- 1Research Department of Medical Sciences, Guangdong Provincial People's Hospital, Guangdong Academy of Medical Sciences, Guangzhou, China
- 2Department of Cardiology, Guangdong Cardiovascular Institute, Guangdong Provincial People's Hospital, Guangdong Academy of Medical Sciences, Guangzhou, China
- 3Department of Epidemiology, Centre for Global Cardiometabolic Health, Brown University, Providence, RI, United States
Background: Although many cardiovascular disease studies have focused on the microRNAs of circulating exosomes, the profile and the potential clinical diagnostic value of plasma exosomal long RNAs (exoLRs) are unknown for acute myocardial infarction (AMI).
Methods: In this study, the exoLR profile of 10 AMI patients, eight stable coronary artery disease (CAD) patients, and 10 healthy individuals was assessed by RNA sequencing. Bioinformatic approaches were used to investigate the characteristics and potential clinical value of exoLRs.
Results: Exosomal mRNAs comprised the majority of total exoLRs. Immune cell types analyzed by CIBERSORT showed that neutrophils and monocytes were significantly enriched in AMI patients, consistent with clinical baseline values. Biological process enrichment analysis and co-expression network analysis demonstrated neutrophil activation processes to be enriched in AMI patients. Furthermore, two exosomal mRNAs, ALPL and CXCR2, were identified as AMI biomarkers that may be useful for evaluation of the acute inflammatory response mediated by neutrophils.
Conclusions: ExoLRs were assessed in AMI patients and found to be associated with the acute inflammatory response mediated by neutrophils. Exosomal mRNAs, ALPL and CXCR2, were identified as potentially useful biomarkers for the study of AMI.
Introduction
Exosomes secreted by most cell types are a class of lipid membrane-enclosed extracellular vesicles ranging in size from 40 to 100 nm (1, 2). These small vesicles not only contain proteins, lipids, RNAs, and metabolites of the source cell but also maintain the stability of vesicle constituents (3). Exosomes are considered crucial mediators of cell–cell communication and are promising biomarkers for disease diagnosis (4, 5).
To date, most studies have focused on examination of microRNAs in circulating exosomes with emphasis on the characterization of exosomal microRNAs associated with cardiovascular disease (4, 6, 7). However, those studies have been limited by the small quantity and specificity of available exosomal microRNAs (8).
Circular RNA (circRNA), long noncoding RNAs (lncRNA), and messenger RNA (mRNA) are long RNAs present and stabilized in exosomes (9). These exosomal RNAs may have potential functional and clinical applications (10). For example, CCL2 exosomal mRNAs derived from tubular epithelial cells and macrophages are crucial for albumin-induced tubulointerstitial inflammation (11). Indeed, two serum exosomal mRNAs, KRTAP5-4, and MAGEA3, may be potential biomarkers for the detection of colorectal cancer (12). However, very few studies have assessed the characteristics of exosomal long RNAs (exoLRs) in cardiovascular disease, e.g., AMI, which has the associated health consequences of mortality, morbidity, and monetary costs to society (13). And the profile of circulating exoLRs in AMI patients is unknown.
In this study, we explored the plasma exoLRs profiles of individuals with AMI and stable coronary artery disease (CAD), as well as healthy individuals. This was accomplished by RNA sequencing analysis, which was used to investigate the characteristics and potential clinical diagnostic value of such profiles. Plasma exoLRs profiles may not only reflect the circulating immune cell types but also distinguish patients with AMI and CAD from healthy individuals. In this manner we have identified potential biomarkers for AMI diagnosis, which may provide insight into the intrinsic basis for AMI.
Materials and Methods
Patients
This is a case-control study. Ten AMI, eight CAD patients, and 10 healthy individuals were recruited at the Guangdong Provincial People's Hospital from December 2018 to January 2019. The AMI and CAD patients were diagnosed by laboratory tests and coronary angiography based on the European Society of Cardiology guidelines (14, 15). Participants were 18–75 years of age and included both genders.
The healthy individuals' recruitment inclusion criteria included: normal renal and liver function; and no history of smoking, malignancy, recent cardiovascular or cerebrovascular events, rheumatologic disorders, chronic heart failure, diabetes, acute or chronic infectious disease, aortic dissection, pulmonary embolism, myocarditis, pericarditis, or congenital heart disease.
Ethical approval of human sample collection was obtained from the Ethics Committee of Guangdong Provincial People's Hospital (No. 2018160A). All patients provided informed consent.
Extraction of Exosomal RNAs From Serum and Library Construction, Sequencing, and Data Analysis
Two milliliters of venous blood were collected into ethylene diamine tetraacetic acid (EDTA) routine blood tubes immediately at admission before coronary angiography. Plasma was separated by centrifugation at 2,000 × g for 10 min at 4°C and stored in cryogenic vials at −80°C. The exoRNeasy Serum/Plasma kit (Qiagen) was used to extract exosomal total RNA based on the manufacturer's instructions. Exosomal RNA was extracted from 1 mL of plasma. SMART technology (Clontech) was used to construct the RNA-seq libraries. RNA sequencing was performed using a illumine Nova-Seq 6000 System with the technical support of the Guangzhou Epibiotek Co., Ltd. HISAT2 was used to align sequencing reads (16). The GENCODE database was used to annotate mRNAs and lncRNAs. CircRNAs from unmapped reads were identified with the Accurate CircRNA Finder Suite (17).
Measurement and Characterization of Exosomes
Exosome morphological characteristics were assessed by transmission electron microscopy (TEM). Size and distribution were measured using NanoSight NS500 (NanoSight Ltd., Amesbury, United Kingdom). Exosomal protein markers CD9 and CD63 were detected by western blot.
Immune Cells Landscape Analysis
The abundance of immune cells in serum of study groups were quantified using CIBERSOFT algorithm. The standardized gene expression data of the cells was uploaded to a publicly available online database (https://cibersort.stanford.edu/index.php). Overall, we characterized the serum distribution of 22 human immune cells based on 547 marker genes (18).
