- 1Department of Pediatric Cardiology, Center for Congenital Heart Diseases, Beatrix Children’s Hospital, University Medical Center Groningen, University of Groningen, Groningen, Netherlands
- 2Central Medical Library, University Medical Center Groningen, University of Groningen, Groningen, Netherlands
Introduction: Currently, risk stratification is the cornerstone of determining treatment strategy for patients with pulmonary arterial hypertension (PAH). Since the 2015 European Society of Cardiology/European Respiratory Society (ESC/ERS) guidelines for the diagnosis and treatment of pulmonary hypertension recommended risk assessment, the number of studies reporting risk stratification has considerably increased. This systematic review aims to report and compare the variables and prognostic value of the various risk stratification models for outcome prediction in adult and pediatric PAH.
Methods: A systematic search with terms related to PAH, pediatric pulmonary hypertension, and risk stratification was performed through databases PubMed, EMBASE, and Web of Science up to June 8, 2022. Observational studies and clinical trials on risk stratification in adult and pediatric PAH were included, excluding case reports/series, guidelines, and reviews. Risk of bias was assessed using the Prediction model Risk Of Bias Assessment Tool. Data on the variables used in the models and the predictive strength of the models given by c-statistic were extracted from eligible studies.
Results: A total of 74 studies were eligible for inclusion, with this review focusing on model development (n = 21), model validation (n = 13), and model enhancement (n = 9). The variables used most often in current risk stratification models were the non-invasive WHO functional class, 6-minute walk distance and BNP/NT-proBNP, and the invasive mean right atrial pressure, cardiac index and mixed venous oxygen saturation. C-statistics of current risk stratification models range from 0.56 to 0.83 in adults and from 0.69 to 0.78 in children (only two studies available). Risk stratification models focusing solely on echocardiographic parameters or biomarkers have also been reported.
Conclusion: Studies reporting risk stratification in pediatric PAH are scarce. This systematic review provides an overview of current data on risk stratification models and its value for guiding treatment strategies in PAH.
Systematic review registration: [https://www.crd.york.ac.uk/prospero/display_record.php?ID=CRD42022316885], identifier [CRD42022316885].
Introduction
Pulmonary hypertension (PH) is a condition defined by an increased pulmonary arterial pressure. Based on pathophysiological mechanisms, clinical presentation, and hemodynamic characteristics, PH can be classified into five main groups: pulmonary arterial hypertension (PAH, group 1), PH due to left heart disease (group 2), PH due to lung disease and/or hypoxia (group 3), PH due to pulmonary artery obstructions (group 4), and PH with unclear and/or multifactorial mechanisms (group 5) (1). Each PH type can be further divided into multiple subgroups. Group 1 PAH is a progressive and eventually fatal pulmonary vascular disease. Occlusion of small pulmonary arteries leads to increased right ventricular afterload, which eventually results in right ventricular failure.
Initially, the only available treatment option for PAH was calcium channel blockers. However, these calcium channel blockers showed only beneficial to a small subset of patients with a response to acute pulmonary vasodilator testing during right heart catheterization (RHC) (2). Over the last decades, various PAH-targeted therapies have become available, such as endothelin receptor antagonists, phosphodiesterase type 5 inhibitors, guanylate cyclase stimulators, prostacyclin analogues, and selective prostacyclin receptor agonists (3). With the availability of these drugs, the treatment of PAH was initially focused on preventing disease progression and prolonging patient survival. When a patient deteriorated on initial therapy, therapy was escalated to double, triple, or maximal combination therapies. These strategies led to improved patient survival after which the focus of treatment strategies started shifting toward clinical improvement. According to current treatment algorithms, treatment decisions are recommended to be based on the assessment of mortality risk of the individual patient, estimated by using clinical prognosticators, both at initiation of therapy as well as for evaluating treatment response (3–5). Therefore, adequate prediction of risk of mortality is pivotal in the treatment of PAH patients.
To estimate patient risk status, various risk equations and risk stratification models have been established. Initially, risk equations were developed to estimate patient outcome by expressing their chances of survival in a percentage. The first time survival was estimated for PAH patients was in 1991 when D’Alonzo et al. (6) developed the NIH (National Institute of Health registry) risk equation, based on the mean pulmonary arterial pressure (mPAP), mean right atrial pressure (mRAP), and cardiac index (CI). Since then other risk equations have been developed, such as the French PAH registry equation (7), the PHC (Pulmonary Hypertension Connection) survival equation (8), and the REVEAL (Registry to Evaluate Early and Long-term Pulmonary Arterial Hypertension Disease Management) risk equation (9). From this original REVEAL risk equation, consisting of nineteen etiologic factors and parameters, the first risk stratification model was derived (10).
Currently, treatment strategies are guided by risk stratification, as proposed by the consecutive European Society of Cardiology/European Respiratory Society (ESC/ERS) guidelines for the diagnosis and treatment of PH (3, 4) and the American College of Cardiology Foundation/American Heart Association (ACCF/AHA) expert consensus document on PH (11). According to these strategies, patients are categorized as having low, intermediate, or high risk for mortality, where the aim is to achieve and maintain a low-risk status. The estimated risk is based on multiple clinical, hemodynamic, and echocardiographic parameters with their own cut-off values for each risk category. A risk stratification guided treatment strategy has also been proposed for children with PAH during the World Symposium on Pulmonary Hypertension (WSPH), using the binary strata low and high risk (12, 13).
The aim of this systematic review is to provide an overview of the current risk stratification models in adult and pediatric PAH. With the growing number of risk stratification models it is crucial to assess the reliability and accuracy of these models, especially since their use in daily practice is advocated. Therefore, the two research questions addressed in this systematic review are: (1) which variables are used for risk stratification models in PAH and (2) what is the prognostic value of risk stratification models for transplant-free survival or all-cause mortality?
Methods
This review is reported according to the Preferred Reporting Items for Systematic Reviews and Meta-Analysis for Scoping Reviews (PRISMA) (14). The objectives, inclusion criteria and methods adopted in this systematic review were specified and documented in advance (Prospero registration number: CRD42022316885).
Eligibility criteria
Clinical trials and observational studies focused on risk stratification models both in adult (age ≥ 18 years) and pediatric (age < 18 years) PAH patients were eligible for inclusion. Pediatric patients with PH due to lung disease were also considered eligible for inclusion because of the pathological crossover between PAH and the abnormal pulmonary vascular development, seen in developmental lung diseases such as bronchopulmonary dysplasia and congenital diaphragmatic hernia. In these studies the diagnosis had to be confirmed by RHC, or echocardiography in infants with developmental lung disease, and meet the hemodynamic definitions (1). Additionally, the risk stratification model was considered a model only if it comprised at least three variables. Results and conclusions had to be supported by appropriate statistical methods with endpoints defined as transplant-free survival or all-cause mortality. Furthermore, studies had to be written in English.
Studies reporting risk stratification models in adult patients with PH group 2, 3, 4, and 5 according to the Nice 2018 classification (1), and pediatric patients with PH group 2, 4, and 5 were excluded, as well as case reports, case series, guidelines, and reviews. If less than three variables were used for risk stratification models or endpoints other than transplant-free survival or all-cause mortality, studies were excluded as “no risk stratification model” or “not eligible endpoint,” respectively. Studies not meeting the inclusion criteria and not fitting any of the above mentioned exclusion reasons were excluded as “other.” In this review, survival or risk equations were not considered as risk stratification models.
Information sources and search strategy
Systematic literature searches were conducted in the electronic databases MEDLINE (PubMed), Embase (Elsevier), and Web of Science (Clarivate). The search strategies were developed in collaboration with an information specialist (SW). The structure of the search strategies is based on two concepts: (1) PAH, pediatric PH and (2) risk stratification, risk tooling, prediction modeling. For each concept a search block was developed based on index terms and free text words including synonyms and related terms. No time or language restrictions were applied. The search strategies were initially run at March 3, 2022 and updated at June 8, 2022. The full search strategies can be found in Supplementary Table 1.
Managing references and selection process
The results of the database searches were exported to the reference management program EndNote, version 20. In EndNote, duplicate items were determined and removed following the steps described by Bramer et al. (15). The de-duplicated results were exported to the screening program Rayyan.
Two researchers independently performed the screening in Rayyan in two steps. In the title-abstract screening, articles were excluded that were clearly not relevant. Potentially relevant articles and articles with insufficient information in the titles or abstract selected by at least one of the researchers were selected for the full-text screening. In the full-text screening, the two researchers independently judged if the selection criteria were met. Disagreements in decisions between the screeners were solved by a third reviewer. Finally, articles that met the criteria, as agreed by the researchers, were included and divided into four classes judged on the primary aim of the article: (1) model development, (2) model validation, (3) model enhancement, and (4) serial risk stratification. In accordance with the aims of the systematic review, the authors focused on the studies belonging to class 1, 2, and 3. Studies in class 4 focused on risk stratification at follow-up and/or changes in risk score or stratum between baseline and follow-up, whether or not under the influence of intervention, and were hence disregarded from the current review.
Data collection
Data was extracted from the included studies using a standardized data extraction form. Extracted data included: study setting, population demographics and baseline characteristics, variables used in the risk stratification model including cut-off points and defined endpoint, statistical methodology, and the prognostic value of the model.
Analysis
To present an overview of the variables used in risk stratification models, multiple tables were produced. Each table reports the model name or basis, the used definition of risk, the number of risk strata, the number of variables, and specifies which variables are used for the model. Separate tables were created for the renowned risk stratification models (containing both development and validation), the lesser studied models, model enhancement, and pediatric risk stratification strategies.
For the evaluation of the prognostic value of the risk stratification models, the reported c-statistic was used. The c-statistic is equivalent to the area under the receiver operating characteristic curve (AUROC) and is a measure of the discriminatory ability. It can be interpreted as the probability that a patient who died had a higher predicted probability of death than a patient who survived. A c-statistic of 1.0 shows a perfect prediction, whereas a c-statistic of 0.5 is indicative of poor prediction and the model is no better than chance. Hence, the model with the higher c-statistic (or greater AUROC) is better at discriminating between survival and death (17).
