM, Ahrens J, Oikonomou D, Osman NF, Gitsioudis G, et al. Left ventricular diastolic function in type 2 diabetes mellitus is associated with myocardial triglyceride content but not with impaired myocardial perfusion reserve. J Magn Reson Imaging. (2012) 35:804–11. doi: 10.1002/jmri.22879
70. Spottiswoode BS, Zhong X, Lorenz CH, Mayosi BM, Meintjes EM, Epstein FH. Motion-guided segmentation for cine DENSE MRI. Med Image Anal. (2009) 13:105–15. doi: 10.1016/j.media.2008.06.016
71. Chamsi-Pasha MA, Zhan Y, Debs D, Shah DJ. CMR in the evaluation of diastolic dysfunction and phenotyping of HFpEF: Current role and future perspectives. JACC Cardiovasc Imaging. (2020) 13(1 Pt 2):283–96. doi: 10.1016/j.jcmg.2019.02.031
72. Kermer J, Traber J, Utz W, Hennig P, Menza M, Jung B, et al. Assessment of diastolic dysfunction: comparison of different cardiovascular magnetic resonance techniques. ESC Heart Fail. (2020) 7:2637–49. doi: 10.1002/ehf2.12846
73. Kwong RY, Sattar H, Wu H, Vorobiof G, Gandla V, Steel K, et al. Incidence and prognostic implication of unrecognized myocardial scar characterized by cardiac magnetic resonance in diabetic patients without clinical evidence of myocardial infarction. Circulation. (2008) 118:1011–20. doi: 10.1161/CIRCULATIONAHA.107.727826
74. Schelbert EB, Cao JJ, Sigurdsson S, Aspelund T, Kellman P, Aletras AH, et al. Prevalence and prognosis of unrecognized myocardial infarction determined by cardiac magnetic resonance in older adults. JAMA. (2012) 308:890–6. doi: 10.1001/2012.jama.11089
75. Ng AC, Auger D, Delgado V, van Elderen SG, Bertini M, Siebelink HM, et al. Association between diffuse myocardial fibrosis by cardiac magnetic resonance contrast-enhanced T(1) mapping and subclinical myocardial dysfunction in diabetic patients: a pilot study. Circ Cardiovasc Imaging. (2012) 5:51–9. doi: 10.1161/CIRCIMAGING.111.965608
76. Jellis C, Wright J, Kennedy D, Sacre J, Jenkins C, Haluska B, et al. Association of imaging markers of myocardial fibrosis with metabolic and functional disturbances in early diabetic cardiomyopathy. Circ Cardiovasc Imaging. (2011) 4:693–702. doi: 10.1161/CIRCIMAGING.111.963587
77. Khan JN, Wilmot EG, Leggate M, Singh A, Yates T, Nimmo M, et al. Subclinical diastolic dysfunction in young adults with Type 2 diabetes mellitus: a multiparametric contrast-enhanced cardiovascular magnetic resonance pilot study assessing potential mechanisms. Eur Heart J Cardiovasc Imaging. (2014) 15:1263–9. doi: 10.1093/ehjci/jeu121
78. Levelt E, Gulsin G, Neubauer S, McCann GP. MECHANISMS IN ENDOCRINOLOGY: Diabetic cardiomyopathy: pathophysiology and potential metabolic interventions state of the art review. Eur J Endocrinol. (2018) 178:R127–39. doi: 10.1530/EJE-17-0724
79. Rijzewijk LJ, van der Meer RW, Smit JW, Diamant M, Bax JJ, Hammer S, et al. Myocardial steatosis is an independent predictor of diastolic dysfunction in type 2 diabetes mellitus. J Am Coll Cardiol. (2008) 52:1793–9. doi: 10.1016/j.jacc.2008.07.062
80. Ng AC, Delgado V, Bertini M, van der Meer RW, Rijzewijk LJ, Hooi Ewe S, et al. Myocardial steatosis and biventricular strain and strain rate imaging in patients with type 2 diabetes mellitus. Circulation. (2010) 122:2538–44. doi: 10.1161/CIRCULATIONAHA.110.955542
81. Hammer S, Snel M, Lamb HJ, Jazet IM, van der Meer RW, Pijl H, et al. Prolonged caloric restriction in obese patients with type 2 diabetes mellitus decreases myocardial triglyceride content and improves myocardial function. J Am Coll Cardiol. (2008) 52:1006–12. doi: 10.1016/j.jacc.2008.04.068
82. Szczepaniak LS, Dobbins RL, Metzger GJ, Sartoni-D’Ambrosia G, Arbique D, Vongpatanasin W, et al. Myocardial triglycerides and systolic function in humans: in vivo evaluation by localized proton spectroscopy and cardiac imaging. Magn Reson Med. (2003) 49:417–23. doi: 10.1002/mrm.10372
