- 1School of Biomedical Engineering and Imaging Sciences, King's College London, London, United Kingdom
- 2Leeds Institute of Cardiovascular and Metabolic Medicine, University of Leeds, Leeds, United Kingdom
Functionally significant coronary artery disease impairs myocardial blood flow and can be detected non-invasively by myocardial perfusion imaging. While multiple myocardial perfusion imaging modalities exist, the high spatial and temporal resolution of cardiovascular magnetic resonance (CMR), combined with its freedom from ionising radiation make it an attractive option. Dynamic contrast enhanced CMR perfusion imaging has become a well-validated non-invasive tool for the assessment and risk stratification of patients with coronary artery disease and is recommended by international guidelines. This article presents an overview of CMR perfusion imaging and its clinical application, with a focus on chronic coronary syndromes, highlighting its strengths and challenges, and discusses recent advances, including the emerging role of quantitative perfusion analysis.
Introduction
Myocardial perfusion imaging (MPI) plays a central role in the diagnosis, management, and risk stratification of patients with coronary artery disease (CAD) and is recommended by international guidelines (1). Unlike angiographic imaging, which provides anatomical data on the patency of major epicardial coronary arteries, MPI offers information on the downstream effects of epicardial coronary stenoses, as well as the function of the coronary microcirculation (2). Whilst there are a several non-invasive imaging modalities capable of MPI, cardiovascular magnetic resonance (CMR) is unique in its ability to provide high-resolution myocardial perfusion data alongside global and regional biventricular function, assessment of myocardial infarction, and without need for ionising radiation. This article presents an overview of the CMR method of dynamic contrast enhanced (DCE) perfusion imaging.
Dynamic Contrast Enhanced Perfusion Imaging by CMR
DCE perfusion imaging is designed to track and display the first passage of a contrast agent (CA) bolus through the myocardium during maximal coronary vasodilation, and often during resting conditions (Figure 1) (3).
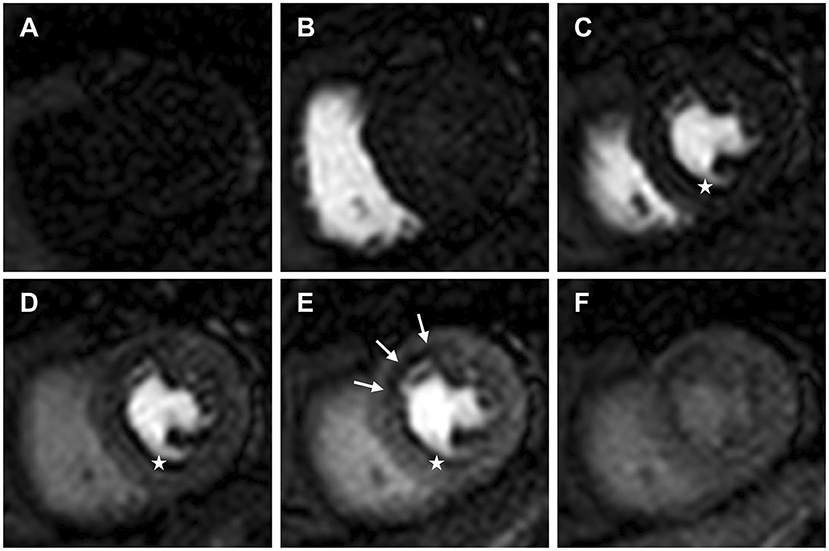
Figure 1. Dynamic contrast enhanced perfusion CMR tracks and displays the first passage of an injected contrast agent bolus through the heart. Mid ventricular slice (A) pre-contrast arrival; (B) contrast arrival in the right ventricle; (C) contrast arrival in the left ventricle (LV); (D) contrast arrival in the LV myocardium; (E) maximal myocardial contrast between remote myocardium and the subendocardial region of relative hypoperfusion (white arrows); (F) second pass and redistribution of the contrast agent. White stars identify a dark rim artefact, which can be seen on arrival of contrast in the LV prior to myocardial contrast enhancement.
The technique relies upon the heterogeneity of contrast perfusion in myocardium supplied by obstructed vs. unobstructed coronary arteries. Flow limiting stenoses blunt the augmentation of myocardial perfusion during hyperaemia, manifesting as a relative perfusion defect at stress, which is not seen at rest, compared with myocardium subtended by unobstructed coronary arteries (Figure 2) (4). Maximal coronary vasodilation is typically achieved with an intravenous adenosine infusion or a bolus injection of the adenosine receptor agonist regadenoson. Both adenosine and regadenoson produce coronary vasodilatation by their agonistic action on the A2a receptors found in coronary smooth muscle and endothelial cells, inducing hyperpolerization of smooth muscle and release of nitric oxide (5). The phosphodiesterase enzyme inhibitor dipyridamole can also be used to induce coronary vasodilation. Dipyridamole inhibits cyclic adenosine monophosphate degradation and blocks cellular reuptake of adenosine, thereby increasing the circulating concentration of endogenous adenosine (6).
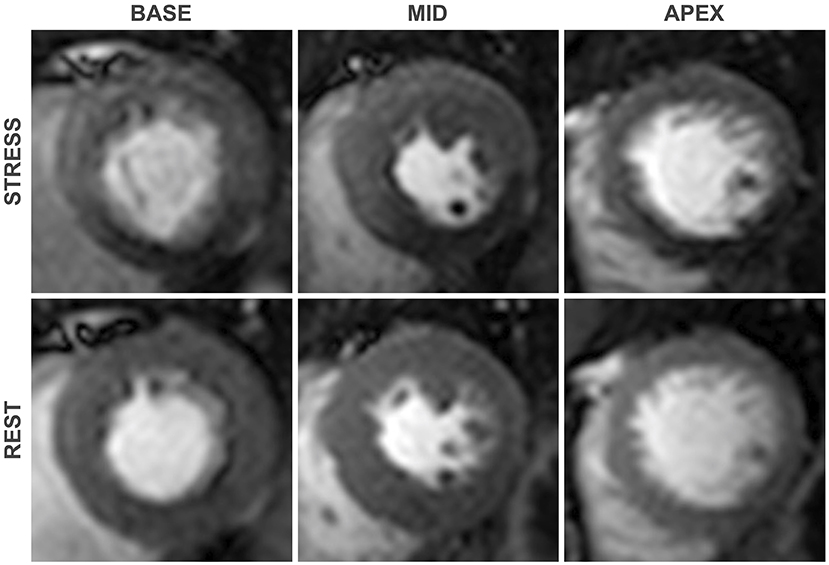
Figure 2. First-pass perfusion images at pharmacologically induced stress (top) and at rest (bottom). An inducible perfusion defect is seen in the Inferior and inferoseptal segments when compared with the remote segments, consistent with flow limiting disease in the right coronary artery. As is typical of perfusion defects secondary to obstructive coronary stenoses, contrast hypoenhancement is most pronounced in the subendocardial region.
Basic Principles
The clinical feasibility of tracking the first-pass of contrast through the myocardium with CMR was first demonstrated by Atkinson et al. in 1990 who used an inversion recovery gradient echo (GRE) sequence to track the first-pass of CA through rodent and human hearts (2, 7). Following an intravenous injection of CA, multiple (typically 3 short-axis) images of the heart are acquired, each with a different anatomical location and cardiac phase, which remain constant across sequential cardiac cycles (8). On arrival of the CA to the myocardium, the paramagnetic gadolinium chelate interacts with water molecules within the extracellular space, reducing T1 relaxation times and thus increasing signal intensity on a T1 weighted image. Areas of relative hypoperfusion therefore appear hypointense in comparison to well-perfused myocardium (4). In contemporary perfusion sequences, T1-weighting of images is typically achieved by use of a 90° saturation recovery (SR) radiofrequency pulse. A 180° inversion recovery pulse can generate greater contrast between normal and hypoperfused myocardium but is limited by longer imaging times and sensitivity to heart rate variations and miss-triggers, which can result in incomplete magnetisation recovery and signal intensity variation. Thus, saturation-prepared sequences are the current standard (2, 4). Preparation pulses are typically non-selective in order to reduce myocardial sensitivity to through-plane motion as well as achieve uniform contrast enhancement in the left ventricular (LV) blood pool. Whilst use of a single shared SR preparation offers increased efficiency, typically, separate SR preparations are used for each imaging slice to ensure uniform image quality (4).
To ensure adequate coverage of the 16 standard AHA segments, guidelines recommend a minimum acquisition of 3 short-axis myocardial slices, in addition to a minimum spatial resolution of 3 × 3 mm (3, 9). In order to accurately display changes in signal intensity over time, imaging should ideally be acquired for consecutive R-R intervals during the first passage of the contrast bolus (3). During pharmacologically induced stress, the R-R interval can be short and thus rapid data acquisition is needed to meet these high spatial and temporal demands. A standard spatial resolution of 2–3 mm can be achieved with a fast read-out sequence, such as fast GRE, balanced steady state free procession (bSSFP) or hybrid echo planar imaging, combined with spatial under-sampling (2). Increasing data sampling speeds further can be used to achieve even higher in-plane spatial resolution (1–2 mm) and/or greater spatial coverage. Conventional spatial-under-sampling techniques, such as parallel imaging, are limited to ~2-fold acceleration owing to a significant signal to noise (SNR) penalty above this acceleration level. However, under-sampling in both the spatial and-temporal domain can significantly increase data acquisition speed without compromising on either SNR or temporal resolution (10). Higher in-plane spatial resolution (1–2 mm) can reduce endocardial dark-rim artefact and improve the image quality and diagnostic accuracy for the detection of CAD including in single-vessel and multivessel disease (10, 11). If the spatio-temporal acceleration is used to increase spatial coverage, additional short-axis myocardial slices or even 3-dimensional (3D) myocardial perfusion data for whole-heart coverage can be acquired. 3D perfusion CMR is highly accurate to detect CAD as defined by invasive coronary physiology, however, any clinical benefit over conventional 3 short-axis high-resolution myocardial slices remains unclear (12, 13). Use of multiband radiofrequency pulses for simultaneous multi-slice data acquisition has been proposed as a strategy to increase spatial coverage whilst maintaining in-plane spatial resolution, however, this method still awaits clinical validation in patients with CAD (14).