Risk of bias
Risk of bias (ROB) was assessed using the Prediction model Risk Of Bias Assessment Tool (PROBAST) for every study and in case of studies including multiple models, separately for the different risk stratification models (16). This tool consists of four domains - participants, predictors, outcome, and analysis–with a total of 20 signaling questions to assist in assessing ROB. These questions can be answered as (probably) yes, (probably) no, or no information, with “no” indicating potential bias. For model development studies, the development signaling questions were answered, and for validation studies the validation questions. In the case of studies reporting both the development of a model and the validation of this model or other models, both the development and validation signaling questions were answered for each model separately. For model enhancement studies, the development questions were acknowledged, as well as the validation questions when the original model was also validated. Besides ROB, the applicability of the model was evaluated to determine the relevance of the participants, predictors, and outcome to the research question. ROB and applicability assessment was performed by one researcher, but when in doubt, a second researcher was consulted.
Results
Identified studies
In Figure 1, the PRISMA flowchart for the identification of studies is shown. A total of 2,395 records were identified from the databases. After duplicate removal, 1,539 studies remained for abstract screening of which 1,385 were excluded during abstract screening. Of the 154 full-text screened studies, 80 were excluded (Supplementary Table 2). The remaining 74 studies were considered eligible for inclusion (Supplementary Table 3), of which two studies involved pediatric PAH patients. No studies concerning risk stratification in pediatric PH due to lung disease were retrieved, as such our results focus on RHC confirmed PAH only. The 31 studies concerning serial risk stratification were disregarded, since the current study focusses solely on model development (n = 21), validation (n = 13), and enhancement (n = 9) of risk stratification models, resulting in a total of 43 studies to be discussed in this review. The main characteristics of these 43 studies are presented in Tables 1–3 for respective development, validation, and enhancement studies.
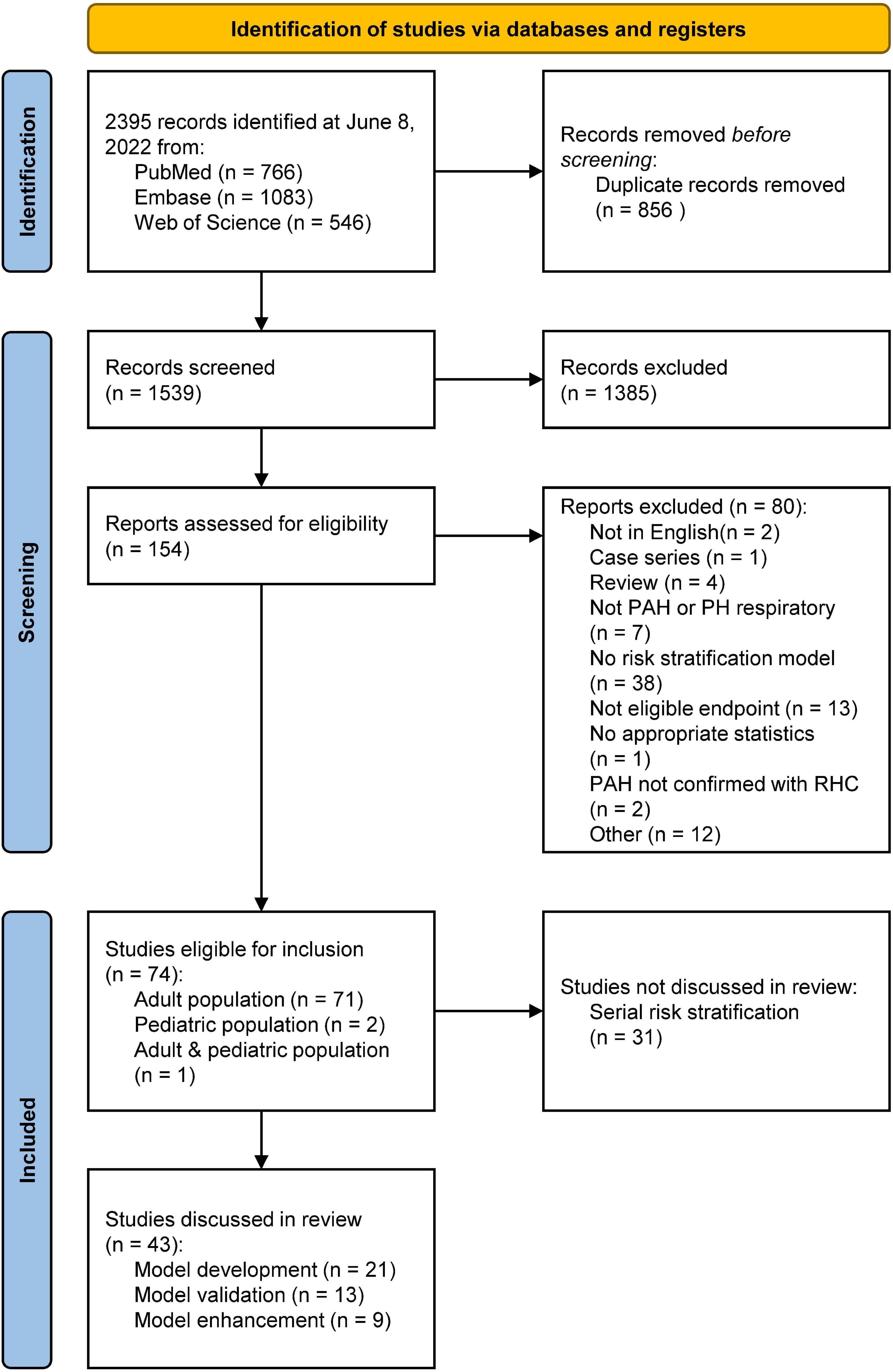
Figure 1. PRISMA flowchart showing the study selection. PAH, pulmonary arterial hypertension; PH, pulmonary hypertension; RHC, right heart catheterization. Other reasons included editorials, retracted articles, and commentaries.
Variables in risk stratification
We have identified multiple risk stratification models, such as the REVEAL risk calculator and the ESC/ERS 2015 guidelines-based COMPERA, SPARH, FPRH invasive and non-invasive models, and other abbreviated versions of the ESC/ERS 2015 guidelines. In Table 4 an overview of the variables used for these risk stratification models is given, along with the total number of variables used in each model, the risk definition, and the number of strata.
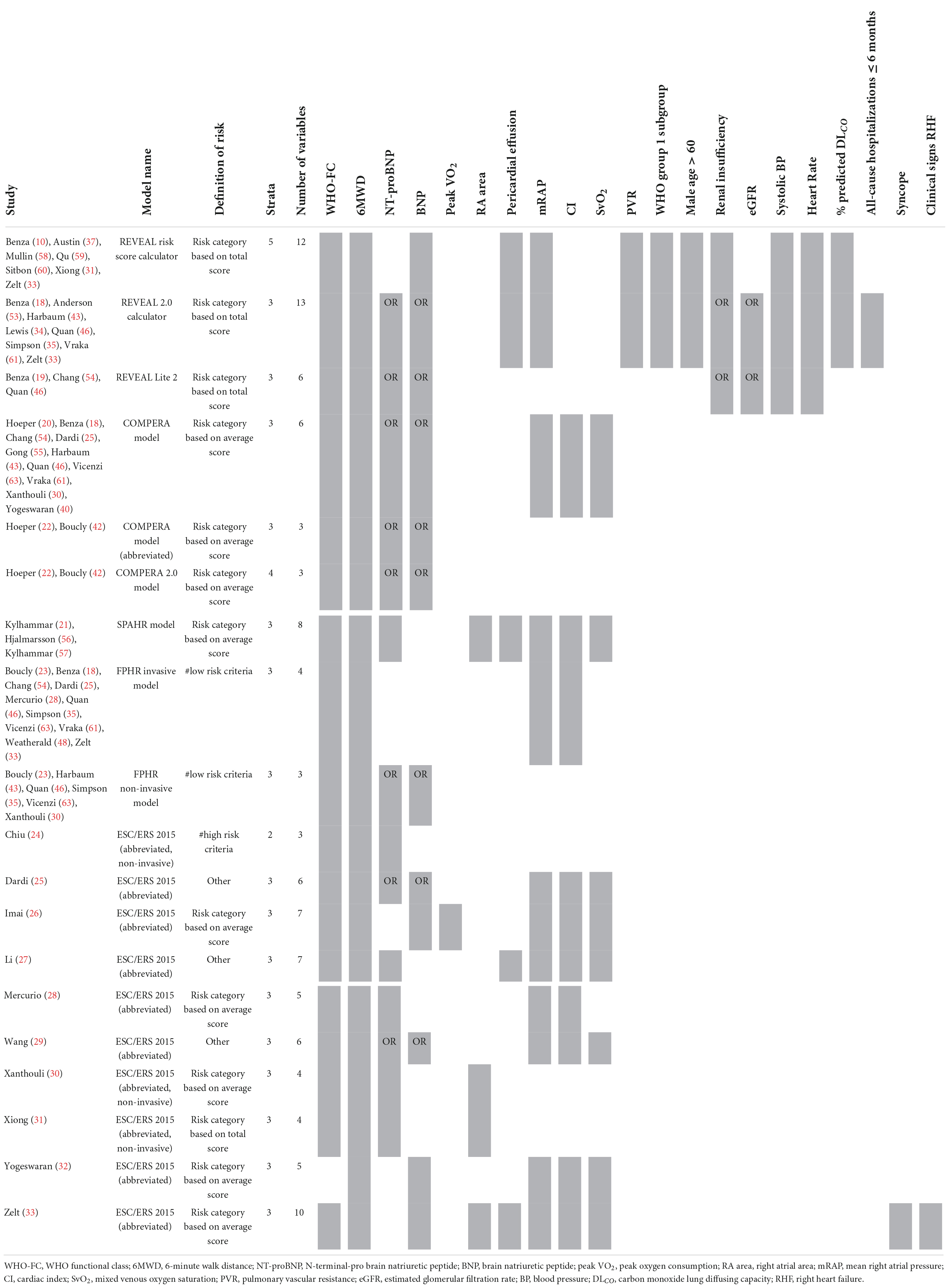
Table 4. Variables used in REVEAL and ESC/ERS 2015 guideline-based risk stratification models: development and validation studies.
