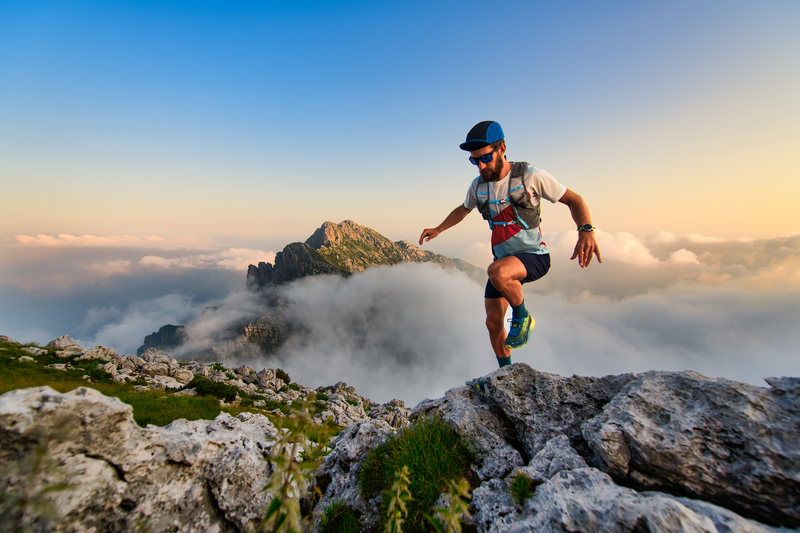
94% of researchers rate our articles as excellent or good
Learn more about the work of our research integrity team to safeguard the quality of each article we publish.
Find out more
ORIGINAL RESEARCH article
Front. Cardiovasc. Med. , 18 November 2021
Sec. General Cardiovascular Medicine
Volume 8 - 2021 | https://doi.org/10.3389/fcvm.2021.761605
This article is part of the Research Topic Insights in Heart Failure and Transplantation: 2021 View all 15 articles
Background: Although many risk prediction models have been released internationally, the application of these models in the Chinese population still has some limitations.
Aims: The purpose of the study was to establish a heart failure (HF) prognosis model suitable for the Chinese population.
Methods: According to the inclusion criteria, we included patients with chronic heart failure (CHF) who were admitted to the Department of Cardiac Rehabilitation of Tongji Hospital from March 2007 to December 2018, recorded each patient's condition and followed up on the patient's re-admission and death. All data sets were randomly divided into derivation and validation cohorts in a ratio of 7/3. Least absolute shrinkage and selection operator regression and Cox regression were used to screen independent predictors; a nomogram chart scoring model was constructed and validated.
Results: A total of 547 patients were recruited in this cohort, and the median follow-up time was 519 days. The independent predictors screened out by the derivation cohort included age, atrial fibrillation (AF), percutaneous coronary intervention (PCI), diabetes mellitus (DM), peak oxygen uptake (peak VO2), heart rate at the 8th minute after the cardiopulmonary exercise peaked (HR8min), C-reaction protein(CRP), and uric acid (UA). The C indexes values of the derivation and the validation cohorts were 0.69 and 0.62, respectively, and the calibration curves indicate that the model's predictions were in good agreement with the actual observations.
Conclusions: We have developed and validated a multiple Cox regression model to predict long-term mortality and readmission risk of Chinese patients with CHF.
Registration Number: ChicTR-TRC-00000235.
Heart failure (HF) is a serious manifestation of the late stage of various heart diseases. Its morbidity has an increasing trend, and the mortality and rehospitalization rates remain high, posing a huge economic burden for health care systems (1–3). According to recent studies, the 1-year mortality rate of patients with chronic heart failure (CHF) is 6.4%, and the combined death or HF hospitalization rate within 1 year is 14.5% (4). Therefore, stratifying patients according to the risk of future results (re-admission and death), and optimizing treatment strategies for patients with different needs would be beneficial to all healthcare systems and patients.
The 2019 American College of Cardiology (ACC) Expert Consensus on HF proposes that the assessment of risk-increasing factors can help guide decision-making for preventive intervention (5). Many risk prediction models have been released internationally (6–10). The 2017 A systems Biology Study to Tailored Treatment in Chronic Heart Failure (BIOSTAT-CHF) research plan included 2,516 HF patients from 69 centers in 11 international centers. It concluded that the strongest predictors of mortality are age, urea nitrogen, N-terminal B-type natriuretic peptide, hemoglobin, and beta blockers. The predictors of hospitalization rate are age, hospitalization history for HF, edema, systolic blood pressure, and estimated glomerular filtration rate (6). In 2019, the Korean Acute Heart Failure registry used 12 predictors to establish a risk score that predicts the risk of HF-specific readmission or death at 30 days after discharge (7). The 2020 Prospective Comparison of angiotensin receptor neprilysin inhibitor (ARNI) with angiotensin-converting enzyme inhibitor (ACEI) to Determine Impact on Global Mortality and Morbidity in Heart Failure (PARADIGM-HF) model can accurately predict the morbidity and mortality of ambulatory patients with CHF with reduced ejection fraction at 1 and 2 years (8). It can be seen from the above that the current existing forecasting models have the following advantages: Indicators are easily available and not focusing on a single risk factor. However, the application of these models in the Chinese population still has some limitations. Most of these models use static indicators, which are not sufficient to reflect the overall condition of the patients (6–8, 10, 11). Further, most studies have a short follow-up time and cannot judge the long-term prognosis (7, 10, 11). As there are regional differences in the mortality and rehospitalization rates of patients with CHF and scarce data from the Chinese population, the applicability of the international HF risk prediction model to the Chinese population is controversial (4, 12). Therefore, it is necessary to establish a new HF risk prediction model suitable for the Chinese population.