83. van der Meer RW, Rijzewijk LJ, Diamant M, Hammer S, Schar M, Bax JJ, et al. The ageing male heart: myocardial triglyceride content as independent predictor of diastolic function. Eur Heart J. (2008) 29:1516–22. doi: 10.1093/eurheartj/ehn207
84. Neubauer S. The failing heart–an engine out of fuel. N Engl J Med. (2007) 356:1140–51. doi: 10.1056/NEJMra063052
85. Levelt E, Rodgers CT, Clarke WT, Mahmod M, Ariga R, Francis JM, et al. Cardiac energetics, oxygenation, and perfusion during increased workload in patients with type 2 diabetes mellitus. Eur Heart J. (2016) 37:3461–9. doi: 10.1093/eurheartj/ehv442
86. Rider OJ, Apps A, Miller J, Lau JYC, Lewis AJM, Peterzan MA, et al. Noninvasive in vivo assessment of cardiac metabolism in the healthy and diabetic human heart using hyperpolarized (13)C MRI. Circ Res. (2020) 126:725–36. doi: 10.1161/CIRCRESAHA.119.316260
87. Sorensen MH, Bojer AS, Pontoppidan JRN, Broadbent DA, Plein S, Madsen PL, et al. Reduced myocardial perfusion reserve in type 2 diabetes is caused by increased perfusion at rest and decreased maximal perfusion during stress. Diabetes Care. (2020) 43:1285–92. doi: 10.2337/dc19-2172
88. Plein S, Radjenovic A, Ridgway JP, Barmby D, Greenwood JP, Ball SG, et al. Coronary artery disease: myocardial perfusion MR imaging with sensitivity encoding versus conventional angiography. Radiology. (2005) 235:423–30. doi: 10.1148/radiol.2352040454
89. Greenwood JP, Maredia N, Younger JF, Brown JM, Nixon J, Everett CC, et al. Cardiovascular magnetic resonance and single-photon emission computed tomography for diagnosis of coronary heart disease (CE-MARC): a prospective trial. Lancet. (2012) 379:453–60. doi: 10.1016/S0140-6736(11)61335-4
90. Heydari B, Juan YH, Liu H, Abbasi S, Shah R, Blankstein R, et al. Stress perfusion cardiac magnetic resonance imaging effectively risk stratifies diabetic patients with suspected myocardial ischemia. Circ Cardiovasc Imaging. (2016) 9:e004136. doi: 10.1161/CIRCIMAGING.115.004136
91. Kellman P, Hansen MS, Nielles-Vallespin S, Nickander J, Themudo R, Ugander M, et al. Myocardial perfusion cardiovascular magnetic resonance: optimized dual sequence and reconstruction for quantification. J Cardiovasc Magn Reson. (2017) 19:43. doi: 10.1186/s12968-017-0355-5
92. Kotecha T, Martinez-Naharro A, Boldrini M, Knight D, Hawkins P, Kalra S, et al. Automated pixel-wise quantitative myocardial perfusion mapping by CMR to detect obstructive coronary artery disease and coronary microvascular dysfunction: Validation against invasive coronary physiology. JACC Cardiovasc Imaging. (2019) 12:1958–69. doi: 10.1016/j.jcmg.2018.12.022
93. Knott KD, Seraphim A, Augusto JB, Xue H, Chacko L, Aung N, et al. The prognostic significance of quantitative myocardial perfusion: An artificial intelligence-based approach using perfusion mapping. Circulation. (2020) 141:1282–91. doi: 10.1161/CIRCULATIONAHA.119.044666
94. Xue H, Davies RH, Brown LAE, Knott KD, Kotecha T, Fontana M, et al. Automated inline analysis of myocardial perfusion MRI with deep learning. Radiol Artif Intell. (2020) 2:e200009. doi: 10.1148/ryai.2020200009
95. Hendel RC, Berman DS, Di Carli MF, Heidenreich PA, Henkin RE, Pellikka PA, et al. ACCF/ASNC/ACR/AHA/ASE/SCCT/SCMR/SNM 2009 Appropriate use criteria for cardiac radionuclide imaging: A Report of the American college of cardiology foundation appropriate use criteria task force, the American society of nuclear cardiology, the american college of radiology, the american heart association, the american society of echocardiography, the society of cardiovascular computed tomography, the society for cardiovascular magnetic resonance, and the society of nuclear medicine. J Am Coll Cardiol. (2009) 53:2201–29.
