EP-PINNs: Cardiac Electrophysiology Characterisation Using Physics-Informed Neural Networks
- Department of Cardiology, Cardiovascular Research Institute Maastricht (CARIM), Maastricht University, Maastricht, Netherlands
A Commentary on
EP-PINNs: Cardiac electrophysiology characterisation using physics-informed neural networks
by Herrero Martin, C., Oved, A., Chowdhury, R. A., Ullmann, E., Peters, N. S., Bharath, A. A., et al. (2022). Front Cardiovasc Med. 8:768419. doi: 10.3389/fcvm.2021.768419
Introduction
Atrial fibrillation (AF) is the most common cardiac arrhythmia with a significant medical and socioeconomic impact (1). Current AF management is centered around stroke-prevention, control of comorbidities and risk factors, and symptom management by rate- and rhythm control. Recent work has suggested that rhythm control may even improve clinical outcomes compared to rate control, particularly when initiated early, as demonstrated by the pivotal EAST AFNET-4 trial (2). Nevertheless, current options for rhythm-control therapy remain suboptimal due to the potential proarrhythmic side-effects of antiarrhythmic drugs (3) and limited efficacy of both drugs and catheter ablation, primarily attributable to a one-size-fits-most therapeutic approach (3, 4). A better understanding of the mechanisms underlying AF in an individual patient is expected to improve therapeutic success. However, identifying patient-specific mechanisms through cardiac mapping is challenging, invasive, time-consuming, and only gives partial information about the underlying electrophysiology under specific conditions (4). It is likely that novel approaches providing more detailed information on the underlying mechanisms will facilitate a more accurate localization of AF drivers, thereby enabling more personalized and better treatments.
Computer models offer perfect control and observability, facilitating a detailed investigation of arrhythmia mechanisms. In general, computer models can be divided into data-driven models and mechanistic models (3). Data-driven models use techniques like machine learning to identify complex relationships between datapoints to predict specific outcomes. For example, Attia et al. employed a neural network (NN) trained on a large dataset of electrocardiogram (ECG) recordings in sinus rhythm to identify patients with a diagnosis of AF (5). On the other hand, mechanistic models incorporate biophysical laws (typically as a system of differential equations) to simulate the dynamics of cardiac electrophysiology at the cellular-, tissue-, and/or organ-level (3). Mechanistic models have a long history and are increasingly being used in clinical and industry applications. For example, the “comprehensive in-vitro proarrhythmia assay” (CiPA) initiative from the pharmaceutical industry and regulatory agencies includes cellular mechanistic models to perform in-silico safety pharmacology screening for drug-induced ventricular arrythmias (3, 6). In addition, patient-specific organ-level models (“digital twins”) are starting to be used in clinical decision making. For example, Boyle et al. (7) used an organ-level modeling approach with patient-specific fibrotic patterns for preprocedural identification of AF ablation targets. The clinical value of such personalized simulation-guided AF ablation strategies is currently evaluated in a randomized clinical trial (ClinicalTrials.gov identifier NCT04101539).
Both mechanistic and data-driven modeling have pros and cons: data-driven models are, in theory, more easily applicable to clinical practice, since they use clinical parameters as inputs and outputs, and are hypothesis-free. However, the black-box nature of many algorithms hampers model interpretability and the models can only identify associations, which may not be causal. By contrast, mechanistic models are inherently causal and, in principle, could be personalized to reflect pathophysiology in an individual patient. However, at present, these models typically cannot predict clinically relevant outcomes. Furthermore, the tremendous computational power, together with the complex integration of imaging and cardiac mapping required for organ-level modeling, hampers its clinical application. Finally, both data-driven and mechanistic models require large amounts of (pre)clinical electrophysiological data from humans, which are challenging to obtain.
EP-PINNs
Recently, a novel “hybrid” modeling approach, called physics-informed NNs (PINNs), has been proposed, which incorporates biophysical knowledge (i.e., mechanistic models of ordinary- and partial differential equations) to constrain the NN (4). In particular, PINNs are trained to minimize a loss function containing terms for the data mismatch, the concordance with physical laws, as well as initial and boundary conditions (Figure 1). The concordance with physical laws is defined as agreement with the differential equations of the model (i.e., the difference between the actual change in a model variable and its predicted change according to the differential equations). The training of this PINN requires fewer experimental data, since the solution space is constrained by the known relationships embedded in the differential equations, and ultimately provides parameters for both the NN and the mechanistic model, enabling identification of local properties captured by the mechanistic model. The latter could be particularly important for gaining a better understanding of the patient-specific mechanistical underpinnings of arrhythmias. In cardiac electrophysiology, PINNs have previously been used to estimate activation times and conduction velocities (CVs) to overcome the limited spatial resolution of clinical measurements, but the performance was suboptimal and could not easily be extended to other electrophysiological parameters (4, 8–10).