The first REVEAL risk calculator was developed by Benza et al. (10) in 2012 and consisted of twelve variables: WHO Functional Class (WHO-FC), 6-minute walk distance (6MWD), N-terminal-pro brain natriuretic peptide (NT-proBNP, or brain natriuretic peptide–BNP), pericardial effusion, mRAP, pulmonary vascular resistance (PVR), WHO group 1 subgroup, male older than 60 years of age, renal insufficiency, systolic blood pressure (SBP), heart rate (HR), and percentage predicted carbon monoxide lung diffusing capacity (DLCO). Points are assigned to every variable, with its weight based on the results of the multivariable Cox proportional hazard model. In 2019, Benza et al. (18) updated some of the cut-off values of the variables and added an extra variable to the model, all-cause hospitalizations within the last 6 months, resulting in the REVEAL 2.0 calculator. The REVEAL Lite 2, a non-invasive, abbreviated version of the REVEAL 2.0 calculator, was published by Benza et al. (19) in 2021.
Many different methods have been developed based on the risk stratification as recommended by the ESC/ERS 2015 guidelines. In 2017, Hoeper et al. (20) reported the COMPERA (Comparative, Prospective Registry of Newly Initiated Therapies for Pulmonary Hypertension) model which uses six variables: WHO-FC, 6MWD, NT-proBNP (or BNP), mRAP, CI, and mixed venous oxygen saturation (SvO2). Each variable is assigned a grade 1 (low risk), 2 (intermediate risk), or 3 (high risk) according to the cut-off values derived from the ESC/ERS 2015 guidelines. To determine the risk class, the sum of these grades is divided by the number of available variables and rounded to the nearest integer. Kylhammar et al. (21) created a similar method with SPAHR (Swedish PAH Register), but included two more variables: right atrial (RA) area and pericardial effusion. Since many patients were stratified as having intermediate risk, Hoeper et al. (22) created the abbreviated version COMPERA 2.0 model in 2021, where the intermediate risk stratum is split into intermediate-low and intermediate-high risk, resulting in a four-strata model consisting of three variables: WHO-FC, 6MWD, and NT-proBNP. The FPHR (French pulmonary hypertension registry) invasive and non-invasive method, published by Boucly et al. (23) in 2017, uses the number of low-risk criteria to estimate the mortality risk. WHO-FC, 6MWD, mRAP, and CI are used in the invasive method, whereas WHO-FC, 6MWD, and NT-proBNP (or BNP) are used in the non-invasive method. A major limitation of this method is that it cannot be applied if one of the variables is missing. Besides COMPERA, SPAHR, and FPHR models, other abbreviated versions of the ESC/ERS 2015 guidelines were reported (24–33). The variables used in these models are also shown in Table 4. From this table it can be observed that most often used variables in risk stratification models are WHO-FC, 6MWD, NT-proBNP, mRAP, CI, and SvO2.
The enhancement of above mentioned risk stratification models has been explored by several studies by adding one or more imaging or biomarker variables to the models, such as the right ventricular end-systolic volume index (34), estimated glomerular filtration rate (eGFR) (33), or endostatin (35). To enhance the performance of the REVEAL 2.0 calculator, Kanwar et al. (36) produced a tree-augmented naïve Bayes version using the same variables and cut-off values as the REVEAL 2.0 calculator. In Table 5 an overview of the model enhancement studies with the variables is presented.
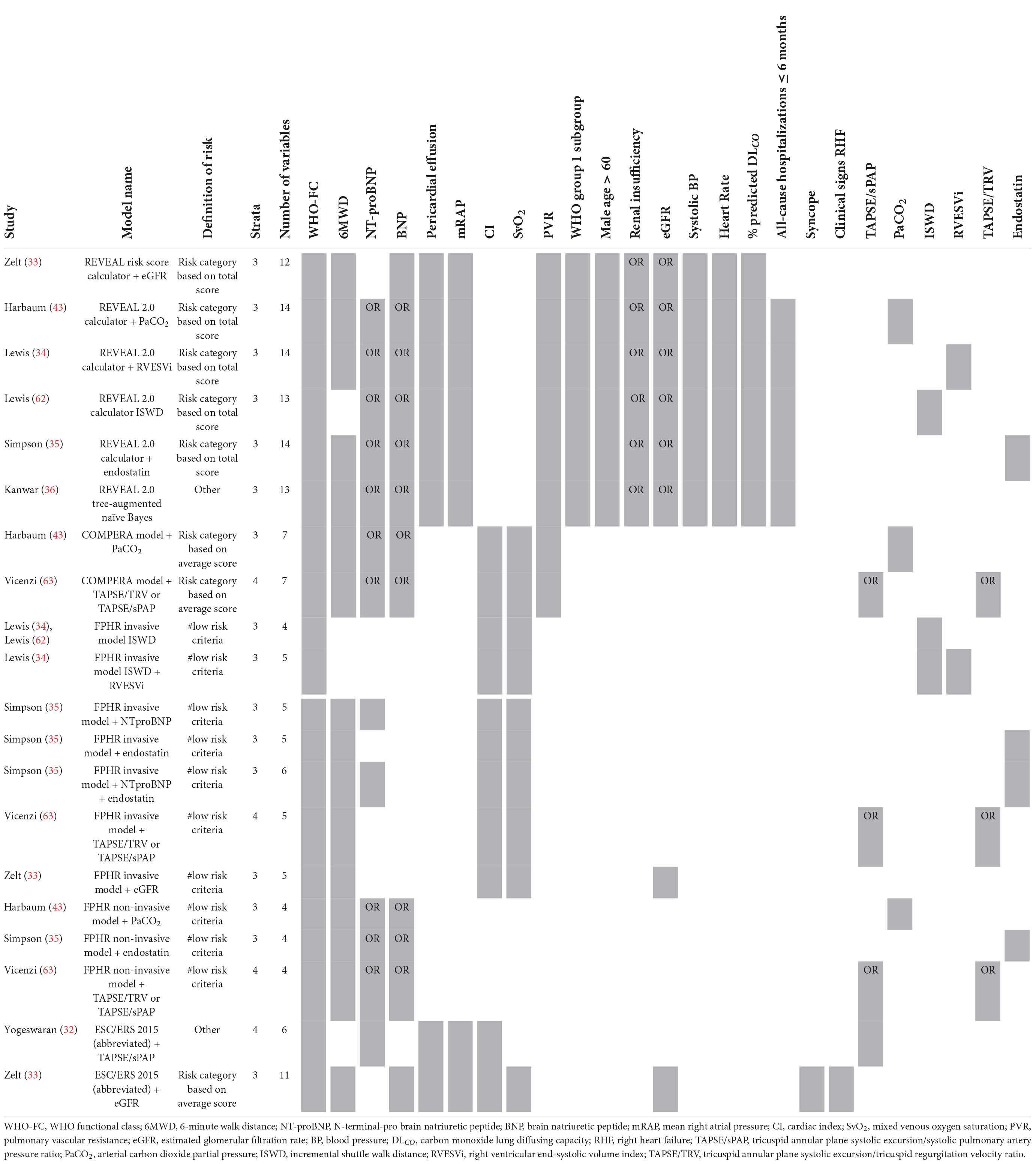
Table 5. Variables used in REVEAL and ESC/ERS 2015 guideline-based risk stratification models: enhancement studies.
Additionally, others have tried to create risk stratification models based solely on echocardiographic parameters (37, 38) or biomarkers (39, 40) (Table 6). For example, Ghio et al. (38) used the echocardiographic parameters tricuspid annular plane systolic excursion (TAPSE), degree of tricuspid regurgitation (TR) and a marker of systemic venous congestion represented by inferior vena cava diameter. Yogeswaran et al. (40) developed a model with the biomarkers ƴ-glutamyl transferase (GGT), aspartate aminotransferase/alanine aminotransferase (AST/ALT) ratio, and neutrophil-to-lymphocyte ratio (NLR). A different approach for developing a risk stratification model was shown by Haddad et al. (41). They attempted to model the data architecture by creating a network graph. This graph shows the connectivity of every parameter with the other parameters and identified NT-proBNP as the most central (important) parameter.
Prognostic value of risk stratification models
The prognostic value of the REVEAL risk scores in different studies and populations is shown in Figure 2 by a forest plot of the c-statistic with its 95% confidence interval (95% CI). The c-statistic was found to range from 0.70 to 0.75 for the REVEAL risk score calculator and from 0.65 to 0.74 for the REVEAL 2.0 calculator. REVEAL Lite 2 had a c-statistic of 0.70.
