- 1School of Public Health, North China University of Science and Technology, Tangshan, China
- 2Shantou University Medical College, Shantou, China
- 3Department of Epidemiology, School of Public Health, Air Force Medical University, Xi'an, China
- 4Division of Health Research, Faculty of Health and Medicine, Lancaster University, Lancaster, United Kingdom
- 5Department of Cardiology, Kailuan General Hospital, Tangshan, China
- 6College of Nursing and Rehabilitation, North China University of Science and Technology, Tangshan, China
- 7Department of Neurosurgery, Kailuan General Hospital, Tangshan, China
Background: Lifestyles generally change across the life course yet no prospective study has examined direct associations between healthy lifestyle trajectories and subsequent cardiovascular disease (CVD) or all-cause mortality risk.
Methods: Healthy lifestyle score trajectories during 2006–2007, 2008–2009, and 2010–2011 were collated through latent mixture modeling. An age-scale based Cox proportional hazard regression model was implemented to calculate hazard ratios (HR) with corresponding 95% confidence intervals (CI) for developing CVD or all-cause mortality across healthy lifestyle trajectories.
Results: 52,248 participants were included with four distinct trajectories identified according to healthy lifestyle scores over 6 years i.e., low-stable (n = 11,248), high-decreasing (n = 7,374), low-increasing (n = 7,828), and high-stable (n = 25,799). Compared with the low-stable trajectory, the high-stable trajectory negatively correlated with lower subsequent risk of developing CVD (HR, 0.73; 95% CI, 0.65–0.81), especially stroke (HR, 0.70; 95% CI, 0.62–0.79), and all-cause mortality (HR, 0.89; 95% CI, 0.80–0.99) under a multivariable-adjusted model. A protective effect for CVD events was observed only in men and in those without diabetes, while a reduced risk of all-cause mortality was observed only in those older than 60 years, though interactions were not statistically significant. Marginally significant interactions were observed between the changing body mass index (BMI) group, healthy lifestyle score trajectories and stratified analysis. This highlighted an inverse correlation between the high-stable trajectory and CVD in BMI decreased and stable participants as well as all-cause mortality in the stable BMI group. The low-increasing trajectory also had reduced risk of CVD only when BMI decreased and in all-cause mortality only when BMI was stable.
Conclusions: Maintaining a healthy lifestyle over 6 years corresponds with a 27% lower risk of CVD and an 11% lower risk in all-cause mortality, compared with those engaging in a consistently unhealthy lifestyle. The benefit of improving lifestyle could be gained only after BMI change is considered further. This study provides further evidence from China around maintaining/improving healthy lifestyles to prevent CVD and early death.
Introduction
Cardiovascular disease (CVD) has a high global prevalence and remains the leading contributor to global mortality (1). Liu et al. conducted an exhaustive analysis of the burden of CVD in China and found that the prevalence of CVD doubled since 1990, reaching 94 million in 2016 (2). Aside from calculating that CVD is responsible for 42% of all-cause mortality (3), evidence also appeared to suggest that the burden of this disease maybe lower in coastal provinces where there is a higher level of economic development. However, diversity in China is not based solely upon regional differences nor should it be based upon geo-specific investment because lifestyles are culturally embedded, and there are employment-based migratory patterns which determine accessibility and health-seeking behaviors. Therefore, it remains necessary to develop the evidence-base around healthy lifestyles for public health interventions.
Aside from the established risk factors such as sex and age, chronic comorbidities, modifiable unhealthy lifestyle factors, including smoking, excessive alcohol intake, physical inactivity, sedentary behaviors and unhealthy diet, have proven to increase the risk of CVD and CVD-related mortality (4, 5). The converse is also true, with a higher number of healthy lifestyle factors correlating with significantly lower risk of developing CVD (6) and mortality (7, 8). Indeed, obesity (9), which is linked to type 2 diabetes (10) and hypertension (11), is considered a primary comorbidity of CVD. However, there is a distinct clinical iceberg when it comes to type 2 diabetes and hypertension where obese people teeter on the edge, in a pre-diabetic state, suffering only mild, and perhaps even infrequent symptoms. This means that aside from analyzing prevalence rates we must focus on lifestyles to develop public health interventions and to maximize the benefit.
Lifestyles however, often change across the life-course therefore retrospective, cross-sectional studies are often unable to disentangle the minutia involved in such change. That is, given the prospects of change, retrospectives have a tendency to assess non-causal explanations and can be constrained by data availability. Researchers in the field have managed to identify a number of key biological and lifestyle factors which correspond with an increased likelihood of developing CVD, as well as other types of diseases across three generations of participants. Yet, to the best of our knowledge, there has been no prospective study published which examines direct associations between healthy lifestyle trajectories and subsequent CVD or all-cause mortality risk in China.
Materials and Methods
Study Design and Participants
The Kailuan cohort was established in 2006 in Tangshan, Hebei province, China, where participants undergo a comprehensive check-up every two years. Please see The Kailuan Study, trial identification: ChiCTR-TNC-11001489; registration site: http://www.chictr.org.cn/index.aspx; registration number: 11001489 (12) for further details. Since June 2006, 101,510 adults have been enrolled including 81,110 men and 20,400 women. Participants were enrolled from 11 hospitals in the Kailuan community and completed questionnaires, clinical examinations, and laboratory tests. After excluding 7,953 participants i.e., 3,394 with CVD diagnosis and 4,559 who did not have sufficient data around lifestyles, 93,557 remained. We excluded a further 3,589 who developed CVD or died and 37,720 who did not complete questionnaires etc. after the second visit. Finally, 52,249 participants were included for analysis, please see the Supplementary Material, Figure 1 for further details. The Ethics Committees at Kailuan General Hospital approved this study and all participants were asked to provide informed consent before analysis took place.