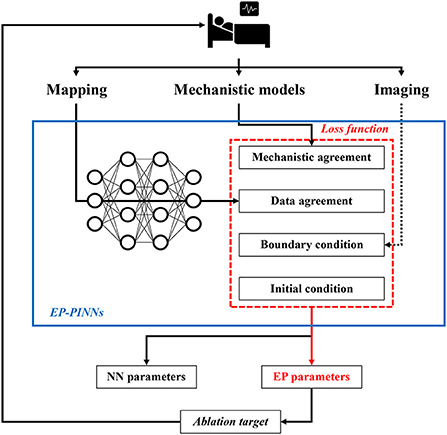
Figure 1. Potential future application of EP-PINNs. EP-PINNs use a neural network that integrates mechanistic models with its associated biophysical laws as constraints, while simultaneously fitting the observed mapping data. The estimated electrophysiological parameters can be used to identify ablation targets, thereby facilitating personalized therapy. *The dotted line highlights potential future additions of other data sources such as cardiac imaging.
Recently, Herrero Martin et al. introduced “EP-PINNs” wherein the Aliev-Panfilov model of action-potential generation and propagation was used to constrain a NN fed with 2D simulation or experimental optical mapping data (4). The Aliev-Panfilov model uses two differential equations and six parameters to model action-potential propagation and refractoriness, enabling estimation of local electrophysiological parameters like action potential duration and CV. The EP-PINN was trained and evaluated on 1D and 2D in-silico data, whereafter it could reproduce a variety of spatio-temporal activation patterns (e.g., planar, centrifugal, and spiral waves). Proarrhythmic electrical heterogeneities (e.g., fibrosis, ischemic lesions) were incorporated by lowering the conductivity of a specific region. Importantly, the EP-PINN model could estimate the electrophysiological parameters (i.e., action-potential duration, excitability, and conductivity) related to these heterogeneities, albeit with lower accuracy than the homogeneous data, particularly in the presence of spiral waves. To further validate the findings from the in-silico data, the model's performance was evaluated on optical mapping data from neonatal rat ventricular cardiomyocytes in response to pharmacological interventions.
Discussion
The main strengths of the EP-PINNs are the ability to predict, with varying accuracies, action-potential dynamics and parameters, even though the input data were noisy and sparse. Indeed, the in-vitro validation of the model shows that the model still performs well with noisy experimental data, although it required more training data. Moreover, the fact that the EP-PINNs performed well on a more complex canine atrial cardiomyocyte model suggests that the results are robust and that the model can be applied in a variety of settings. In the future, alternative, more complex mechanistic models could be integrated. Since the EP-PINNs approach provides estimates for model parameters that cannot be measured directly, these models may inform on specific remodeling processes. However, combined estimation of multiple parameters, particularly excitability combined with CV, proved challenging in the present study (4), highlighting potential difficulties for simultaneous parameter estimates in more complex models.
The use of biophysical laws for regularization also increased the computational efficiency tremendously, while still ensuring realistic behavior. Nonetheless, EP-PINNs remain complex and computationally expensive models due to their fully connected architecture and intricate training protocol, where higher-dimensional simulations will increase the training time substantially, particularly when more complex mechanistic models are going to be used.
Identification of spatial heterogeneities in underlying electrophysiological parameters, some of which cannot be measured directly experimentally, suggests that EP-PINNs could be used in the future to identify personalized ablation targets. However, in the study by Herrero Martin et al. (4) the EP-PINNs were trained on 2D spatio-temporal points from in-silico tissue simulations or optical mapping experiments, whereas the arrhythmogenic substrate in patients is 3D. It is not known whether EP-PINNs can resolve the complex 3D patterns of AF in clinical practice, which input data would be needed for this task, and what the computational requirements would be, although this could be investigated using available 3D mechanistic computational models. Moreover, the flexible structure of EP-PINNs in theory makes it possible to include additional constraints, e.g., based on mechanical or structural information from imaging data (Figure 1). Of note, for mechanical data, several mechanistic models operating on different scales are also available, which could be used in conjunction with electrophysiological models.
Taken together, Herrero Martin et al. showed that EP-PINNs are a potentially promising tool for integrating mechanistic modeling and machine learning. Although significant additional work is needed, EP-PINNs could ultimately aid personalized AF therapy by identifying local electrophysiological targets for ablation therapy based on patient-specific mapping and imaging data, combined with state-of-the-art understanding of AF pathophysiology captured by mechanistic models (Figure 1). The EP-PINNs' code is publicly available, which facilitates the first steps toward future clinical applications, e.g., by applying the EP-PINNs to more complex mechanistic models, simulate more chaotic arrhythmogenic patterns like multiple wavelets, and improve the computational efficiency of the model.
Author contributions
All authors listed have made a substantial, direct, and intellectual contribution to the work and approved it for publication.
Funding
This work was supported by the Netherlands Organization for Scientific Research (NWO/ZonMW Vidi 09150171910029 to JH).
