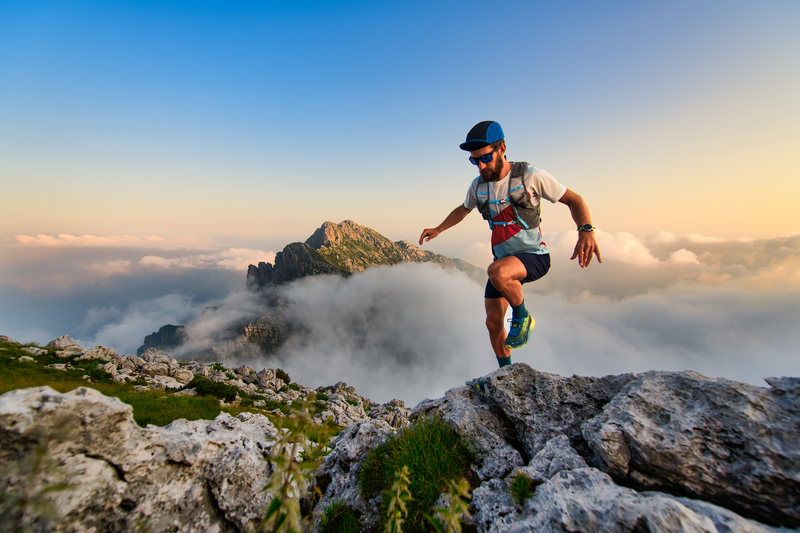
95% of researchers rate our articles as excellent or good
Learn more about the work of our research integrity team to safeguard the quality of each article we publish.
Find out more
ORIGINAL RESEARCH article
Front. Cardiovasc. Med. , 28 October 2021
Sec. General Cardiovascular Medicine
Volume 8 - 2021 | https://doi.org/10.3389/fcvm.2021.762576
This article is part of the Research Topic Ischemic Myocardial Injury and Protection: From bench to bedside View all 14 articles
Background: Contrast induced nephropathy (CIN) is a common complication in patients receiving intravascular contrast media. In 2020, the American College of Radiology and the National Kidney Foundation issued a new contrast induced acute kidney injury (CI-AKI) criteria. Therefore, we aimed to explore the potential risk factors for CIN under the new criteria, and develop a predictive model for patients with coronary artery disease (CAD) with relatively normal renal function (NRF).
Methods: Patients undergoing coronary angiography or percutaneous coronary intervention at Zhongshan Hospital, Fudan University between May 2019 and April 2020 were consecutively enrolled. Eligible candidates were selected for statistical analysis. Univariate and multivariate logistic regression analyses were used to identify the predictive factors. A stepwise method and a machine learning (ML) method were used to construct a model based on the Akaike information criterion. The performance of our model was evaluated using the area under the receiver operating characteristic curves (AUC) and calibration curves. The model was further simplified into a risk score.
Results: A total of 2,009 patients with complete information were included in the final statistical analysis. The results showed that the incidence of CIN was 3.2 and 1.2% under the old and new criteria, respectively. Three independent predictors were identified: baseline uric acid level, creatine kinase-MB level, and log (N-terminal pro-brain natriuretic peptide) level. Our stepwise model had an AUC of 0.816, which was higher than that of the ML model (AUC = 0.668, P = 0.09). The model also achieved accurate predictions regarding calibration. A risk score was then developed, and patients were divided into two risk groups: low risk (total score < 10) and high risk (total score ≥ 10).
Conclusions: In this study, we first identified important predictors of CIN in patients with CAD with NRF. We then developed the first CI-AKI model on the basis of the new criteria, which exhibited accurate predictive performance. The simplified risk score may be useful in clinical practice to identify high-risk patients.
