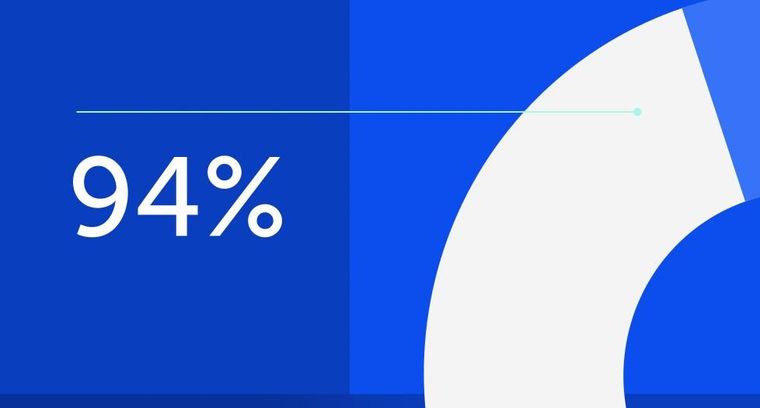
94% of researchers rate our articles as excellent or good
Learn more about the work of our research integrity team to safeguard the quality of each article we publish.
Find out more
MINI REVIEW article
Front. Cardiovasc. Med., 23 November 2022
Sec. Cardiovascular Imaging
Volume 9 - 2022 | https://doi.org/10.3389/fcvm.2022.1031205
This article is part of the Research TopicAdvanced Quantitative Indexes in Cardiovascular Magnetic Resonance ImagingView all 19 articles
Cardiomyopathy (CM) is the leading cause of death for individuals with Duchenne muscular dystrophy (DMD). While DMD CM progresses rapidly and fatally for some in teenage years, others can live relatively symptom-free into their thirties or forties. Because CM progression is variable, there is a critical need for biomarkers to detect early onset and rapid progression. Despite recent advances in imaging and analysis, there are still no reliable methods to detect the onset or progression rate of DMD CM. Cardiac strain imaging is a promising technique that has proven valuable in DMD CM assessment, though much more work has been done in adult CM patients. In this review, we address the role of strain imaging in DMD, the mechanical and functional parameters used for clinical assessment, and discuss the gaps where emerging imaging techniques could help better characterize CM progression in DMD. Prominent among these emerging techniques are strain assessment from 3D imaging and development of deep learning algorithms for automated strain assessment. Improved techniques in tracking the progression of CM may help to bridge a crucial gap in optimizing clinical treatment for this devastating disease and pave the way for future research and innovation through the definition of robust imaging biomarkers and clinical trial endpoints.
Duchenne muscular dystrophy (DMD) is a severe, progressive, X-linked genetic disease that affects roughly 1 in every 5,000 live male births (1–4). DMD results from a mutation in the gene which encodes for the protein dystrophin, leading to a progressive impairment of muscle function (5, 6). A lack of dystrophin leads to a loss of sarcolemma integrity triggering muscle degradation and inflammation followed by necrosis, fibrosis, and fibro-fatty replacement (7, 8). While the average lifespan is now entering the third decade, significant morbidity exists with loss of ambulation by age 13 and death for some in late teens or twenties from respiratory or cardiovascular complications (9, 10). While all DMD patients have inexorably progressive CM, the age of onset and clinical progression of cardiac complications are varied. However, early diagnosis and treatment of CM in DMD patients have been shown to improve both quality and length of life (1, 9).
Cardiac imaging provides both a method for early identification of DMD CM and an opportunity for personalized treatment planning. While advances in imaging technology and data processing have allowed for significant improvements in the diagnosis of DMD CM, there is still more work to be done to fully characterize this disease process using imaging-based metrics. Transthoracic echocardiography (TTE) and cardiac magnetic resonance (CMR) are primarily used in the assessment of cardiac function in DMD patients, and each has distinct advantages in this population. TTE is faster, cost-effective, more accessible, and generally easier to perform on a younger patient population. However, as the disease progresses, TTE is often limited by poor echocardiographic windows due to scoliosis and fat deposition (11–13). CMR is not limited by acoustic windows and it allows for accurate volumetric assessment and tissue characterization through methods such as T1 mapping and late gadolinium enhancement (LGE) (13, 14). It is for these reasons that CMR has become the gold standard for evaluating CM in the DMD patient population for its ability to provide superior diagnostic capability (15–19). Additional cardiac imaging modalities such as magnetic resonance spectroscopy, single-photon emission computed tomography, and x-ray computed tomography can be used for assessment, but are not commonly used in assessment of CM in DMD (20–23). In the DMD patient population, heart function abnormalities such as changes in left ventricular ejection fraction (LVEF) are often masked in the early stages of CM as these patients may be wheelchair-bound and incapable of robust physical activity from skeletal muscle degradation. Thus, metrics such as cardiac image-based strain mapping may be especially useful in this population as they could be more sensitive to subtle mechanistic changes in cardiac disease progression.
The mechanics of cardiac contraction can provide a wealth of information regarding cardiac fitness. The heart wall consists of a group of continuous myocardial fibers oriented in various patterns to produce contraction. For example, many fibers in the subendocardium follow a right handed helical orientation, while in the subepicardium the fibers follow a left handed helical formation with midmyocardial fibers aligning in the circumferential direction (24). The orientation of these fibers is important in determining the mechanical properties of the heart wall as well as the magnitude and principal direction of strain. Subtle changes in these properties can be indicative of cardiac pathology or disease exacerbation (25, 26). This is particularly important in the progression of DMD CM where initial fibrosis is often first seen in the basal subepicardium (21) affecting circumferentially oriented fibers making changes in basal circumferential strain (Eθθ) an early predictor of disease (15, 17).