Identification of ExoLRs and Functional Enrichment Analysis
NetworkAnalyst (http://www.networkanalyst.ca), a visual analytics platform for comprehensive gene expression profiling (19), was used to identify exoLRs that differed between AMI, CAD, and normal samples. The platform has three steps: data filtering, normalization, and difference analysis. First, exoLRs with low expression were filtered from the dataset. Variance percentile rank lower than 15% was filtered and the minimum criterion for retaining an RNA was at least 4 counts per million. Following data filtering, counts were then normalized using the trimmed mean of M-values normalization method. These steps reduce the influence of batch effect on experimental results. Then the “DESeq2” package was applied for differential analysis of count data in order to estimate variance-mean dependence in count data from high-throughput sequencing assays. Differential expression was based on a model using the negative binomial distribution. Subsequently, the data (i.e., P-value, fold changes, or effect sizes) were extracted. Based on this overall evidence, we identified RNAs that were significantly different in expression. RNAs with P < 0.01 and log2 fold change ≥ |1| were considered significantly different exoLRs. The “ClusterProfiler” package was used to investigate Gene Ontology (GO) enrichment analysis including biological processes, cellular components, and molecular functions (20). P < 0.05 was selected as the cut-off value for enriched function.
Co-expression Networks of Exosomal mRNAs
Exosomal mRNAs were initially filtered if expression values were <1 fragment per kilobase per million (FPKM) in at least 90% of the samples. The remaining mRNAs with standard deviations >0.2 were fed into an R package for weighted correlation network analysis (21).
Appropriate soft-threshold power was selected to ensure the co-expression network based on scale-free topology. The weighted adjacencies and correlations were transformed into a topological overlap matrix (TOM), followed by calculation of the corresponding dissimilarity (1-TOM). Next, 1-TOM, as the distance measure, was applied to a hierarchical clustering analysis of genes. A dynamic tree cut algorithm was used to identify modules. Based on module eigengene and clinical trait correlations, significant modules were identified with P < 0.05. The top 50 exosomal mRNA connections, based on topological overlap in significant modules, were used to construct a network diagram using Cytoscape (22). Exosomal mRNAs with eigengene connectivity > 0.8, within the module, were related to clinical traits and considered candidate hub genes (23).
Statistical Analysis
RNA expression levels are shown as means of FPKM. Comparative Venn diagrams were constructed using Venny 2.1.0 (https://bioinfogp.cnb.csic.es/tools/venny/index.html). Principal component analysis (PCA) was applied to evaluate variables within the three groups. Data were transformed into log2 scale and plotted using the plotPCA function in R v.3.5.2. Continuous variables that were normally distributed are displayed as means with standard deviation. One-way ANOVA followed by post-test least significant difference was performed to assess the difference among multiple groups. P < 0.05 was considered statistically significant. Pearson's correlation coefficient was calculated to test statistical correlation and r > 0.5 or r < −0.5 with a P < 0.05 was considered statistically significant. The area under the curve (AUC) of receiver operating characteristic (ROC) was applied to evaluate the specificity and sensitivity of exosomal mRNA for AMI diagnosis with 95% confidence interval (95% CI) calculated. Due to the relatively small sample size for ROC analysis, statistical power was calculated using PASS (version 15.0) with the following conditions: α = 0.05, AUC = 0.5, and n = 30. P < 0.05 was considered statistically significant. PASW Statistics 18.0 software was used for all statistical analyses.
Results
Clinical Baseline Characteristics of Patients
The clinical characteristics of the three groups (10 AMI, 8 CAD, and 10 controls) are presented in Table 1. Of the 10 AMI patients, nine presented with ST-elevated myocardial infarction and one with non-ST-elevated myocardial infarction. The CAD group and control groups had similar acute inflammation levels, whereas the AMI group had significantly higher levels at study entry. MB isoenzyme of creatine kinase (CKMB) and hydroxybutyrate-dehydrogenase (HBDH) were also higher in AMI patients than in the other subjects.
Brief Workflow for Plasma ExoLRs-Seq for Group Characterization
Reliable exoLRs-seq data were obtained by plasma isolation, purification of exosomes, exosome RNA extraction, and RNA-seq library construction (Figure 1A). TEM results showed membrane-enclosed exosome structures without similar size or uniform distribution (Figure 1B). The average diameter of the isolated exosomes was 75.83 nm measured with a NanoSight instrument (Figure 1C). Membrane markers of exosomes, CD9 and CD63, were demonstrated by Western blot (Figure 1D). mRNA constituted 58.46% of total mapped reads. Pseudogenes and circRNAs accounted for 12.80 and 11.73%, respectively, whereas lncRNAs and antisense RNAs were 7.94 and 7.55%, respectively (Figure 1E). The number of mRNAs, circRNAs, lncRNAs, and pseudogenes for the AMI group were all significantly higher than the control group (Figure 1F). Compared with the CAD group, the AMI group had a significantly higher number of mRNAs (Figure 1F).