In the clinical setting, perfusion CMR is performed at either 1.5 Tesla (T) or 3T field strengths, with 1.5T being more widely available. A major advantage of perfusion CMR at 3T is the superior SNR that can be obtained. The higher field strength also improves contrast enhancement, and importantly, offers improved diagnostic accuracy for the detection of single vessel and multivessel CAD (15, 16). Despite the overall image quality being superior at 3T (10), the associated increased field inhomogeneities heighten the sensitivity to susceptibility artefacts (17). Use of a GRE readout as opposed to a bSSFP readout is therefore preferred at 3T to minimise this undesirable consequence (18).
Artefacts
An in-depth review of imaging artefacts is beyond the scope of this review, however, two types of artefact are of particular importance in DCE perfusion CMR imaging and warrant brief discussion. Despite high data acquisition speeds, DCE perfusion imaging is susceptible to both in-plane and through-plane motion, which can be cardiac or respiratory related, and results in image artefacts (4, 19). This can be exacerbated by a high respiratory rate during pharmacologically induced stress. In-plane motion can be reliably corrected in-line after data acquisition (20), however, through-plane motion remains problematic and highlights the importance of patient education and focus on gentle controlled breathing during the acquisition of stress images. Another important artefact in DCE perfusion CMR imaging is the subendocardial dark-rim artefact. There are several causes of dark-rim artefacts, the most common being Gibbs ringing, cardiac motion and magnetic field inhomogeneities resulting from the strong paramagnetic properties of a gadolinium based CA arriving in the heart (19). The dark-rim artefact is of particular importance as it can mimic inducible perfusion defects and reduce diagnostic accuracy. However, dark-rim artefacts do have features which enable an experienced reader to differentiate them from true perfusion defects. Typically, unlike true inducible perfusion defects, dark-rim artefacts appear on arrival of contrast in the LV blood pool, lead to a signal reduction compared with baseline (pre-contrast), are usually only one pixel wide, and most frequently appear in the phase encoding direction (21). An example of a dark-rim artefact is shown in Figure 1.
Qualitative Stress Perfusion CMR
Qualitative stress perfusion CMR is one of the most robust non-invasive methods for the detection of CAD (22). The visual assessment of myocardial contrast enhancement during the first-passage of CA enables detection of regions of relative hypoperfusion. Comparison of myocardial perfusion is made between endocardial and epicardial regions as well as between myocardial segments. Significant inducible perfusion defects are more severe at the subendocardium, appear on the arrival of CA to the myocardium, are more than 2 pixels wide, and must persist beyond the peak myocardial enhancement. Furthermore, for a perfusion defect to be significant for ischemia, it should be present during stress but not at rest (if available) and, in the context of CAD, have a distribution consistent with one or more coronary territories (21). A transmural gradient of perfusion, with more severe hypoperfusion in the subendocardial layers, is usually observed in the involved segments (21, 23). Perfusion imaging is read alongside the corresponding late gadolinium enhancement (LGE) imaging, with matching LGE-perfusion defects being considered negative for inducible ischemia (21, 24).
There are numerous single centre and multi-centre studies demonstrating the accuracy of qualitative stress perfusion CMR. In a large meta-analysis by Jaarsma et al. data was pooled from 22 studies that evaluated qualitative stress perfusion CMR against anatomical luminal stenosis on invasive coronary angiography (ICA) and found a patient level sensitivity and specificity of 90 and 74%, respectively (25). Using invasive fraction flow reserve (FFR) as the reference standard, a more recent meta-analysis by Kiaos et al. pooled 6 studies and found sensitivity and specificity of 90 and 85%, respectively (26). More important for guiding revascularisation decision making is the ability of stress perfusion CMR to accurately detect ischemia at the level of the perfusion territory. In 2013 Ebersberger et al. evaluated 116 patients with suspected or known CAD with qualitative stress perfusion CMR at 3 Tesla and found a high diagnostic accuracy for detecting diseased vessels (defined by invasive coronary angiography with FFR) with an area under the receiver operator characteristic curve (AUC) of 0.93, sensitivity of 89% and specificity of 95% (27). Similar high diagnostic accuracies have also been reported by others (23, 28, 29).
The accuracy of qualitative stress perfusion CMR has been extensively compared with other non-invasive MPI modalities. In 2008, the MR-IMPACT study was the first multicentre multivendor study to demonstrate non-inferiority of stress perfusion CMR to the then clinical standard single-photon emission computerised tomography (SPECT) for the detection of CAD in 42 patients against coronary angiography (lumen stenosis > 50%) with AUCs of 0.86 and 0.75 for CMR and SPECT, respectively (30). The subsequent larger MR-IMPACT II study with 515 patients across 33 centres found superior sensitivity of perfusion CMR over SPECT (0.67 vs. 0.59) but inferior specificity (0.61 vs. 0.72) (31). In 2011 the single centre CE-MARC trial was the first large scale prospective comparison of CMR vs. SPECT for the detection of CAD on ICA (>70% stenosis) and found superior sensitivities (87 vs. 67%) and similar specificities (83 vs. 83%) for CMR vs. SPECT (32, 33). In 2015, a meta-analysis by Takx et al. compared the diagnostic accuracy of perfusion CMR with other non-invasive MPI techniques and found perfusion CMR had a similarly high diagnostic performance to positron emission tomography (PET) and computerised tomography (CT) perfusion and superior performance to SPECT and myocardial contrast echocardiography. This analysis, which included 15 perfusion CMR studies with 798 patients, found a pooled sensitivity and specificity for perfusion CMR of 0.89 and 0.87, respectively, at the patient level and 0.87 and 0.91 at the vessel level (22). It is noteworthy that this meta-analysis included CMR studies analysed qualitatively as well as quantitatively.
Multiple retrospective and prospective trials have demonstrated the prognostic value of qualitative stress perfusion CMR for risk stratification of patients with known or suspected CAD (34, 35). A large meta-analysis of 15 pooled studies evaluating 7,606 patients with known or suspected CAD undergoing stress perfusion CMR found a positive stress perfusion CMR was associated with an annualised event rate of 4.9% compared with only 0.9% in those with a negative study (35). Consistent with this, and its high diagnostic performance, stress perfusion CMR is an effective gatekeeper to invasive evaluation and management of patients with angina. In 2016, the CE-MARC II trial in patients with suspected angina found initial investigation by CMR resulted in a lower probability of unnecessary invasive coronary angiography than the since updated UK National Institute for Health and Care Excellence (NICE) guidelines–directed care, with no increase in adverse events (36). More recently, the multi-centre MR-INFORM trial demonstrated stress perfusion CMR can be used with the same efficacy and safety as invasive FFR in the initial management of patients with stable angina and risk factors for CAD (37). The study randomly assigned 918 patients to either a perfusion CMR scan or invasive coronary angiography with FFR, and found non-inferiority of the CMR strategy for the composite primary outcome of death, non-fatal myocardial infarction, or target-vessel revascularization within 1 year. In addition, the CMR based strategy was associated with a lower incidence of coronary revascularisation. Using stress perfusion CMR as a gate-keeper prior to ICA is also a cost effective approach in patients at intermediate risk of obstructive CAD (38).
Challenges of Qualitative Stress Perfusion CMR
In addition to its strengths, qualitative stress perfusion CMR also has its challenges:
(1) Operator training - Perfusion data interpretation is subjective and as such is dependent on operator training and experience. The high diagnostic accuracies reported in the literature mostly come from experienced academic CMR centres with expert readers. A study from Villa et al. demonstrated that the level of reader training is the main determinant of diagnostic accuracy in the identification of CAD. They found Level 3 readers to have an 83.6% diagnostic accuracy compared with 65.7 and 55.7% for level 2 and level 1 readers, respectively (39).