In this study, we selected individuals with CHF in China as the target population for modeling and conducted relevant cardiopulmonary exercise tests (CPETs) to monitor the respiratory and circulatory parameters of patients under exercise to obtain comprehensive indicators of cardiopulmonary function. According to the literature, CPET parameters are predictors of the prognosis of HF, especially the level of peak oxygen uptake (peak VO2) (13–15). Of note, our follow-up time is long (the longest follow-up time is as long as 12 years) which is helpful to assess the long-term prognosis of patients with CHF. Our research combines the experimental indicators of cardiopulmonary exercise to propose a long-term prognosis and readmission model for CHF in the Chinese population, which provides a basis for the treatment of clinical CHF and reveals potential targets for interventions to improve prognosis.
This study follows the Transparent Reporting of a multivariable prediction model for Individual Prognosis or Diagnosis (TRIPOD) report (16). The clinical data of the study were from 2,136 patients with CHF in the Outpatient or Cardiac Rehabilitation Department in Shanghai Tongji Hospital Affiliated to Tongji University from March 1, 2007 to December 31, 2018. The available information includes demographics, medical history, CPET indicators, echocardiography, laboratory testing, and drugs. Other data extracted include the number of hospital admissions, length of stay, and New York Heart Association (NYHA) classification at admission. Inclusion criteria include CHF diagnosed in accordance with the “Chinese Heart Failure Diagnosis and Treatment Guidelines 2018” with a medical history of more than 3 months; age ≥18 years; NYHA heart function level 1–3; and ability to cooperate to complete the CPET. Exclusion criteria include chronic obstructive pulmonary disease, pulmonary heart disease or pulmonary vascular disease; acute myocardial infarction; second or third degree atrioventricular block; acute pericarditis, rheumatic heart disease; thrombophlebitis or intracardiac thrombosis; severe supraventricular or ventricular arrhythmia; and uncontrolled hypertension. According to the criteria of acceptance, 1,589 patients were excluded, and 547 patients with HF were finally included (Figure 1). The study was conducted in accordance with the Declaration of Helsinki as revised in 2013 and was approved by the Ethics Committee of Tongji Hospital Affiliated to Tongji University [LL(H)-08-04]. Informed consent was taken from all the patients. Before analysis, the patient's records/information were anonymized and de-identified.
Potential predictors include the following characteristics of the patient: demographic characteristics (e.g., gender, age, height, weight, and body mass index), basic heart disease history (e.g., coronary heart disease, dilated heart disease, and hypertension), other related medical history (e.g., diabetes, hyperlipidemia, and stroke), CPET indicators [e.g., peak VO2, end-tidal CO2 pressure (PETCO2)], cardiac ultrasonography [e.g., left ventricular ejection fraction (LVEF)], laboratory tests (e.g., blood lipids, and blood potassium), and medications for HF treatment [e.g., angiotensin-converting enzyme inhibitors (ACEI), angiotensin receptor blockers (ARB), angiotensin receptor neprilysin inhibitors (ARNI), β-blockers].
A total of 90 variables were included in the study as potential prognostic factors. Patients with readmission or death records mainly for HF during the follow- up were defined as the readmitted or died group. Patients who did not have a re-admission or death record during the follow up were defined as the not readmitted, alive group. This is an open-label design trial. In order to reduce bias, examiners, researchers collecting data on outcome indicators, data managers, and statisticians do not know the patient's name.
Readers should refer to the definitions of key clinical data elements and cardiovascular endpoint events issued by the ACC/American Heart Association (AHA) in conjunction with the US Food and Drug Administration and the Cardiovascular Trial Standard Data Collection Program. The endpoint of this study was the composite endpoint of all-cause death and all-cause admission. The data from the electronic medical records and our follow-up results were used to measure this result.