With the application of interventional therapy in cardiovascular diseases, coronary angiography (CAG) has become the gold standard for diagnosing coronary artery disease (CAD). Percutaneous coronary intervention (PCI) has also become one of the most important treatments for patients with CAD. Regardless of the above treatments, use of contrast media (CM) is essential. However, due to the nephrotoxicity of CM, patients exposed to them may develop contrast induced nephropathy (CIN), also known as contrast induced acute kidney injury (CI-AKI). CIN is the third leading cause of hospital-acquired acute kidney injury (1). This complication prolongs the patient's hospital stay and increases medical expenses, resulting in irreversible kidney injury, need for dialysis, or even death (2). Since CIN does not have effective therapies, early identification of high-risk patients and effective interventions are extremely important.
CIN is usually defined as an increase of ≥0.5 mg/dL or ≥25% in baseline serum creatinine (SCr), within 48–72 h after exposure to CM, excluding other causes of renal function impairment (3). In January 2020, the American College of Radiology and the National Kidney Foundation jointly issued a consensus (4) and recommended that the diagnostic criteria of CA-AKI/CI-AKI be referred to the one proposed by kidney disease improving global outcomes (KDIGO), that is, when within 48 h of CM administration, the SCr increased by ≥0.3 mg/dL (26.5 μmol/L) or ≥1.5 times the baseline value (5). To date, few studies have compared the incidence of CIN based on these two diagnostic criteria, and no model has been constructed on the basis of the new criteria.
Baseline renal insufficiency is the most important risk factor for CIN, and many other risk factors, such as advanced age and diabetes, have also been recognized (6). In clinical practice, patients with chronic kidney disease (CKD) undergo hydration before and after surgery (7, 8). However, for those with relatively normal renal function (NRF), it remains unknown whether there are new, unique indicators that can predict the occurrence of CIN. Therefore, we aimed to explore the potential risk factors for CIN among patients with CAD with NRF and establish a predictive model based on new criteria.
Consecutive patients undergoing CAG or PCI at Zhongshan Hospital, Fudan University, between May 2019 and April 2020, were retrospectively enrolled. Demographic data and baseline clinical characteristics were recorded, including age, sex, blood pressure, comorbidities, medical history, laboratory examinations, and procedure-associated factors. The endpoint was CIN occurrence. Inclusion criteria were patients diagnosed with CAD by CAG, with documented renal function (SCr level) at baseline and 48 h after the procedure. Exclusion criteria were patients receiving continuous dialysis for end-stage renal disease, exposure to CM within 1 week before surgery, use of nephrotoxic drugs, severe infections and liver insufficiency, combined with tumors, allergy to CM, and women during pregnancy and lactation. All included patients used low- or iso-osmolarity contrast agents and did not receive hydration therapy. The study was approved by the ethics committee of Zhongshan Hospital, Fudan University. A written informed consent was obtained from all patients upon admission, which allow for the analysis of clinical data for the purpose of scientific study.
“Contrast induced nephropathy (CIN)” or “Contrast induced acute kidney injury (CI-AKI)” was defined as an increase of ≥50% or 0.3 mg/dL in pre-PCI serum creatinine at 48 h after surgery (4). “Coronary artery disease (CAD)” was defined as at least one major coronary artery (left main artery, left anterior descending artery, left circumflex artery, or right coronary artery) stenosis ≥50%, confirmed by CAG (9). “Hypertension” was defined as systolic blood pressure ≥140 mmHg and/or diastolic blood pressure ≥90 mmHg, or previous diagnosis of hypertension and taking antihypertensive medications (10, 11). “Diabetes mellitus” was defined on the basis of the American Diabetes Association criteria (12). “Moderate to severe congestive heart failure (CHF)” was defined as New York Heart Association (NYHA) functional class III–IV (13). “Anemia” was defined as a hematocrit value <39% for men and <36% for women (14). Hyperuricemia was defined as fasting serum uric acid level >7.0 mg/dL in men and >6.0 mg/dL in women (15). Perioperative myocardial infarction was defined on the basis of the fourth universal definition of MI (16).