(2) Balanced ischemia, multi-vessel disease and ischemic burden - Evidence of inducible myocardial ischemia is associated with adverse prognosis (35). Furthermore, prognosis worsens as the ischemic burden increases, and only revascularisation of flow-limiting coronary stenoses is associated with improved outcomes (40, 41). Therefore, accurate detection and quantification of the myocardial ischemic burden is of paramount importance when stratifying patient risk and considering coronary revascularisation. As aforementioned, qualitative stress perfusion CMR relies upon the heterogeneity of myocardial contrast perfusion associated with the presence or absence of flow-limiting coronary stenoses. In situations of global ischemia, such as three vessel disease (3VD) or microvascular dysfunction (MVD), there is often an absence of normally perfused reference myocardium and hence qualitative assessment can be challenging. The detrimental impact of “balanced ischemia” on diagnostic accuracy is well-documented in SPECT with up to 20% of patients with 3VD being incorrectly reported as normal (42), and as few as 29% of patients having perfusion defects reported in all coronary territories despite angiographically proven 3VD (43). Perfusion CMR has superior spatial resolution to SPECT (typically 1.5–3 vs. 12–15 mm) and is able to overcome this limitation to some extent, however, the diagnostic accuracy of qualitative assessment remains lower in patients with multivessel CAD and MVD (44–47). Kotecha et al. reported a diagnostic accuracy for qualitative stress perfusion CMR at 1.5 Tesla of 40 and 48% for correct classification of 3-vessel and 2-vessel CAD, respectively, as proven with invasive coronary angiography and FFR (48). Using a high-resolution perfusion CMR sequence, Motwani et al. demonstrated a diagnostic accuracy of 57% for detecting perfusion defects in all coronary territories in patients with angiographically proven 3VD (49). Rahman et al. recently reported a qualitative stress perfusion CMR sensitivity of 41% and specificity of 83% with an AUC of 0.60 to detect MVD defined by invasive physiology (44).
(3) Prior coronary artery bypass grafting (CABG) - Evaluation of myocardial perfusion in patients with prior CABG is challenging. These patients frequently have complex multivessel disease, established myocardial infarction, and extensive collateralisation (50, 51). Increased contrast dispersion, delayed contrast arrival at the myocardium (52), and the variability of flow dynamics associated with bypass grafts (53) add to the complexity and likely contribute to the reduced diagnostic accuracy of qualitative perfusion CMR compared with patients without prior CABG (24, 50). In the largest study to date of 110 patients with prior CABG (and 236 with previous percutaneous coronary intervention (PCI)), Bernhardt et al. reported a sensitivity and specificity of 73 and 77%, respectively, for detecting obstructive angiographic disease in patients with prior CABG, while in patients with previous PCI, a sensitivity and specificity of 88 and 90% were reported (24).
Quantitative Perfusion CMR
Analysis of myocardial and LV signal intensity (SI)-time curves from DCE perfusion CMR enables quantitative and semi-quantitative analysis of myocardial perfusion, which has been proposed to offer a solution to some of the challenges of qualitative assessment (54).
Semi-Quantitative Myocardial Perfusion CMR
Whilst the field is moving toward absolute perfusion quantification, prior to the recent technical developments that made full quantification of myocardial perfusion possible, various semi-quantitative measures of myocardial perfusion were proposed. These methods describe the characteristics of the myocardial SI-time curves without attempting to estimate absolute myocardial blood flow (MBF). The most commonly used of these is the maximal myocardial “upslope” parameter, but others including “upslope integral ratio,” “contrast enhancement ratio (CER),” and the “time to peak” have also been evaluated (Figure 3) (21, 54, 55). Since myocardial perfusion is driven by systemic arterial perfusion, semi-quantitative parameters are dependent upon the underlying haemodynamic conditions and can be normalised to enable comparison of rest and stress values, as well as a comparison between individuals (54).
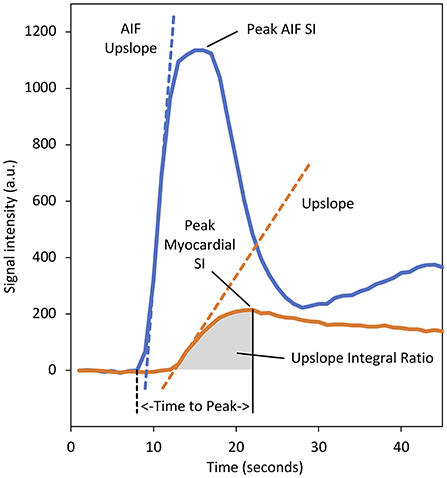
Figure 3. Baseline corrected signal intensity (SI)–time curves for myocardial tissue (orange) and the arterial input function (AIF) (blue) sampled from the left ventricular (LV) blood-pool. Various semi-quantitative perfusion parameters are demonstrated. The dashed orange line represents the “upslope” parameter and denotes the maximal rate of myocardial contrast enhancement. Division of the myocardial upslope by the equivalent AIF upslope (dashed blue line) defines the “upslope index,” which normalises for the haemodynamic conditions and enables comparison between stress and rest, and calculation of a myocardial perfusion reserve index. The area under the myocardial tissue curve from the arrival of contrast at the myocardium to the time of peak enhancement defines the “upslope integral ratio.” The area under the myocardial curve from contrast arrival to the time of peak AIF enhancement has also been used to define this parameter. The “time to peak” myocardial enhancement is measured from the arrival of contrast in the LV blood pool (dashed black line) to the time of peak myocardial enhancement. The “contrast enhancement ratio” parameter is not displayed as this requires an uncalibrated baseline (defined as SIpeak−SIbaseline)/SIbaseline (55). a.u., arbitrary units.
Underlying haemodynamic conditions are partially reflected by the shape of the arterial input, which can be measured from the LV blood pool. Division of the myocardial parameter by the equivalent arterial input function (AIF) parameter serves to normalise perfusion parameters and defines a perfusion index (PI). The ratio of the normalised stress and rest perfusion indices defines a myocardial perfusion reserve index (MPRI), a non-invasive surrogate for coronary flow reserve (CFR) and an index of the functional severity of a coronary lesion (54, 56, 57). It is noteworthy that this method of PI normalisation represents a heuristic approach, and one which has been shown to under-estimate perfusion reserve when compared against microspheres. A more accurate MPRI requires the PI to be normalised by the analogous AIF parameter as well as the time delay between the foot of the tissue curve and peak tissue enhancement (58).
Perfusion dyssynchrony has previously been proposed for the identification of hemodynamically significant CAD, as defined by FFR, and is based on the analysis of the variance of the time to peak across the LV myocardium (59).
Semi-quantitative parameters have been validated against microspheres and coronary angiography for detection of CAD with a high diagnostic accuracy (60–62). A recent meta-analysis of 6 studies using semi-quantitative analyses of myocardial perfusion at the territory level found pooled sensitivity of 77% and specificity of 84% (63). However, there are a number of drawbacks to semi-quantitative perfusion reserve indices:
(1) The only modest gains over qualitative assessment come at the expense of a significant time penalty necessary for processing the perfusion data (64).
(2) When validated against microspheres, semi-quantitative parameters underestimate MBF at flow rates above 1.5 ml/g/min. This can cause underestimation of PIs and MPRI in healthy myocardium where typical stress MBF rates exceed 2 ml/g/min (55).
(3) MPRI from different semi-quantitative perfusion parameters tend to have different thresholds to identify myocardial ischemia and their magnitude cannot be directly compared to that of an invasively measured coronary flow reserve (54).
(4) MPRI requires the acquisition of DCE perfusion imaging during stress and rest, partially conflicting with current international imaging guidelines, which are moving away from the routine acquisition of rest imaging in a quest to reduce CMR scan duration (3).
(5) MPRI is unable to distinguish between a state of globally reduced stress MBF with normal resting MBF (for example multivessel epicardial coronary disease), and a state of globally preserved stress MBF but increased resting MBF (for example hypertension or aortic stenosis). Both clinical scenarios result in a diminished global MPRI. Only by quantifying absolute MBF in millilitres per gramme of myocardium per minute (ml/g/min) during rest and maximal hyperaemia can we differentiate these two very different clinical scenarios (54).
Quantitative Myocardial Perfusion CMR
Measurement of absolute MBF in ml/g/min is possible through the application of tracer-kinetic models to the perfusion data. Myocardial perfusion reserve (MPR), a useful indicator of the significance of coronary artery stenoses, is defined as the ratio of MBF at stress and rest (57).
Several different methodologies for perfusion quantification have been developed. The technical aspects of the various approaches are beyond the scope of our review, however, in brief the methods can be broadly divided into two distinct groups; “tracer-kinetic model dependent” and “tracer-kinetic model independent” (54). Model-dependent methods, of which there are numerous, make the assumption that the tissue structures can be divided into distinct compartments, typically an intravascular and interstitial compartment, and use complex mathematical equations to describe the contrast exchange occurring between these compartments. Tracer-kinetic models are applied to perfusion data beyond the first pass of contrast and can infer knowledge on the permeability surface area product and intravascular volumes in addition to MBF (54). The accuracy of the measurements is dependent on assumptions made with respect to parameters such as signal saturation, relaxivity of contrast agent, baseline T1 and T2 values in the myocardium and blood, homogeneity of the magnetic and RF excitation fields, blood heamatocrit and others (65).
Tracer-kinetic model-independent approaches are centred around the central volume principle, which dictates that MBF can be measured from knowledge of the contrast transit times through the vascular system (66). This value can be estimated from the transfer function, obtained by normalising the myocardial SI curves by the AIF curve using a deconvolution operation. The initial amplitude of the transfer function is proportional to MBF (67). The transfer function is, in practise, obtained using a forward modelling approach that involves the use of different mathematical models and data fitting procedures (67). One of the most widely used and validated deconvolution technique employs the use of a Fermi function to constrain the transfer function to fit the likely behaviour of an intravascular tracer (54, 67).