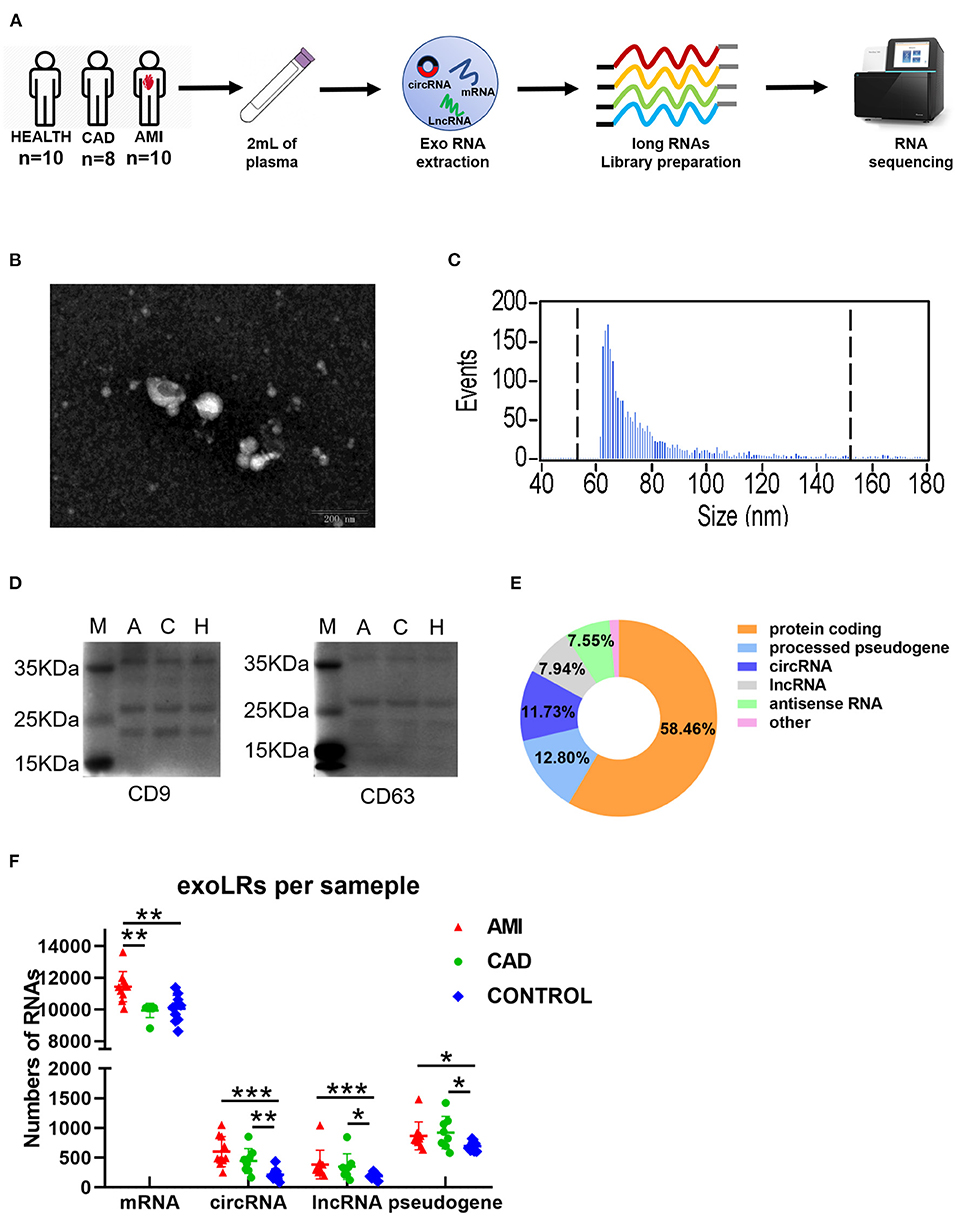
Figure 1. A brief view of the workflow of human plasma exosomal long RNA-seq and its characteristics in each group. (A) Work flow of exosomal long RNA-seq of human plasma. (B) Electron microscopy image of isolated exosomes. (C) Size distribution measurements of isolated exosomes. (D) Western blot analysis of exosomal markers (M, marker; A, acute myocardial infarction; C, coronary artery disease; H, healthy individuals). (E) Distribution of mapped reads to the genes with annotation and identified circRNA. (F) The number of exoLRs for each group displayed by scatterplot. Results are described as the mean ± SD (*P < 0.05, **P < 0.01, and ***P < 0.001; Exo RNA, exosomal RNA; exoLRs, exosomal long RNAs).
ExoLRs May Reflect Immune Cell Types
Twenty-two types of immune cells were assessed using the exosomal sequencing data with an established computational resource (CIBERSORT) (Figure 2A). PCA of the immunological profiles showed a non-uniform distribution (Figure 2C). Memory B cells were least in the CAD group, whereas naïve B cells of the CAD group were the highest. Neutrophils and monocytes were significantly enriched in the AMI group, which is consistent with clinical baseline characteristics (Figure 2B). Results suggest that circulating exoLRs may reflect the circulating immune cell profile.
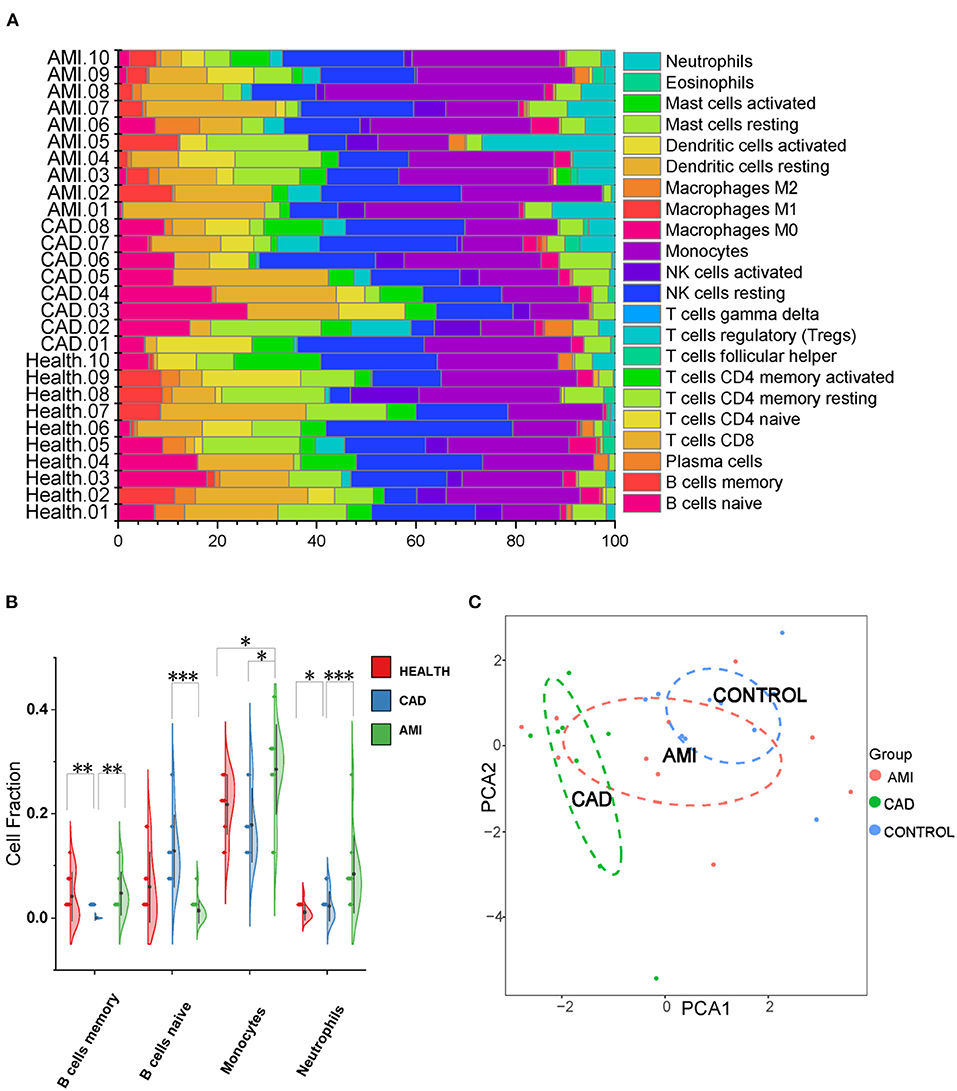