The data were preprocessed before the formal analysis, and missing values of eGFR were handled on the basis of the CKD-EPI formula (consistent with previously recorded data) (17). Values in the variables of “Hypertension” and “Diabetes Mellitus” were corrected on the basis of the definitions, in combination with actual blood pressure and blood glucose level (or glycosylated hemoglobin) of patients. Univariate and multivariate logistic regression analyses were used to identify the predictive factors. Variables with a P < 0.1 in the univariate analysis were available for further multivariate regression. A stepwise selection method was used to construct the best model on the basis of the Akaike information criterion (AIC). We also used the machine learning (ML) method (elastic net) to select statistically significant variables as a supplementary verification.
To evaluate the performance of our stepwise model, we used the area under the receiver operating characteristic curves (AUC) and calibration curves. Subsequently, to facilitate clinical use, we simplified the stepwise model and converted it into a risk score. Receiver operating characteristic (ROC) curves were used to identify the cut-off values of the continuous variables. Weighted scores were assigned to each risk factor on the basis of their new odds ratios (ORs). On the basis of their total scores, patients were further divided into different risk groups.
All statistical analyses and plotting were performed using R 3.6.3 (Lucent Technologies, New Providence, the United States, https://www.r-project.org) and GraphPad Prism 8 (GraphPad Software, LaJolla, CA, USA, https://www.graphpad.com/scientific-software/prism). Some of the R packages “tidyverse,” “MASS,” “pROC,” “RMS” were used. Continuous variables conforming to the normal distribution were represented as mean ± standard deviation (SD); otherwise, they are presented as median (interquartile range). Categorical variables were expressed as frequency (percentage) using the chi-square test or Fisher's exact test. All P-values were two-sided, and statistical significance was set at P < 0.05.
A total of 2,383 patients with CAD after the procedure were included consecutively in this study. Some patients were excluded from the study: 41 cases without significant coronary artery lesions, 66 with a history of chronic kidney disease (CKD), 265 with eGFR <60 mL/min/1.73 m2, and 2 with hepatic insufficiency. In all, 2,009 patients were included in the statistical analysis. Based on the old criteria, the incidence of CIN was 3.2%, whereas it declined to 1.2% under the new criteria (Figure 1).
Figure 1. Incidence of CIN under different criteria. CIN, contrast induced nephropathy; Old, old criteria; New, new criteria.
The baseline demographic and clinicopathologic features are listed in Table 1. Overall, the mean age of all patients was 63.3 years, and 79.0% were male. Regarding CAD subtypes and comorbidities, 22.9% had ACS, 74.4% had hypertension, and 40.9% had diabetes mellitus. Patients who underwent low-osmolarity CM accounted for 69.7% of the patients, and the mean volume of contrast was 150.17 ± 4.5 mL. Patients who developed CIN (CINs) and those who did not (non-CINs) differed substantially with respect to the following variables: diastolic blood pressure, smoking status, CAD subtype, previous acute myocardial infarction (AMI) history, and laboratory examinations (left ventricular ejection fraction, left ventricular end-systolic dimension, neutrophils, lymphocytes, neutrophil to lymphocyte ratio, eosinophils, platelet distribution width, aspartate aminotransferase [AST], low density lipoprotein, non-high-density lipoprotein, apolipoprotein B, creatine kinase-MB [CK-MB], high-sensitivity C-reactive protein, cardiac troponin T [cTnT], and N-terminal pro-brain natriuretic peptide [NT-proBNP] levels).
All the variables in Table 1 were used in the subsequent univariate analysis, and some skewed variables (for example, alanine transaminase [ALT] and AST) were log-transformed. Table 2 shows the factors with a P < 0.1. A total of 22 variables were significantly associated with the development of CIN, namely vital signs, medical history, comorbidities, cardiac ultrasound, and laboratory indexes. Afterward, considering the collinearity of some existing variables, we retained systolic blood pressure and LVEF instead of diastolic blood pressure and LVESD. A stepwise logistic regression was performed to construct the model. The final multivariate results are shown in Table 3. Baseline uric acid (UA), CK-MB, and log (NT-proBNP) levels were identified as independent predictors of CIN. The AIC of the model was 213.63 (Table 3).