Put simply, cardiac strain is a measurement of the “stretch” or deformation of the heart wall and is often measured relative to the heart at end-diastole when the ventricle is filled with blood. This simplification is useful, but it is not a perfect definition as pre-strain and pre-stress are present in the myocardium at end-diastole due to the load imposed by the blood and the structural properties of cardiac tissue. Strain is a tensor quantity with normal and shear components, however, a simpler linear approximation of strain, referred to as engineering strain, can be used. The linear approximation of strain is calculated as a change in relative length compared to a reference length and typically assumes strain levels are small (typically below 5%) and may not be as accurate at higher values (27).
From a mechanics perspective, a more robust definition of strain can be calculated in the Lagrangian reference frame to account for multi-directional components of deformation. The 3-dimensional Green-Lagrange strain tensor (E) depends on the 3D deformation gradient tensor (F) and the identity matrix (I) (28, 29) shown below in Equation (1).
This generic formula can be adapted to describe deformation of the myocardium with respect to cylindrical coordinates. Given the complex three-dimensional shape of the heart, this is particularly helpful with cardiac strains often described using three directional components (radial, Err; longitudinal, Ell; and circumferential, Eθθ), with three corresponding shear components of strain used to define twist or rotational motion (Erl, Erθ, Elθ) (30, 31). Additional strain metrics unique to three-dimensional imaging can also be defined, including surface area strain (EA). Surface area strain is defined by the relative change in the size of the endocardial or epicardial surface area of the ventricle from a three-dimensional segmentation (32).
Strain is measured from clinical TTE using three primary techniques: 1) speckle tracking echocardiography (STE) (Figures 1A,B, Table 1), 2) velocity vector imaging (VVI) (Figures 1G,H, Table 1), and 3) tissue Doppler imaging (TDI) (Figures 1E,F, Table 1). Beginning at end-diastole, speckle tracking echocardiography relies on a computational algorithm to track the black and white speckle pattern of the myocardium as it deforms throughout the cardiac cycle. By tracking the motion of these speckles through space and time around a given point in the tissue, one can derive the “motion description” of strain, i.e., Lagrangian strain, in which deformation of the myocardium is tracked from a fixed reference point at end-diastole (30). Strain mapping with 2D-STE has been shown to be useful for early detection of cardiac dysfunction retrospectively in DMD patients and prospectively in canine models of DMD even prior to gross changes in LVEF (12, 37, 40, 41). In one notable recent study of 38 ambulatory boys with DMD (mean age 8.8 ± 1.6 years) 9% showed reduced global strain (< 18%) on 2D-STE prior to clinical features suggestive of cardiac dysfunction (41).
Figure 1. Example Cardiac Strain Imaging Techniques. 2D speckle tracking Transthoracic echocardiogram (TTE) image in the 4-chamber (A) and mid-ventricular short-axis (B)[adapted from Mele et al. (33)]. 3D speckle tracking TTE images in the 4-chamber (C) and short-axis (D)[adapted from Maffessanti et al. (34)]. Tissue Doppler imaging TTE images in the 2-chamber long-axis (E) and short axis (F) in a DMD patient. Velocity vector TTE images in the 4-chamber (G) and short axis (H) in a DMD patient. Cardiovascular magnetic resonance (CMR) strain method depicting myocardial tagging in the 4-chamber (I) and mid-ventricular short-axis (J) views in a DMD patient. CMR feature tracking example images in the 4-chamber (K) and short axis (L) views. Displacement Encoding with Stimulated Echoes (DENSE) example images in the 4-chamber (M) and short-axis (N) views [adapted from Cao et al. (35)]. Strain Encoded example images in the 4-chamber (O) and short-axis (P) view plane in a DMD patient. Multiple visualizations are available for each technique and the images presented here are only representative.
Another method for estimating strain is tracking the relative velocity of each speckle throughout the cardiac cycle with a fixed spatial reference frame. This “spatial description” of strain, i.e., Eulerian or “normal” strain (30). Both TDI and VVI rely on velocity tracking within the tissue and calculate the Eulerian strain. However, in practice, many imaging software packages have the capability of converting between Eulerian and Lagrangian frames of reference (42). TDI specifically utilizes a one-dimensional approach to estimate relative changes in velocity using Doppler ultrasound, while VVI utilizes a two-dimensional B-mode image to track speckle velocities. Both modalities have a relatively high temporal resolution, though TDI is angle-dependent and is only capable of analyzing velocities in one region of the myocardium at a time making comprehensive LV assessment cumbersome. Despite these limitations, both TDI (39) and VVI (12) have been used successfully to show strain differences in small DMD populations compared to age-matched controls (n = 32 and n = 24 respectively).
Advances in ultrasound technology in recent decades have allowed for three-dimensional analysis of myocardial mechanics. 3D imaging has shown good agreement with other techniques and reduced interuser variability, though its use clinically is still limited and more research is needed to characterize its incremental advantages over conventional 2D measurements (43). While 2D-STE is prone to error propagation and relies on geometric assumptions regarding the left ventricle, 3D-STE is more reproducible in both clinical and experimental models (44, 45) (Figures 1C,D). In addition, 3D imaging allows for the calculation of unique strain metrics such as surface area strain (EA). In DMD patients, strain mapping with 3D-STE can detect early clinical progression despite these patients having normal LVEF (38). Yu et al. demonstrated that (EA) derived from 3D-STE had an 85.7% sensitivity and a 71.0% specificity for differentiating DMD patients (n = 56) from controls (n = 31; (38); Table 1). While these metrics show improvement over 2D-STE-derived metrics, this method still suffers from limited echocardiographic windows, low spatial and temporal resolution, relatively small study populations, and inter-vendor differences (11, 32, 46–48).