Starting from December 2018, a telephone follow-up or electronic medical record review was conducted every 6 months to collect information on hospitalization or death in the past period, until the patient's first readmission or death or the study was terminated by the follow-up on June 30, 2020. The time of hospitalization or death was recorded by the follow-up staff. A wrong telephone number, lack of response, and refusal to follow up were considered as being lost to follow up.
We used R language [R software (version 3.6.1, R Foundation for Statistical Computing, Vienna, Austria)] and SPSS software (version 20.0; IBM Corp, Armonk, NY, USA) for statistical analysis. The measurement data were tested for normal distribution. Normally distributed measurement data were expressed as mean ± standard deviation. The comparison between two groups was done using an independent sample t-test and the comparison between multiple groups using one-way analysis of variance (ANOVA). The least significant difference test was used for multiple comparisons between groups. Non-normal distribution measurement data were expressed as medians and interquartile ranges (IQR) and compared by the non-parametric Mann-Whitney U-test. Categorical data were expressed as frequency (percentage), and χ2-test was used for comparison between groups. In addition, variables with missing values <20% were subjected to multiple imputation using the R language mice package. P < 0.05 indicates that the difference is statistically significant.
Through random sampling, the entire data set was divided into derivation and validation cohorts in a ratio of 7:3. Least absolute shrinkage and selection operator (LASSO) regression and Cox multivariate analysis were used to screen independent risk factors that could affect the prognosis of CHF, and a nomogram was used to establish a risk prediction model. The C-index values were calculated for the derivation and validation groups. The degree of discrimination was determined and a calibration curve drawn to evaluate the degree of calibration. Finally, the patients were classified into high (greater than the mean risk score) and low risk (no greater than the mean risk score) categories according to the score, and the Kaplan-Meier (KM) curve was drawn for survival analysis (17).
A total of 547 patients with HF were screened; 18.3% were women; the median age was 63 years; the median follow-up time was 519 days and the loss to follow up rate was 10.79%; The incidence of composite endpoints (all-cause death and all-cause admission) in 1/3/5/10 years were 40.95%, 56.86%, 60.15%, and 63.44%, respectively. Compared with the not readmitted and alive group, the readmitted or died group had the following characteristics: (i) older subjects; (ii) a high proportion of patients with a history of ischemic heart disease (IHD) (65%), atrial fibrillation (AF) (17.4%), myocardial infarction (MI) (45%), percutaneous coronary intervention (PCI) (48.4%), and diabetes mellitus (DM) (33.6%) (P < 0.05); (iii) lower oxygen consumption at anaerobic threshold (VO2 AT), peak VO2, heart rate at anaerobic threshold (HRAT), and peak average blood pressure (Peak MBP); (iv) higher ventilation/carbon dioxide production (VE/VCO2) slope; (v) lower levels of biochemical indicator, troponin I (TnI) (Table 1).
Using computer-generated random numbers, the majority (70%) of the cohort were randomly assigned to the derivation cohort (n = 384), and the remaining 30% were assigned to the validation cohort (n = 163). There was no significant difference in the characteristics between the derivation and validation groups (Supplementary Table 1). After 90 variables entered LASSO regression screening, 14 variables [age, AF, MI, PCI, DM, height, peak VO2, heart rate at the 8th minute after the cardiopulmonary exercise peaked (HR8min), peak diastolic blood pressure (peak DBP), peak MBP, c-reactive protein (CRP), b-type natriuretic peptide (BNP), TnI, uric acid (UA)] were obtained (Supplementary Figures 1A,B), and eight variables were retained after univariate analysis, multivariable analysis, and stepwise regression (Table 2; Supplementary Table 2).
In univariate regression analysis, 12 variables were significantly related to the prognosis of heart failure (the composite endpoint of death and rehospitalization). In the multivariate Cox regression analysis, the regression coefficients of the indicators in Table 3, and construct the prognostic risk proportion model [the calculation formula is h(t,x) = h0 (t)exp(0.025Age+0.560AF+0.803PCI+0.423DM−0.056Peak VO2+0.056HR8min+0.039 CRP+0.002UA)] according to the multivariate Cox regression coefficients of the eight indicators (18). Factors—age, AF, PCI, DM, peak VO2, HR8min, CRP, and UA—that were independently associated with a higher likelihood of readmission or death were construct a risk scoring model, which was expressed in the form of a nomogram (Tables 2, 3; Figure 2; Supplementary Figure 2).
Figure 2. Nomogram for the prediction of mortality and hospitalization in Chinese heart failure patients. Instructions for use of nomogram: draw a vertical line, and then add up the points corresponding to each feature, and then draw the vertical line according to the total points until it intersects each survival axis to determine the survival probability of 1, 3, 5, and 10 years. For binary variables, 0 = no, 1 = yes. AF, atrial fibrillation; CRP, c-reactive protein; DM, diabetes mellitus; HR8min, heart rate at the 8th minute after the cardiopulmonary exercise peaked; PCI, percutaneous coronary intervention; Peak VO2, peak oxygen uptake; UA, uric acid.