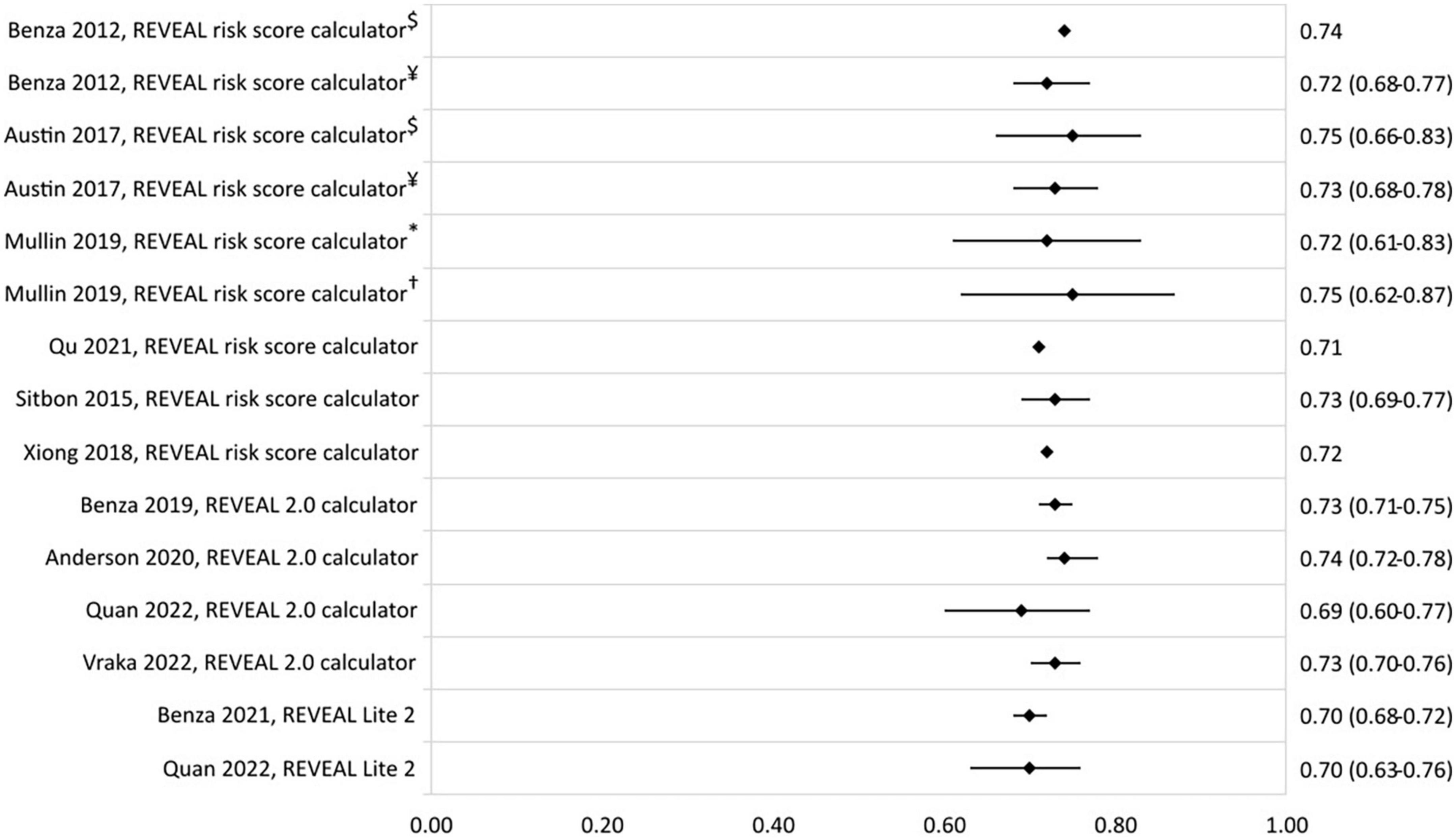
Figure 2. C-statistic (95% CI) of the development and validation of REVEAL risk stratification models. $Derivation cohort, ¥validation cohort, *JHPHP, and †PHAROS.
In Figure 3, the c-statistics of the risk stratification models based on the ESC/ERS 2015 guidelines are presented. C-statistic ranged from 0.62 to 0.77 for the COMPERA model, and from 0.56 to 0.73 and 0.39 to 0.69 for the FPHR invasive and non-invasive method, respectively. The COMPERA 2.0 model showed a c-statistic of 0.67 in a validation study by Boucly et al. (42). Other abbreviated versions of the ESC/ERS 2015 guidelines c-statistic ranged from 0.60 to 0.73. Highest c-statistic was reported by Xiong et al. (31) with a model consisting of the non-invasive variables WHO-FC, 6MWD, NT-proBNP, and RA area.
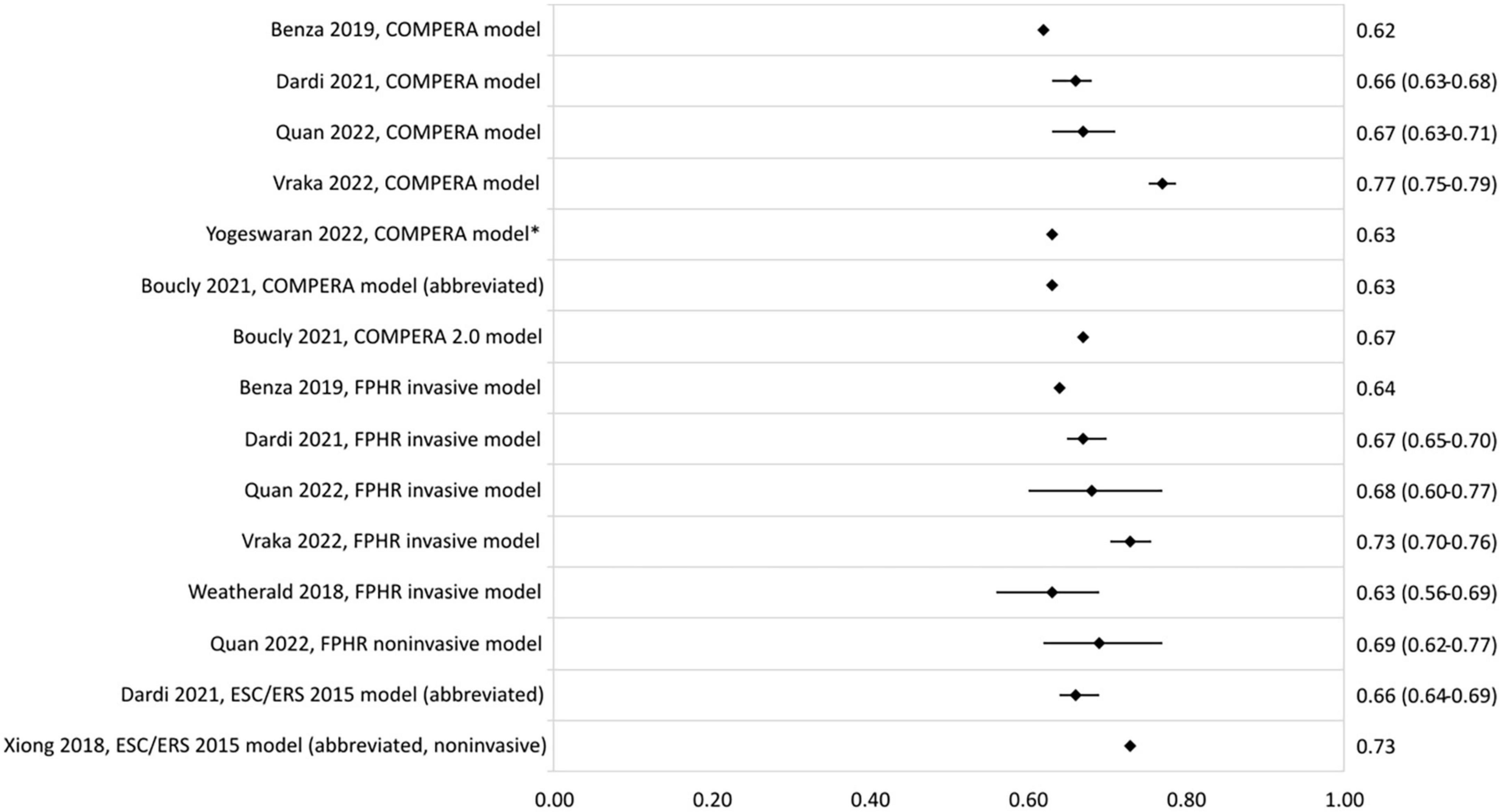
Figure 3. C-statistic (95% CI) of the development and validation of ESC/ERS 2015 guideline-based models. *Cohort includes PAH and chronic thromboembolic PH patients.
Several enhancement studies were found to have an increase in c-statistic upon the addition of an imaging or serum biomarker to a previously described model (Figure 4). Lewis et al. reported an increase in c-statistic of the REVEAL 2.0 calculator from 0.74 (0.65–0.83) to 0.78 (0.70–0.87) upon addition of the right ventricular end-systolic volume index. Harbaum et al. (43) increased the c-statistic of the COMPERA model from 0.62 (0.52–0.73) to 0.67 (0.57–0.79) by adding arterial carbon dioxide partial pressure to the model. Addition of biomarkers NT-proBNP and endostatin to the FPHR invasive method was shown to increase the c-statistic from 0.62 to 0.72 (35), and endostatin also increased the c-statistic of the FPHR non-invasive method from 0.68 to 0.71 (35).