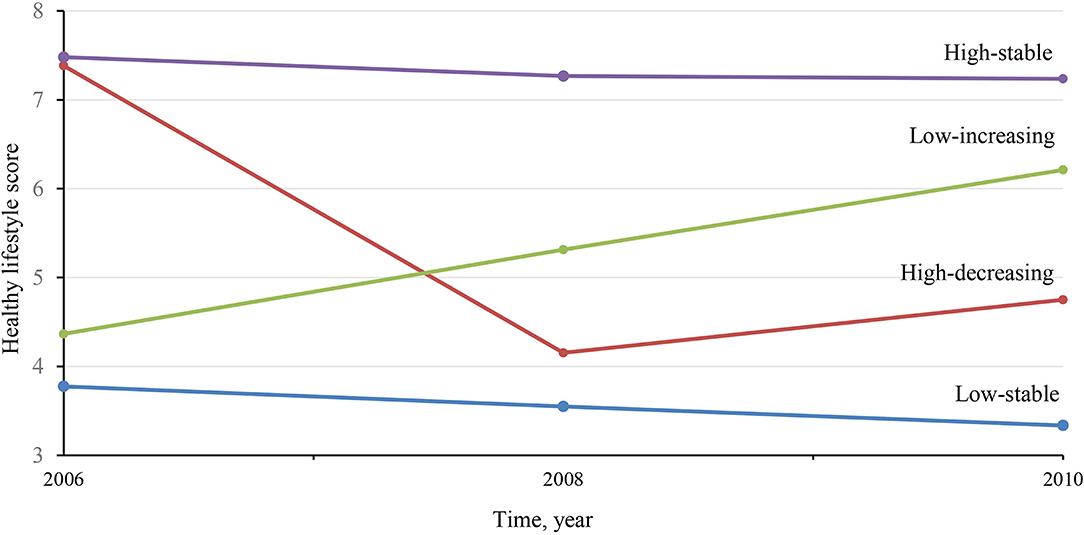
Figure 1. Mean healthy lifestyle score in 2006, 2008 and 2010, according to four healthy lifestyle score trajectories.
Definitions of Lifestyles Status at Baseline
Information was gathered over three biennial visits. Data around lifestyle status, including: smoking status, alcohol intake, physical activity, sedentary behaviors and salt intake, were collected face-to-face by trained staff using a standard questionnaire, implemented in previous research (13). A score was assigned for each component of the five predefined lifestyles, with zero representing a poor level, intermediate represented by 1 and ideal was given 2 points. Please see Supplementary Table 1 for further details. Totals ranged from 0 which was considered the worst possible scenario and 10 considered optimal.
Current smokers were defined as participants who smoked at least one cigarette, every day for at least 6 months prior. While past smokers were defined as participants who reported smoking previously but quit smoking before or during the survey. Likewise, current drinkers were defined as participants who drank at least one time per month over the past year. Whereas, past drinkers were defined as those who reported having drank previously but had quit before or during the survey.
Poor, intermediate or an ideal amount of sedentary behavior was defined with an average sedentary time ≥8 h/day, 4–7 h/day and <4 h/day, respectively. Intermediate physical activity was assigned to those taking physical activity for 20+ min per time and 1–2 times per week. While an ideal level activity was defined as ≥3 times per week during leisure time.
Due to the lack of detailed dietary data between 2006–2010 and considering the influence of salt intake over CVD risks among the Chinese population, total salt intake was determined by questionnaire which can be considered a surrogate measure for diet quality. Poor, intermediate and ideal diet was defined with a salt intake ≥10 g/day, 6–9 g/day and <6 g/day. As described previously, a validation was made according 2014 dataset when diet information was collected through a semi-quantitative, validated food-frequency questionnaire, suggesting a strong association between higher perceived salt intake and lower healthy diet score (14).
Covariates at Baseline
Demographics including age, sex, level of education, comorbidities and related medicaments, as well as laboratory tests were collected. Education was classified as less than high school and high school or above. Weight and height were measured, before calculating body mass index (BMI) as weight in kilograms divided by height in meters squared. Overweight/obesity was defined as BMI ≥ 24 kg/m2 which is in accordance with Chinese standards (15). Hypertension was defined as blood pressure ≥140/90 mmHg, administration of antihypertensive medication, or self-reported previous diagnosis. Diabetes mellitus was defined as a fasting blood glucose (FBG) at concentrations of ≥7.0 mmol/L, administration of glucose-lowering medication, or self-reported previous diagnosis. Dyslipidemia was defined as achieving one of the following criteria according to Chinese standards: (1) triglyceride (TG) ≥ 2.3 mmol/L; (2) total cholesterol (TC) ≥ 6.4 mmol/L; (3) low density lipoprotein-cholesterol (LDL-C) ≥ 4.1 mmol/L, or (4) high density lipoprotein cholesterol (HDL-C) <1.0 mmol/L (16).
Ascertainment of Incident CVD Events and Death
The main outcome was incidence of CVD events including myocardial infarction and stroke. As previously described (17), all participants were linked to the Municipal Social Insurance Institution and the Hospital Discharge Register for incidence of CVD, which covers all of the Kailuan study participants. To further identify potential CVD events, we reviewed discharge lists from 11 hospitals during 2006–2019 and asked for a history of CVD via a questionnaire during biennial interviews.
For all suspected CVD events, three experienced clinical adjudicators, who were blinded to the study design, reviewed medical records. An incident myocardial infarction was diagnosed according to the World Health Organization's (WHO) Multinational Monitoring of Trends and Determinants in Cardiovascular Disease criteria on the basis of clinical symptoms and dynamic changes in cardiac enzymes and/or biomarker concentrations and electrocardiogram results (18). Stroke was diagnosed according to symptoms and neuroimages captured using computed tomographic (CT) or magnetic resonance imaging (MRI), with other diagnostic reports provided in accordance with WHO criteria (19), as described previously (20). All-cause mortality data was collected via provincial vital statistics offices.
Statistical Analyses
Healthy lifestyle scores for each of the three visits across 2006–2007, 2008–2009, and 2010–2011 were computed separately. Trajectories were then determined using latent mixture modeling, within the PROC TRAJ procedure (21). Model fit was assessed using Bayesian information criterion and the number of participants in each trajectory i.e. >5% of the overall population.
The basic characteristics among trajectories were compared using one-way ANOVA or the Kruskal-Wallis H test for continuous variables and chi-square test for categorical variables. Person-time at follow-up was computed for each participant from the date of the third survey (which was the baseline) to the date of CVD diagnosis, death, or the end of follow-up on the 31st December, 2019), whichever came first. The proportional hazards assumption was satisfied and an age-scale based Cox proportional hazard regression model was implemented to calculate the hazard ratios (HR) and 95% confidence intervals (CI) for developing CVD or all-cause mortality across healthy lifestyle score trajectories. A multivariable-adjusted model was then developed for sex, educational level, BMI, FBG, systolic blood pressure (SBP), estimated glomerular filtration rate (eGFR), LDL-C, and HDL-C. In order to ensure the association could not be explained by a single healthy lifestyle score during follow-up, we additionally adjusted for 2006 and 2010 healthy lifestyle score.