There are a variety of methods for estimating strain using CMR. Given the breadth of this field, this review focuses on techniques shown to have utility in DMD CM assessment including CMR-tagging (Figures 1I,J), HARmonic Phase (HARP) analysis, and CMR-Feature Tracking (CMR-FT) (Figures 1K,L), in addition to a brief discussion of additional sequences such as Tissue Phase Mapping (TPM), Displacement Encoding with Stimulated Echoes (DENSE) (Figures 1M,N), and Strain Encoded MRI (SENC) (Figures 1O,P) that are useful strain techniques, but that are currently less studied in the DMD population. The following reviews on strain imaging using CMR are excellent and provide more comprehensive descriptions of these techniques outside of DMD-specific applications (31, 49–53).
CMR tagging is a strain estimation method that utilizes parallel radiofrequency pulses to impose orthogonal planes of saturated myocardial magnetization to rapidly create a grid of dark saturated pixels overlaying the myocardium at end-diastole [Figures 1I,J; (50, 54)]. These tags, or lines of saturation, remain throughout the cardiac cycle and fade according to the strength of the magnetic field. The deformation of this grid pattern can be tracked and analyzed using post-processing software to estimate regional and global strain. This method allows for longitudinal and circumferential strain estimates, but is often not reliable for radial strain estimates given the large spacing of the grid pattern leading to low resolution to estimate radial deformation (55).
HARmonic Phase (HARP) analysis is a commonly used strain imaging technique used in a large number of CMR post-processing software packages (55–57). HARP analysis in conjunction with CMR tagging has been used to estimate strain in DMD patients establishing strain as a reliable method to stratify cardiac disease severity (15, 17). The HARP technique relies on isolating one Fourier component of the amplitude modulated data and tracks the phase on a pixel-by-pixel basis (56). This technique allows for more rapid and reproducible strain estimation. Some drawbacks to the HARP method include a relatively low spatial resolution, tag fading, additional scan time, and a lack of standardization (50, 54).
CMR-feature tracking (CMR-FT) is another deformation estimation technique that utilizes characteristic features in a CMR scan to estimate strain (Table 1). A spatial deformation map is produced by tracking tissue edges, brightness, and homogeneity throughout the cardiac cycle. However, given the relative homogeneity within the myocardium in conventional CMR images, regional estimates of strain are often misrepresented and there is additional work needed to improve these estimations. Despite these drawbacks, CMR-FT does not require any additional scan time as it does not need any specialized sequences, unlike most other CMR strain techniques. Additionally, in DMD patients, CMR-FT has been shown to detect morphologic changes in the absence of late gadolinium enhancement (LGE) as well as detect changes between DMD and controls not seen using 3D-STE (36). Another study by Raucci et al. showed that CMR-FT in DMD could be used to detect the presence or absence of LGE (OR 2.6[1.1,6.0], p = 0.029, and OR 2.3[1.0,5.1], p = 0.049, respectively), though the authors suggest these metrics should not replace LGE when contrast can be administered safely (58).
DENSE, SENC, and TPM are additional methods for strain estimation using CMR but are less studied in DMD populations (Figures 1M–P, Table 1). DENSE, SENC, and TPM techniques all rely on specialized image sequences that take additional scan time, but they produce relatively high spatial and temporal resolution needed for strain estimates. DENSE is generally accepted as one of the most accurate and reproducible methods for strain imaging. DENSE uses a stimulated echo to produce the phase information that is directly proportional to tissue displacement. Through the analysis of directional-encoded phase images, the Lagrangian displacement fields can be produced to estimate strain. A recent study showed that regional circumferential strain as measured by DENSE CMR in healthy pediatric controls and DMD patients was both accurate and reproducible (ICC>75%) (59).
SENC, like HARP, uses radiofrequency pulses to create parallel tagged lines with out-of-plane phase encoding gradients to estimate strain. Tracked frequency changes can then be visualized to show contracting and non-contracting tissue by generating a high-resolution color-coded strain map (Figures 1O,P). While SENC has been used to visualize strain changes in DMD in research studies (14), to our knowledge, comprehensive studies showing its clinical use are still forthcoming. Finally, TPM is a technique that derives spatial deformation and strain directly from the pixel phase to encode velocity from each image. TPM has been shown to be useful for comprehensive analysis of myocardial motion with a high spatial and temporal resolution in pulmonary hypertension (60), obesity (61), measuring myocardial torsion (62), and identifying sex and age-specific differences in adults (63). TPM, SENC, and DENSE are not currently being widely used clinically or in DMD specifically, but they are being studied extensively in research settings (57, 64, 65) and in the future may provide additional value for assessing the rate of CM progression in DMD.
The field of machine and deep learning has been progressing rapidly and is the fastest-growing approach for cardiac image segmentation in recent years (66). Fully convolutional neural networks, and in particular the U-Net network structure, have been particularly powerful in automating biomedical image segmentation and analysis (67, 68). These techniques, however, are susceptible to bias and rely on a large training dataset for “ground-truth” analysis. Although deep learning techniques have been applied to DMD phenotyping through genetic classification (69), to our knowledge, no one has applied these techniques to DMD cardiac image segmentation and patient classification and prognosis. This constitutes a promising area for future study and innovation.