Quantitative perfusion (QP) measurements require the existence of a linear relationship between CA concentration and measured SI. However, the relationship between these parameters becomes non-linear at higher contrast concentrations due to saturation of the T1-weighted contrast enhancement and T2* effects (68, 69). This phenomenon is more frequently observed in the AIF where CA concentration is highest. Any underestimation of the AIF will result in an overestimation of MBF with a magnitude relative to the magnitude of the saturation effect (54). Signal saturation must therefore be avoided or corrected for accurate MBF measurements. Use of a lower CA dose can avoid signal saturation in the blood pool but would provide inadequate contrast-to-noise for myocardial assessment. Proposed solutions include; (1) a dual-bolus acquisition; (2) a dual-sequence acquisition; and (3) retrospective correction using calibration curves (54). Whilst calibration curves can be generated from knowledge of the sequence parameters and the pre-contrast T1 measurements, this is a somewhat cumbersome approach to correct blood-pool signal saturation, and minor changes in the perfusion sequence parameters can necessitate the need for re-calibration (70). The dual bolus approach measures the AIF from a dilute CA pre-bolus, which maintains the linearity of SI to CA concentration in the blood pool. The pre-bolus is followed by a neat CA bolus for myocardial assessment (71, 72). As the dilution ratio is known, the AIF SI-time curve can be rescaled (and time shifted) prior to perfusion quantification (54). This technique has been validated against microspheres for measurement of MBF (55, 73) and has clinical validation against PET and invasive FFR for the detection of flow limiting CAD (23, 74, 75). However, clinical implementation of the dual bolus technique is onerous owing to the need for multiple injections and longer sequence acquisition times (72).
A dual sequence acquisition uses a single bolus of neat contrast but acquires an addition imaging slice with a short saturation delay, low resolution and reduced T1-weighting (69). This low resolution slice enables measurement of the AIF without blood pool signal saturation. Higher resolution perfusion slices are acquired following the low-resolution image and during the same R-R interval, permitting myocardial and AIF assessment within the same cardiac cycle (69, 76, 77). When a dual sequence approach is employed, and prior to quantification of MBF, SI-time curves must be converted to correspond to CA concentrations (69). A comparison of MBF estimates in pigs found a good correlation between dual-bolus and dual-sequence methods (77). The dual sequence method has been validated against invasive coronary physiology and PET, and is becoming the method of choice for quantitative CMR perfusion owing to its easier integration within the clinical workflow (78, 79).
Another important consideration prior to perfusion quantification is the intrinsic spatial variations in receive-coil sensitivity, which can produce SI variation across the myocardium and lead to inaccuracies when quantifying MBF. This can be corrected for by acquisition of proton-density weighted maps. Alternatively, coil sensitivity can be estimated from pre-contrast images and corrected for by dividing the myocardial signal by its pre-contrast value (54).
Automation of Perfusion Quantification by CMR
The quantification process is complex and requires multiple data processing steps (Figure 4). Perfusion images must first be reconstructed from the raw data. The dynamic image series require correction for respiratory motion in addition to correction for coil sensitivity bias (80, 81). Segmentation of the left ventricle and myocardium is required to enable extraction of the AIF and myocardial tissue curves. A point of reference, typically the superior RV insertion point, must be identified to enable standardised AHA cardiac segmentation (9). If a dual sequence approach has been employed, SI data requires conversion to CA concentration. Only at this stage are quantification models applied to the perfusion data to calculate MBF (81). Until recently, these multiple processing steps required time-consuming and laborious manual input, which restricted the application of quantitative perfusion CMR from mainstream clinical practise. However, recent developments in quantification pipelines now enables full automation of the quantification process and generation of pixel-wise perfusion maps either offline, or in-line and within minutes of data acquisition (79).
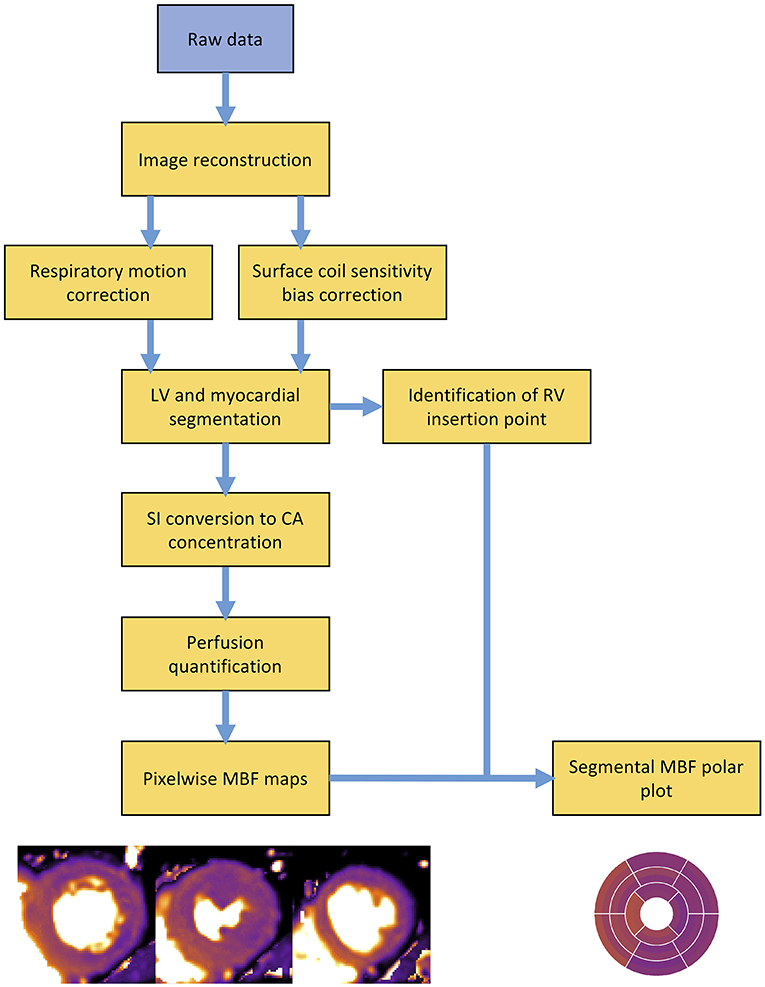
Figure 4. Overview schematic outlining the processing steps involved in a typical perfusion quantification pipeline. LV, left ventricle; RV, right ventricle; SI, signal intensity; CA, contrast agent; MBF, myocardial blood flow.
In 2017, Kellman et al. presented a fully automated quantification pipeline, utilising a dual-sequence approach for derivation of the AIF and a blood tissue exchange model for quantification of MBF. All reconstruction and processing steps were implemented in-line within the opensource Gadgetron software framework and pixel-wise perfusion maps were output within minutes of data acquisition (69, 82). In the same year this approach was validated against PET with good agreement between perfusion values (78), and later demonstrated good repeatability in a study of healthy volunteers, with within subject coefficient of variations between 8 and 12% for both rest and stress MBF measurements (20). In 2019, Kotecha et al. validated the same automated perfusion quantification pipeline against invasive coronary physiology and found high diagnostic accuracy with an AUC of 0.90 for detecting of functionally significant epicardial coronary disease (79). Knott et al. recently demonstrated a strong prognostic value of the same automated pipeline (83). Other fully automated perfusion quantification pipelines have since been developed. Using a two-compartment exchange model for perfusion quantification, Scannell et al. demonstrated highly accurate MBF values from an automated deep-learning based pre-processing pipeline when compared with manual pre-processing (84). Using model-constrained deconvolution, Hsu et al. demonstrated excellent correlation between perfusion values from fully automated and manual processing pipelines, as well as high diagnostic accuracy for the detection of CAD with AUCs between 0.86 and 0.93 for automated quantitative perfusion metrics (85).
Clinical Value of Quantitative Perfusion CMR
Several clinical advantages of QP CMR have been demonstrated over qualitative assessment:
(1) The diagnostic accuracy of QP CMR for the detection of CAD is at least equivalent to a level 3 experienced reader, thus offering an observer independent solution to smaller centres that may lack the experience and volume of the larger academic CMR laboratories. A study from Villa et al. found the diagnostic accuracy of QP CMR at the patient level was similar to the qualitative report of a level 3 trained reader and superior to a level 2 trained reader (QP: 86.3%, qualitative level 3: 83.6%, qualitative level 2: 65.7%) (39). This is consistent with a sub-study of the CE-MARC trial that compared stress MBF, MPR, and qualitative assessment (by expert readers in academic centres) and found no difference in diagnostic accuracies (86).
(2) In multivessel coronary disease QP CMR has superior diagnostic accuracy for the detection of CAD at the vessel level (Figure 5). A study by Kotecha et al. with a cohort of 151 patients (95 patients with multivessel disease defined by invasive angiography with FFR), found stress MBF to have superior diagnostic accuracy than qualitative perfusion CMR to identify 3-vessel (87 vs. 40%) and 2-vessel disease (71 vs. 48%) but similar accuracy for the detection of single vessel disease (71 vs. 71%) (48). In line with these findings, QP CMR also enables a more accurate estimation of the myocardial ischemic burden in cases of multivessel disease. In a study of 41 patients, Patel et al. found qualitative assessment was unable to differentiate the ischemic burdens of single-vessel and three-vessel CAD (21 vs. 31%, p = 0.26) unlike myocardial perfusion reserve (MPR), which found a significant difference (25 vs. 60%, p = 0.02) (45). A similar advantage of QP CMR is also seen in patients with MVD, in whom global ischemia is often present without a region of reference myocardium. A recent study by Rahman et al. of 75 patients with non-obstructed coronary disease, found MPR had a diagnostic accuracy of 79% for the detection of MVD (defined by invasive physiology), significantly superior to qualitative assessment, which had a diagnostic accuracy of 58% (44). Similar findings were reported by Kotecha et al. who found stress MBF had 71% sensitivity, 70% specificity and an AUC of 0.73 for detecting MVD. This study went on to demonstrate that global stress MBF thresholds could be used to non-invasively differentiate 3-vessel coronary disease form MVD (79).