In addition, analyses were conducted using the incident of myocardial infarction and stroke, separately. In order to gain insight into a potential correlation between healthy lifestyle score trajectories and CVD or all-cause mortality, data were arranged and, in some instances stratified according to sex, age, overweight/obesity, hypertension, diabetes mellitus, dyslipidemia and BMI change. Potential interactions were assessed between healthy lifestyle score trajectories and factors after controlling for the aforementioned covariates. Stratified results of these factors are presented for consideration.
Sensitivity analyses were also conducted strengthen the connection. To ensure the association was not influenced by imputation, repeated analysis was conducted in the dataset without imputating. To strengthen the temporality of connections, lag-analysis was conducted by excluding CVD incidents or death, separately, with onset during the first 2 years of follow-up, which may not be caused by lifestyles in such a short time.
Missing data for covariates are presented in the Supplementary Table 2 and were imputed using Multivariate Imputation by Chained Equations (MICE) within the PROC MI procedure in SAS. All tests were two-sided with the threshold for statistical significance set at p < 0.05. All analyses were performed using SAS version 9.4 (SAS Institute, Inc, Cary, NC).
Results
Population Characteristics
52,248 participants including 39,793 men and 12,455 women were involved. The average age was 52.6 +/- 11.8 years. Please see Supplementary Figure 1 for further demographic summary data. Four distinct trajectories were identified according to healthy lifestyle scores over the 6 years (Figure 1). There was a low-stable trajectory which consisted of 11,248 participants with a mean healthy lifestyle score (mHLS) and ranged from 3.3–3.8 between 2006–2010. There was also a high-decreasing trajectory (n = 7,374, mean mHLS decreased from 7.4 in 2006 to 4.8 in 2010). We also observed a low-increasing trajectory (n = 7,828, mHLS increased from 4.4 in 2006 to 6.2 in 2010), and high-stable trajectory (n = 25,799, mHLS range 7.2–7.5 during 2006–2010).
Demographics within the four trajectories are provided in Table 1. Age, sex, level of education, medical history i.e., hypertension and diabetes, and biological data including BMI, SBP, diastolic blood pressure (DBP), FBG, eGFR, TG, LDL-C, and HDL-C were quite different among trajectories.
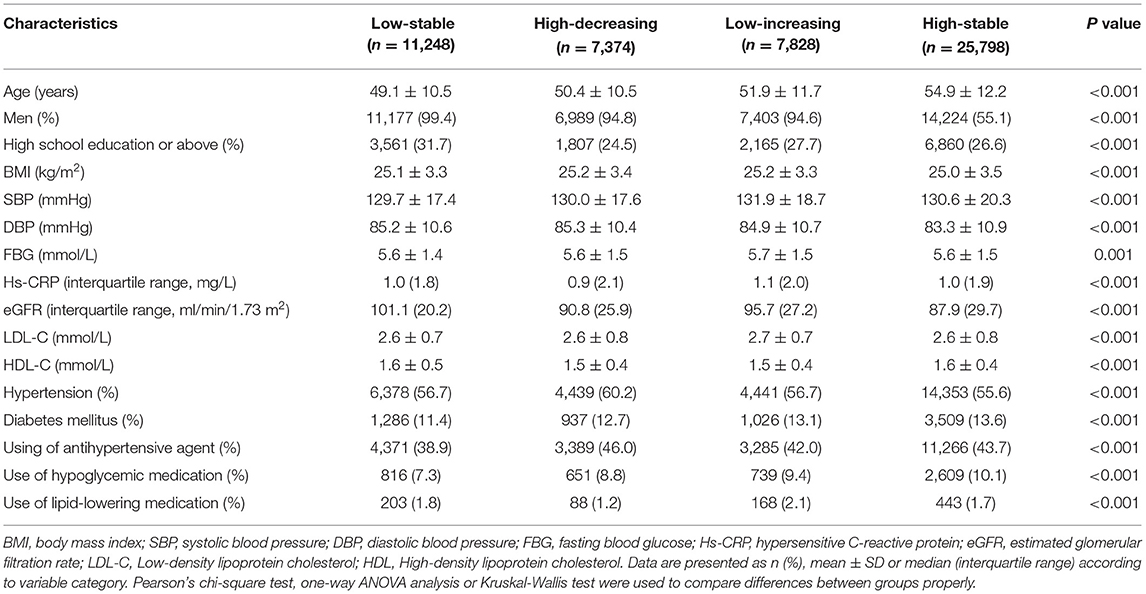
Table 1. Characteristics of 52,248 participants according to healthy lifestyle score trajectories from 2006 to 2010.
Healthy Lifestyle Score Trajectories and CVD Incidence and All-Cause Mortality
2,724 CVD events were observed over the 8 years follow-up period. As can be seen in Table 2, the incidence of low-stable, high-decreasing, low-increasing and high-stable trajectories was 6.23, 6.56, 7.10 and 5.58 per 1,000 person-years (PYs), respectively. Compared with low-stable trajectory, high-stable trajectory appeared to negatively correlate with a lower subsequent risk of developing CVD, with an HR of 0.63 (95% CI, 0.57–0.69) in unadjusted model and 0.73 (95% CI, 0.65–0.81) in the multivariable-adjusted model 1.