The performance of our stepwise model was evaluated using the AUC and the calibration curve (Figure 2). The AUC of the stepwise model was compared with that of the machine learning (ML) model, and the calibration was performed using internal validation. The AUC of the stepwise model was 0.816, which was higher than that of the ML model (AUC = 0.668, P = 0.09, Figure 2A). The calibration curve showed that the predictive incidence was significantly associated with the actual probability of CIN (Figure 2B).
Figure 2. (A) Receiver operating characteristic (ROC) curves of stepwise model and machine learning (ML) model. (B) Calibration curve of stepwise model. AUC, area under the receiver operating characteristic curves; CIN, contrast induced nephropathy.
To facilitate implementation in clinical practice, we simplified the stepwise model to a risk score. The cut-off values of the three variables were identified using ROC curves (Supplementary Figure 1). We chose baseline UA ≥450 μmol/L, CK-MB ≥48 U/L, and NT-proBNP ≥850 pg/mL as the cut-off values, and the new multivariate results are shown in Supplementary Table 1. A weighted score of 1 was assigned to each OR value, and the final risk score is shown in Figure 3. The total scores of the patients in our cohort were between 0 and 17 points. Based on the total scores, we divided patients into two risk groups (low risk and high risk, corresponding to a total score of <10 and ≥10, respectively). The CIN incidence of patients in the low-risk group was 1.0%, while the incidence increased to 14.8% when the total score was ≥10 (Figure 4).
Figure 3. Simplified predictive score for CIN. UA, uric acid; CK-MB, creatine kinase MB; NT-proBNP, N-terminal pro-brain natriuretic peptide.
In this study, we first compared the incidence of CIN using two different criteria. The incidence of CIN was generally low, with 3.4% under the old criteria and 1.6% under the new criteria. The incidence under the old criteria was very similar to the results of Rihal et al. (18). Therefore, based on the KDIGO criteria, the incidence of CIN decreased dramatically, indicating that the old definition of CIN, to some extent, may have exaggerated the development of CI-AKI. In addition, other factors such as the different study populations and the nature of the CAG procedure may also lead to the variance of incidence rate of CIN (19).
Next, we explored the risk factors for CIN development. The results showed that, except for traditional risk factors, there were other predictors of great importance for patients with relatively NRF. In the multivariate logistic regression analysis, we determined the predictive value of baseline UA, CK-MB, and log (NT-proBNP) levels. A stepwise model and a risk score were then constructed, and to the best of our knowledge, these two are the first CIN predictive models based on the new KDIGO criteria. Our model exhibited good predictive ability, with an AUC of 0.816. Furthermore, we simplified the model to a risk score, which facilitates its use by clinicians. Two risk groups were further defined: low-risk (<10 points) and high-risk (≥10 points). The incidence of CIN increased significantly as the score increased (Figure 4).
Hyperuricemia (or baseline UA level) has been widely confirmed to influence the occurrence of CIN (20). In the present study, baseline UA level was an independent risk factor (OR, 1.004; P = 0.04). Similarly, other studies have proved that hyperuricemia is significantly associated with a higher risk of CI-AKI, regardless of renal function (15, 16). Nevertheless, it remains unknown whether UA lowering therapy can effectively reduce the incidence of CIN. A randomized controlled trial explored the role of allopurinol in preventing CIN after PCI and failed to show its efficacy (21). At any rate, it warned us that patients with high UA levels before PCI have the potential to develop CIN.