Figure 2. The Exosomal long RNA reflected relative fractions of different immune cell types. (A) Relative fraction of twenty-two types of immunocyte assessed from exosomal long RNA-seq data by CIBERSORT. (B) Violin plot of the fraction of four types of immune cells. Results are presented as the mean ± SD (*P < 0.05, **P < 0.01, and ***P < 0.001). (C) The PCA of all types of immune cells.
Comparative ExoLR Identification and Functional Enrichment Analysis of AMI and Control Groups
Compared with the control group, 296 different exosomal mRNAs, consisting of 254 up- and 42 down-regulated (Supplementary Table 1), were identified for the AMI group. The top 15 up and top 15 down regulated exosomal mRNAs are shown in Table 2. Only 16 different circRNAs or lncRNAs were identified (Figures 3A,B). ClusterProfiler was used to analyze and visualize functional profiles (Gene Ontology) of the 296 different exosomal mRNAs with identification of enrichment maps of biological processes by https://www.networkanalyst.ca. Results of the functional profile analysis (Supplementary Table 2) are shown as a bar plot (Figure 3C) and the enrichment map of biological processes as a network (Figure 3D). Among the top 10 biological processes in Figures 3C,D, neutrophil degranulation, neutrophil activation, and neutrophil activation involved in immune response were significantly enriched. The enrichment map (Figure 3D) showed that the inflammatory response may be the core biological process.
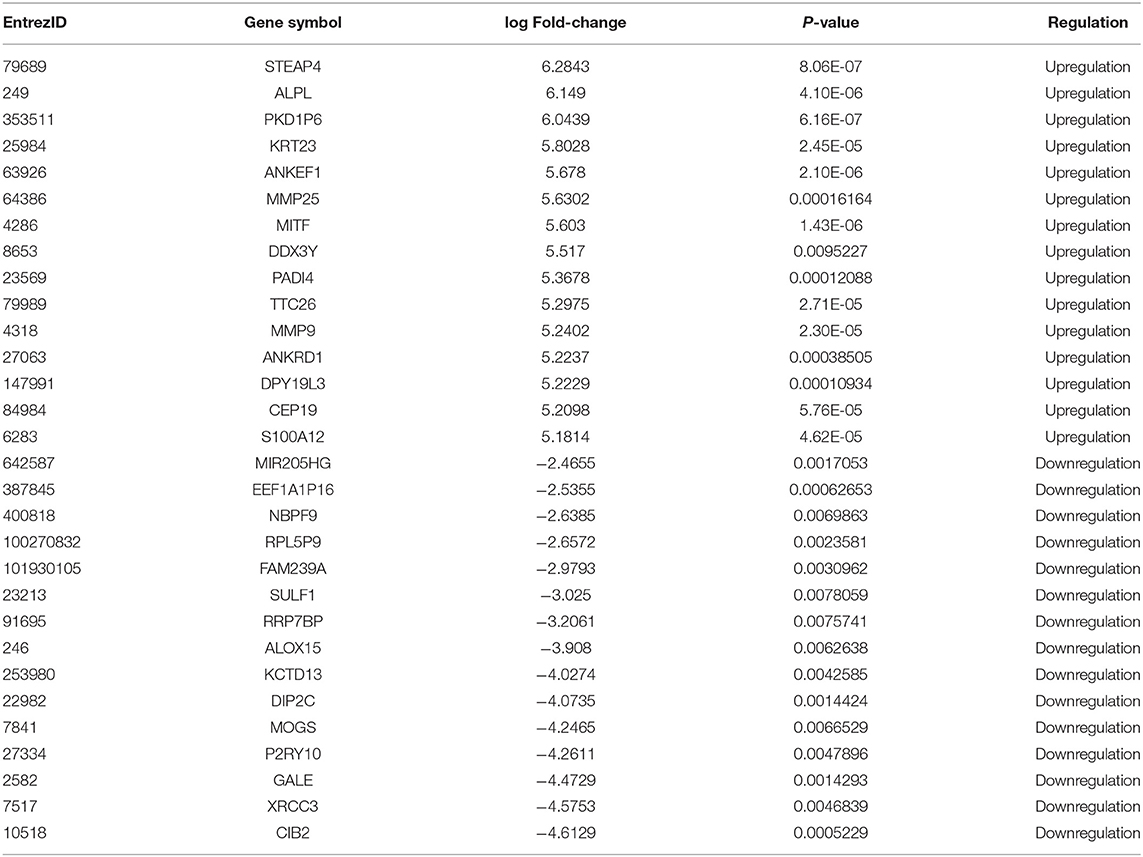
Table 2. The top 15 upregulated and top 15 downregulated exosomal mRNAs in AMI group compared with the control group.