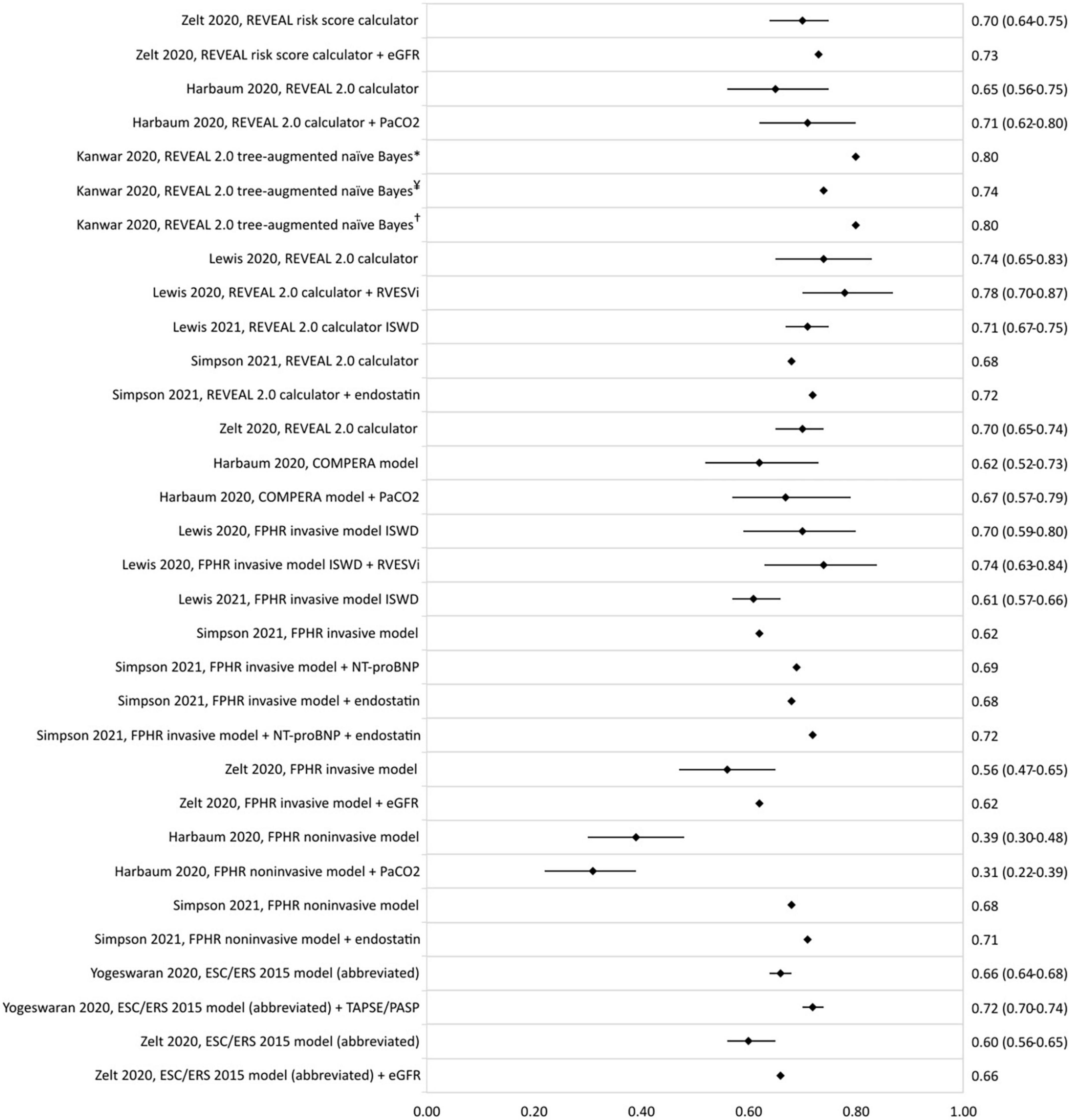
Figure 4. C-statistic (95% CI) of the enhancement of REVEAL and ESC/ERS 2015 guideline-based risk stratification models, as well as the c-statistic of the original model in the same population. eGFR, estimated glomerular filtration rate; PaCO2, arterial carbon dioxide partial pressure; RVESVi, right ventricular end-systolic volume index; ISWD, incremental shuttle walk distance; NT-proBNP, N-terminal-pro brain natriuretic peptide; TAPSE/PASP, tricuspid annular plane systolic excursion/systolic pulmonary artery pressure ratio. *REVEAL cohort, ¥COMPERA cohort, and †PHSANZ cohort.
The c-statistics of other developed risk stratification models are presented in Figure 5. A model using the plasma proteome had c-statistics of 0.82 (0.77–0.88) and 0.74 (0.63–0.85) in the derivation and validation cohort, respectively (39). The eigenvector centrality model developed by Haddad et al. (41) had a c-statistic of 0.81 (0.77–0.85).
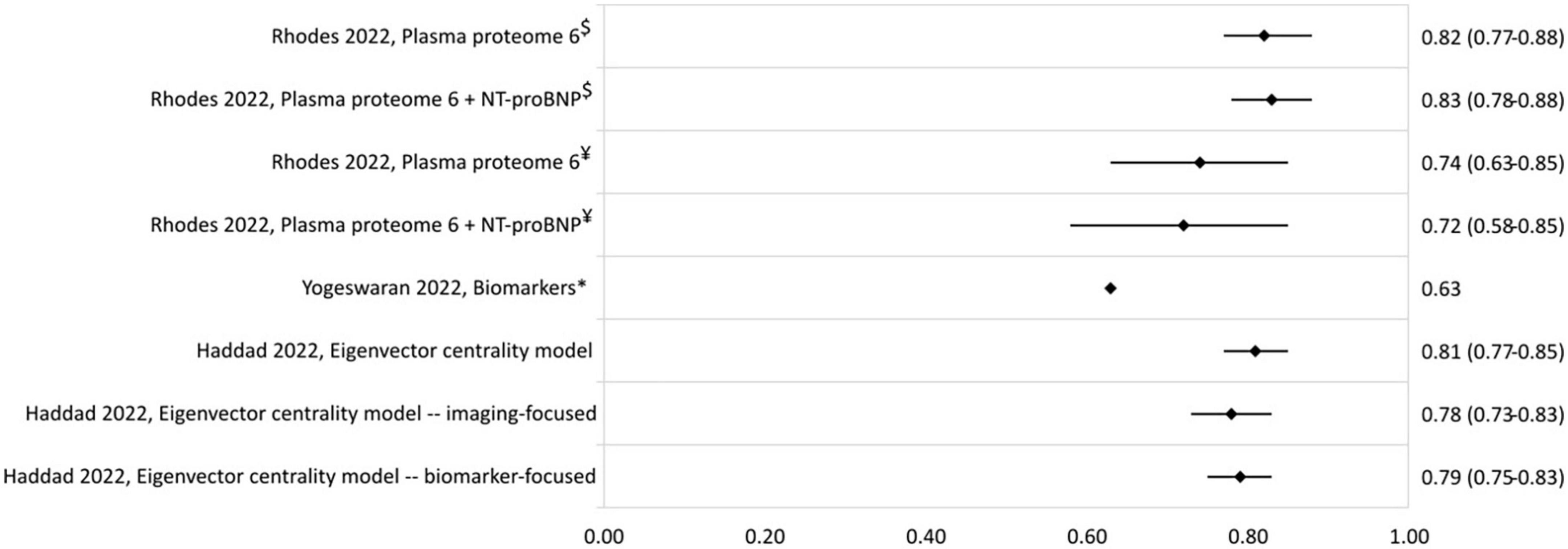
Figure 5. C-statistic (95% CI) of biomarker and eigenvector centrality risk stratification models. NT-proBNP, N-terminal-pro brain natriuretic peptide. $Derivation cohort, ¥validation cohort, *cohort includes PAH and chronic thromboembolic PH patients.
Risk stratification in pediatric pulmonary arterial hypertension
Only two studies reporting risk stratification in pediatric PAH were found eligible for inclusion. Griffiths et al. (44) applied the REVEAL 2.0 calculator in 182 children with a median age (interquartile range–IQR) of 13 (8–17) years. They used the variables and cut-off values from the REVEAL 2.0 calculator, except for renal insufficiency, and categorized the patients according to the five risk strata from the original REVEAL risk score calculator (Table 7). The reported c-statistic (Figure 6) of the model in this pediatric population was 0.69 (0.56–0.83). Addition of soluble suppressor of tumorigenicity-2 increased the c-statistic to 0.78 (0.65–0.89). The other pediatric PAH study was by Haarman et al. (45) and described the development of two risk stratification models in 58 children with a median age of 6.8 (2.2–13.4) years. The models were based on the variables and cut-off values recommended by the WSPH 2013 pediatric task force (12) with the addition of two variables from the ESC/ERS 2015 guideline (3) and risk was defined as the number of low risk criteria. The first model consisted of the following variables: WHO-FC, NT-proBNP, mRAP, CI, TAPSE, syncope, height, body mass index, mPAP/mean systemic arterial pressure ratio, indexed PVR, acute vasoreactivity, SvO2, and RA area. The second model contained only the non-invasive variables of the first model (Table 7). C-statistic of the full model was 0.78 (0.64–0.92), and remained almost similar in the non-invasive version to 0.76 (0.62–0.90) (Figure 6).
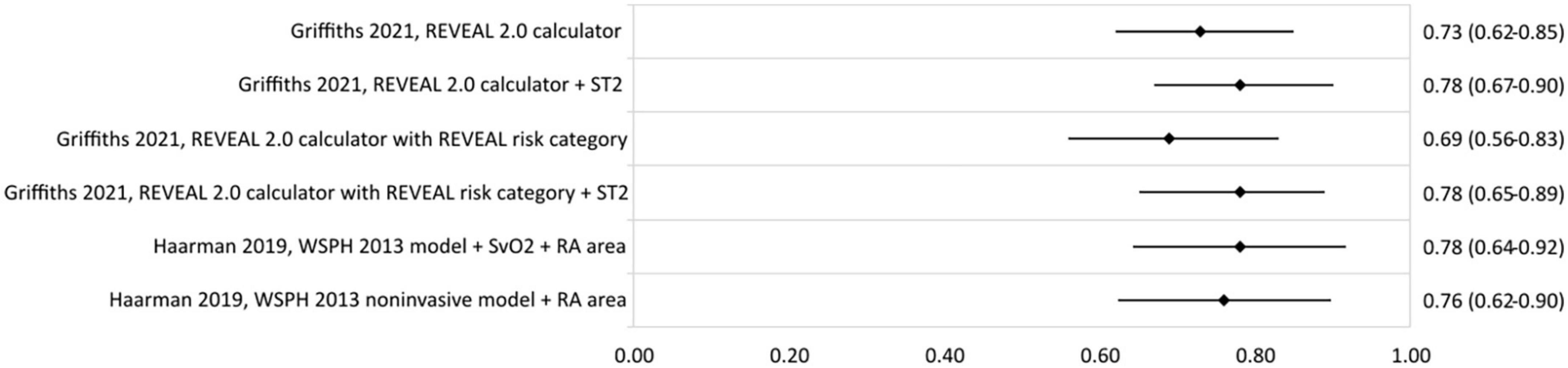
Figure 6. C-statistic (95% CI) of risk stratification models used in pediatric PAH. ST2, soluble suppressor of tumorigenicity-2; SvO2, mixed venous oxygen saturation; RA area, right atrial area.
Risk of bias
The PROBAST results of the ROB analysis are presented in Table 8. The ROB for the domains participants, predictors, and outcome was low for almost every study. However, many studies were judged as having a high ROB based on the described analysis, causing an overall high ROB for nearly all studies. To differentiate between studies scoring poorly on one or two signaling questions and those failing on nearly all aspects of the analysis, the judgment is marked with one, two or three asterixis. These asterixis correspond to respective one to three, four to six, and seven or more negatively answered questions (“no” or “no information”) out of nine for development studies and out of six for validation studies. There was low concern regarding applicability of models for participants, predictors, and outcome.