(3) Another potential advantage of QP CMR is its ability to correct for scarred myocardium. The pixel-wise nature of perfusion quantification enables pixel-wise exclusion of LGE from the analysis. This was demonstrated in a study by Villa et al. who fused myocardial perfusion reserve maps with LGE maps to quantify microvascular ischemia in patients with hypertrophic cardiomyopathy. They demonstrated that not accounting for LGE leads to a significant overestimation of the ischemic burden (87). This combined QP and LGE analysis has also been demonstrated feasible in patients with ischemic cardiomyopathy in whom high scar burdens are often present and revascularisation decision making is complex (88). The prognostic value of such an approach to predict myocardial recovery post revascularisation remains unclear.
(4) There is emerging data that QP CMR enables improved patient risk stratification. In 395 patients with suspected CAD and a median follow up of 460 days, Sammut et al. found that the ischemic burden as measured by MPR provided incremental prognostic value to qualitative assessment (89). In another study of 1,049 patients with suspected or known CAD and a median follow up of 605 days, Knott et al. found stress MBF and MPR were both independently associated with death and major adverse cardiovascular events (83).
(5) Unlike semi-quantitative analysis, fully quantitative analysis has the potential to improve clinical workflow by automated post processing, and potential to reduce scan time by acquiring only stress data. There is some evidence that the accuracy of stress MBF is not enhanced by measurements of MBF at rest (79, 90), however, this remains a contentious issue as evidence to the contrary also exists, particularly in patients with MVD (44, 74, 91).
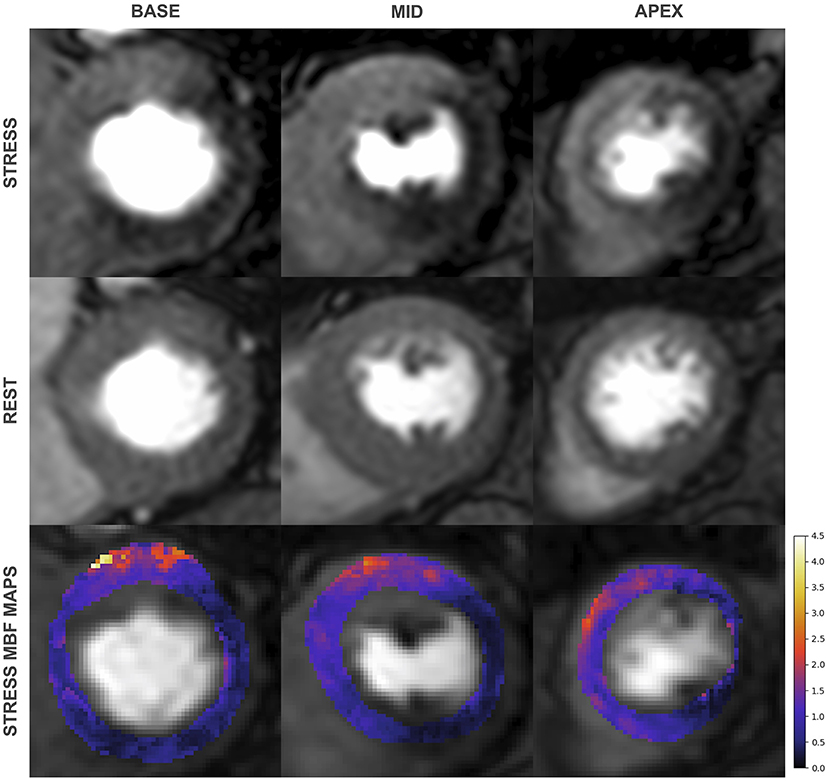
Figure 5. First-pass perfusion images during adenosine induced stress (top) and at rest (middle) with corresponding pixel-wise myocardial blood flow (MBF) maps (bottom) from a 79-year old man with significant 3 vessel disease on invasive coronary angiography. Qualitative assessment identifies inducible perfusion defects in the left circumflex and right coronary artery territories but only perfusion mapping identifies 3 vessel disease.
Quantitative Perfusion CMR and PET
Cardiac PET enables highly accurate measurements of myocardial perfusion, particularly when tracers with linear or near linear extraction are used, and is currently the clinical standard for the non-invasive quantification of myocardial perfusion (78, 92). Recently, perfusion quantification by CMR has been validated against PET with good agreement between perfusion measures (78). As compared to cardiac PET, CMR offers the advantage of higher spatial resolution, wider availability, lower costs, and freedom from ionising radiation. However, where-as the volumetric acquisition of cardiac PET allows for full heart coverage, 3D whole-heart perfusion imaging by CMR remains confined to a research tool and clinical CMR perfusion imaging is typically planned to sample the 16 standard AHA myocardial segments using 3 short-axis slices (3, 13).
Future Directions
DCE stress perfusion CMR has made significant strides over the past 2 decades to become an accurate, well-validated, and safe non-invasive method for assessing the functional significance of CAD (26). Advances in data acquisition methods appear promising and high-resolution full LV coverage is likely to be achieved in the coming years (14). The development and validation of QP CMR offers incremental diagnostic and prognostic value, particularly in patients with advanced CAD (48, 89). Advances in artificial intelligence technology are likely to play an increasingly important role in the clinical interpretation of perfusion maps (83). However, for the widespread use of QP CMR outside of the academic institutions, cross vendor standardisation and regulatory approval for the use of in-line myocardial perfusion quantification is required.
Conclusion
Stress perfusion CMR is a well-validated and guideline-backed non-invasive tool for the assessment and risk stratification of patients with CAD. Qualitative analysis has high diagnostic accuracy and prognostic value when performed by experienced readers. Contemporary, fully automated perfusion quantification pipelines can provide an accessible, reliable, observer independent analysis with superior diagnostic and prognostic performance, particularly in patients with complex multivessel CAD and MVD.
Author Contributions
RF: writing—original draught, review and editing. SP and AC: writing—review and editing. All authors contributed to the article and approved the submitted version.
Funding
The authors acknowledge financial support from: The British Heart Foundation [PG/18/71/34009 and TG/18/2/33768]; The Department of Health via the National Institute for Health Research (NIHR) comprehensive Biomedical Research Centre award to Guy's & St Thomas' NHS Foundation Trust in partnership with King's College London and King's College Hospital NHS Foundation Trust; The NIHR Cardiovascular MedTech Co-operative; Wellcome/EPSRC Centre for Medical Engineering [WT 203148/Z/16/Z].
Author Disclaimer
The views expressed are those of the authors and not necessarily those of the NHS or funding bodies. The funding bodies did not have a role in the writing of this manuscript.
Conflict of Interest
The authors declare that the research was conducted in the absence of any commercial or financial relationships that could be construed as a potential conflict of interest.
The handling editor declared a shared research group with one of the author AC at time of review.
Publisher's Note
All claims expressed in this article are solely those of the authors and do not necessarily represent those of their affiliated organizations, or those of the publisher, the editors and the reviewers. Any product that may be evaluated in this article, or claim that may be made by its manufacturer, is not guaranteed or endorsed by the publisher.
Acknowledgments
The authors would like to acknowledge Drs. Xenios Milidonis and Cian Scannell of King's College London, School of Biomedical Engineering and Imaging Sciences for providing the perfusion maps displayed in Figures 4, 5, respectively.