CK-MB is an early marker of myocardial injury with high specificity. Our analysis showed that CK-MB has an important value in predicting CIN (OR: 1.01, 95% CI: 1.01–1.02, P < 0.001). Previously, Zbierska-Rubinkiewicz et al. found that increased baseline CK-MB level was an independent risk factor for CIN among patients treated with PCI (P = 0.001) (22). The prediction model constructed by Gurm et al. also incorporated CK-MB as an important predictor (23). These findings are consistent with our results. Regarding the reason why cTnT was excluded from the model, we believe that this is due to the fact that the level of CK-MB is less affected by renal function; therefore, it can reflect patients' myocardial ischemia to a large extent. This makes CK-MB more favorable for predicting CIN.
Another finding in our study pertained to the level of NT-proBNP. In multivariate analysis, log (NT-proBNP) was statistically significant (OR, 1.94; 95%CI: 1.45–2.63, P < 0.001). BNP plays an important role in maintaining the circulating blood volume and osmotic pressure. In contrast, NT-proBNP, the precursor of BNP, is an important biomarker for the diagnosis of heart failure due to its long half-life and good in vitro stability (24). Nevertheless, since NT-proBNP is mainly filtered and cleared by the glomerulus, it is greatly affected by renal function. NT-proBNP has shown a predictive value for CIN in both STEMI and elective surgery patients (25–27). In 2015, Liu et al. found that preprocedural NT-proBNP levels could predict CIN as effectively as the Mehran risk score (13, 27). Our results are consistent with their work. When developing the simple risk score, we identified a cut-off value of NT-proBNP as ≥850 pg/mL and incorporated it into the model to achieve better prediction.
It is not clear why elevated NT-proBNP levels are associated with a higher risk of CIN. Some studies have proposed that BNP reduces the effects of catecholamines and potentiates the generation of nitric oxide, thereby potentially resulting in systemic vasodilation and renal hypoperfusion (27). Future research may further explore this potential mechanism.
Other recognized risk factors include advanced age, diabetes, CHF (NYHA III-IV), and anemia. In particular, Mehran et al. published a risk score that included eight variables: hypotension, IABP, CHF, CKD, diabetes, age >75 years, anemia, and volume of contrast (13). None of these variables showed statistical significance in our cohort, indicating that the three predictors (levels of UA, CK-MB, and NT-proBNP) were more significant. The type and amount of contrast medium are also associated with the development of CIN. It has been reported that the effect of the volume of contrast and CIN was dose dependent. In our study, the effect of contrast volume was not statistically significant (OR: 1.002, P = 0.256). We speculated that the amount of CM had little effect on patients with relatively NRF. Additionally, compared with studies conducted in Western countries, a smaller average amount of CM in our study (150.17 ± 4.5 mL) may also make the effect insignificant.
Our study is a well-designed research exploring the risk factors for predicting CIN in Chinese people, with a large sample size. The established stepwise model exhibited outstanding performance (AUC = 0.816) and could predict CIN precisely (Figure 2). The limitations of our work lie in the following aspects. First, the number of patients with CIN was relatively small. In other words, the incidence of CIN in our cohort was below average (new criteria: 1.2%). This may add some difficulty in determining the effects of the predictors. Moreover, the study was retrospective and could not control for all confounding factors. Finally, we used internal validation to evaluate the model in order to make full use of the data to construct the model. Therefore, additional external validation is required for further studies and model evaluation.
In the present study, we first identified three independent risk factors in patients with CAD with relatively NRF: baseline UA level, CK-MB level, and NT-proBNP level of CIN. Meanwhile, we developed the first stepwise model and risk score based on the new CI-AKI criteria, which exhibited accurate predictive ability. Two risk groups were defined on the basis of the total score of the patients. This simplified risk score may be helpful in clinical practice to identify high-risk patients in the future.
The raw data supporting the conclusions of this article will be made available by the authors, without undue reservation.
The studies involving human participants were reviewed and approved by the Ethics Committee of Zhongshan Hospital Fudan University (B2021-219). The patients/participants provided their written informed consent to participate in this study.