Discussion
In this systematic review we identified twenty different risk stratification models that have been proposed for adult PAH and only two for pediatric PAH. The REVEAL risk calculators are the most frequently validated models in literature, followed by the COMPERA model and FPHR invasive and non-invasive models. For the enhancement of existing risk stratification models, the FPHR invasive method and REVEAL 2.0 calculator have been studied most frequently. The non-invasive WHO-FC, 6MWD, and BNP/NT-proBNP, and the invasive mRAP, CI, and SvO2 were found to be the variables that are most often used for the risk stratification of PAH. Reported c-statistics representing model predictive strength range from 0.39 to 0.77. Studies enhancing models by adding new variables report improvement of model strength.
Most risk stratification models include the non-invasive variables of WHO-FC, 6MWD, and BNP/NT-proBNP. The inclusion of these parameters in risk stratification may be due to the extensive studies on the prognostic value of these parameters, and stresses their important prognostic abilities in adult PAH patients. Based on the comparable predictive strength of non-invasive models and models including invasive parameters reported in three studies (19, 45, 46), a fully non-invasive risk stratification may be feasible. However, data supporting fully non-invasive risk stratification models are still scarce. Therefore, it may still be too early to set aside the invasive parameters included in most risk stratification models.
In the identified risk stratification models, different methods are used to combine cut-off scores of individual variables to determine the overall risk status. The three main definitions of risk are (1) the number of low risk criteria, (2) risk category based on an average score, and (3) risk category based on the total sum of the score. Furthermore, the risk stratification models can use weighted or unweighted variables. Risk stratification models using the number of low risk criteria (e.g., FPHR invasive and non-invasive method) or an average score (e.g., COMPERA and SPAHR models) do not take the weight of the variables into account for their determination of risk. This may lead to an underestimation or overestimation of patient risk. The REVEAL risk calculators were the only models found to consider the weighted values for individual variables in the calculation of risk. Variables that showed at least a twofold increase in hazard for mortality according to the multivariable Cox proportional hazard model were assigned two points, whereas variables with lower hazard received one point (10). This inclusion of variable weight in the risk estimation does appear to have an effect on the discriminatory ability of the model. C-statistics found in studies using the REVEAL risk calculators were, in general for most studies, higher (0.70–0.75) than those reported for COMPERA and FPHR models (0.62–0.69). These findings may favor the use of weighted risk scores instead of averages or number of low or high risk criteria in further development of risk stratification models.
Overall, the c-statistic of most studies was found to range between 0.6 and 0.8. Considering that a c-statistic of 0.5 indicates a poor prediction and 1.0 a perfect prediction, we may consider the current risk stratification models to have a moderate predictive ability. Whether or not this is sufficient enough to rely on for optimal treatment strategies can be debated. In the recently released 2022 ESC/ERS guidelines for the diagnosis and treatment of PH (4), the four-strata COMPERA 2.0 model of Hoeper et al. (22) using WHO-FC, 6MWD, and BNP/NT-proBNP is recommended for risk stratification at follow-up to guide treatment strategies in adult patients with PAH. The c-statistic for 1 year mortality of this four-strata model was reported to be 0.67 at baseline and 0.73 at follow-up, in an external validation study by Boucly et al. (42). According to this, the authors would advocate that we should strive for improving current risk stratification models.
A possible approach for improving risk stratification models may be the addition of new parameters. The increase of the c-statistic in all enhancement studies, except for the addition of arterial carbon dioxide partial pressure to the FPHR non-invasive model (43), shows that the predictive strength of risk stratification models can be improved by adding imaging or serum biomarkers. Of all the enhancement studies, the addition of the right ventricular end-systolic volume index seems most promising (34). Prospective and external validation studies are needed to further establish the predictive value of enhanced models.
Furthermore, the use of risk stratification is not restricted to estimate risk at diagnosis or initiation of therapy. Also serial risk stratification every 3–6 months is proposed in order to use follow-up risk estimates to evaluate treatment response and to identify the need to escalate therapy (47). Recent reports show that risk stratifications may have a better discrimination of outcome at first follow-up RHC compared to baseline (48), and that changes in risk status are predictive of survival (49). Moreover, the addition of serial changes in NT-proBNP or right heart reverse remodeling (a combination of three echocardiographic parameters) increased the c-statistic of respective the eigenvector centrality model of Haddad et al. (41) (0.81–0.85) and the REVEAL 2.0 calculator (0.69–0.87) (50). As such the strength of risk stratification models may lie in serial assessments.
Data regarding the use of risk stratification models in pediatric PAH is extremely scarce. In this review, only two pediatric PAH studies were found, one based on the variables recommended by the WSPH 2013 pediatric task force and one based on the REVEAL 2.0 calculator. Nonetheless, risk stratification to guide treatment strategies is currently recommended also in the pediatric population. The updated guideline of the European Pediatric Pulmonary Vascular Disease for the diagnosis and treatment of pediatric pulmonary hypertension presents a risk score sheet for pediatric PH based solely on expert opinion (51). However, no validation yet exists and in the guideline it is stated that it is not clear which cut-offs should be used for the risk stratification variables. For this reason, Haarman et al. (45) in their study used cut-off values derived from separate prognosticator studies in children with PAH. Considering the reference class problem, which dictates that the prediction for the individual patient depends on the reference class the patient is assigned to, it is recommended to develop a risk stratification model with variable cut-offs and weights designed specifically for the pediatric population.
Nearly all studies included in this systematic review were judged to have a high ROB based on their analysis. This can be explained with closer observation of the analysis domain of PROBAST (16), the tool that was used to rate ROB. First, according to PROBAST, the number of events (death or death + transplant) per variable should be higher than 10 for development studies, and for validation studies at least 100 participants with the outcome are required. These criteria were met by approximately only half of the included adult studies. Since pediatric PAH is a rare disease, none of the included pediatric studies met the criteria for the number of participants, which shows the limitation of the applicability of PROBAST in a rare disease. Secondly, if continuous variables were dichotomized or categorized for the development of a model, according to PROBAST the model could have a high ROB. However, categorization forms the basis of risk stratification and thus many model development were rated to have a high ROB. For validation studies categorization of continuous variables was allowed if the cut-offs were similar to the original model. Third aspect in PROBAST is the inclusion of all enrolled participants in the analysis and the appropriate handling of missing data, since excluding patients with missing data may cause selection bias. Besides, selection bias is a reasonable risk of registry studies since there are nearly always missing data due to the data not being collected according to a protocol or for the research question at hand. Fourth, multiple studies did not report c-statistic or AUROC, where PROBAST demands reporting of both calibration and discrimination measures. Information on model overfitting and optimism in model performance was also often not described. Finally, the weights of the variables in the final model had to correspond to the results from the reported multivariable analysis. As discussed earlier, models defining risk by the number of low risk criteria or based on an average score do not take the weight of a variable into account, and thus these studies were also at high ROB.
This study has several limitations. Not all included studies reported a c-statistic, which may have caused a bias in the judgment of the prognostic value of the models. The patients included in studies performed more recently were receiving treatment according to the risk stratification-based treatment algorithms. This may have influenced the outcome of those patients, which could have affected the prognostic value of risk stratification models of these studies. No meta-analysis was performed limiting direct conclusions on which model performs best. In order to keep focus, the studies concerning serial risk stratification were disregarded, limiting the ability to discuss the value of serial follow-up risk stratification.
For future purposes, it is recommended to perform prospective validation studies of the risk stratification models since now only retrospective studies of risk stratification exist. Studies developing new models or validating existing models should consider including both calibration and discrimination measures as both are needed to thoroughly describe the performance of the model. Furthermore, an individual patient data systematic review is recommended to define which risk stratification model has the best performance.
Conclusion
This systematic review contributes to our current knowledge on risk stratification in PAH and emphasizes the very limited presence of studies reporting risk stratification in pediatric PAH. The variables found to be used the most frequently in risk stratification models are WHO-FC, 6MWD, NT-proBNP (or BPN), mRAP, CI, and SvO2. The prognostic value of current risk stratification models is moderate to good, at best, and may be improved by adding new imaging and serum biomarkers, using weighted risk stratification variables, and adding changes in clinical parameters at serial risk stratification during follow-up. Moreover, there is a need for prospective validation of risk stratification models and more research into risk stratification for pediatric PAH has to be pursued.
Data availability statement
The raw data supporting the conclusions of this article will be made available by the authors, without undue reservation.
Author contributions
All authors contributed to the development of the research question and selection criteria. CL and SW developed the search strategy and drafted the initial manuscript. Title-abstract and full-text screening was performed by CL and JD, where RB was consulted in case of disagreement. Data extraction, risk of bias, and data analysis was performed by CL in consultation with RB and JD. RB and JD reviewed and revised the manuscript.
Funding
This study was supported by the Sebald Fund.
Conflict of interest
RB reports that the University Medical Center Groningen contracts with Janssen, Ferrer, and MSD, for advisory board and steering committee activities, outside the submitted work.
The remaining authors declare that the research was conducted in the absence of any commercial or financial relationships that could be construed as a potential conflict of interest.
Publisher’s note
All claims expressed in this article are solely those of the authors and do not necessarily represent those of their affiliated organizations, or those of the publisher, the editors and the reviewers. Any product that may be evaluated in this article, or claim that may be made by its manufacturer, is not guaranteed or endorsed by the publisher.