The type of machine and/or deep learning technique being used depends on the task that is being investigated (e.g., image classification, image segmentation, image enhancement). In the context of strain analysis, one common approach is to segment the cine image sequence followed by post-processing deformation analysis. The most common architecture used in medical image segmentation is a convolutional neural net (CNN) (70). A typical CNN functions by applying convolution filters to images followed by pooling layers which downsample the image data and allow for the CNN to incorporate contextual information. While this downsampling lowers image resolution, upsampling with concatenation allows for image resolution to be restored, thus allowing for the neural net to incorporate both low- and high-resolution features. The prime example of this type of CNN is a U-Net, so-called for its architecture incorporating convolution, pooling, and upscaling used for feature identification. The U-Net architecture functions by inputting a batch set of CMR images followed by feature classification. Through each iteration, multiscale features are learned through a series of convolutional layers and max-pooling operations. These learned features are then combined through upsampling and convolution to generate pixel-wise predictions for the selected class features (i.e., left ventricle, wall, right ventricle, etc. (67). The U-Net architecture has been shown to perform remarkably well in segmenting 2D and 3D CMR datasets (66, 67). These segmentations can in turn be used for rapid and automated cardiac strain analysis.
Numerous studies have shown the feasibility and efficacy of a deep learning algorithm using CMR data for automatic segmentation and detection of LV geometry and function (66, 67, 71, 72). Though this type of analysis has not been applied to a registry of DMD patient imaging data, future applications of these methods and algorithms could be used to greatly benefit the early identification of cardiac dysfunction and progression rate to aid in treatment planning. In addition, the use of these techniques on a large cohort of DMD patients could allow for generalizability of findings to other populations of interest such as female carriers of DMD who only exhibit subtle cardiac dysfunction (73).
A limitation of the deep learning approach is that the chosen algorithm may be susceptible to perturbations in image quality and artifacts, especially at the base and apex of the heart. One such example is a breathing artifact in CMR scans that appears as sections of myocardium offset from the rest of the scan, a problem especially pervasive in the younger DMD population (74). An alternative approach to overcome variability in image acquisition and artifacts could be to incorporate different available views from CMR imaging datasets (four-chamber long axis, two-chamber long axis, short axis) to correct for these abnormalities. Another potential limitation is the generalizability of this deep learning network to CMR images taken at a variety of locations where different imaging approaches, magnets, gradients, or magnetic field strengths may affect the quality of segmentation. Alternative structures and data processing such as the application of recurrent neural networks, data normalization, or data augmentation might improve the generalizability of these algorithms as demonstrated by Chen et al. (66, 67).
This literature assessment contextualizes cardiac strain imaging techniques in the setting of DMD CM. Here we reviewed the general pathophysiology of DMD-associated CM, discussed an overview of the most relevant imaging techniques, and reviewed the cardiac strain imaging methods generally and those that are important for characterizing DMD. We concluded by examining potential future applications that may fill these gaps with a particular focus on machine learning and emerging methods in artificial intelligence that could be used to guide treatment.
CE, CG, JS, and LM helped with the conception, literature review presented in this article, helped draft, and critically revise the manuscript providing intellectual content. JS provided protected access to example CMR and ultrasound images. LM and JS provided key DMD-specific clinical expertise and expert imaging guidance. All authors approved the final version of this manuscript.
This publication was supported by the Ackerman/Nicholoff Family (Indianapolis, IN) (LM), Fighting Duchenne Foundation and the Fight DMD/Jonah and Emory Discovery Grant (Nashville, TN) (JS), the Food and Drug Administration Orphan Products Grant R01FD006649 (JS), the National Center for Research Resources, Grant UL1 RR024975-01, and is now at the National Center for Advancing Translational Sciences, Grant 2 UL1 TR000445-06 (Bethesda, MD) (JS), and the National Heart, Lung, and Blood Institute of the National Institutes of Health under award Number K23HL123938 and R56HL141248 (Bethesda, MD) (JS) and F30HL162452 (CE). This publication was also made possible with support from Grant Number, UL1TR002529 (S. Moe and S. Wiehe, co-PIs) from the National Institutes of Health, National Center for Advancing Translational Sciences, Clinical and Translational Sciences Award (CE) and the Leslie A. Geddes Endowment at Purdue University (CG). Publication of this article was funded in part by Purdue University Libraries Open Access Publishing Fund. The content is solely the responsibility of the authors and does not necessarily represent the official views of the National Institutes of Health.
The authors declare that the research was conducted in the absence of any commercial or financial relationships that could be construed as a potential conflict of interest.
All claims expressed in this article are solely those of the authors and do not necessarily represent those of their affiliated organizations, or those of the publisher, the editors and the reviewers. Any product that may be evaluated in this article, or claim that may be made by its manufacturer, is not guaranteed or endorsed by the publisher.