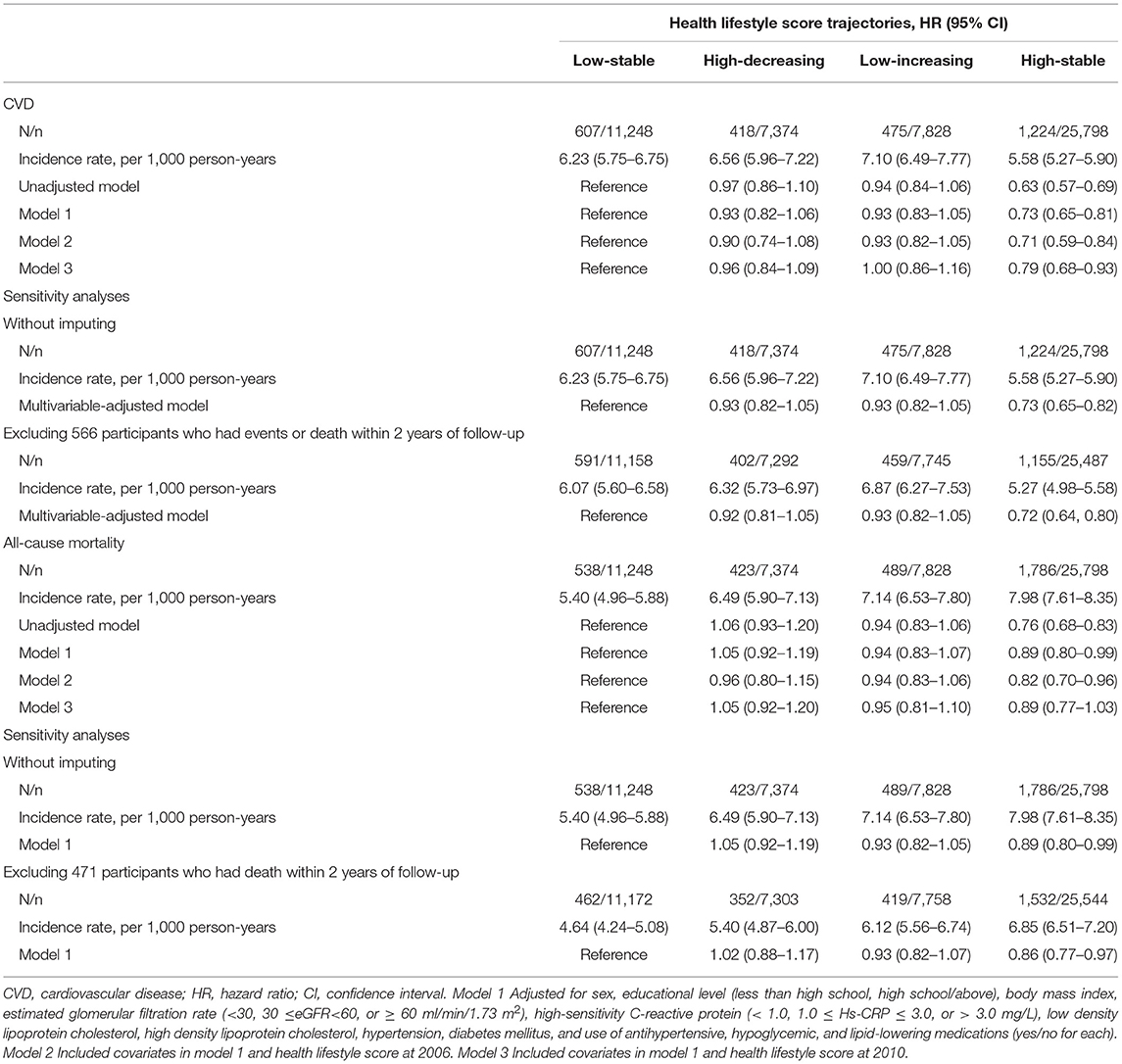
Table 2. Incidence of CVD and all-cause mortality according to healthy lifestyle score trajectories from 2006 to 2010.
After additional adjustments for 2006 or 2010 healthy lifestyle scores, the association remained with HRs of 0.71 (95% CI, 0.59–0.84) and 0.79 (95% CI, 0.68–0.93). Sensitivity analyses was conducted without imputing or excluding CVD events or death which occurred during the first 2 years of follow-up, the result remained stable.
Among 2,724 CVD events, 2,172 were strokes and 505 were myocardial infarction, 47 involved both (Supplementary Table 3). A decreased risk was also observed between healthy lifestyle score trajectories and stroke incidence, with HRs of 0.62 (95% CI, 0.55–0.69), 0.70 (95% CI, 0.62–0.79), 0.70 (95% CI, 0.58–0.84) and 0.77 (95% CI, 0.65–0.92) in unadjusted model and multivariable-adjusted model 1–3. Significantly associations with myocardial infarction were only observed in unadjusted model (HR, 0.67; 95% CI, 0.53–0.82).
Our analysis of all-cause mortality was provided in Table 2. 3,236 deaths were observed during the follow-up period. The incidence rate of low-stable, high-decreasing, low-increasing and high-stable trajectories were 5.40, 6.49, 7.14 and 7.98 per 1,000 person-years (PYs), respectively. Compared with low-stable trajectory, high-stable trajectory appeared to correlate with lower subsequent risk of all-cause mortality, with HRs of 0.76 (95% CI, 0.68–0.83) in unadjusted model and 0.89 (95% CI, 0.80–0.99) in the multivariable-adjusted model. These findings did not change after additional adjustment for the 2006 or 2010 healthy lifestyle scores, without imputing, or excluding CVD incidence which occurred during the first 2 years of follow-up. The HRs with corresponding confidence intervals were as follows: 0.82 (95% CI, 0.70–0.96), 0.89 (95% CI, 0.77–1.03), 0.89 (95% CI, 0.80–0.99) and 0.89 (95% CI, 0.80–0.99).
Stratified Analyses
The results of stratified analyses according to sex and age in CVD events and all-cause mortality were present in Tables 3, 4. No significant interaction was observed between age, sex, and trajectories of healthy lifestyle scores (p > 0.050). There was however a negative relationship within the sexes, with high-stable trajectory and CVD incidence was observed in men (HR, 0.74; 95% CI, 0.67–0.83), and not in women (HR, 0.94; 95% CI, 0.13–6.74). Healthy lifestyle score trajectories were identified separately for men and women (Supplementary Figures 2, 3), and similar results were observed (Supplementary Tables 4, 5). A reduced risk of all-cause mortality appeared significant only in participants older than 60 years (HR, 0.82; 95% CI, 0.71–0.95).
Stratified analyses according to overweight/obesity, hypertension, diabetes and dyslipidemia were present in Supplementary Tables 6, 7. No significant interaction was observed between overweight/obesity, hypertension, diabetes and dyslipidemia and trajectories of healthy lifestyle scores either (p > 0.050). According to hypertension and diabetes status, reduced risk of CVD incidence was observed in those with hypertension (HR, 0.73; 95% CI, 0.64–0.82) and without diabetes (HR, 0.71; 95% CI, 0.62–0.80), and marginally in non-hypertensive participants (HR, 0.76; 95% CI, 0.59–1.00), but not in diabetes (HR, 0.83; 95% CI, 0.66–1.04). A reduced risk of all-cause mortality appeared marginally significant only in those without dyslipidemia (HR, 0.87; 95% CI, 0.76–1.00).