The AUC of the prediction model is shown in Supplementary Figure 3. To evaluate the effectiveness of risk prediction for the composite endpoint of heart failure death and readmission. The sensitivity, specificity, positive predictive value, and negative predictive value of the training group's prediction model are shown in Supplementary Table 3.
In the derivation and validation cohorts, the calculated model discrimination C-index values were 0.69 (95%CI 0.65–0.72) and 0.62 (95%CI 0.57–0.67), respectively, and the model's discrimination ability was moderate. The calibration chart shows that the occurrence of end-point events at 1 year/3 years/5 years/10 years was in good agreement with the actual observations (Supplementary Figure 4).
Based on the score calculated by the nomogram, the derivation cohort was divided into two groups, high- and low- risk groups, and KM curve survival analysis was performed. The results of the LogRank method showed that the KM curves of the high-risk and low-risk groups were significantly different in the three cohorts (derivation group, validation group, and the whole group). In the derivation group, the risk of rehospitalization or death at 1 year/3 years/5 years/10 years was 69.5, 87.8, 92.7, and 100% for the high-risk group and 32.5,61.9, 75.8, and 95.7% for the low-risk group, respectively (Figure 3; Supplementary Figure 5).
In this study, we selected simple parameters based on clinical conditions, laboratory indicators, cardiopulmonary exercise tests, and other measurements, and developed a new prediction model based on the Chinese HF population, which can assess the risk of readmission or death of patients with CHF. The C index values of the derivation cohort and validation cohorts were 0.69 (95%CI 0.65–0.72) and 0.62 (95%CI 0.57–0.67), respectively, which indicate moderate levels of predictive ability. Our C-index values are high among those from previously published HF risk prediction models (19). Moreover, the calibration curve at 1 year/3 years/5 years/10 years showed good consistency. Compared with the traditional HF risk prediction model, our model has some advantages for CHF patients in China: (i) it adds relevant CPET indicators; (ii) has a long follow-up time, and (iii) the target population is based on the Chinese population. This model can comprehensively assess the long-term prognosis of patients with HF and provide data for the Chinese population for inclusion in the world's CHF prognosis assessment.
In our cohort study, it was observed that patients with CHF who were hospitalized or who died were older, had more complications, and had poorer exercise endurance. The indicators included in the model were age, AF, PCI, DM, peak VO2, HR8min, CRP, and UA. As a protective factor, peak VO2 has been used as a tool to assess the severity of the disease, judge the short-term and long-term prognosis of patients with HF, and select patients for heart transplantation (20, 21). Lewis and Zlotoff (22) summarized the application of risk stratification based on cardiopulmonary exercise experiments in the management of advanced HF. They showed that exercise response patterns are predictive: Peak oxygen consumption can predict the lifespan of ordinary people, and CPET can effectively divide patients into high-risk and low-risk categories of HF events (22). Of note, as the peak VO2 index can be improved by exercise (23), this model evaluation encourages high-risk HF patients to undergo exercise-based cardiac rehabilitation. Therefore, we believe that during continuous exercise, with the improvement of exercise endurance, the risk score may continue to improve, and finally improve the long-term prognosis.
In addition, we noted that the risk factors that affect the prognosis also include heart rate (specific time), CRP, and UA, which is consistent with the risk factors mentioned in the current study (24–30). Heart rate is a determinant of myocardial oxygen demand, coronary blood flow, and myocardial function, and is the key to adapting cardiac output to metabolic demands. An elevated heart rate can predict adverse outcomes in patients with CHF, which may be related to neurohormonal activation (24). Inflammation plays a key role in the etiology and progression of atherosclerosis. Studies have shown that in patients undergoing PCI for the first ST elevation myocardial infarction, there is a clear relationship between the in-hospital CRP plasma concentration and the development of HF after infarction (25). Uric acid can affect cardiovascular disease through platelet aggregation and endothelial inflammation activation. The change in serum uric acid levels in the hospital can predict adverse outcomes in patients with HF (26, 31).