1. Mcnally EM, Kaltman JR, Benson DW, Canter CE, Cripe LH, Duan D, et al. Contemporary cardiac issues in Duchenne muscular dystrophy. Circulation. (2015) 131:1590–8. doi: 10.1161/CIRCULATIONAHA.114.015151
2. Romitti PA, Zhu Y, Puzhankara S, James KA, Nabukera SK, Zamba GKD, et al. Prevalence of Duchenne and becker muscular dystrophies in the United States. Pediatrics. (2015) 135:513–21. doi: 10.1542/peds.2014-2044
3. Ryder S, Leadley RM, Armstrong N, Westwood M, De Kock S, Butt T, et al. The burden, epidemiology, costs and treatment for Duchenne muscular dystrophy: an evidence review. Orphanet J Rare Dis. (2017) 12:79. doi: 10.1186/s13023-017-0631-3
4. Yiu EM, Kornberg AJ. Duchenne muscular dystrophy. J Paediatr Child Health. (2015) 51:759–64. doi: 10.1111/jpc.12868
5. Hoffman EP, Brown RH, Kunkel LM. Dystrophin: the protein product of the Duchenne muscular dystrophy locus. Cell. (1987) 51:919–28. doi: 10.1016/0092-8674(87)90579-4
6. Muntoni F, Torelli S, Ferlini A. Dystrophin and mutations: one gene, several proteins, multiple phenotypes. Lancet Neurol. (2003) 2:731–40. doi: 10.1016/S1474-4422(03)00585-4
7. Boland BJ, Silbert PL, Groover RV, Wollan PC, Silverstein MD. Skeletal, cardiac, and smooth muscle failure in Duchenne muscular dystrophy. Pediatr Neurol. (1996) 14:7–12. doi: 10.1016/0887-8994(95)00251-0
8. Carlson CG. The dystrophinopathies: an alternative to the structural hypothesis. Neurobiol Dis. (1998) 5:3–15. doi: 10.1006/nbdi.1998.0188
9. Birnkrant DJ, Bushby K, Bann CM, Alman BA, Apkon SD, Blackwell A, et al. Diagnosis and management of Duchenne muscular dystrophy, part 2: respiratory, cardiac, bone health, and orthopaedic management. Lancet Neurol. (2018) 17:347–61. doi: 10.1016/S1474-4422(18)30025-5
10. Passamano L, Taglia A, Palladino A, Viggiano E, D'AMBROSIO P, Scutifero M, et al. Improvement of survival in Duchenne muscular dystrophy: retrospective analysis of 835 patients. Acta Myologica. (2012) 31:121.
11. Van Bockel EAP, Lind JS, Zijlstra JG, Wijkstra PJ, Meijer PM, Van Den Berg MP, et al. Cardiac assessment of patients with late stage Duchenne muscular dystrophy. Netherlands Heart J. (2009) 17:232–7. doi: 10.1007/BF03086253
12. Jo WH, Eun LY, Jung JW, Choi JY, Gang SW. Early marker of myocardial deformation in children with Duchenne muscular dystrophy assessed using echocardiographic myocardial strain analysis. Yonsei Med J. (2016) 57:900. doi: 10.3349/ymj.2016.57.4.900
13. Soslow JH, Xu M, Slaughter JC, Stanley M, Crum K, Markham LW, et al. Evaluation of echocardiographic measures of left ventricular function in patients with Duchenne muscular dystrophy: assessment of reproducibility and comparison to cardiac magnetic resonance imaging. J Am Soc Echocardiogr. (2016) 29:983–91. doi: 10.1016/j.echo.2016.07.001
14. Lee S, Lee M, Hor KN. The role of imaging in characterizing the cardiac natural history of Duchenne muscular dystrophy. Pediatr Pulmonol. (2021) 56:766–81. doi: 10.1002/ppul.25227
15. Hor KN, Kissoon N, Mazur W, Gupta R, Ittenbach RF, Al-Khalidi HR, et al. Regional circumferential strain is a biomarker for disease severity in Duchenne muscular dystrophy heart disease: a cross-sectional study. Pediatr Cardiol. (2015) 36:111–9. doi: 10.1007/s00246-014-0972-9
16. Hor KN, Baumann R, Pedrizzetti G, Tonti G, Gottliebson WM, Taylor M, et al. Magnetic resonance derived myocardial strain assessment using feature tracking. J Vis Exp. (2011) 48:2356. doi: 10.3791/2356
17. Hor KN, Wansapura J, Markham LW, Mazur W, Cripe LH, Fleck R, et al. Circumferential strain analysis identifies strata of cardiomyopathy in Duchenne muscular dystrophy: a cardiac magnetic resonance tagging study. J Am Coll Cardiol. (2009) 53:1204–10. doi: 10.1016/j.jacc.2008.12.032
18. Puchalski MD, Williams RV, Askovich B, Sower CT, Hor KH, Su JT, et al. Late gadolinium enhancement: precursor to cardiomyopathy in Duchenne muscular dystrophy?. Int J Cardiovasc Imaging. (2009) 25:57–63. doi: 10.1007/s10554-008-9352-y
19. Raman SV, Hor KN, Mazur W, Halnon NJ, Kissel JT, He X, et al. Eplerenone for early cardiomyopathy in Duchenne muscular dystrophy: a randomised, double-blind, placebo-controlled trial. The Lancet Neurology. (2015) 14:153–61. doi: 10.1016/S1474-4422(14)70318-7
20. Krommydas A, Rajani R, Hart N, Kapetanakis S. The Olympic rings of Duchenne muscular dystrophy - cardiac computed tomography wins gold. Revista Portuguesa de Cardiologia. (2016) 35:551–2. doi: 10.1016/j.repc.2015.12.010
21. Poonja S, Power A, Mah JK, Fine NM, Greenway SC. Current cardiac imaging approaches in Duchenne muscular dystrophy. J Clin Neuromuscul Dis. (2018) 20:85–93. doi: 10.1097/CND.0000000000000204