96. Peterson LR, Gropler RJ. Metabolic and Molecular Imaging of the Diabetic Cardiomyopathy. Circ Res. (2020) 126:1628–45. doi: 10.1161/CIRCRESAHA.120.315899
97. Stevens RJ, Kothari V, Adler AI, Stratton IM. United Kingdom prospective diabetes study g. the ukpds risk engine: a model for the risk of coronary heart disease in type ii diabetes (UKPDS 56). Clin Sci (Lond). (2001) 101:671–9. doi: 10.1042/cs1010671
98. Hippisley-Cox J, Coupland C. Development and validation of QDiabetes-2018 risk prediction algorithm to estimate future risk of type 2 diabetes: cohort study. BMJ. (2017) 359:j5019. doi: 10.1136/bmj.j5019
99. Segar MW, Vaduganathan M, Patel KV, McGuire DK, Butler J, Fonarow GC, et al. Machine learning to predict the risk of incident heart failure hospitalization among patients with diabetes: The WATCH-DM Risk Score. Diabetes Care. (2019) 42:2298–306. doi: 10.2337/dc19-0587
100. Elharram M, Ferreira JP, Huynh T, Ni J, Giannetti N, Verma S, et al. Prediction of heart failure outcomes in patients with type 2 diabetes mellitus: Validation of the Thrombolysis in Myocardial Infarction Risk Score for Heart Failure in Diabetes (TRS-HFDM) in patients in the ACCORD trial. Diabetes Obes Metab. (2021) 23:782–90. doi: 10.1111/dom.14283
Keywords: diabetic heart disease, diabetic cardiomyopathy, heart failure reduced ejection fraction (HFrEF), heart failure preserved ejection fraction (HFpEF), echocardiography, CT coronary angiography, cardiac magnetic resonance (CMR), MRI spectroscopy
Citation: Wamil M, Goncalves M, Rutherford A, Borlotti A and Pellikka PA (2022) Multi-modality cardiac imaging in the management of diabetic heart disease. Front. Cardiovasc. Med. 9:1043711. doi: 10.3389/fcvm.2022.1043711
Received: 13 September 2022; Accepted: 17 October 2022;
Published: 03 November 2022.
Edited by:
Grigorios Korosoglou, GRN-Klinik Weinheim, GermanyReviewed by:
Alexandros Kallifatidis, St. Luke’s Hospital S.A., GreeceCopyright © 2022 Wamil, Goncalves, Rutherford, Borlotti and Pellikka. This is an open-access article distributed under the terms of the Creative Commons Attribution License (CC BY). The use, distribution or reproduction in other forums is permitted, provided the original author(s) and the copyright owner(s) are credited and that the original publication in this journal is cited, in accordance with accepted academic practice. No use, distribution or reproduction is permitted which does not comply with these terms.
*Correspondence: Malgorzata Wamil, d2FtaWwubWFsZ29yemF0YUBtYXlvLmVkdQ==
Disclaimer: All claims expressed in this article are solely those of the authors and do not necessarily represent those of their affiliated organizations, or those of the publisher, the editors and the reviewers. Any product that may be evaluated in this article or claim that may be made by its manufacturer is not guaranteed or endorsed by the publisher.
Research integrity at Frontiers
Learn more about the work of our research integrity team to safeguard the quality of each article we publish.