There are other combined CPET HF risk scores, whose predictive accuracy is variable, depending on population selection, treatment, event selection, and follow-up time (32–35). The 2012 Heart Failure: A Controlled Trial Investigating Outcomes of Exercise TraiNing (HF-ACTION) predictive risk score model is the first risk predictive model for HF patients caused by systolic insufficiency. It analyzes multiple candidate variables, including demographics, medical history, laboratory values, CPET exercise parameters, quality of life, and the level of depression (32). The HF-ACTION represents heart failure with reduced ejection fraction (HFrEF) patients, and its score contains indicators that are easily available. However, the limitation is that patients with preserved systolic function are excluded, and the representativeness of people from outside the United States are limited. Our study included patients with heart failure with a moderately reduced ejection fraction (HFmrEF) and heart failure with preserved ejection fraction (HFpEF), and included the Chinese population for modeling, which has good applicability to the Chinese population. At the same time, the indicators we included are easily available clinically. In 2013, the Metabolic Exercise test data combined with Cardiac and Kidney Indexes (MECKI) score combined CPET indicators with established clinical, laboratory and echocardiography parameters, and finally included six indicators of hemoglobin, Na+, modification of diet in renal disease, LVEF, peak VO2, and VE/VCO2 slope (33). These continuous variables define the MECKI score, identify the risk of cardiovascular death and heart transplantation, and have been validated in different settings (34). However, it is mainly used for white people, and its applicability to the Chinese population is unknown. In 2020, Pugliese et al. identified five independent predictors including peak oxygen consumption, which can determine the increased risk of adverse events in patients with HF with preserved ejection fraction (35). However, the sample size of that study is small, the follow-up time is short, and its applicability in Chinese population needs to be investigated.
Although there are many HF risk prediction models in the world, a model based on Chinese population data is an unmet need. On the one hand, our current model highlights the importance of CPET in risk scoring, helps optimize the risk stratification and management of HF patients, and supports cardiac rehabilitation programs. On the other hand, the indicators included in our model are simple and easy to obtain, which helps its vigorous promotion in primary hospitals.
Our study has the following limitations: First, as all the patients are from Shanghai Tongji Hospital, this is a single-center study with regional limitations and an insufficient sample size. Our future research will include people from other parts of China and expand the sample size of the research. Second, the included population comprises people who are around 60 years old, which may limit the generality of our model. However, compared with younger patients, older patients tend to have a higher risk of death and readmission. Third, there is currently a lack of external verification. At the same time, there is also a lack of comparison data with other international forecasting models in the same Chinese cohort. In the future, we will promote this model, conduct external verification, compare it with other international models in the same Chinese cohort, and continue to improve the model to provide a theoretical basis for treatment decisions for Chinese HF patients. Finally, the loss to follow-up rate in this study exceeded 5%. The main reasons include patients' lack of attention to the study, poor compliance, and insufficient communication skills of the follow-up staff. Moreover, due to the long follow-up time (the longest follow-up time is up to 12 years), the patient's telephone number and place of residence have changed, resulting in a high rate of loss to follow-up. In the future follow-up, we will strengthen patient education, increase doctor-patient contact, and conduct communication training for follow-up personnel, so as to reduce the rate of loss to follow-up as much as possible.
In this study, a scoring model for CHF in China was constructed based on CPET indicators. This model can evaluate the long-term risk of death or rehospitalization due to CHF and provide decision-making basis for clinicians, patients, and their families.
Clinicians use this model to assess the prognosis of patients with heart failure and identify high-risk patients, so as to better guide the implementation of treatment plans.
Although there are many models for evaluating the mortality and hospitalization risk of patients with heart failure, this study combines the experimental indicators of cardiopulmonary exercise to propose a long-term prognosis and readmission model for chronic heart failure in the Chinese population, laying the foundation for more accurate risk stratification in the future.
The raw data supporting the conclusions of this article will be made available by the authors, without undue reservation.
The studies involving human participants were reviewed and approved by Ethics Committee of Tongji Hospital Affiliated to Tongji University. The patients/participants provided their written informed consent to participate in this study.
BZ and TS prepared the manuscript and all the authors participated in the clinical and related research. All authors gave final approval and agreed to be accountable for the integrity and accuracy of all aspects of the work.
This work was supported by National Natural Science Foundation (81974359 and 81700316) and advanced proper technology promotion project of Municipal Health and Health Commission in Shanghai, China (2019SY014).
The authors declare that the research was conducted in the absence of any commercial or financial relationships that could be construed as a potential conflict of interest.
All claims expressed in this article are solely those of the authors and do not necessarily represent those of their affiliated organizations, or those of the publisher, the editors and the reviewers. Any product that may be evaluated in this article, or claim that may be made by its manufacturer, is not guaranteed or endorsed by the publisher.
We would like to thank all of the subjects, research coordinators, and investigators who participated in the trials.