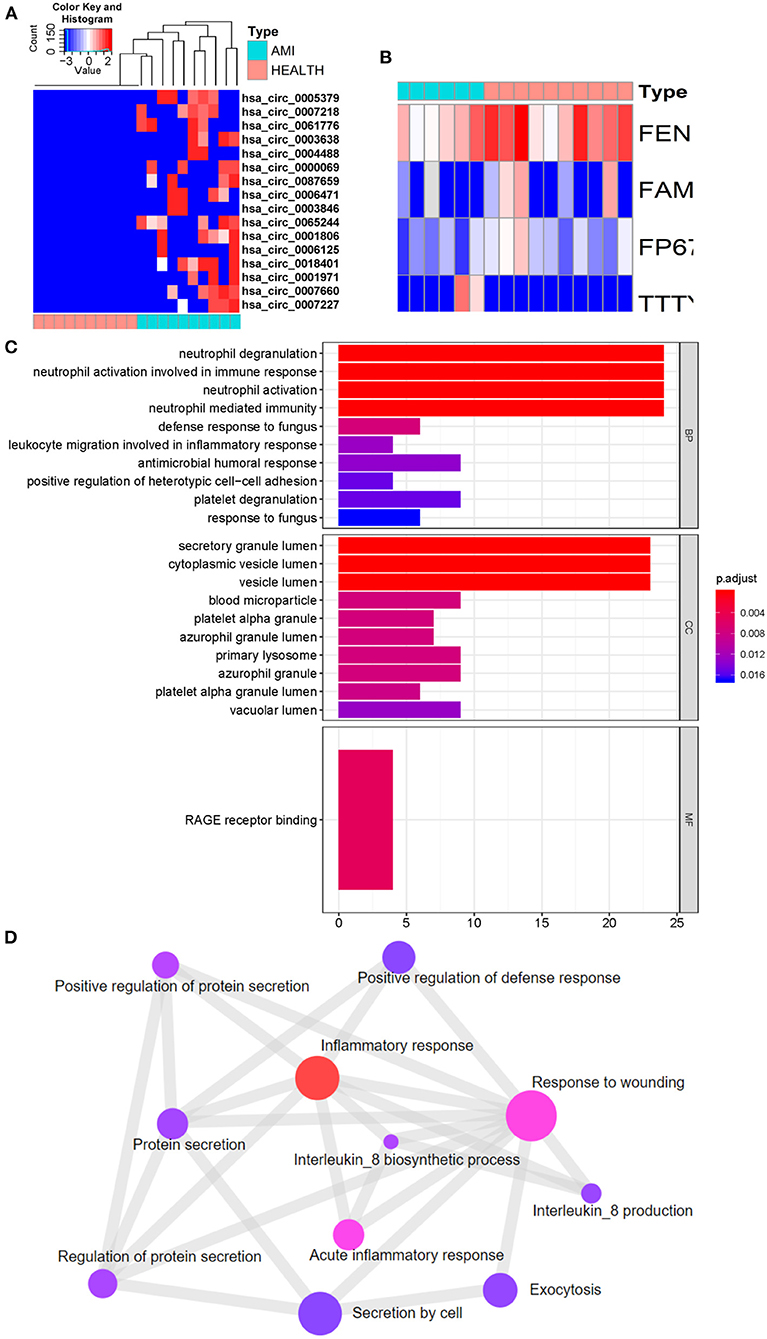
Figure 3. Identification of different exosomal long RNAs and functional enrichment analysis between the AMI and the control. (A) Heatmap of significantly circRNA between AMI and control. (B) Heatmap of significantly lncRNA between AMI and control. (C,D) Functional enrichment analysis of significantly different exosomal mRNA.
By comparison of AMI and control exoLRs, we found that circulating AMI exosomal mRNAs may play a crucial role in the acute inflammation response mediated by neutrophils.
Comparative Exosomal mRNA Analysis of AMI and CAD Groups
When compared with the CAD group, 230 different exosomal mRNAs (Supplementary Table 3), consisting of 120 up- and 110 down-regulated, were identified for the AMI group (Figures 4A,B). Functional profile analysis (Figure 4C and Supplementary Table 4) found neutrophil activation to play a leading role in associated biological processes. To further investigate the relationship between AMI and CAD, intersections of differently up- or down-regulated exosomal mRNAs for the AMI, CAD, and control groups are depicted in Figures 4A,B. There were 35 different exosomal mRNAs (31 up- and 4 down-regulated) that overlapped between the AMI and control groups and AMI and CAD (Supplementary Table 5). GO analysis of these 35 different exosomal mRNAs (Supplementary Table 6) found that myeloid leukocyte activation was mainly enriched (Figure 4D). ALPL mRNA is especially worth noting in that it was the only up-regulated exosomal mRNA among the three groups (Figure 4A) and may serve as a potential biomarker for AMI diagnosis.