HM, FY, YD, SJ, and JG conceived and designed the study. HM, FY, ZM, YZ, and YD conducted the statistical analyses. HM and SJ drafted the manuscript. SJ and YD had full access to all the data and took responsibility for the integrity of the data and the accuracy of the data analysis. All authors participated in the interpretation of the results, revised the manuscript, read, and approved the final manuscript.
This study was supported by the National Key Research and Development Program of China (Grant No. 2016YFC1301200), the Program for Shanghai Outstanding Medical Academic Leader (Grant No. 2019LJ15), the Scientific Research Project of Shanghai Science and Technology Commission (Grant No. 17ZR1404900), Clinical Research Plan of SHDC (Grant No. SHDC2020CR1007A), Animal Model Project of Shanghai Scientific Committee (Grant No. 19140900900), Natural Science Foundation of Shanghai (Grant No. 20ZR1439700), and Exploratory Clinical Research Projects of National Clinical Research Center for Interventional Medicine (Grant No. 2021-002). The funding agencies had no role in the design and conduct of the study, in the collection, management, analysis, interpretation of the data or in the preparation, review or approval of the manuscript, or decision to submit for publication.
The authors declare that the research was conducted in the absence of any commercial or financial relationships that could be construed as a potential conflict of interest.
All claims expressed in this article are solely those of the authors and do not necessarily represent those of their affiliated organizations, or those of the publisher, the editors and the reviewers. Any product that may be evaluated in this article, or claim that may be made by its manufacturer, is not guaranteed or endorsed by the publisher.
The Supplementary Material for this article can be found online at: https://www.frontiersin.org/articles/10.3389/fcvm.2021.762576/full#supplementary-material
1. Nash K, Hafeez A, Hou S. Hospital-acquired renal insufficiency. Am J Kidney Dis. (2002) 39:930–6. doi: 10.1053/ajkd.2002.32766
2. Almendarez M, Gurm HS, Mariani J Jr, Montorfano M, Brilakis ES, Mehran R, et al. Procedural strategies to reduce the incidence of contrast-induced acute kidney injury during percutaneous coronary intervention. JACC Cardiovasc Interv. (2019) 12:1877–88. doi: 10.1016/j.jcin.2019.04.055
3. Khwaja A. KDIGO Clinical practice guidelines for acute kidney injury. Nephron Clinical Practice. (2012) 120:C179–C84. doi: 10.1159/000339789
4. Davenport MS, Perazella MA, Yee J, Dillman JR, Fine D, McDonald RJ, et al. Use of intravenous iodinated contrast media in patients with kidney disease: consensus statements from the American college of radiology and the national kidney foundation. Radiology. (2020) 294:660–8. doi: 10.1148/radiol.2019192094
5. Palevsky PM, Liu KD, Brophy PD, Chawla LS, Parikh CR, Thakar CV, et al. KDOQI US commentary on the 2012 KDIGO clinical practice guideline for acute kidney injury. Am J Kidney Dis. (2013) 61:649–72. doi: 10.1053/j.ajkd.2013.02.349
6. Azzalini L, Spagnoli V, Ly HQ. Contrast-Induced Nephropathy: From Pathophysiology to Preventive Strategies. Can J Cardiol. (2016) 32:247–55. doi: 10.1016/j.cjca.2015.05.013
7. Liu Y, Tan N, Huo Y, Chen S, Liu J, Chen YD, et al. Hydration for prevention of kidney injury after primary coronary intervention for acute myocardial infarction: a randomised clinical trial. Heart (British Cardiac Society). (2021). doi: 10.1136/heartjnl-2021-319716. [Epub ahead of print].