The Supplementary Material for this article can be found online at: https://www.frontiersin.org/articles/10.3389/fcvm.2021.761605/full#supplementary-material
ACC, American College of Cardiology; ACEI, angiotensin-converting enzyme inhibitors; AF, atrial fibrillation; AHA, American Heart Association; ANOVA, analysis of variance; ARB, angiotensin receptor blockers; ARNI, angiotensin receptor neprilysin inhibitor; BIOSTAT-CHF, Biology Study to Tailored Treatment in Chronic Heart Failure; BNP, B-type natriuretic peptide; CHF, chronic heart failure; CPETs, cardiopulmonary exercise tests; CRP, C-reactive protein; DCM, dilated cardiomyopathy; DM, diabetes mellitus; HBP, high blood pressure; HF, heart failure; HF-ACTION, Heart Failure: A Controlled Trial Investigating Outcomes of Exercise TraiNing; HFmrEF, heart failure with a moderately reduced ejection fraction; HFpEF, heart failure with preserved ejection fraction; HFrEF, heart failure with reduced ejection fraction; HR8min, heart rate at the 8th minute after the cardiopulmonary exercise peaked; HRAT, heart rate at anaerobic threshold; IHD, ischemic heart disease; IQR, interquartile ranges; KM, Kaplan-Meier; LASSO, least absolute shrinkage and selection operator; LVEF, left ventricular ejection fraction; MECKI, The Metabolic Exercise test data combined with Cardiac and Kidney Indexes; MI, myocardial infarction; NYHA, New York Heart Association; PARADIGM-HF, Global Mortality and Morbidity in Heart Failure; PCI, percutaneous coronary intervention; Peak DBP, peak diastolic blood pressure; Peak MBP, peak average blood pressure; Peak VO2, peak oxygen uptake; PETCO2, end-tidal CO2 pressure; TnI, troponin I; TRIPOD, transparent reporting of a multivariable prediction model for individual prognosis or diagnosis; UA, uric acid; VE/VCO2, ventilation/carbon dioxide production; VO2 AT, oxygen consumption at anaerobic threshold; WT, weight.
1. Urbich M, Globe G, Pantiri K, Heisen M, Bennison C, Wirtz HS, et al. A systematic review of medical costs associated with heart failure in the USA (2014-2020). Pharmacoeconomics. (2020) 38:1219–36. doi: 10.1007/s40273-020-00952-0
2. Al-Omary MS, Davies AJ, Evans TJ, Bastian B, Fletcher PJ, Attia J, et al. Mortality and readmission following hospitalisation for heart failure in Australia: a systematic review and meta-analysis. Heart Lung Circ. (2018) 27:917–27. doi: 10.1016/j.hlc.2018.01.009
3. Sadiq AM, Chamba NG, Sadiq AM, Shao ER, Temu GA. Clinical characteristics and factors associated with heart failure readmission at a tertiary hospital in North-Eastern Tanzania. Cardiol Res Pract. (2020) 2020:2562593. doi: 10.1155/2020/2562593
4. Crespo-Leiro MG, Anker SD, Maggioni AP, Coats AJ, Filippatos G, Ruschitzka F, et al. European Society of Cardiology Heart Failure Long-Term Registry (ESC-HF-LT): 1-year follow-up outcomes and differences across regions. Eur J Heart Fail. (2016) 18:613–25. doi: 10.1002/ejhf.566
5. Arnett DK, Blumenthal RS, Albert MA, Buroker AB, Goldberger ZD, Hahn EJ, et al. 2019 ACC/AHA guideline on the primary prevention of cardiovascular disease: a report of the American College of Cardiology/American Heart Association Task Force on Clinical Practice Guidelines. Circulation. (2019) 140:e596–646. doi: 10.1161/CIR.0000000000000678
6. Voors AA, Ouwerkerk W, Zannad F, van Veldhuisen DJ, Samani NJ, Ponikowski P, et al. Development and validation of multivariable models to predict mortality and hospitalization in patients with heart failure. Eur J Heart Fail. (2017) 19:627–34. doi: 10.1002/ejhf.785
7. Lim NK, Lee SE, Lee HY, Cho HJ, Choe WS, Kim H, et al. Risk prediction for 30-day heart failure-specific readmission or death after discharge: data from the Korean Acute Heart Failure (KorAHF) registry. J Cardiol. (2019) 73:108–13. doi: 10.1016/j.jjcc.2018.07.009
8. Simpson J, Jhund PS, Lund LH, Padmanabhan S, Claggett BL, Shen L, et al. Prognostic models derived in PARADIGM-HF and validated in ATMOSPHERE and the Swedish heart failure registry to predict mortality and morbidity in chronic heart failure. JAMA Cardiol. (2020) 5:432–41. doi: 10.1001/jamacardio.2019.5850
9. Chirinos JA, Orlenko A, Zhao L, Basso MD, Cvijic ME, Li Z, et al. Multiple plasma biomarkers for risk stratification in patients with heart failure and preserved ejection fraction. J Am Coll Cardiol. (2020) 75:1281–95. doi: 10.1016/j.jacc.2019.12.069