To gain deeper insight to the influence of BMI change included by many metrics, we performed a stratified analysis according to BMI change from 2006 to 2010: BMI decreased (<-1 kg/m2); BMI stable (−1–0.9 kg/m2); BMI increased (≥1 kg/m2), present in Table 5. Marginally significant interactions were observed between BMI change and trajectories of healthy lifestyle scores both on CVD and all-cause mortality, suggesting great difference among different BMI change level (p < 0.100). As for CVD, compared with low-stable trajectory, high-stable trajectory appears to negatively correlate with a lower subsequent risk of developing CVD in both BMI decreased (HR, 0.67; 95% CI, 0.55–0.82) and BMI stable group (HR, 0.72; 95% CI, 0.61–0.84). Surprisingly, low-increasing trajectory showed a reduced risk in BMI decreased (HR, 0.73; 95% CI, 0.57–0.93). As for all-cause mortality, apart from high-stable trajectory (HR, 0.83; 95% CI, 0.70–0.97), low-increasing trajectory (HR, 0.80; 95% CI, 0.66–0.96) showed reduced risk of death in BMI stable group. In addition, high-decreasing trajectory in BMI decreased group appeared higher risk of death (HR, 1.14; 95% CI, 1.13–1.83).
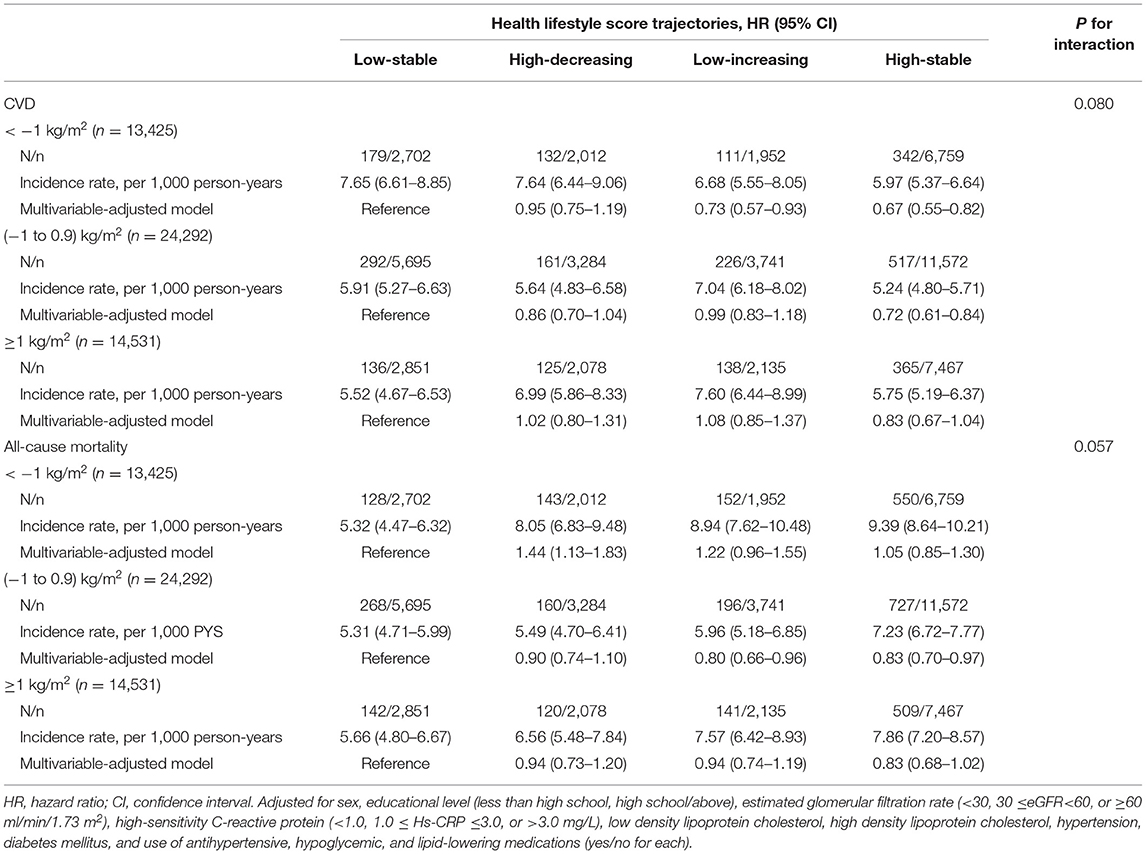
Table 5. Stratified analysis of CVD and all-cause mortality according to BMI change from 2006 to 2010.
Discussion
In this prospective study, four distinct healthy lifestyle trajectories were identified and maintaining a healthy lifestyle over 6 years was associated with a 27% lower risk of CVD incidence and an 11% lower risk of all-cause mortality, when compared with a consistently unhealthy lifestyle. Such correlations are seldom influenced by demographics or disease history, though reduced risk of encountering CVD events was observed only in men and in those without diabetes, while a reduced risk of all-cause mortality was only observed in those older than 60 years and without dyslipidemia. However, such correlations are quite different when considering BMI change. Maintaining a healthy lifestyle appeared to negatively correlate with a lower subsequent risk of developing CVD when BMI decreased or stable and all-cause mortality when BMI was stable. Changing lifestyle to a healthy way was showed a reduced risk of cardiovascular disease only when BMI decreased and all-cause mortality only when BMI was stable.
A previous study based on the same cohort found a protective impact in maintaining good cardiovascular health defined according to the American Heart Association (AHA) guidelines for ischemic and intracerebral hemorrhagic stroke (14). However, these healthy lifestyle patterns have two components i.e., lifestyle and physiological measures, such as BMI. These data could be manifestations of specific medicines or lifestyles, and therefore these combined metrics could confound the effect of lifestyle. Therefore, we focused on lifestyle trajectories and our findings are consistent with previous studies, suggesting long-term maintenance of a healthy lifestyle is associated with decreased CVD risk (HR, 0.73; 95% CI, 0.65–0.81) and all-cause mortality (HR, 0.89; 95% CI, 0.80–0.99). Considering BMI, we found baseline BMI level did not change such a negative relationship. However, when further considering the influence of BMI change, we observed a negative association between a healthy lifestyle and reduced risk of CVD in those with reduced (HR, 0.67; 95% CI, 0.55–0.82) or stable BMI (HR, 0.72; 95% CI, 0.61–0.84). The causal relationship between increased adiposity and the risk of incident CVD events was proved by observational and genetics-driven studies (22). Similarly, we also observed that the high-stable trajectory was associated with lower risk of all-cause mortality in those with stable BMI (HR, 0.83; 95% CI, 0.70–0.97), compared with low-stable trajectory, These results highlighted the importance of the maintenance of BMI in preventing early death, as pronounced weight change, not only weight gain, is associated with an increase in all-cause mortality risk (23, 24).