Supplementary material
The Supplementary Material for this article can be found online at: https://www.frontiersin.org/articles/10.3389/fcvm.2022.1035453/full#supplementary-material
References
1. Simonneau G, Montani D, Celermajer DS, Denton CP, Gatzoulis MA, Krowka M, et al. Haemodynamic definitions and updated clinical classification of pulmonary hypertension. Eur Respir J. (2019) 53:1801913. doi: 10.1183/13993003.01913-2018
2. Medarov BI, Judson MA. The role of calcium channel blockers for the treatment of pulmonary arterial hypertension: How much do we actually know and how could they be positioned today? Respir Med. (2015) 109:557–64. doi: 10.1016/j.rmed.2015.01.004
3. Galie N, Humbert M, Vachiery JL, Gibbs S, Lang I, Torbicki A, et al. 2015 Esc/Ers guidelines for the diagnosis and treatment of pulmonary hypertension: the joint task force for the diagnosis and treatment of pulmonary hypertension of the european society of cardiology (esc) and the european respiratory society (Ers): Endorsed By: Association for european paediatric and congenital cardiology (Aepc), International society for heart and lung transplantation (Ishlt). Eur Respir J. (2015) 46:903–75. doi: 10.1183/13993003.01032-2015
4. Humbert M, Kovacs G, Hoeper MM, Badagliacca R, Berger RMF, Brida M, et al. 2022 Esc/Ers Guidelines for the diagnosis and treatment of pulmonary hypertension. Eur Respir J. (2022) 43:3618–731. doi: 10.1183/13993003.00879-2022
5. Galie N, Channick RN, Frantz RP, Grunig E, Jing ZC, Moiseeva O, et al. Risk stratification and medical therapy of pulmonary arterial hypertension. Eur Respir J. (2019) 53:1801889. doi: 10.1183/13993003.01889-2018
6. D’Alonzo GE, Barst RJ, Ayres SM, Bergofsky EH, Brundage BH, Detre KM, et al. Survival in patients with primary pulmonary hypertension. results from a national prospective registry. Ann Intern Med. (1991) 115:343–9. doi: 10.7326/0003-4819-115-5-343
7. Humbert M, Sitbon O, Yaici A, Montani D, O’Callaghan DS, Jais X, et al. Survival in incident and prevalent cohorts of patients with pulmonary arterial hypertension. Eur Respir J. (2010) 36:549–55. doi: 10.1183/09031936.00057010
8. Thenappan T, Shah SJ, Rich S, Tian L, Archer SL, Gomberg-Maitland M. Survival in pulmonary arterial hypertension: A reappraisal of the nih risk stratification equation. Eur Respir J. (2010) 35:1079–87. doi: 10.1183/09031936.00072709
9. Benza RL, Miller DP, Gomberg-Maitland M, Frantz RP, Foreman AJ, Coffey CS, et al. Predicting survival in pulmonary arterial hypertension: insights from the registry to evaluate early and long-term pulmonary arterial hypertension disease management (Reveal). Circulation. (2010) 122:164–72. doi: 10.1161/CIRCULATIONAHA.109.898122
10. Benza RL, Gomberg-Maitland M, Miller DP, Frost A, Frantz RP, Foreman AJ, et al. The reveal registry risk score calculator in patients newly diagnosed with pulmonary arterial hypertension. Chest. (2012) 141:354–62. doi: 10.1378/chest.11-0676
11. McLaughlin VV, Archer SL, Badesch DB, Barst RJ, Farber HW, Lindner JR, et al. Accf/Aha 2009 Expert consensus document on pulmonary hypertension a report of the american college of cardiology foundation task force on expert consensus documents and the american heart association developed in collaboration with the american college of chest physicians; american thoracic society, inc.; and the pulmonary hypertension association. J Am Coll Cardiol. (2009) 53:1573–619. doi: 10.1016/j.jacc.2009.01.004
12. Ivy DD, Abman SH, Barst RJ, Berger RM, Bonnet D, Fleming TR, et al. Pediatric pulmonary hypertension. J Am Coll Cardiol. (2013) 62(25 Suppl):D117–26. doi: 10.1016/j.jacc.2013.10.028
13. Rosenzweig EB, Abman SH, Adatia I, Beghetti M, Bonnet D, Haworth S, et al. Paediatric pulmonary arterial hypertension: updates on definition, classification, diagnostics and management. Eur Respir J. (2019) 53:1801916. doi: 10.1183/13993003.01916-2018
14. Moher D, Shamseer L, Clarke M, Ghersi D, Liberati A, Petticrew M, et al. Preferred reporting items for systematic review and meta-analysis protocols (Prisma-P) 2015 Statement. Syst Rev. (2015) 4:1. doi: 10.1186/2046-4053-4-1
15. Bramer WM, Giustini D, de Jonge GB, Holland L, Bekhuis T. De-Duplication of database search results for systematic reviews in endnote. J Med Libr Assoc. (2016) 104:240–3. doi: 10.3163/1536-5050.104.3.014
16. Moons KGM, Wolff RF, Riley RD, Whiting PF, Westwood M, Collins GS, et al. Probast: a tool to assess risk of bias and applicability of prediction model studies: Explanation and Elaboration. Ann Intern Med. (2019) 170:W1–33. doi: 10.7326/M18-1377
17. Petrie A, Sabin C. Diagnostic Tools Medical Statistics at a Glance. 3rd ed. Hoboken, NJ: Wiley-Blackwell (2009). p. 115–7.
18. Benza RL, Gomberg-Maitland M, Elliott CG, Farber HW, Foreman AJ, Frost AE, et al. Predicting survival in patients with pulmonary arterial hypertension: The reveal risk score calculator 2.0 and comparison with Esc/Ers-Based risk assessment strategies. Chest. (2019) 156:323–37. doi: 10.1016/j.chest.2019.02.004
19. Benza RL, Kanwar MK, Raina A, Scott JV, Zhao CL, Selej M, et al. Development and validation of an abridged version of the reveal 2.0 risk score calculator, reveal lite 2, for use in patients with pulmonary arterial hypertension. Chest. (2021) 159:337–46. doi: 10.1016/j.chest.2020.08.2069
20. Hoeper MM, Kramer T, Pan Z, Eichstaedt CA, Spiesshoefer J, Benjamin N, et al. Mortality in pulmonary arterial hypertension: prediction by the 2015 european pulmonary hypertension guidelines risk stratification model. Eur Respir J. (2017) 50:1700740. doi: 10.1183/13993003.00740-2017
21. Kylhammar D, Kjellstrom B, Hjalmarsson C, Jansson K, Nisell M, Soderberg S, et al. A comprehensive risk stratification at early follow-up determines prognosis in pulmonary arterial hypertension. Eur Heart J. (2018) 39:4175–81. doi: 10.1093/eurheartj/ehx257
22. Hoeper MM, Pausch C, Olsson KM, Huscher D, Pittrow D, Grunig E, et al. Compera 2.0: A refined 4-strata risk assessment model for pulmonary arterial hypertension. Eur Respir J. (2021) 60:2102311. doi: 10.1183/13993003.02311-2021
23. Boucly A, Weatherald J, Savale L, Jais X, Cottin V, Prevot G, et al. Risk assessment, prognosis and guideline implementation in pulmonary arterial hypertension. Eur Respir J. (2017) 50:1700889. doi: 10.1183/13993003.00889-2017
24. Chiu SN, Weng KP, Lin MC, Wang JN, Hwang BT, Dai ZK, et al. Congenital heart disease with pulmonary artery hypertension in an asian cohort-initial report from tachyon (Taiwan Congenital Heart Disease Associated with Pulmonary Arterial Hypertension) Registry. Int J Cardiol. (2020) 317:49–55. doi: 10.1016/j.ijcard.2020.05.086
25. Dardi F, Manes A, Guarino D, Zuffa E, De Lorenzis A, Magnani I, et al. A pragmatic approach to risk assessment in pulmonary arterial hypertension using the 2015 european society of cardiology/european respiratory society guidelines. Open Heart. (2021) 8:e001725. doi: 10.1136/openhrt-2021-001725
26. Imai R, Adachi S, Yoshida M, Shimokata S, Nakano Y, Okumura N, et al. Single-Center prognostic validation of the risk assessment of the 2015 Esc/Ers guidelines in patients with pulmonary arterial hypertension in japan. Can J Physiol Pharmacol. (2020) 98:653–8. doi: 10.1139/cjpp-2019-0640
27. Li X, Sun X, Huang Y, Wang Y, Yang X, Wang J, et al. Simplified risk stratification for pulmonary arterial hypertension associated with connective tissue disease. Clin Rheumatol. (2019) 38:3619–26. doi: 10.1007/s10067-019-04690-3
28. Mercurio V, Diab N, Peloquin G, Housten-Harris T, Damico R, Kolb TM, et al. Risk assessment in scleroderma patients with newly diagnosed pulmonary arterial hypertension: Application of the Esc/Ers Risk Prediction Model. Eur Respir J. (2018) 52:1800497. doi: 10.1183/13993003.00497-2018
29. Wang J, Li M, Wang Q, Zhang X, Qian J, Zhao J, et al. Pulmonary arterial hypertension associated with primary sjogren’s syndrome: A multicentre cohort study from china. Eur Respir J. (2020) 56:1902157. doi: 10.1183/13993003.02157-2019
30. Xanthouli P, Koegler M, Marra AM, Benjamin N, Fischer L, Eichstaedt CA, et al. Risk stratification and prognostic factors in patients with pulmonary arterial hypertension and comorbidities a cross-sectional cohort study with survival follow-up. Respir Res. (2020) 21:127. doi: 10.1186/s12931-020-01393-1
31. Xiong W, Zhao Y, Xu M, Pudasaini B, Guo X, Liu J. A modified risk score in one-year survival rate assessment of group 1 pulmonary arterial hypertension. BMC Pulm Med. (2018) 18:161. doi: 10.1186/s12890-018-0712-7
32. Yogeswaran A, Richter MJ, Sommer N, Ghofrani HA, Seeger W, Tello K, et al. Advanced risk stratification of intermediate risk group in pulmonary arterial hypertension. Pulm Circ. (2020) 10:2045894020961739. doi: 10.1177/2045894020961739
33. Zelt JGE, Hossain A, Sun LY, Mehta S, Chandy G, Davies RA, et al. Incorporation of renal function in mortality risk assessment for pulmonary arterial hypertension. J Heart Lung Transplant. (2020) 39:675–85. doi: 10.1016/j.healun.2020.03.026