References
1. Knuuti J, Wijns W, Saraste A, Capodanno D, Barbato E, Funck-Brentano C, et al. 2019 ESC Guidelines for the diagnosis and management of chronic coronary syndromes: the task force for the diagnosis and management of chronic coronary syndromes of the European Society of Cardiology (ESC). Eur Heart J. (2020) 41:407–77. doi: 10.1093/eurheartj/ehz425
2. Gerber BL, Raman SV, Nayak K, Epstein FH, Ferreira P, Axel L, et al. Myocardial first-pass perfusion cardiovascular magnetic resonance: history, theory, and current state of the art. J Cardiovasc Magn Reson. (2008) 10:18. doi: 10.1186/1532-429X-10-18
3. Kramer CM, Barkhausen J, Bucciarelli-Ducci C, Flamm SD, Kim RJ, Nagel E. Standardized cardiovascular magnetic resonance imaging (CMR) protocols: 2020 update. J Cardiovasc Magn Reson. (2020) 22:17. doi: 10.1186/s12968-020-00607-1
4. Kellman P, Arai AE. Imaging sequences for first pass perfusion –a review. J Cardiovasc. (2007) 9:525–37. doi: 10.1080/10976640601187604
5. Mustafa SJ, Morrison RR, Teng B, Pelleg A. Adenosine receptors and the heart: role in regulation of coronary blood flow and cardiac electrophysiology. Handb Exp Pharmacol. (2009) 193:161–88. doi: 10.1007/978-3-540-89615-9_6
7. Atkinson DJ, Burstein D, Edelman RR. First-pass cardiac perfusion: evaluation with ultrafast MR imaging. Radiology. (1990) 174:757–62. doi: 10.1148/radiology.174.3.2305058
8. Biglands JD, Radjenovic A, Ridgway JP. Cardiovascular magnetic resonance physics for clinicians: part II. J Cardiovasc Magn Reson. (2012) 14:66. doi: 10.1186/1532-429X-14-66
9. Cerqueira MD, Weissman NJ, Dilsizian V, Jacobs AK, Kaul S, Laskey WK, et al. Standardized myocardial segmentation and nomenclature for tomographic imaging of the heart. A statement for healthcare professionals from the Cardiac Imaging Committee of the Council on Clinical Cardiology of the American Heart Association. Circulation. (2002) 105:539–42. doi: 10.1161/hc0402.102975
10. Plein S, Schwitter J, Suerder D, Greenwood JP, Boesiger P, Kozerke S. k-Space and time sensitivity encoding-accelerated myocardial perfusion MR imaging at 3.0 T: comparison with 1.5 T. Radiology. (2008) 249:493–500. doi: 10.1148/radiol.2492080017
11. Motwani M, Maredia N, Fairbairn TA, Kozerke S, Radjenovic A, Greenwood JP, et al. High-resolution versus standard-resolution cardiovascular MR myocardial perfusion imaging for the detection of coronary artery disease. Circ Cardiovasc Imaging. (2012) 5:306–13. doi: 10.1161/CIRCIMAGING.111.971796
12. Motwani M, Jogiya R, Kozerke S, Greenwood JP, Plein S. Advanced cardiovascular magnetic resonance myocardial perfusion imaging: high-spatial resolution versus 3-dimensional whole-heart coverage. Circ Cardiovasc Imaging. (2013) 6:339–48. doi: 10.1161/CIRCIMAGING.112.000193
13. Manka R, Wissmann L, Gebker R, Jogiya R, Motwani M, Frick M, et al. Multicenter evaluation of dynamic three-dimensional magnetic resonance myocardial perfusion imaging for the detection of coronary artery disease defined by fractional flow reserve. Circ Cardiovasc Imaging. (2015) 8:e003061. doi: 10.1161/CIRCIMAGING.114.003061
14. Nazir MS, Neji R, Speier P, Reid F, Stäb D, Schmidt M, et al. Simultaneous multi slice (SMS) balanced steady state free precession first-pass myocardial perfusion cardiovascular magnetic resonance with iterative reconstruction at 1.5 T. J Cardiovasc Magn Reson. (2018) 20:84. doi: 10.1186/s12968-018-0502-7
15. Cheng ASH, Pegg TJ, Karamitsos TD, Searle N, Jerosch-Herold M, Choudhury RP, et al. Cardiovascular magnetic resonance perfusion imaging at 3-tesla for the detection of coronary artery disease: a comparison with 1.5-tesla. J Am Coll Cardiol. (2007) 49:2440–9. doi: 10.1016/j.jacc.2007.03.028
16. Araoz PA, Glockner JF, McGee KP, Potter DDJ, Valeti VU, Stanley DW, et al. 3 Tesla MR imaging provides improved contrast in first-pass myocardial perfusion imaging over a range of gadolinium doses. J Cardiovasc Magn Reson. (2005) 7:559–64. doi: 10.1081/JCMR-200060622
17. Holtackers RJ, Wildberger JE, Wintersperger BJ, Chiribiri A. Impact of field strength in clinical cardiac magnetic resonance imaging. Invest Radiol. (2021). 56:764–72. doi: 10.1097/RLI.0000000000000809
18. Oshinski JN, Delfino JG, Sharma P, Gharib AM, Pettigrew RI. Cardiovascular magnetic resonance at 3.0T: current state of the art. J Cardiovasc Magn Reson. (2010) 12:55. doi: 10.1186/1532-429X-12-55
19. Ferreira PF, Gatehouse PD, Mohiaddin RH, Firmin DN. Cardiovascular magnetic resonance artefacts. J Cardiovasc Magn Reson. (2013) 15:41. doi: 10.1186/1532-429X-15-41
20. Brown LAE, Onciul SC, Broadbent DA, Johnson K, Fent GJ, Foley JRJ, et al. Fully automated, inline quantification of myocardial blood flow with cardiovascular magnetic resonance: repeatability of measurements in healthy subjects. J Cardiovasc Magn Reson. (2018) 20:48. doi: 10.1186/s12968-018-0462-y
21. Schulz-Menger J, Bluemke DA, Bremerich J, Flamm SD, Fogel MA, Friedrich MG, et al. Standardized image interpretation and post-processing in cardiovascular magnetic resonance - 2020 update : society for Cardiovascular Magnetic Resonance (SCMR): board of trustees task force on standardized post-processing. J Cardiovasc Magn Reson. (2020) 22:19. doi: 10.1186/s12968-020-00610-6
22. Takx RAP, Blomberg BA, El Aidi H, Habets J, de Jong PA, Nagel E, et al. Diagnostic accuracy of stress myocardial perfusion imaging compared to invasive coronary angiography with fractional flow reserve meta-analysis. Circ Cardiovasc Imaging. (2015) 8:e002666. doi: 10.1161/CIRCIMAGING.114.002666
23. Chiribiri A, Hautvast GL, Lockie T, Schuster A, Bigalke B, Olivotti L, et al. Assessment of coronary artery stenosis severity and location: quantitative analysis of transmural perfusion gradients by high-resolution MRI versus FFR. JACC Cardiovasc Imaging. (2013) 6:600–9. doi: 10.1016/j.jcmg.2012.09.019
24. Bernhardt P, Spiess J, Levenson B, Pilz G, Höfling B, Hombach V, et al. Combined Assessment of myocardial perfusion and late gadolinium enhancement in patients after percutaneous coronary intervention or bypass grafts. A multicenter study of an integrated cardiovascular magnetic resonance protocol. JACC Cardiovasc Imaging. (2009) 2:1292–300. doi: 10.1016/j.jcmg.2009.05.011
25. Jaarsma C, Leiner T, Bekkers SC, Crijns HJ, Wildberger JE, Nagel E, et al. Diagnostic performance of noninvasive myocardial perfusion imaging using single-photon emission computed tomography, cardiac magnetic resonance, and positron emission tomography imaging for the detection of obstructive coronary artery disease: a meta-anal. J Am Coll Cardiol. (2012) 59:1719–28. doi: 10.1016/j.jacc.2011.12.040
26. Kiaos A, Tziatzios I, Hadjimiltiades S, Karvounis C, Karamitsos TD. Diagnostic performance of stress perfusion cardiac magnetic resonance for the detection of coronary artery disease: a systematic review and meta-analysis. Int J Cardiol. (2018) 252:229–33. doi: 10.1016/j.ijcard.2017.11.066
27. Ebersberger U, Makowski MR, Schoepf UJ, Platz U, Schmidtler F, Rose J, et al. Magnetic resonance myocardial perfusion imaging at 3.0 Tesla for the identification of myocardial ischaemia: comparison with coronary catheter angiography and fractional flow reserve measurements. Eur Heart J Cardiovasc Imaging. (2013) 14:1174–80. doi: 10.1093/ehjci/jet074
28. Lockie T, Ishida M, Perera D, Chiribiri A, De Silva K, Kozerke S, et al. High-resolution magnetic resonance myocardial perfusion imaging at 3.0-tesla to detect hemodynamically significant coronary stenoses as determined by fractional flow reserve. J Am Coll Cardiol. (2010) 57:70–5. doi: 10.1016/j.jacc.2010.09.019
29. Bettencourt N, Chiribiri A, Schuster A, Ferreira N, Sampaio F, Duarte R, et al. Cardiac magnetic resonance myocardial perfusion imaging for detection of functionally significant obstructive coronary artery disease: a prospective study. Int J Cardiol. (2013) 168:765–73. doi: 10.1016/j.ijcard.2012.09.231
30. Schwitter J, Wacker CM, van Rossum AC, Lombardi M, Al-Saadi N, Ahlstrom H, et al. MR-IMPACT: comparison of perfusion-cardiac magnetic resonance with single-photon emission computed tomography for the detection of coronary artery disease in a multicentre, multivendor, randomized trial. Eur Heart J. (2008) 29:480–9. doi: 10.1093/eurheartj/ehm617
31. Schwitter J, Wacker CM, Wilke N, Al-Saadi N, Sauer E, Huettle K, et al. MR-IMPACT II: magnetic resonance imaging for myocardial perfusion assessment in coronary artery disease trial: perfusion-cardiac magnetic resonance vs. single-photon emission computed tomography for the detection of coronary artery disease: a comparative. Eur Heart J. (2013) 34:775–81. doi: 10.1093/eurheartj/ehs022
32. Greenwood JP, Motwani M, Maredia N, Brown JM, Everett CC, Nixon J, et al. Comparison of cardiovascular magnetic resonance and single-photon emission computed tomography in women with suspected coronary artery disease from the Clinical Evaluation of Magnetic Resonance Imaging in Coronary Heart Disease (CE-MARC) trial. Circulation. (2014) 129:1129–38. doi: 10.1161/CIRCULATIONAHA.112.000071
33. Schuster A. Validation of Quantitative Myocardial Perfusion Magnetic Resonance Imaging. Ph.D. thesis, King's College London, London, United Kingdom (2012).