A shift toward a healthier lifestyle during early life will decrease long-term risk of CVD has been reported by many short- or long-term, prospective interventional studies. For example, we know that those in engaging in a healthy lifestyle intervention is associated with a lower level of risk and therefore a reduction in predicted CVD risk (25–27). However, lifestyle changes which occur across the life course, with the application of interventions are becoming increasingly less likely. Further qualitative research focusing on dynamics of lifestyles and development/aging is needed. Although, two prospective cohorts reported having observed improved lifestyles in the short term could reduce risk of CVD or death, but both studies included BMI (28, 29). However, we did not observe such benefits when focused on lifestyles only, as we did not observe any association between CVD or all-cause mortality risk and the low-increasing trajectory. Similarly, a study in the UK reported a decrease in unhealthy lifestyle scores was not associated with a beneficial effect on mortality (HR, 0.93; 95% CI, 0.83–1.04), either (30). When we further divided participants according to BMI change, a negative relationship between the low-increasing trajectory and CVD incidence was observed in the decreased BMI group (HR, 0.73; 95% CI, 0.57–0.93), and in deaths in the stable BMI group (HR, 0.80; 95% CI, 0.66–0.96). This suggests that the protective effect of improved lifestyles may be gained only when BMI is reduced, which is consistent with previous research (31). However, maintaining BMI, even with a lifestyle changes, is important due to a “U” shaped relationship between BMI and all-cause mortality (24).
After sex, age, overweight/obesity, hypertension, diabetes and dyslipidemia were stratified, we observed a reduced risk in CVD in men. It is acknowledged that men are more likely to have unhealthy lifestyles like smoking and drinking (32), which was also can be observed in our trajectories in men and women, so the benefit would be more obvious in men who maintaining a healthy lifestyle. In another way, women sample in our cohort is relatively limited leading to a small number of outcomes, so it may be hard to see the association. All patients could benefit from healthy lifestyles, but different from the overweight/obesity and hypertension, reduced risk in CVD was only observed in those without diabetes in our study. Maybe for diabetes patients, changing/maintenance of healthy lifestyles was not enough, intensive intervention for glucose control was needed. As for all-cause mortality, we observed reduced risk in those older than 60 years, as they are facing greater death threat and are likely to expose to unhealthy lifestyles for a longer time. A decreased risk was observed between the high-stable trajectory and stroke incidence with an HR of 0.70 (95% CI, 0.62–0.79), but not myocardial infarction (HR, 0.82; 95% CI, 0.64–1.04) after adjusting potential confounders. In our cohort, stroke accounted for about 80% of CVD, so the myocardial infarction incidence was relatively low. This insignificant phenomenon on myocardial infarction was prevalent in Kailuan cohort studies (33, 34). Also, myocardial infarction was all ischemic, but stroke was both ischemic and hemorrhagic. So, the effect may be different depending on whether CVD is either ischemic and hemorrhagic. In this instance, we did not gather detailed information around further classifications and therefore we were unable to analyse this further.
Foundational research has shown that Chinese lifestyles are quite different from global communities. Kim et al. found lifestyles were slightly healthier in China than in the US, especially in terms of diet quality, physical activity, and smoking (35). However, this appears controversial because the Report on Nutrition and Chronic Disease Status of Chinese Residents suggested that unhealthy lifestyles remain prevalent (36). Therefore, it is safe to assume that lifestyles across Chinese populations are incredibly diverse. Zhu et al. found that compared to the differences between urban and rural areas, the differences between project areas are more profound. For example, in Henan, which is a neighboring province to Tangshan, Hebei Province, had relatively healthier lifestyles (32). Therefore, caution must be taken because trajectories can (at best) only represent Hebei region, rather than every region, or indeed China in general.
On that note, before making any recommendations it is important to first discuss the limitations involved. Firstly, our cohort only included Chinese adults from the Kailuan community. As has been mentioned, findings may not be generalizable to other populations but this does provide an opportunity to make both national and international comparisons. Similar associations between individuals and a number of healthy lifestyle metrics and CVD/mortality risks have been shown in multiple racial/ethnic groups, highlighting the broad generalizable nature of the data (28–30). Another limitation is the substitution of daily salt intake for diet quality as detailed dietary components data were not available until 2014 in our cohort. Given that salt intake was consistently found to be associated with higher CVD risk (37) and excessive salt intake is a problem in China, salt intake was used as a surrogate for diet quality.
Conclusion
In this prospective study, four distinct healthy lifestyle score trajectories were identified. Maintaining a healthy lifestyle over 6 years was associated with a 27% lower risk of incident CVD and an 11% lower risk of all-cause mortality, compared with those with a consistently unhealthy lifestyle. Improving lifestyle is likely to reduce risk of CVD when BMI also decreases although the risk of all-cause mortality reduces when BMI remains stable. Our findings highlight the importance of strong public health efforts to improve lifestyle for CVD prevention.
Data Availability Statement
The original contributions presented in the study are included in the article/Supplementary Material, further inquiries can be directed to the corresponding authors.
Ethics Statement
The studies involving human participants were reviewed and approved by the Ethics Committee of the Kailuan General Hospital. The patients/participants provided their written informed consent to participate in this study.
Author Contributions
SW, XiaodY, and YL: designed the study, reviewed and revised the manuscript. XD, WF, and XiaojY: coded and analyzed the data. XD, WF, XY, and SS: wrote the manuscript. SC and GW: collected data. YW and HZ: helped interpret the data. All authors read and approved the final manuscript.
Funding
This study was supported by the Natural Science Foundation of Hebei Province (H2021209018) and Tangshan Science and Technology Innovation Team Program (20130206D).
Conflict of Interest
The authors declare that the research was conducted in the absence of any commercial or financial relationships that could be construed as a potential conflict of interest.
Publisher's Note
All claims expressed in this article are solely those of the authors and do not necessarily represent those of their affiliated organizations, or those of the publisher, the editors and the reviewers. Any product that may be evaluated in this article, or claim that may be made by its manufacturer, is not guaranteed or endorsed by the publisher.
Acknowledgments
The authors thank the investigators and the participants who made this cohort study possible.