22. Power LC, O'Grady GL, Hornung TS, Jefferies C, Gusso S, Hofman PL. Imaging the heart to detect cardiomyopathy in Duchenne muscular dystrophy: a review. Neuromuscular Disorders. (2018) 28:717–30. doi: 10.1016/j.nmd.2018.05.011
23. Van Der Bijl P, Delgado V, Bootsma M, Bax JJ. Risk stratification of genetic, dilated cardiomyopathies associated with neuromuscular disorders. Circulation. (2018) 137:2514–27. doi: 10.1161/CIRCULATIONAHA.117.031110
24. Goergen CJ, Sosnovik DE. From molecules to myofibers: multiscale imaging of the myocardium. J Cardiovasc Transl Res. (2011) 4:493–503. doi: 10.1007/s12265-011-9284-0
25. Helm P, Beg MF, Miller MI, Winslow RL. Measuring and mapping cardiac fiber and laminar architecture using diffusion tensor MR imaging. Ann N York Acad Sci. (2005) 1047:296. doi: 10.1196/annals.1341.026
26. Streeter Jr DD, Spotnitz HM, Patel DP, Ross Jr J, Sonnenblick EH. Fiber orientation in the canine left ventricle during diastole and systole. Circ Res. (1969) 24:339–347. doi: 10.1161/01.RES.24.3.339
28. Humphrey J, Strumpf R, Yin F. Determination of a constitutive relation for passive myocardium: I. A new functional form. J Biomech Eng. (1990) 112:333–9. doi: 10.1115/1.2891193
29. Ligas M, Banaś M, Szafarczyk A. A method for local approximation of a planar deformation field. Rep Geodesy Geoinform. (2019) 108:1–8. doi: 10.2478/rgg-2019-0007
30. Geyer H, Caracciolo G, Abe H, Wilansky S, Carerj S, Gentile F, et al. Assessment of myocardial mechanics using speckle tracking echocardiography: fundamentals and clinical applications. J Am Soc Echocardiogr. (2010) 23:351–69. doi: 10.1016/j.echo.2010.02.015
31. Tee M, Noble JA, Bluemke DA. Imaging techniques for cardiac strain and deformation: comparison of echocardiography, cardiac magnetic resonance and cardiac computed tomography. Expert Rev Cardiovasc Ther. (2013) 11:221. doi: 10.1586/erc.12.182
32. Jasaityte R, Heyde B, D'Hooge J. Current state of three-dimensional myocardial strain estimation using echocardiography. J Am Soc Echocardiogr. (2013) 26:15–28. doi: 10.1016/j.echo.2012.10.005
33. Mele D, Rizzo P, Pollina AV, Fiorencis A, Ferrari R. Cancer therapy-induced cardiotoxicity: role of ultrasound deformation imaging as an aid to early diagnosis. Ultrasound Med Biol. (2015) 41:627–43. doi: 10.1016/j.ultrasmedbio.2014.11.015
34. Maffessanti F, Nesser HJ, Weinert L, Steringer-Mascherbauer R, Niel J, Gorissen W, et al. Quantitative evaluation of regional left ventricular function using three-dimensional speckle tracking echocardiography in patients with and without heart disease. Am J Cardiol. (2009) 104:1755–62. doi: 10.1016/j.amjcard.2009.07.060
35. Cao JJ, Ngai N, Duncanson L, Cheng J, Gliganic K, Chen Q. A comparison of both DENSE and feature tracking techniques with tagging for the cardiovascular magnetic resonance assessment of myocardial strain. J Cardiovasc Magn Reson. (2018) 20:1–9. doi: 10.1186/s12968-018-0448-9
36. Siegel B, Olivieri L, Gordish-Dressman H, Spurney CF. Myocardial strain using cardiac MR feature tracking and speckle tracking echocardiography in Duchenne muscular dystrophy patients. Pediatr Cardiol. (2018) 39:478–83. doi: 10.1007/s00246-017-1777-4
37. Song G, Zhang J, Wang X, Zhang X, Sun F, Yu X. Usefulness of speckle-tracking echocardiography for early detection in children with Duchenne muscular dystrophy: a meta-analysis and trial sequential analysis. Cardiovasc Ultrasound. (2020) 18:26. doi: 10.1186/s12947-020-00209-y
38. Yu HK, Xia B, Liu X, Han C, Chen W, Li Z. Initial application of three-dimensional speckle-tracking echocardiography to detect subclinical left ventricular dysfunction and stratify cardiomyopathy associated with Duchenne muscular dystrophy in children. Int J Cardiovasc Imaging. (2019) 35:67–76. doi: 10.1007/s10554-018-1436-8
39. Mertens L, Ganame J, Claus P, Goemans N, Thijs D, Eyskens B, et al. Early regional myocardial dysfunction in young patients with Duchenne muscular dystrophy. J Am Soc Echocardiogr. (2008) 21:1049–54. doi: 10.1016/j.echo.2008.03.001
40. Takano H, Fujii Y, Yugeta N, Takeda S, Wakao Y. Assessment of left ventricular regional function in affected and carrier dogs with duchenne muscular dystrophy using speckle tracking echocardiography. BMC Cardiovasc Disord. (2011) 11:23. doi: 10.1186/1471-2261-11-23
41. Prakash N, Suthar R, Sihag BK, Debi U, Kumar RM, Sankhyan N. Cardiac MRI and echocardiography for early diagnosis of cardiomyopathy among boys with Duchenne muscular dystrophy: a cross-sectional study. Front Pediatr. (2022) 10:818608. doi: 10.3389/fped.2022.818608