34. Kwong RY, Ge Y, Steel K, Bingham S, Abdullah S, Fujikura K, et al. Cardiac magnetic resonance stress perfusion imaging for evaluation of patients with chest pain. J Am Coll Cardiol. (2019) 74:1741–55. doi: 10.1016/j.jacc.2019.07.074
35. Lipinski MJ, McVey CM, Berger JS, Kramer CM, Salerno M. Prognostic value of stress cardiac magnetic resonance imaging in patients with known or suspected coronary artery disease: a systematic review and meta-analysis. J Am Coll Cardiol. (2013) 62:826–38. doi: 10.1016/j.jacc.2013.03.080
36. Greenwood JP, Ripley DP, Berry C, McCann GP, Plein S, Bucciarelli-Ducci C, et al. Effect of care guided by cardiovascular magnetic resonance, myocardial perfusion scintigraphy, or NICE guidelines on subsequent unnecessary angiography rates: the CE-MARC 2 randomized clinical trial. JAMA. (2016) 316:1051–60. doi: 10.1001/jama.2016.12680
37. Nagel E, Greenwood JP, McCann GP, Bettencourt N, Shah AM, Hussain ST, et al. Magnetic resonance perfusion or fractional flow reserve in coronary disease. N Engl J Med. (2019) 380:2418–28. doi: 10.1056/NEJMoa1716734
38. Ge Y, Pandya A, Steel K, Bingham S, Jerosch-Herold M, Chen Y-Y, et al. Cost-effectiveness analysis of stress cardiovascular magnetic resonance imaging for stable chest pain syndromes. JACC Cardiovasc Imaging. (2020) 13:1505–17. doi: 10.1016/j.jcmg.2020.02.029
39. Villa ADM, Corsinovi L, Ntalas I, Milidonis X, Scannell C, Di Giovine G, et al. Importance of operator training and rest perfusion on the diagnostic accuracy of stress perfusion cardiovascular magnetic resonance. J Cardiovasc Magn Reson. (2018) 20:74. doi: 10.1186/s12968-018-0493-4
40. Shaw LJ, Berman DS, Picard MH, Friedrich MG, Kwong RY, Stone GW, et al. Comparative definitions for moderate-severe ischemia in stress nuclear, echocardiography, and magnetic resonance imaging. JACC Cardiovasc Imaging. (2014) 7:593–604. doi: 10.1016/j.jcmg.2013.10.021
41. Shaw LJ, Berman DS, Maron DJ, Mancini GBJ, Hayes SW, Hartigan PM, et al. Optimal medical therapy with or without percutaneous coronary intervention to reduce ischemic burden: results from the Clinical Outcomes Utilizing Revascularization and Aggressive Drug Evaluation (COURAGE) trial nuclear substudy. Circulation. (2008) 117:1283–91. doi: 10.1161/CIRCULATIONAHA.107.743963
42. Martin W, Tweddel AC, Hutton I. Balanced triple-vessel disease: enhanced detection by estimated myocardial thallium uptake. Nucl Med Commun. (1992) 13:149–53. doi: 10.1097/00006231-199203000-00005
43. Christian TF, Miller TD, Bailey KR, Gibbons RJ. Noninvasive identification of severe coronary artery disease using exercise tomographic thallium-201 imaging. Am J Cardiol. (1992) 70:14–20. doi: 10.1016/0002-9149(92)91382-E
44. Rahman H, Scannell CM, Demir OM, Ryan M, McConkey H, Ellis H, et al. High-resolution cardiac magnetic resonance imaging techniques for the identification of coronary microvascular dysfunction. JACC Cardiovasc Imaging. (2021) 14:978–86. doi: 10.1016/j.jcmg.2020.10.015
45. Patel AR, Antkowiak PF, Nandalur KR, West AM, Salerno M, Arora V, et al. Assessment of advanced coronary artery disease: advantages of quantitative cardiac magnetic resonance perfusion analysis. J Am Coll Cardiol. (2010) 56:561–9. doi: 10.1016/j.jacc.2010.02.061
46. Chung S-Y, Lee K-Y, Chun EJ, Lee W-W, Park EK, Chang H-J, et al. Comparison of stress perfusion MRI and SPECT for detection of myocardial ischemia in patients with angiographically proven three-vessel coronary artery disease. AJR Am J Roentgenol. (2010) 195:356–62. doi: 10.2214/AJR.08.1839
47. Driessen RS, Raijmakers PG, Stuijfzand WJ, Knaapen P. Myocardial perfusion imaging with PET. Int J Cardiovasc Imaging. (2017) 33:1021–31. doi: 10.1007/s10554-017-1084-4
48. Kotecha T, Chacko L, Chehab O, O'Reilly N, Martinez-Naharro A, Lazari J, et al. Assessment of multivessel coronary artery disease using cardiovascular magnetic resonance pixelwise quantitative perfusion mapping. JACC Cardiovasc Imaging. (2020) 13:2546–57. doi: 10.1016/j.jcmg.2020.06.041
49. Motwani M, Maredia N, Fairbairn TA, Kozerke S, Greenwood JP, Plein S. Assessment of ischaemic burden in angiographic three-vessel coronary artery disease with high-resolution myocardial perfusion cardiovascular magnetic resonance imaging. Eur Heart J Cardiovasc Imaging. (2014) 15:701–8. doi: 10.1093/ehjci/jet286
50. Klein C, Nagel E, Gebker R, Kelle S, Schnackenburg B, Graf K, et al. Magnetic resonance adenosine perfusion imaging in patients after coronary artery bypass graft surgery. JACC Cardiovasc Imaging. (2009) 2:437–45. doi: 10.1016/j.jcmg.2008.12.016
51. Seraphim A, Knott KD, Beirne AM, Augusto JB, Menacho K, Artico J, et al. Use of quantitative cardiovascular magnetic resonance myocardial perfusion mapping for characterization of ischemia in patients with left internal mammary coronary artery bypass grafts. J Cardiovasc Magn Reson. (2021) 23:82. doi: 10.1186/s12968-021-00763-y
52. Kelle S, Graf K, Dreysse S, Schnackenburg B, Fleck E, Klein C. Evaluation of contrast wash-in and peak enhancement in adenosine first pass perfusion CMR in patients post bypass surgery. J Cardiovasc Magn Reson. (2010) 12:28. doi: 10.1186/1532-429X-12-28
53. Chong WCF, Collins P, Webb CM, De Souza AC, Pepper JR, Hayward CS, et al. Comparison of flow characteristics and vascular reactivity of radial artery and long saphenous vein grafts [NCT00139399]. J Cardiothorac Surg. (2006) 1:4. doi: 10.1186/1749-8090-1-4
54. Jerosch-Herold M. Quantification of myocardial perfusion by cardiovascular magnetic resonance. J Cardiovasc Magn Reson. (2010) 12:57. doi: 10.1186/1532-429X-12-57
55. Christian TF, Rettmann DW, Aletras AH, Liao SL, Taylor JL, Balaban RS, et al. Absolute myocardial perfusion in canines measured by using dual-bolus first-pass MR imaging. Radiology. (2004) 232:677–84. doi: 10.1148/radiol.2323030573
56. Cullen JHS, Horsfield MA, Reek CR, Cherryman GR, Barnett DB, Samani NJ. A myocardial perfusion reserve index in humans using first-pass contrast-enhanced magnetic resonance imaging. J Am Coll Cardiol. (1999) 33:1386–94. doi: 10.1016/S0735-1097(99)00004-2
57. Gould KL, Lipscomb K. Effects of coronary stenoses on coronary flow reserve and resistance. Am J Cardiol. (1974) 34:48–55. doi: 10.1016/0002-9149(74)90092-7
58. Jerosch-Herold M, Hu X, Murthy NS, Rickers C, Stillman AE. Magnetic resonance imaging of myocardial contrast enhancement with MS-325 and its relation to myocardial blood flow and the perfusion reserve. J Magn Reson Imaging. (2003) 18:544–54. doi: 10.1002/jmri.10384
59. Chiribiri A, Villa ADM, Sammut E, Breeuwer M, Nagel E. Perfusion dyssynchrony analysis. Eur Heart J Cardiovasc Imaging. (2016) 17:1414–23. doi: 10.1093/ehjci/jev326
60. Klocke FJ, Simonetti OP, Judd RM, Kim RJ, Harris KR, Hedjbeli S, et al. Limits of detection of regional differences in vasodilated flow in viable myocardium by first-pass magnetic resonance perfusion imaging. Circulation. (2001) 104:2412–6. doi: 10.1161/hc4501.099306
61. Nagel E, Klein C, Paetsch I, Hettwer S, Schnackenburg B, Wegscheider K, et al. Magnetic resonance perfusion measurements for the noninvasive detection of coronary artery disease. Circulation. (2003) 108:432–7. doi: 10.1161/01.CIR.0000080915.35024.A9
62. Al-Saadi N, Nagel E, Gross M, Bornstedt A, Schnackenburg B, Klein C, et al. Noninvasive detection of myocardial ischemia from perfusion reserve based on cardiovascular magnetic resonance. Circulation. (2000) 101:1379–83. doi: 10.1161/01.CIR.101.12.1379
63. van Dijk R, van Assen M, Vliegenthart R, de Bock GH, van der Harst P, Oudkerk M. Diagnostic performance of semi-quantitative and quantitative stress CMR perfusion analysis: a meta-analysis. J Cardiovasc Magn Reson. (2017) 19:92. doi: 10.1186/s12968-017-0393-z
64. Knott KD, Fernandes JL, Moon JC. Automated quantitative stress perfusion in a clinical routine. Magn Reson Imaging Clin N Am. (2019) 27:507–20. doi: 10.1016/j.mric.2019.04.003
65. Sourbron SP, Buckley DL. Tracer kinetic modelling in MRI: estimating perfusion and capillary permeability. Phys Med Biol. (2012) 57:R1–33. doi: 10.1088/0031-9155/57/2/R1