8. Zhang F, Lu Z, Wang F. Advances in the pathogenesis and prevention of contrast-induced nephropathy. Life Sci. (2020) 259:118379. doi: 10.1016/j.lfs.2020.118379
9. Cassar A, Holmes DR Jr, Rihal CS, Gersh BJ. Chronic coronary artery disease: diagnosis and management. Mayo Clin Proc. (2009) 84:1130–46. doi: 10.4065/mcp.2009.0391
10. Liu J. Highlights of the 2018 Chinese hypertension guidelines. Clini Hypertens. (2020) 26:8. doi: 10.1186/s40885-020-00141-3
11. Joint Committee for Guideline Revision. 2018 Chinese guidelines for prevention and treatment of hypertension-a report of the revision committee of chinese guidelines for prevention and treatment of hypertension. J Geriatr Cardiol. (2019) 16:182–241. doi: 10.11909/j.issn.1671-5411.2019.03.014
12. American Diabetes Association. Classification and diagnosis of diabetes: standards of medical care in diabetes-2019. Diabetes Care. (2019) 42:S13–s28. doi: 10.2337/dc19-S002
13. Mehran R, Aymong ED, Nikolsky E, Lasic Z, Iakovou I, Fahy M, et al. A simple risk score for prediction of contrast-induced nephropathy after percutaneous coronary intervention: development and initial validation. J Am Coll Cardiol. (2004) 44:1393–9. doi: 10.1016/S0735-1097(04)01445-7
14. Tziakas D, Chalikias G, Stakos D, Apostolakis S, Adina T, Kikas P, et al. Development of an easily applicable risk score model for contrast-induced nephropathy prediction after percutaneous coronary intervention: a novel approach tailored to current practice. Int J Cardiol. (2013) 163:46–55. doi: 10.1016/j.ijcard.2011.05.079
15. Bardin T, Richette P. Definition of hyperuricemia and gouty conditions. Curr Opin Rheumatol. (2014) 26:186–91. doi: 10.1097/BOR.0000000000000028
16. Thygesen K, Alpert JS, Jaffe AS, Chaitman BR, Bax JJ, Morrow DA, et al. Fourth Universal Definition of Myocardial Infarction (2018). Circulation. (2018) 138:e618–e51. doi: 10.1161/CIR.0000000000000617
17. Levey AS, Stevens LA, Schmid CH, Zhang YL, Castro AF 3rd, Feldman HI, et al. A new equation to estimate glomerular filtration rate. Ann Intern Med. (2009) 150:604–12. doi: 10.7326/0003-4819-150-9-200905050-00006
18. Rihal CS, Textor SC, Grill DE, Berger PB, Ting HH, Best PJ, et al. Incidence and prognostic importance of acute renal failure after percutaneous coronary intervention. Circulation. (2002) 105:2259–64. doi: 10.1161/01.CIR.0000016043.87291.33
19. Hossain MA, Costanzo E, Cosentino J, Patel C, Qaisar H, Singh V, et al. Contrast-induced nephropathy: Pathophysiology, risk factors, and prevention. Saudi J Kidney Dis Transpl. (2018) 29:1–9. doi: 10.4103/1319-2442.225199
20. Barbieri L, Verdoia M, Schaffer A, Cassetti E, Marino P, Suryapranata H, et al. Uric acid levels and the risk of Contrast Induced Nephropathy in patients undergoing coronary angiography or PCI. Nutr Metab Cardiovasc Dis. (2015) 25:181–6. doi: 10.1016/j.numecd.2014.08.008
21. Ghelich Khan Z, Talasaz AH, Pourhosseini H, Hosseini K, Alemzadeh Ansari MJ, Jalali A. Potential role of allopurinol in preventing contrast-induced nephropathy in patients undergoing percutaneous coronary intervention: a randomized placebo-controlled trial. Clin Drug Investig. (2017) 37:853–60. doi: 10.1007/s40261-017-0542-z
22. Zbierska-Rubinkiewicz K, Trebacz O, Tomala M, Rubinkiewicz M, Chrzan I, Gackowski A. Creatine kinase-MB and red cell distribution width as predictors of contrast-induced nephropathy after percutaneous coronary intervention in acute myocardial infarction. Folia Med Cracov. (2017) 57:87–99.