Supplementary Material
The Supplementary Material for this article can be found online at: https://www.frontiersin.org/articles/10.3389/fcvm.2021.790497/full#supplementary-material
References
1. Sacco RL, Roth GA, Reddy KS, Arnett DK, Bonita R, Gaziano TA, et al. The Heart of 25 by 25: achieving the goal of reducing global and regional premature deaths from cardiovascular diseases and stroke: a modeling study from the American Heart Association and World Heart Federation. Circulation. (2016) 133:e674–90. doi: 10.1161/CIR.0000000000000395
2. Liu S, Li Y, Zeng X, Wang H, Yin P, Wang L, et al. Burden of cardiovascular diseases in China, 1990-2016: findings from the 2016 Global Burden of Disease Study. JAMA Cardiol. (2019) 4:342–52. doi: 10.1001/jamacardio.2019.0295
3. Zhou M, Wang H, Zhu J, Chen W, Wang L, Liu S, et al. Cause-specific mortality for 240 causes in China during 1990-2013: a systematic subnational analysis for the Global Burden of Disease Study 2013. Lancet. (2016) 387:251–72. doi: 10.1016/S0140-6736(15)00551-6
4. Yusuf S, Joseph P, Rangarajan S, Islam S, Mente A, Hystad P, et al. Modifiable risk factors, cardiovascular disease, and mortality in 155 722 individuals from 21 high-income, middle-income, and low-income countries (PURE): a prospective cohort study. Lancet. (2020) 395:795–808. doi: 10.1016/S0140-6736(19)32008-2
5. Li M, Xu Y, Wan Q, Shen F, Xu M, Zhao Z, et al. Individual and Combined Associations of modifiable lifestyle and metabolic health status with new-onset diabetes and major cardiovascular events: the China Cardiometabolic Disease and Cancer Cohort (4C) Study. Diabetes Care. (2020) 43:1929–36. doi: 10.2337/dc20-0256
6. Barbaresko J, Rienks J, Nöthlings U. Lifestyle indices and cardiovascular disease risk: a meta-analysis. Am J Prev Med. (2018) 55:555–64. doi: 10.1016/j.amepre.2018.04.046
7. Pan XF, Li Y, Franco OH, Yuan JM, Pan A, Koh WP. Impact of combined lifestyle factors on all-cause and cause-specific mortality and life expectancy in Chinese: the Singapore Chinese Health Study. J Gerontol A Biol Sci Med Sci. (2020) 75:2193–9. doi: 10.1093/gerona/glz271
8. Bonaccio M, Di Castelnuovo A, Costanzo S, De Curtis A, Persichillo M, Cerletti C, et al. Impact of combined healthy lifestyle factors on survival in an adult general population and in high-risk groups: prospective results from the Moli-sani Study. J Intern Med. (2019) 286:207–20. doi: 10.1111/joim.12907
9. Shook RP, Halpin K, Carlson JA, Davis A, Dean K, Papa A, et al. Adherence with multiple national healthy lifestyle recommendations in a Large Pediatric Center electronic health record and reduced risk of obesity. Mayo Clin Proc. (2018) 93:1247–55. doi: 10.1016/j.mayocp.2018.04.020
10. Li H, Khor CC, Fan J, Lv J, Yu C, Guo Y, et al. Genetic risk, adherence to a healthy lifestyle, and type 2 diabetes risk among 550,000 Chinese adults: results from 2 independent Asian cohorts. Am J Clin Nutr. (2020) 111:698–707. doi: 10.1093/ajcn/nqz310
11. Lelong H, Blacher J, Baudry J, Adriouch S, Galan P, Fezeu L, et al. Combination of healthy lifestyle factors on the risk of hypertension in a Large Cohort of French Adults. Nutrients. (2019) 11:1687. doi: 10.3390/nu11071687
12. Zheng M, Zhang X, Chen S, Song Y, Zhao Q, Gao X, et al. Arterial stiffness preceding diabetes: a longitudinal study. Circ Res. (2020) 28:317950. doi: 10.1161/CIRCRESAHA.120.317950
13. Wang Z, Zhao X, Chen S, Wang Y, Cao L, Liao W, et al. Associations between nonalcoholic fatty liver disease and cancers in a large cohort in China. Clin Gastroenterol Hepatol. (2020) 11:30643–1. doi: 10.1016/j.cgh.2020.05.009
14. Wu S, An S, Li W, Lichtenstein AH, Gao J, Kris-Etherton PM, et al. Association of trajectory of cardiovascular health score and incident cardiovascular disease. JAMA Netw Open. (2019) 2:e194758. doi: 10.1001/jamanetworkopen.2019.4758
15. China Obesity Working Group. Guidelines for the prevention and control of overweight and obesity in Chinese Adults. Biomed. Environ. Sci. (2004) 17:1–36. doi: 10.3321/j.issn:0512-7955.2004.01.001
16. Chu J, Gao R, Zhao S, Lu G, Zhao D, Li J. Guidelines for the prevention and treatment of dyslipidemia in Chinese adults (In Chinese). Chin. Circ. J. (2016) 31:937–53. doi: 10.3760/j.issn:0253-3758.2007.05.003
17. Wu Z, Jin C, Vaidya A, Jin W, Huang Z, Wu S, et al. Longitudinal patterns of blood pressure, incident cardiovascular events, and all-cause mortality in normotensive diabetic people. Hypertension. (2016) 68:71–7. doi: 10.1161/HYPERTENSIONAHA.116.07381
18. Tunstall-Pedoe H, Kuulasmaa K, Amouyel P, Arveiler D, Rajakangas AM, Pajak A. Myocardial infarction and coronary deaths in the World Health Organization MONICA Project. Registration procedures, event rates, and case-fatality rates in 38 populations from 21 countries in four continents. Circulation. (1994) 590:83–612. doi: 10.1161/01.CIR.90.1.583
19. Recommendations Recommendations on stroke prevention, diagnosis, and therapy. Report of the WHO Task Force on Stroke and other Cerebrovascular Disorders. Stroke. (1989) 20:1407–31. doi: 10.1161/01.STR.20.10.1407
20. Zhang Q, Zhou Y, Gao X, Wang C, Zhang S, Wang A, et al. Ideal cardiovascular health metrics and the risks of ischemic and intracerebral hemorrhagic stroke. Stroke. (2013) 44: 2451–6. doi: 10.1161/STROKEAHA.113.678839