42. Yip G, Abraham T, Belohlavek M, Khandheria BK. Clinical applications of strain rate imaging. J Am Soc Echocardiogr. (2003) 16:1334–42. doi: 10.1067/j.echo.2003.09.004
43. Muraru D, Niero A, Rodriguez-Zanella H, Cherata D, Badano L. Three-dimensional speckle-tracking echocardiography: benefits and limitations of integrating myocardial mechanics with three-dimensional imaging. Cardiovasc Diagn Ther. (2018) 8:101. doi: 10.21037/cdt.2017.06.01
44. Ferraiuoli P, Fixsen LS, Kappler B, Lopata RGP, Fenner JW, Narracott AJ. Measurement of in vitro cardiac deformation by means of 3D digital image correlation and ultrasound 2D speckle-tracking echocardiography. Med Eng Phys. (2019) 74:146–52. doi: 10.1016/j.medengphy.2019.09.021
45. Wu VCC, Takeuchi M. Three-dimensional echocardiography: current status and real-life applications. Acta Cardiol Sin. (2017) 33:107. doi: 10.6515/acs20160818a
46. Gayat E, Ahmad H, Weinert L, Lang RM, Mor-Avi V. Reproducibility and inter-vendor variability of left ventricular deformation measurements by three-dimensional speckle-tracking echocardiography. J Am Soc Echocardiogr. (2011) 24:878–85. doi: 10.1016/j.echo.2011.04.016
47. Mor-Avi V, Lang RM, Badano LP, Belohlavek M, Cardim NM, Derumeaux G, et al. Current and evolving echocardiographic techniques for the quantitative evaluation of cardiac mechanics: ASE/EAE consensus statement on methodology and indications endorsed by the Japanese society of echocardiography. Eur J Echocardiogr. (2011) 12:167–205. doi: 10.1016/j.echo.2011.01.015
48. Tsuburaya RS, Uchizumi H, Ueda M, Demura Y, Mukaida S, Sudou S, et al. Utility of real-time three-dimensional echocardiography for Duchenne muscular dystrophy with echocardiographic limitations. Neuromusc Disord. (2014) 24:402–8. doi: 10.1016/j.nmd.2013.12.007
49. Dandel M, Lehmkuhl H, Knosalla C, Suramelashvili N, Hetzer R. Strain and strain rate imaging by echocardiography-basic concepts and clinical applicability. Curr Cardiol Rev. (2009) 5:133–48. doi: 10.2174/157340309788166642
50. Ibrahim ESH. Myocardial tagging by cardiovascular magnetic resonance: evolution of techniques-pulse sequences, analysis algorithms, and applications. J Cardiovasc Magn Reson. (2011) 13:36. doi: 10.1186/1532-429X-13-36
51. Scatteia A, Baritussio A, Bucciarelli-Ducci C. Strain imaging using cardiac magnetic resonance. Heart Fail Rev. (2017) 22:465–476. doi: 10.1007/s10741-017-9621-8
52. Voigt JU, Cvijic M. 2-and 3-dimensional myocardial strain in cardiac health and disease. JACC Cardiovasc Imaging. (2019) 12:1849–63. doi: 10.1016/j.jcmg.2019.01.044
53. Amzulescu MS, De Craene M, Langet H, Pasquet A, Vancraeynest D, Pouleur AC, et al. Myocardial strain imaging: review of general principles, validation, and sources of discrepancies. Eur Heart J Cardiovasc Imaging. (2019) 20:605–19. doi: 10.1093/ehjci/jez041
54. Amundsen BH, Crosby J, Steen PA, Torp H, Slørdahl SA, Støylen A. Regional myocardial long-axis strain and strain rate measured by different tissue Doppler and speckle tracking echocardiography methods: a comparison with tagged magnetic resonance imaging. Eur Heart J Cardiovasc Imaging. (2009) 10:229–37. doi: 10.1093/ejechocard/jen201
55. Magrath P, Maforo N, Renella P, Nelson SF, Halnon N, Ennis DB. Cardiac MRI biomarkers for Duchenne muscular dystrophy. Biomark Med. (2018) 12:1271–89. doi: 10.2217/bmm-2018-0125
56. Castillo E, Osman NF, Rosen BD, El-Shehaby I, Pan L, Jerosch-Herold M, et al. Quantitative assessment of regional myocardial function with MR-tagging in a multi-center study: interobserver and intraobserver agreement of fast strain analysis with Harmonic Phase (HARP) MRI. J Cardiovasc Magn Reson. (2005) 7:783–91. doi: 10.1080/10976640500295417
57. Osman NF, Sampath S, Atalar E, Prince JL. Imaging longitudinal cardiac strain on short-axis images using strain-encoded MRI. Magn Reson Med. (2001) 46:324–34. doi: 10.1002/mrm.1195
58. Raucci FJ, Xu M, George-Durrett K, Crum K, Slaughter JC, Parra DA, et al. Non-contrast cardiovascular magnetic resonance detection of myocardial fibrosis in Duchenne muscular dystrophy. J Cardiovasc Magn Reson. (2021) 23:48. doi: 10.1186/s12968-021-00736-1
59. Liu ZQ, Maforo NG, Renella P, Halnon N, Wu HH, Ennis DB. Reproducibility of left ventricular CINE DENSE strain in pediatric subjects with duchenne muscular dystrophy. In: International Conference on Functional Imaging and Modeling of the Heart. Chicago, IL: Springer (2021). p. 232–41.