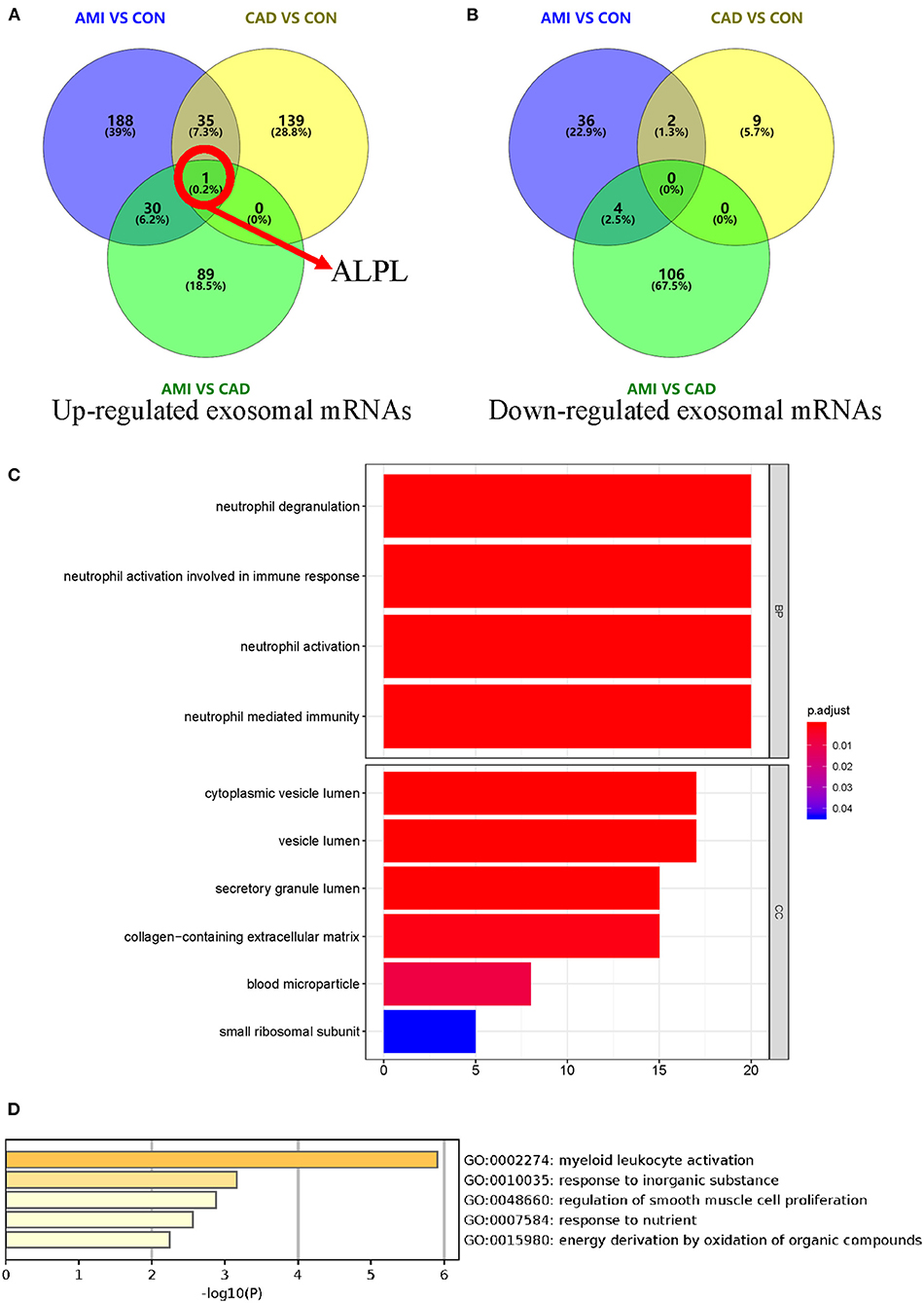
Figure 4. Identification of different exosomal long RNAs and functional enrichment analysis between AMI and CAD. (A,B) Comparation of differently up- or down-regulated exosomal mRNAs between AMI and control, AMI and CAD, and CAD and control. These three comparison sets were intersected with each other. (C) Functional enrichment analysis of significantly different exosomal mRNA between AMI and CAD. (D) Gene ontology analysis result of 35 different exosomal mRNAs between the comparison of AMI and control and comparison of AMI and CAD.
Co-expression Network Analysis of AMI Exosomal mRNAs
Weighted gene co-expression network analysis was used to identify key modules and highly correlated exosomal mRNAs associated with AMI. Forty-three exosomal mRNAs modules were identified by hierarchical clustering dendrogram (Figure 5A). The association of the 43 co-expression modules was analyzed by topological overlap matrix plot that consisted of the modules and the corresponding hierarchical clustering dendrogram (Figure 5B). For module-trait analyses, only the light-yellow module containing 175 exosomal mRNAs (Supplementary Table 7) was related to AMI (Figure 5C). Although there was no significantly enriched Kyoto Encyclopedia of Genes and Genomes (KEGG) biological pathways in the light-yellow module, the results of GO enrichment analysis indicated that the light-yellow module was involved in inflammation mediated by neutrophils, i.e., neutrophil aggregation and chemokine production (Figure 5D). The association between the light-yellow module and AMI was also supported by enrichment analyses of different exosomal mRNAs between AMI (Figure 3C) and control or between AMI and CAD (Figure 4D). To further analyze the core mRNA of the light-yellow module, the top 50 exosomal mRNAs of the topological overlap matrix of this module were used to construct a network diagram (Figure 5E). In this network, exosomal mRNAs (with eigengene connectivity > 0.8) in the light-yellow module were considered core mRNAs and labeled with yellow (Figure 5E). Six mRNAs including ALPL, CXCR2, ELL2, EMC9, FAM129A, and DBF4B were identified as core mRNAs.