21. Nagin DS, Odgers CL. Group-based trajectory modeling in clinical research. Annu Rev Clin Psychol. (2010) 6:109–38. doi: 10.1146/annurev.clinpsy.121208.131413
22. Kim MS, Kim WJ, Khera AV, Kim JY, Yon DK, Lee SW, et al. Association between adiposity and cardiovascular outcomes: an umbrella review and meta-analysis of observational and Mendelian randomization studies. Eur Heart J. (2021) 42:3388–403. doi: 10.1093/eurheartj/ehab454
23. Lee YB, Kim B, Park J, Kim M, Choi MS, Kim G, et al. Early mortality and cardiovascular disease, varied association with body mass index and its changes in insulin-treated diabetes: a nationwide study. Int J Obes (Lond). (2021) 45:2482–9. doi: 10.1038/s41366-021-00922-2
24. Xing Z, Pei J, Huang J, Peng X, Chen P, Hu X, et al. Weight change is associated with increased all-cause mortality and non-cardiac mortality among patients with type 2 diabetes mellitus. Endocrine. (2019) 64:82–9. doi: 10.1007/s12020-019-01892-2
25. Khare MM, Cursio JF, Locklin CA, Bates NJ, Loo RK. Lifestyle intervention and cardiovascular disease risk reduction in low-income Hispanic immigrant women participating in the Illinois WISEWOMAN program. J Community Health. (2014) 39:737–46. doi: 10.1007/s10900-014-9820-3
26. Lotfaliany M, Sathish T, Shaw J, Thomas E, Tapp RJ, Kapoor N, et al. Effects of a lifestyle intervention on cardiovascular risk among high-risk individuals for diabetes in a low- and middle-income setting: Secondary analysis of the Kerala Diabetes Prevention Program. Prev Med. (2020) 139:106068. doi: 10.1016/j.ypmed.2020.106068
27. Lönnberg L, Ekblom-Bak E, Damberg M. Reduced 10-year risk of developing cardiovascular disease after participating in a lifestyle programme in primary care. Ups J Med Sci. (2020) 125:250–6. doi: 10.1080/03009734.2020.1726533
28. Hulsegge G, Looman M, Smit HA, Daviglus ML, van der Schouw YT, Verschuren WM. Lifestyle changes in young adulthood and middle age and risk of cardiovascular disease and all-cause mortality: the Doetinchem Cohort Study. J Am Heart Assoc. (2016) 5:e002432. doi: 10.1161/JAHA.115.002432
29. Berstad P, Botteri E, Larsen I, Løberg M, Kalager M, Holme Ø, et al. Lifestyle changes at middle age and mortality: a population-based prospective cohort study. J Epidemiol Community Health. (2017) 71:59–66. doi: 10.1136/jech-2015-206760
30. White J, Greene G, Kivimaki M, Batty G. Association between changes in lifestyle and all-cause mortality: the Health and Lifestyle Survey. J Epidemiol Community Health. (2018) 72:711–4. doi: 10.1136/jech-2017-210363
31. Honda T, Ishida Y, Oda M, Noguchi K, Chen S, Sakata S, et al. Changes in body weight and concurrent changes in cardiovascular risk profiles in community residents in Japan: the Hisayama Study. J Atheroscler Thromb. (2021). doi: 10.5551/jat.59394. [Epub ahead of print].
32. Zhu NB, Zhou M, Yu CQ, Guo Y, Bian Z, Tan YL, et al. Prevalence of “healthy lifestyle” in Chinese adults. Zhonghua Liu Xing Bing Xue Za Zhi. (2019) 40:136–41. doi: 10.3760/cma.j.issn.0254-6450.2019.02.003
33. Wang L, Lee Y, Wu Y, Zhang X, Jin C, Huang Z, et al. A prospective study of waist circumference trajectories and incident cardiovascular disease in China: the Kailuan Cohort Study. Am J Clin Nutr. (2021) 113:338–47. doi: 10.1093/ajcn/nqaa331
34. Lu X, Niu X, Shen C, Liu F, Liu Z, Huang K, et al. Development and validation of a polygenic risk score for stroke in the Chinese Population. Neurology. (2021) 97:e619–28. doi: 10.1212/WNL.0000000000012263
35. Kim S, Popkin BM, Siega-Riz AM, Haines PS, Arab L. A cross-national comparison of lifestyle between China and the United States, using a comprehensive cross-national measurement tool of the healthfulness of lifestyles: the Lifestyle Index. Prev Med. (2004) 38:160–71. doi: 10.1016/j.ypmed.2003.09.028
36. Yang L, Ding G. Report on Nutrition and Chronic Disease Status of Chinese Residents. Ying Yang Xue Bao. (2020) 42:521. doi: 10.13325/j.cnki.acta.nutr.sin.2020.06.002
Keywords: lifestyle, cardiovascular disease, all-cause mortality, trajectory, BMI change, cohort
Citation: Ding X, Fang W, Yuan X, Seery S, Wu Y, Chen S, Zhou H, Wang G, Li Y, Yuan X and Wu S (2021) Associations Between Healthy Lifestyle Trajectories and the Incidence of Cardiovascular Disease With All-Cause Mortality: A Large, Prospective, Chinese Cohort Study. Front. Cardiovasc. Med. 8:790497. doi: 10.3389/fcvm.2021.790497
Received: 06 October 2021; Accepted: 29 November 2021;
Published: 20 December 2021.
Edited by:
Gen-Min Lin, Hualien Armed Forces General Hospital, TaiwanReviewed by:
Asiiat Alieva, Almazov National Medical Research Centre, RussiaIrene R. Degano, Centro de Investigación Biomédica en Red en Enfermedades Cardiovasculares (CIBERCV), Spain
Copyright © 2021 Ding, Fang, Yuan, Seery, Wu, Chen, Zhou, Wang, Li, Yuan and Wu. This is an open-access article distributed under the terms of the Creative Commons Attribution License (CC BY). The use, distribution or reproduction in other forums is permitted, provided the original author(s) and the copyright owner(s) are credited and that the original publication in this journal is cited, in accordance with accepted academic practice. No use, distribution or reproduction is permitted which does not comply with these terms.
*Correspondence: Shouling Wu, ZHJ3dXNsQDE2My5jb20=; Xiaodong Yuan, eXhkNjlAc29odS5jb20=; Yun Li, bGl5dW44MDIyQDE2My5jb20=
†These authors have contributed equally to this work and share first authorship