60. Knight DS, Steeden JA, Moledina S, Jones A, Coghlan JG, Muthurangu V. Left ventricular diastolic dysfunction in pulmonary hypertension predicts functional capacity and clinical worsening: a tissue phase mapping study. J Cardiovasc Magn Reson. (2015) 17:1–11. doi: 10.1186/s12968-015-0220-3
61. Rider OJ, Ajufo E, Ali MK, Petersen SE, Nethononda R, Francis JM, et al. Myocardial tissue phase mapping reveals impaired myocardial tissue velocities in obesity. Int J Cardiovasc Imaging. (2015) 31:339–47. doi: 10.1007/s10554-014-0548-z
62. Chitiboi T, Schnell S, Collins J, Carr J, Chowdhary V, Honarmand AR, et al. Analyzing myocardial torsion based on tissue phase mapping cardiovascular magnetic resonance. J Cardiovasc Magn Reson. (2016) 18:1–13. doi: 10.1186/s12968-016-0234-5
63. Foll D, Jung B, Schilli E, Staehle F, Geibel A, Hennig J, et al. Magnetic resonance tissue phase mapping of myocardial motion: new insight in age and gender. Circ Cardiovasc Imaging. (2010) 3:54–64. doi: 10.1161/CIRCIMAGING.108.813857
64. Mcginley G, Bendiksen BA, Zhang L, Aronsen JM, Nordén ES, Sjaastad I, et al. Accelerated magnetic resonance imaging tissue phase mapping of the rat myocardium using compressed sensing with iterative soft-thresholding. PLoS ONE. (2019) 14:e0218874. doi: 10.1371/journal.pone.0218874
65. Neizel M, Lossnitzer D, Korosoglou G, Schäufele T, Lewien A, Steen H, et al. Strain-encoded (SENC) magnetic resonance imaging to evaluate regional heterogeneity of myocardial strain in healthy volunteers: comparison with conventional tagging. J Magn Reson Imaging. (2009) 29:99–105. doi: 10.1002/jmri.21612
66. Chen C, Qin C, Qiu H, Tarroni G, Duan J, Bai W, et al. Deep learning for cardiac image segmentation: a review. Front Cardiovasc Med. (2020) 7:25. doi: 10.3389/fcvm.2020.00025
67. Chen C, Bai W, Davies RH, Bhuva AN, Manisty CH, Augusto JB, et al. Improving the generalizability of convolutional neural network-based segmentation on CMR images. Front Cardiovasc Med. (2020) 7:105. doi: 10.3389/fcvm.2020.00105
68. Ronneberger O, Fischer P, Brox T. U-net: convolutional networks for biomedical image segmentation. In:Navab N, Hornegger J, Wells W, Frangi A, , editors. Medical Image Computing and Computer-Assisted Intervention-MICCAI 2015. MICCAI 2015. Lecture Notes in Computer Science(), Vol. 9351. Cham: Springer (2015). p. 234–41.
69. Khamparia A, Singh A, Anand D, Gupta D, Khanna A, Arun Kumar N, et al. A novel deep learning-based multi-model ensemble method for the prediction of neuromuscular disorders. Neural Comput Appl. (2020) 32:11083–95. doi: 10.1007/s00521-018-3896-0
70. Litjens G, Ciompi F, Wolterink JM, de Vos BD, Leiner T, Teuwen J, et al. State-of-the-art deep learning in cardiovascular image analysis. JACC Cardiovasc imaging. (2019) 12(8 Part 1):1549–65. doi: 10.1016/j.jcmg.2019.06.009
71. Dilsizian ME, Siegel EL. Machine meets biology: a primer on artificial intelligence in cardiology and cardiac imaging. Curr Cardiol Rep. (2018) 20:139. doi: 10.1007/s11886-018-1074-8
72. Hammouda K, Khalifa F, Abdeltawab H, Elnakib A, Giridharan GA, Zhu M, et al. A new framework for performing cardiac strain analysis from cine MRI imaging in mice. Sci Rep. (2020) 10:7725. doi: 10.1038/s41598-020-64206-x
73. Mah ML, Cripe L, Slawinski MK, Al-Zaidy SA, Camino E, Lehman KJ, et al. Duchenne and Becker muscular dystrophy carriers: evidence of cardiomyopathy by exercise and cardiac MRI testing. Int J Cardiol. (2020) 316:257–65. doi: 10.1016/j.ijcard.2020.05.052
74. Fratz S, Chung T, Greil GF, Samyn MM, Taylor AM, Valsangiacomo Buechel ER, et al. Guidelines and protocols for cardiovascular magnetic resonance in children and adults with congenital heart disease: SCMR expert consensus group on congenital heart disease. J Cardiovasc Magn Reson. (2013) 15:51. doi: 10.1186/1532-429X-15-51
Keywords: duchenne muscular dystrophy, strain imaging, cardiac magnetic resonance, pediatric cardiology, cardiac imaging, echocardiography, strain
Citation: Earl CC, Soslow JH, Markham LW and Goergen CJ (2022) Myocardial strain imaging in Duchenne muscular dystrophy. Front. Cardiovasc. Med. 9:1031205. doi: 10.3389/fcvm.2022.1031205
Received: 29 August 2022; Accepted: 03 November 2022;
Published: 23 November 2022.
Edited by:
Ming Yen Ng, The University of Hong Kong, Hong Kong SAR, ChinaReviewed by:
Yucheng Chen, Sichuan University, ChinaCopyright © 2022 Earl, Soslow, Markham and Goergen. This is an open-access article distributed under the terms of the Creative Commons Attribution License (CC BY). The use, distribution or reproduction in other forums is permitted, provided the original author(s) and the copyright owner(s) are credited and that the original publication in this journal is cited, in accordance with accepted academic practice. No use, distribution or reproduction is permitted which does not comply with these terms.
*Correspondence: Craig J. Goergen, Y2dvZXJnZW5AcHVyZHVlLmVkdQ==
Disclaimer: All claims expressed in this article are solely those of the authors and do not necessarily represent those of their affiliated organizations, or those of the publisher, the editors and the reviewers. Any product that may be evaluated in this article or claim that may be made by its manufacturer is not guaranteed or endorsed by the publisher.
Research integrity at Frontiers
Learn more about the work of our research integrity team to safeguard the quality of each article we publish.