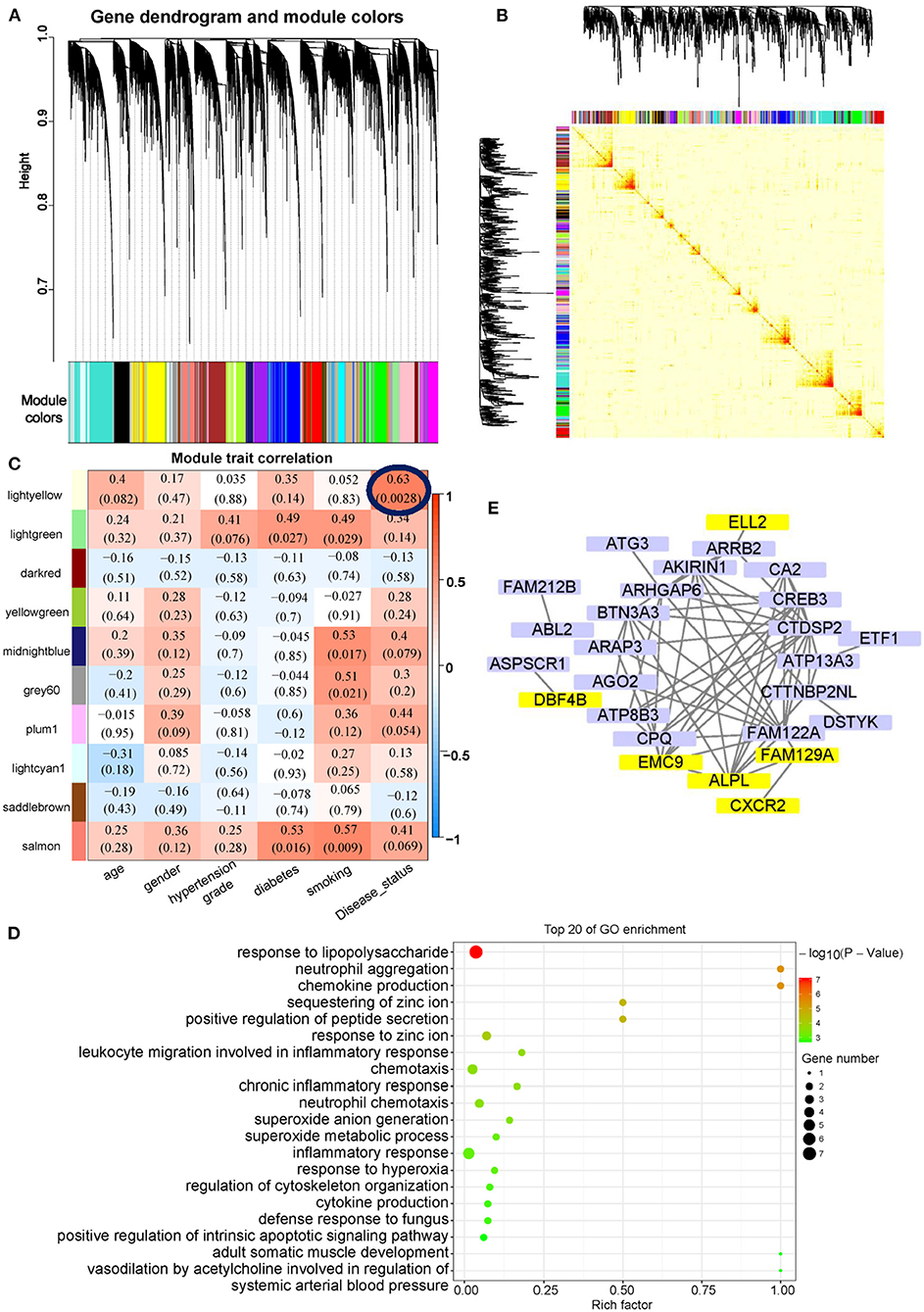
Figure 5. Co-expression network analysis of exosomal mRNAs in AMI. (A) Cluster diagram showing co-expression modules identified by WGCNA. (B) The heat map plot showed the topological overlap matrix (TOM) among all mRNAs. Light color shows low overlap, and red color indicates a higher overlap. The left side and the top side show the gene dendrogram and module assignment. (C) Heatmap of modules-trait relationship. (D) GO enrichment analysis of light-yellow modules. (E) Network visualization of the top 50 exosomal mRNAs of the topological overlap matrix in light-yellow modules. The exosomal mRNA with eigengene connectivity > 0.8 were highlighted in yellow color.
Potential Clinical Value of Exosomal ALPL and CXCR2
To estimate the potential clinical value of the six core exosomal mRNAs, ROC curve analysis was applied (Figure 6A). AUC indicated that there were two mRNAs with excellent predictive accuracy ALPL (AUC: 0.99, 95%CI, 0.9484–1.000, P = 0.0002, power = 0.99754) and CXCR2 (AUC: 0.98, 95%CI, 0.8478–1.000, P = 0.0005, power = 0.68124)]. Correlational analysis of these two exosomal mRNAs and clinical baseline characteristics (Figure 6B) indicated that ALPL and CXCR2 are associated with neutrophil count (R = 0.52 and 0.51, respectively). Statistical analysis suggested expression of exosomal mRNA ALPL and CXCR2, derived from AMI plasma, to be significantly higher than that found in the other groups (Figures 6C,D). Exosomal ALPL in the CAD group was also significantly higher than the control group. These results suggest that circulating exosomal ALPL may increase with the progression of coronary plaque to acute myocardial infarction.