23. Gurm HS, Seth M, Kooiman J, Share D. A novel tool for reliable and accurate prediction of renal complications in patients undergoing percutaneous coronary intervention. J Am Coll Cardiol. (2013) 61:2242–8. doi: 10.1016/j.jacc.2013.03.026
24. Hall C. NT-ProBNP: the mechanism behind the marker. J Card Fail. (2005) 11:S81–3. doi: 10.1016/j.cardfail.2005.04.019
25. Goussot S, Mousson C, Guenancia C, Stamboul K, Brunel P, Brunet D, et al. N-terminal fragment of pro b-type natriuretic peptide as a marker of contrast-induced nephropathy after primary percutaneous coronary intervention for ST-segment elevation myocardial infarction. Am J Cardiol. (2015) 116:865–71. doi: 10.1016/j.amjcard.2015.06.007
26. Jarai R, Dangas G, Huber K, Xu K, Brodie BR, Witzenbichler B, et al. B-type natriuretic peptide and risk of contrast-induced acute kidney injury in acute ST-segment-elevation myocardial infarction: a substudy from the HORIZONS-AMI trial. Circ Cardiovasc Interv. (2012) 5:813–20. doi: 10.1161/CIRCINTERVENTIONS.112.972356
27. Liu Y, He YT, Tan N, Chen JY, Liu YH, Yang DH, et al. Preprocedural N-terminal pro-brain natriuretic peptide (NT-proBNP) is similar to the Mehran contrast-induced nephropathy (CIN) score in predicting CIN following elective coronary angiography. J Am Heart Assoc. (2015) 4:e001410. doi: 10.1161/JAHA.114.001410
Keywords: contrast induced nephropathy (CIN), coronary artery disease, contrast media (CM), incidence, risk factor, predictive model
Citation: Mo H, Ye F, Chen D, Wang Q, Liu R, Zhang P, Xu Y, Cheng X, Mei Z, Zheng Y, Dai Y, Jiang S and Ge J (2021) A Predictive Model Based on a New CI-AKI Definition to Predict Contrast Induced Nephropathy in Patients With Coronary Artery Disease With Relatively Normal Renal Function. Front. Cardiovasc. Med. 8:762576. doi: 10.3389/fcvm.2021.762576
Received: 22 August 2021; Accepted: 04 October 2021;
Published: 28 October 2021.
Edited by:
Hongmei Tan, Sun Yat-sen University, ChinaReviewed by:
Yafeng Li, Shanxi Provincial People's Hospital, ChinaCopyright © 2021 Mo, Ye, Chen, Wang, Liu, Zhang, Xu, Cheng, Mei, Zheng, Dai, Jiang and Ge. This is an open-access article distributed under the terms of the Creative Commons Attribution License (CC BY). The use, distribution or reproduction in other forums is permitted, provided the original author(s) and the copyright owner(s) are credited and that the original publication in this journal is cited, in accordance with accepted academic practice. No use, distribution or reproduction is permitted which does not comply with these terms.
*Correspondence: Sunfang Jiang, amlhbmcuc3VuZmFuZ0B6cy1ob3NwaXRhbC5zaC5jbg==; Yuxiang Dai, ZGFpLnl1eGlhbmdAenMtaG9zcGl0YWwuc2guY24=
†These authors have contributed equally to this work
Disclaimer: All claims expressed in this article are solely those of the authors and do not necessarily represent those of their affiliated organizations, or those of the publisher, the editors and the reviewers. Any product that may be evaluated in this article or claim that may be made by its manufacturer is not guaranteed or endorsed by the publisher.
Research integrity at Frontiers
Learn more about the work of our research integrity team to safeguard the quality of each article we publish.