34. Lewis RA, Johns CS, Cogliano M, Capener D, Tubman E, Elliot CA, et al. Identification of cardiac magnetic resonance imaging thresholds for risk stratification in pulmonary arterial hypertension. Am J Respir Crit Care Med. (2020) 201:458–68. doi: 10.1164/rccm.201909-1771OC
35. Simpson CE, Griffiths M, Yang J, Nies MK, Vaidya RD, Brandal S, et al. The angiostatic peptide endostatin enhances mortality risk prediction in pulmonary arterial hypertension. ERJ Open Res. (2021) 7:00378. doi: 10.1183/23120541.00378-2021
36. Kanwar MK, Gomberg-Maitland M, Hoeper M, Pausch C, Pittrow D, Strange G, et al. Risk stratification in pulmonary arterial hypertension using bayesian analysis. Eur Respir J. (2020) 56:2000008. doi: 10.1183/13993003.00008-2020
37. Austin C, Burger C, Kane G, Safford R, Blackshear J, Ung R, et al. High-Risk echocardiographic features predict mortality in pulmonary arterial hypertension. Am Heart J. (2017) 189:167–76. doi: 10.1016/j.ahj.2017.04.013
38. Ghio S, Mercurio V, Fortuni F, Forfia PR, Gall H, Ghofrani A, et al. A Comprehensive echocardiographic method for risk stratification in pulmonary arterial hypertension. Eur Respir J. (2020) 56:2000513. doi: 10.1183/13993003.00513-2020
39. Rhodes CJ, Wharton J, Swietlik EM, Harbaum L, Girerd B, Coghlan JG, et al. Using the plasma proteome for risk stratifying patients with pulmonary arterial hypertension. Am J Respir Crit Care Med. (2022) 205:1102–11. doi: 10.1164/rccm.202105-1118OC
40. Yogeswaran A, Tello K, Lund J, Klose H, Harbaum L, Sommer N, et al. Risk assessment in pulmonary hypertension based on routinely measured laboratory parameters. J Heart Lung Transplant. (2022) 41:400–10. doi: 10.1016/j.healun.2021.10.018
41. Haddad F, Contrepois K, Amsallem M, Denault AY, Bernardo RJ, Jha A, et al. The right heart network and risk stratification in pulmonary arterial hypertension. Chest. (2022) 161:1347–59. doi: 10.1016/j.chest.2021.10.045
42. Boucly A, Weatherald J, Savale L, de Groote P, Cottin V, Prevot G, et al. External validation of a refined 4-strata risk assessment score from the french pulmonary hypertension registry. Eur Respir J. (2021) 59:2102419. doi: 10.1183/13993003.02419-2021
43. Harbaum L, Fuge J, Kamp JC, Hennigs JK, Simon M, Sinning C, et al. Blood carbon dioxide tension and risk in pulmonary arterial hypertension. Int J Cardiol. (2020) 318:131–7. doi: 10.1016/j.ijcard.2020.06.069
44. Griffiths M, Yang J, Simpson CE, Vaidya D, Nies M, Brandal S, et al. St2 Is a biomarker of pediatric pulmonary arterial hypertension severity and clinical worsening. Chest. (2021) 160:297–306. doi: 10.1016/j.chest.2021.01.085
45. Haarman MG, Douwes JM, Ploegstra MJ, Roofthooft MTR, Vissia-Kazemier TR, Hillege HL, et al. The clinical value of proposed risk stratification tools in pediatric pulmonary arterial hypertension. Am J Respir Crit Care Med. (2019) 200:1312–5. doi: 10.1164/rccm.201902-0266LE
46. Quan R, Zhang G, Yu Z, Zhang C, Yang Z, Tian H, et al. Characteristics, goal-oriented treatments and survival of pulmonary arterial hypertension in china: insights from a national multicentre prospective registry. Respirology. (2022) 27:517–28. doi: 10.1111/resp.14247
47. Wilson M, Keeley J, Kingman M, McDevitt S, Brewer J, Rogers F, et al. Clinical application of risk assessment in pah: Expert center aprn recommendations. Pulm Circ. (2022) 12:e12106. doi: 10.1002/pul2.12106
48. Weatherald J, Boucly A, Launay D, Cottin V, Prevot G, Bourlier D, et al. Haemodynamics and Serial Risk Assessment in Systemic Sclerosis Associated Pulmonary Arterial Hypertension. Eur Respir J. (2018) 52:1800678. doi: 10.1183/13993003.00678-2018
49. Benza RL, Miller DP, Foreman AJ, Frost AE, Badesch DB, Benton WW, et al. Prognostic implications of serial risk score assessments in patients with pulmonary arterial hypertension: A registry to evaluate early and long-term pulmonary arterial hypertension disease management (Reveal) Analysis. J Heart Lung Transplant. (2015) 34:356–61. doi: 10.1016/j.healun.2014.09.016
50. Badagliacca R, Papa S, Manzi G, Miotti C, Luongo F, Sciomer S, et al. Usefulness of adding echocardiography of the right heart to risk-assessment scores in prostanoid-treated pulmonary arterial hypertension. JACC Cardiovasc Imaging. (2020) 13:2054–6. doi: 10.1016/j.jcmg.2020.04.005
51. Hansmann G, Koestenberger M, Alastalo TP, Apitz C, Austin ED, Bonnet D, et al. 2019 Updated consensus statement on the diagnosis and treatment of pediatric pulmonary hypertension: The european pediatric pulmonary vascular disease network (Eppvdn), Endorsed by Aepc, Espr and Ishlt. J Heart Lung Transplant. (2019) 38:879–901. doi: 10.1016/j.healun.2019.06.022
52. Lee WT, Ling Y, Sheares KK, Pepke-Zaba J, Peacock AJ, Johnson MK. Predicting Survival in Pulmonary Arterial Hypertension in the Uk. Eur Respir J. (2012) 40:604–11. doi: 10.1183/09031936.00196611
53. Anderson JJ, Lau EM, Lavender M, Benza R, Celermajer DS, Collins N, et al. Retrospective validation of the reveal 2.0 risk score with the australian and new zealand pulmonary hypertension registry cohort. Chest. (2020) 157:162–72. doi: 10.1016/j.chest.2019.08.2203
54. Chang KY, Duval S, Badesch DB, Bull TM, Chakinala MM, De Marco T, et al. Mortality in pulmonary arterial hypertension in the modern era: Early insights from the pulmonary hypertension association registry. J Am Heart Assoc. (2022) 11:e024969. doi: 10.1161/JAHA.121.024969
55. Gong SG, Wu WH, Li C, Zhao QH, Jiang R, Luo CJ, et al. Validity of the Esc risk assessment in idiopathic pulmonary arterial hypertension in china. Front Cardiovasc Med. (2021) 8:745578. doi: 10.3389/fcvm.2021.745578
56. Hjalmarsson C, Kjellstrom B, Jansson K, Nisell M, Kylhammar D, Kavianipour M, et al. Early risk prediction in idiopathic versus connective tissue disease-associated pulmonary arterial hypertension: Call for a refined assessment. ERJ Open Res. (2021) 7:00854–2020. doi: 10.1183/23120541.00854-2020
57. Kylhammar D, Hjalmarsson C, Hesselstrand R, Jansson K, Kavianipour M, Kjellstrom B, et al. Predicting mortality during long-term follow-up in pulmonary arterial hypertension. ERJ Open Res. (2021) 7:00837–2020. doi: 10.1183/23120541.00837-2020
58. Mullin CJ, Khair RM, Damico RL, Kolb TM, Hummers LK, Hassoun PM, et al. Validation of the reveal prognostic equation and risk score calculator in incident systemic sclerosis-associated pulmonary arterial hypertension. Arthritis Rheumatol. (2019) 71:1691–700. doi: 10.1002/art.40918
59. Qu J, Li M, Zeng X, Zhang X, Wei W, Zuo X, et al. Validation of the reveal prognostic models in systemic lupus erythematosus-associated pulmonary arterial hypertension. Front Med (Lausanne). (2021) 8:618486. doi: 10.3389/fmed.2021.618486
60. Sitbon O, Benza RL, Badesch DB, Barst RJ, Elliott CG, Gressin V, et al. Validation of two predictive models for survival in pulmonary arterial hypertension. Eur Respir J. (2015) 46:152–64. doi: 10.1183/09031936.00004414
61. Vraka A, Yerly P, Aubert JD. Comparison of risk stratification scores in pulmonary arterial hypertension: A monocentric retrospective study at lausanne university hospital. Respiration. (2022) 101:565–76. doi: 10.1159/000520886
62. Lewis RA, Billings CG, Hurdman JA, Smith IA, Austin M, Armstrong IJ, et al. Maximal exercise testing using the incremental shuttle walking test can be used to risk-stratify patients with pulmonary arterial hypertension. Ann Am Thoracic Soc. (2021) 18:34–43. doi: 10.1513/AnnalsATS.202005-423OC
63. Vicenzi M, Caravita S, Rota I, Casella R, Deboeck G, Beretta L, et al. The added value of right ventricular function normalized for afterload to improve risk stratification of patients with pulmonary arterial hypertension. PLoS One. (2022) 17:e0265059. doi: 10.1371/journal.pone.0265059
Keywords: pulmonary arterial hypertension, pediatric pulmonary hypertension, risk stratification, risk assessment, survival, outcome, prognosis, children
Citation: Lokhorst C, van der Werf S, Berger RMF and Douwes JM (2022) Risk stratification in adult and pediatric pulmonary arterial hypertension: A systematic review. Front. Cardiovasc. Med. 9:1035453. doi: 10.3389/fcvm.2022.1035453
Received: 02 September 2022; Accepted: 18 October 2022;
Published: 10 November 2022.
Edited by:
Rachel Hopper, Stanford University, United StatesReviewed by:
Allen Everett, The Johns Hopkins Hospital, United StatesPaul Critser, Cincinnati Children’s Hospital Medical Center, United States
Copyright © 2022 Lokhorst, van der Werf, Berger and Douwes. This is an open-access article distributed under the terms of the Creative Commons Attribution License (CC BY). The use, distribution or reproduction in other forums is permitted, provided the original author(s) and the copyright owner(s) are credited and that the original publication in this journal is cited, in accordance with accepted academic practice. No use, distribution or reproduction is permitted which does not comply with these terms.
*Correspondence: Chantal Lokhorst, c.lokhorst@umcg.nl