Conflict of interest
The authors declare that the research was conducted in the absence of any commercial or financial relationships that could be construed as a potential conflict of interest.
Publisher's note
All claims expressed in this article are solely those of the authors and do not necessarily represent those of their affiliated organizations, or those of the publisher, the editors and the reviewers. Any product that may be evaluated in this article, or claim that may be made by its manufacturer, is not guaranteed or endorsed by the publisher.
References
1. Hindricks G, Potpara T, Dagres N, Arbelo E, Bax JJ, Blomstrom-Lundqvist C, et al. 2020 ESC guidelines for the diagnosis management of atrial fibrillation developed in collaboration with the European association for cardio-thoracic surgery (EACTS): the task force for the diagnosis management of atrial fibrillation of the European society of cardiology (ESC) developed with the special contribution of the European heart rhythm association (EHRA) of the ESC. Eur Heart J. (2021) 42:373–498. doi: 10.1093/eurheartj/ehab648
2. Camm AJ, Naccarelli GV, Mittal S, Crijns H, Hohnloser SH, Ma CS, et al. The increasing role of rhythm control in patients with atrial fibrillation: JACC state-of-the-art review. J Am Coll Cardiol. (2022) 79:1932–48. doi: 10.1016/j.jacc.2022.03.337
3. Heijman J, Sutanto H, Crijns H, Nattel S, Trayanova NA. Computational models of atrial fibrillation: achievements, challenges, and perspectives for improving clinical care. Cardiovasc Res. (2021) 117:1682–99. doi: 10.1093/cvr/cvab138
4. Herrero Martin C, Oved A, Chowdhury RA, Ullmann E, Peters NS, Bharath AA, et al. EP-PINNs: cardiac electrophysiology characterisation using physics-informed neural networks. Front Cardiovasc Med. (2022) 8:768419. doi: 10.3389/fcvm.2021.768419
5. Attia ZI, Noseworthy PA, Lopez-Jimenez F, Asirvatham SJ, Deshmukh AJ, Gersh BJ, et al. An artificial intelligence-enabled ECG algorithm for the identification of patients with atrial fibrillation during sinus rhythm: a retrospective analysis of outcome prediction. Lancet. (2019) 394:861–7. doi: 10.1016/S0140-6736(19)31721-0
6. Li Z, Mirams GR, Yoshinaga T, Ridder BJ, Han X, Chen JE, et al. General Principles for the validation of proarrhythmia risk prediction models: an extension of the CiPA in silico strategy. Clin Pharmacol Ther. (2020) 107:102–11. doi: 10.1002/cpt.1647
7. Boyle PM, Zghaib T, Zahid S, Ali RL, Deng D, Franceschi WH, et al. Computationally guided personalized targeted ablation of persistent atrial fibrillation. Nat Biomed Eng. (2019) 3:870–9. doi: 10.1038/s41551-019-0437-9
8. Sahli Costabal F, Yang Y, Perdikaris P, Hurtado DE, Kuhl E. Physics-Informed neural networks for cardiac activation mapping. Front Phys. (2020) 8:42. doi: 10.3389/fphy.2020.00042
9. Grandits T, Pezzuto S, Costabal FS, Perdikaris P, Pock T, Plank G, et al. Learning atrial fiber orientations and conductivity tensors from intracardiac maps using physics-informed neural networks. Funct Imaging Model Heart. (2021) 2021:650–8. doi: 10.1007/978-3-030-78710-3_62
10. Grandits T, Pezzuto S, Lubrecht JM, Pock T, Plank G, Krause R. PIEMAP: personalized inverse eikonal model from cardiac electro-anatomical maps. arXiv. (2020) arXiv:2008.10724. Available online at: https://ui.adsabs.harvard.edu/abs/2020arXiv200810724G (accessed August 1, 2020).
Keywords: atrial fibrillation, cardiac electrophysiology, computer model, machine learning, physics informed neural networks, parameter estimation
Citation: Meier S and Heijman J (2022) Commentary: EP-PINNs: Cardiac electrophysiology characterisation using physics-informed neural networks. Front. Cardiovasc. Med. 9:1003652. doi: 10.3389/fcvm.2022.1003652
Received: 26 July 2022; Accepted: 11 August 2022;
Published: 24 August 2022.
Edited by:
Michael Brunner, St. Josefskrankenhaus, GermanyReviewed by:
Björn Müller-Edenborn, University Heart Center Freiburg, GermanyCopyright © 2022 Meier and Heijman. This is an open-access article distributed under the terms of the Creative Commons Attribution License (CC BY). The use, distribution or reproduction in other forums is permitted, provided the original author(s) and the copyright owner(s) are credited and that the original publication in this journal is cited, in accordance with accepted academic practice. No use, distribution or reproduction is permitted which does not comply with these terms.
*Correspondence: Jordi Heijman, jordi.heijman@maastrichtuniversity.nl
†These authors have contributed equally to this work