66. Zierler KL. Theoretical basis of indicator-dilution methods for measuring flow and volume. Circ Res. (1962) 10:393–407. doi: 10.1161/01.RES.10.3.393
67. Robinson AA, Salerno M, Kramer CM. Contemporary issues in quantitative myocardial perfusion CMR imaging. Curr Cardiovasc Imaging Rep. (2019) 12:9. doi: 10.1007/s12410-019-9484-6
68. Ichihara T, Ishida M, Kitagawa K, Ichikawa Y, Natsume T, Yamaki N, et al. Quantitative analysis of first-pass contrast-enhanced myocardial perfusion MRI using a Patlak plot method and blood saturation correction. Magn Reson Med. (2009) 62:373–83. doi: 10.1002/mrm.22018
69. Kellman P, Hansen MS, Nielles-Vallespin S, Nickander J, Themudo R, Ugander M, et al. Myocardial perfusion cardiovascular magnetic resonance: optimized dual sequence and reconstruction for quantification. J Cardiovasc Magn Reson. (2017) 19:43. doi: 10.1186/s12968-017-0355-5
70. Cernicanu A, Axel L. Theory-based signal calibration with single-point T1 measurements for first-pass quantitative perfusion MRI studies. Acad Radiol. (2006) 13:686–93. doi: 10.1016/j.acra.2006.02.040
71. Hsu L-Y, Rhoads KL, Holly JE, Kellman P, Aletras AH, Arai AE. Quantitative myocardial perfusion analysis with a dual-bolus contrast-enhanced first-pass MRI technique in humans. J Magn Reson Imaging. (2006) 23:315–22. doi: 10.1002/jmri.20502
72. Ishida M, Schuster A, Morton G, Chiribiri A, Hussain S, Paul M, et al. Development of a universal dual-bolus injection scheme for the quantitative assessment of myocardial perfusion cardiovascular magnetic resonance. J Cardiovasc Magn Reson. (2011) 13:28. doi: 10.1186/1532-429X-13-28
73. Hsu LY, Groves DW, Aletras AH, Kellman P, Arai AE. A quantitative pixel-wise measurement of myocardial blood flow by contrast-enhanced first-pass CMR perfusion imaging: microsphere validation in dogs and feasibility study in humans. JACC Cardiovasc Imaging. (2012) 5:154–66. doi: 10.1016/j.jcmg.2011.07.013
74. Morton G, Chiribiri A, Ishida M, Hussain ST, Schuster A, Indermuehle A, et al. Quantification of absolute myocardial perfusion in patients with coronary artery disease: comparison between cardiovascular magnetic resonance and positron emission tomography J Am Coll Cardiol. (2012) 60:1546–55. doi: 10.1016/j.jacc.2012.05.052
75. Schuster A, Morton G, Chiribiri A, Perera D, Vanoverschelde JL, Nagel E. Imaging in the management of ischemic cardiomyopathy: special focus on magnetic resonance. J Am Coll Cardiol. (2012) 59:359–70. doi: 10.1016/j.jacc.2011.08.076
76. Kim D, Axel L. Multislice, dual-imaging sequence for increasing the dynamic range of the contrast-enhanced blood signal and CNR of myocardial enhancement at 3T. J Magn Reson Imaging. (2006) 23:81–6. doi: 10.1002/jmri.20471
77. Sánchez-gonzález J, Fernandez-jiménez R, Nothnagel ND, López-martín G, Fuster V, Ibañez B. Optimization of dual-saturation single bolus acquisition for quantitative cardiac perfusion and myocardial blood flow maps. J Cardiovasc Magn Reson. (2015) 17:21. doi: 10.1186/s12968-015-0116-2
78. Engblom H, Xue H, Akil S, Carlsson M, Hindorf C, Oddstig J, et al. Fully quantitative cardiovascular magnetic resonance myocardial perfusion ready for clinical use: a comparison between cardiovascular magnetic resonance imaging and positron emission tomography. J Cardiovasc Magn Reson. (2017) 19:78. doi: 10.1186/s12968-017-0388-9
79. Kotecha T, Martinez-Naharro A, Boldrini M, Knight D, Hawkins P, Kalra S, et al. Automated pixel-wise quantitative myocardial perfusion mapping by CMR to detect obstructive coronary artery disease and coronary microvascular dysfunction: validation against invasive coronary physiology. JACC Cardiovasc Imaging. (2019) 12:1958–69. doi: 10.1016/j.jcmg.2018.12.022
80. Scannell CM, Villa ADM, Lee J, Breeuwer M, Chiribiri A. Robust non-rigid motion compensation of free-breathing myocardial perfusion MRI data. IEEE Trans Med Imaging. (2019) 38:1812–20. doi: 10.1109/TMI.2019.2897044
81. Jacobs M, Benovoy M, Chang L-C, Corcoran D, Berry C, Arai AE, et al. Automated segmental analysis of fully quantitative myocardial blood flow maps by first-pass perfusion cardiovascular magnetic resonance. IEEE Access Pract Innov Open Solut. (2021) 9:52796–811. doi: 10.1109/ACCESS.2021.3070320
82. Hansen MS, Sørensen TS. Gadgetron: an open source framework for medical image reconstruction. Magn Reson Med. (2013) 69:1768–76. doi: 10.1002/mrm.24389
83. Knott KD, Seraphim A, Augusto JB, Xue H, Chacko L, Aung N, et al. The prognostic significance of quantitative myocardial perfusion: an artificial intelligence based approach using perfusion mapping. Circulation. (2020) 141:1282–91. doi: 10.1161/CIRCULATIONAHA.119.044666
84. Scannell CM, Veta M, Villa ADM, Sammut EC, Lee J, Breeuwer M, et al. Deep-learning-based preprocessing for quantitative myocardial perfusion MRI. J Magn Reson Imaging. (2020) 51:1689–96. doi: 10.1002/jmri.26983
85. Hsu LY, Jacobs M, Benovoy M, Ta AD, Conn HM, Winkler S, et al. Diagnostic performance of fully automated pixel-wise quantitative myocardial perfusion imaging by cardiovascular magnetic resonance. JACC Cardiovasc Imaging. (2018) 11:697–707. doi: 10.1016/j.jcmg.2018.01.005
86. Biglands JD, Ibraheem M, Magee DR, Radjenovic A, Plein S, Greenwood JP. Quantitative myocardial perfusion imaging versus visual analysis in diagnosing myocardial ischemia a CE-MARC substudy. JACC Cardiovasc Imaging. (2018) 11:711–8. doi: 10.1016/j.jcmg.2018.02.019
87. Villa ADM, Sammut E, Zarinabad N, Carr-White G, Lee J, Bettencourt N, et al. Microvascular ischemia in hypertrophic cardiomyopathy: New insights from high-resolution combined quantification of perfusion and late gadolinium enhancement. J Cardiovasc Magn Reson. (2016) 18:4. doi: 10.1186/s12968-016-0223-8
88. Villa AD, Sammut E, Shome JS, Razavi R, Plein S, Chiribiri A. Combined high-resolution assessment of quantitative perfusion and late enhancement. Towards accurate estimation of the ischaemic burden in patients with coronary artery disease. J Cardiovasc Magn Reson. (2016) 18:Q15. doi: 10.1186/1532-429X-18-S1-Q15
89. Sammut EC, Villa ADM, Di Giovine G, Dancy L, Bosio F, Gibbs T, et al. Prognostic value of quantitative stress perfusion cardiac magnetic resonance. JACC Cardiovasc Imaging. (2018) 11:686–94. doi: 10.1016/j.jcmg.2017.07.022
90. Huber A, Sourbron S, Klauss V, Schaefer J, Bauner KU, Schweyer M, et al. Magnetic resonance perfusion of the myocardium: semiquantitative and quantitative evaluation in comparison with coronary angiography and fractional flow reserve. Invest Radiol. (2012) 47:332–8. doi: 10.1097/RLI.0b013e31824f54cb
91. Schwab F, Ingrisch M, Marcus R, Bamberg F, Hildebrandt K, Adrion C, et al. Tracer kinetic modeling in myocardial perfusion quantification using MRI. Magn Reson Med. (2015) 73:1206–15. doi: 10.1002/mrm.25212
Keywords: myocardial perfusion imaging (MPI), cardiovascular magnetic resonance (CMR), quantitative perfusion, coronary artery disease, dynamic contrast enhance magnetic resonance, first-pass perfusion MRI
Citation: Franks R, Plein S and Chiribiri A (2021) Clinical Application of Dynamic Contrast Enhanced Perfusion Imaging by Cardiovascular Magnetic Resonance. Front. Cardiovasc. Med. 8:768563. doi: 10.3389/fcvm.2021.768563
Received: 31 August 2021; Accepted: 27 September 2021;
Published: 29 October 2021.
Edited by:
Steffen Erhard Petersen, Queen Mary University of London, United KingdomReviewed by:
Michael Jerosch-Herold, Brigham and Women's Hospital and Harvard Medical School, United StatesAlessia Gimelli, Gabriele Monasterio Tuscany Foundation (CNR), Italy
Copyright © 2021 Franks, Plein and Chiribiri. This is an open-access article distributed under the terms of the Creative Commons Attribution License (CC BY). The use, distribution or reproduction in other forums is permitted, provided the original author(s) and the copyright owner(s) are credited and that the original publication in this journal is cited, in accordance with accepted academic practice. No use, distribution or reproduction is permitted which does not comply with these terms.
*Correspondence: Amedeo Chiribiri, YW1lZGVvLmNoaXJpYmlyaSYjeDAwMDQwO2tjbC5hYy51aw==