10. Tan BY, Gu JY, Wei HY, Chen L, Yan SL, Deng N. Electronic medical record-based model to predict the risk of 90-day readmission for patients with heart failure. BMC Med Inform Decis Mak. (2019) 19:193. doi: 10.1186/s12911-019-0915-8
11. Leong KT, Wong LY, Aung KC, Macdonald M, Cao Y, Lee S, et al. Risk stratification model for 30-day heart failure readmission in a multiethnic South East Asian community. Am J Cardiol. (2017) 119:1428–32. doi: 10.1016/j.amjcard.2017.01.026
12. Samsky MD, Ambrosy AP, Youngson E, Liang L, Kaul P, Hernandez AF, et al. Trends in readmissions and length of stay for patients hospitalized with heart failure in Canada and the United States. JAMA Cardiol. (2019) 4:444–53. doi: 10.1001/jamacardio.2019.0766
13. Corra U, Agostoni PG, Anker SD, Coats AJS, Crespo Leiro MG, de Boer RA, et al. Role of cardiopulmonary exercise testing in clinical stratification in heart failure. A position paper from the Committee on Exercise Physiology and Training of the Heart Failure Association of the European Society of Cardiology. Eur J Heart Fail. (2018) 20:3–15. doi: 10.1002/ejhf.979
14. Sato T, Yoshihisa A, Kanno Y, Suzuki S, Yamaki T, Sugimoto K, et al. Cardiopulmonary exercise testing as prognostic indicators: comparisons among heart failure patients with reduced, mid-range and preserved ejection fraction. Eur J Prev Cardiol. (2017) 24:1979–87. doi: 10.1177/2047487317739079
15. Mikkelsen N, Cadarso-Suarez C, Lado-Baleato O, Diaz-Louzao C, Gil CP, Reeh J, et al. Improvement in VO2peak predicts readmissions for cardiovascular disease and mortality in patients undergoing cardiac rehabilitation. Eur J Prev Cardiol. (2020) 27:811–9. doi: 10.1177/2047487319887835
16. Collins GS, Reitsma JB, Altman DG, Moons KG. Transparent reporting of a multivariable prediction model for individual prognosis or diagnosis (TRIPOD): the TRIPOD statement. BMJ. (2015) 350:g7594. doi: 10.1136/bmj.g7594
17. Ceglarek U, Schellong P, Rosolowski M, Scholz M, Willenberg A, Kratzsch J, et al. The novel cystatin C, lactate, interleukin-6, and N-terminal pro-B-type natriuretic peptide (CLIP)-based mortality risk score in cardiogenic shock after acute myocardial infarction. Eur Heart J. (2021) 42:2344–52. doi: 10.1093/eurheartj/ehab110
18. Yang YF, Li PZ, Liang XB, Han XL, Li YP, Cong J. [Study on the prognostic factors of colorectal cancer after radical resection and on suggested model for prediction]. Zhonghua Liu Xing Bing Xue Za Zhi. (2005) 26:214–217.
19. Di Tanna GL, Wirtz H, Burrows KL, Globe G. Evaluating risk prediction models for adults with heart failure: a systematic literature review. PLoS ONE. (2020) 15:e0224135. doi: 10.1371/journal.pone.0224135
20. Mehra MR, Canter CE, Hannan MM, Semigran MJ, Uber PA, Baran DA, et al. The 2016 International Society for Heart Lung Transplantation listing criteria for heart transplantation: a 10-year update. J Heart Lung Transplant. (2016) 35:1–23. doi: 10.1016/j.healun.2015.10.023
21. Paolillo S, Veglia F, Salvioni E, Corra U, Piepoli M, Lagioia R, et al. Heart failure prognosis over time: how the prognostic role of oxygen consumption and ventilatory efficiency during exercise has changed in the last 20 years. Eur J Heart Fail. (2019) 21:208–17. doi: 10.1002/ejhf.1364
22. Lewis GD, Zlotoff DA. Cardiopulmonary exercise testing-based risk stratification in the modern era of advanced heart failure management. JACC Heart Fail. (2021) 9:237–40. doi: 10.1016/j.jchf.2021.01.003
23. Petersen AK, Oestergaard LG, van Tulder M, Laustsen S. A comparison of high versus low dose of exercise training in exercise-based cardiac rehabilitation: a randomized controlled trial with 12-months follow-up. Clin Rehabil. (2020) 34:69–81. doi: 10.1177/0269215519883411
24. Shaaya G, Al-Khazaali A, Arora R. Heart rate as a biomarker in heart failure: role of heart rate lowering agents. Am J Ther. (2017) 24:e532–9. doi: 10.1097/MJT.0000000000000336
25. Stumpf C, Sheriff A, Zimmermann S, Schaefauer L, Schlundt C, Raaz D, et al. C-reactive protein levels predict systolic heart failure and outcome in patients with first ST-elevation myocardial infarction treated with coronary angioplasty. Arch Med Sci. (2017) 13:1086–93. doi: 10.5114/aoms.2017.69327
26. Yamamoto H, Nagatomo Y, Mahara K, Yoshikawa T. In-hospital serum uric acid change predicts adverse outcome in patients with heart failure. J Card Fail. (2020) 26:968–76. doi: 10.1016/j.cardfail.2020.07.002
27. Sciatti E, Vizzardi E, Bonadei I, Dallapellegrina L, Carubelli V. The role of heart rate and ivabradine in acute heart failure. Monaldi Arch Chest Dis. (2019) 89:1091. doi: 10.4081/monaldi.2019.1091
28. Bielecka-Dabrowa A, von Haehling S, Rysz J, Banach M. Novel drugs for heart rate control in heart failure. Heart Fail Rev. (2018) 23:517–25. doi: 10.1007/s10741-018-9696-x
29. Dupuy AM, Curinier C, Kuster N, Huet F, Leclercq F, Davy JM, et al. Multi-marker strategy in heart failure: combination of ST2 and CRP predicts poor outcome. PLoS ONE. (2016) 11:e0157159. doi: 10.1371/journal.pone.0157159
30. Mantovani A, Targher G, Temporelli PL, Lucci D, Gonzini L, Nicolosi GL, et al. Prognostic impact of elevated serum uric acid levels on long-term outcomes in patients with chronic heart failure: a post-hoc analysis of the GISSI-HF (Gruppo Italiano per lo Studio della Sopravvivenza nella Insufficienza Cardiaca-Heart Failure) trial. Metabolism. (2018) 83:205–15. doi: 10.1016/j.metabol.2018.02.007
31. Lee SJ, Oh BK Sung KC. Uric acid and cardiometabolic diseases. Clin Hypertens. (2020) 26:13. doi: 10.1186/s40885-020-00146-y
32. O'Connor CM, Whellan DJ, Wojdyla D, Leifer E, Clare RM, Ellis SJ, et al. Factors related to morbidity and mortality in patients with chronic heart failure with systolic dysfunction: the HF-ACTION predictive risk score model. Circ Heart Fail. (2012) 5:63–71. doi: 10.1161/CIRCHEARTFAILURE.111.963462
33. Agostoni P, Corra U, Cattadori G, Veglia F, La Gioia R, Scardovi AB, et al. Metabolic exercise test data combined with cardiac and kidney indexes, the MECKI score: a multiparametric approach to heart failure prognosis. Int J Cardiol. (2013) 167:2710–8. doi: 10.1016/j.ijcard.2012.06.113
34. Corra U, Agostoni P, Giordano A, Cattadori G, Battaia E, La Gioia R, et al. The metabolic exercise test data combined with Cardiac and Kidney Indexes (MECKI) score and prognosis in heart failure. A validation study. Int J Cardiol. (2016) 203:1067–72. doi: 10.1016/j.ijcard.2015.11.075
35. Pugliese NR, De Biase N, Gargani L, Mazzola M, Conte L, Fabiani I, et al. Predicting the transition to and progression of heart failure with preserved ejection fraction: a weighted risk score using bio-humoural, cardiopulmonary, and echocardiographic stress testing. Eur J Prev Cardiol. (2020) 2020:zwaa129. doi: 10.1093/eurjpc/zwaa129
Keywords: risk prediction, mortality, hospitalization, heart failure, Chinese population
Citation: Zhuang B, Shen T, Li D, Jiang Y, Li G, Luo Q, Jin Y, Shan Z, Che L, Wang L, Zheng L and Shen Y (2021) A Model for the Prediction of Mortality and Hospitalization in Chinese Heart Failure Patients. Front. Cardiovasc. Med. 8:761605. doi: 10.3389/fcvm.2021.761605
Received: 20 August 2021; Accepted: 28 October 2021;
Published: 18 November 2021.
Edited by:
Yan Zhang, Peking University, ChinaReviewed by:
Xianhui Zhou, First Affiliated Hospital of Xinjiang Medical University, ChinaCopyright © 2021 Zhuang, Shen, Li, Jiang, Li, Luo, Jin, Shan, Che, Wang, Zheng and Shen. This is an open-access article distributed under the terms of the Creative Commons Attribution License (CC BY). The use, distribution or reproduction in other forums is permitted, provided the original author(s) and the copyright owner(s) are credited and that the original publication in this journal is cited, in accordance with accepted academic practice. No use, distribution or reproduction is permitted which does not comply with these terms.
*Correspondence: Lemin Wang, d2FuZ2xlbWluMjAwM0AxNjMuY29t; Liang Zheng, emhlbmdsaWFuZ0B0b25namkuZWR1LmNu; Yuqin Shen, c3lfMTk2M0AxMjYuY29t
†These authors have contributed equally to this work and share first authorship
Disclaimer: All claims expressed in this article are solely those of the authors and do not necessarily represent those of their affiliated organizations, or those of the publisher, the editors and the reviewers. Any product that may be evaluated in this article or claim that may be made by its manufacturer is not guaranteed or endorsed by the publisher.
Research integrity at Frontiers
Learn more about the work of our research integrity team to safeguard the quality of each article we publish.