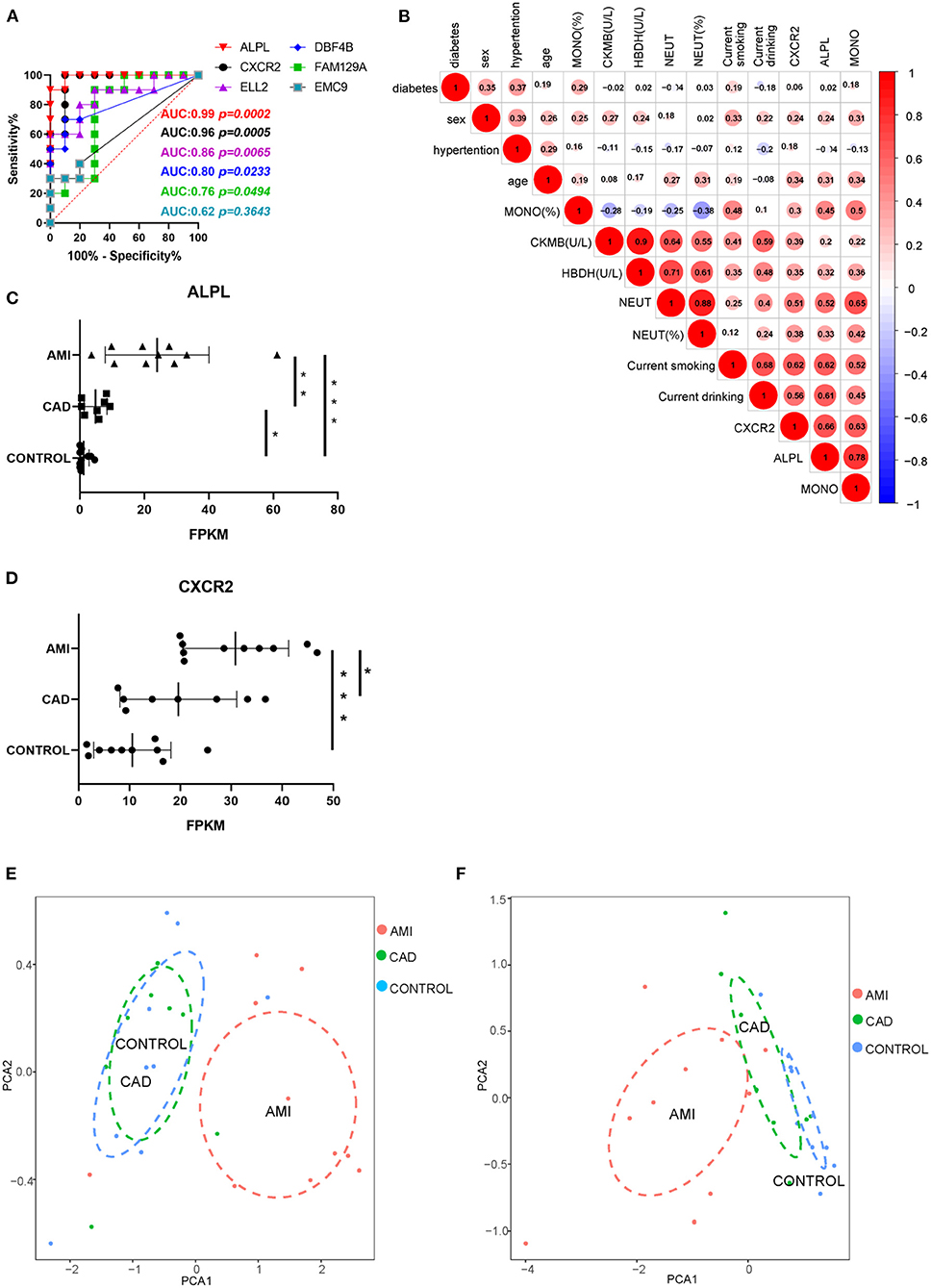
Figure 6. Potential clinical value of ALPL and CXCR2. (A) The ROC curve analysis of six core exosomal mRNAs. (B) The correlation analysis between these two exosomal mRNAs and clinical baseline characteristics. (C,D) The statistical analysis of expression of ALPL and CXCR2 among AMI, CAD, and control group. Results are presented as the mean ± SD (*P < 0.05, **P < 0.01, and ***P < 0.001). (E) PCA of neutrophil count and neutrophil ratio. (F) PCA of the expression of ALPL and CXCR2.
Since this analysis found AMI exosomal mRNAs to be associated with acute inflammation mediated by neutrophils, we applied PCA to the neutrophil count and neutrophil ratio for the three groups. PCA of the neutrophil count and neutrophil ratio showed that although the AMI group could be separate from the other groups, there was much overlap between the CAD and control groups (Figure 6E). However, when we applied PCA to the expression of ALPL and CXCR2, the three groups could be separated (Figure 6F). In summary, exosomal ALPL and CXCR2 have the predictive potential to provide greater accuracy and identification of cardiac disease.
Discussion
Interestingly, when exosomes were initially discovered, they were considered to be waste cargo (24). However, when RNA was detected in exosomes, they were considered as promising tools for the treatment and diagnosis of diseases especially cancer (25). Long RNA species (mRNA, lncRNA, and circRNA) are found in human blood exosomes with potential clinical usefulness (26). These exosomes could serve as diagnostic markers but could also play a significant role in cell-to-cell communication by activating biological signaling pathways (27). Such exosomal RNAs could reflect and affect the progression of disease.
Exosomal microRNAs have been extensively evaluated with relation to cardiovascular disease but very few studies have assessed the features and potential clinical value of exosomal mRNAs. In this study, we found that plasma exoLRs largely consist of mRNAs. In AMI patients, alterations in these mRNAs could indicate neutrophilic inflammation of the circulatory system. Among these mRNAs, we found ALPL and CXCR2 to have good predictive accuracy and may be potential biomarkers for AMI diagnosis.
The exoLRs sequencing data from this study demonstrate plasma exoLRs to be primarily mRNAs. It is not surprising that exosomal circRNAs are expressed in low abundance since they have specific spatiotemporal expression patterns (28–30). A relatively high number of long RNAs were identified in AMI samples and it is worth noting that the AMI samples in this study were from relatively aged patients. Previous studies have demonstrated aging to impact circulating extracellular vesicle concentration, size, and cargo (31, 32). Furthermore, stressors such as hypoxia, inflammation, and injury can induce cardiomyocytes or other cells to secret exosomes (33).
To provide a better understanding of exosomal mRNAs in AMI patients, we used a bioinformatic approach to compare AMI and CAD patients to healthy individuals. We also used co-expression network analysis to investigate the exosomal mRNAs of AMI patients. Results demonstrated the acute inflammatory response mediated by neutrophils to be the core biological process in AMI patients. These findings are consistent with current AMI studies. Since the cardiomyocyte is extremely sensitive to ischemic injury, reduced blood supply to the myocardium could initially cause injury and lead to an intense inflammatory response once AMI had occurred (34, 35). Furthermore, neutrophil extracellular traps have been identified as crucial triggers and structural contributors to various forms of thrombosis (36). Furthermore, neutrophilic inflammation was found to influence infarct size, healing, and cardiac function after myocardial infarction (37). Although the exact physiological role of exosome in AMI is still poorly understood, inflammation-related alterations in exosomal RNAs are associated with the biological process of AMI.
Since miscellaneous immune cells take part in cardiac repair during phases of cardiovascular disease (38), neutrophils have been traditional biomarkers (39). Although the diagnosis of acute myocardial infarction is dependent on an elevation of the serum levels of cardiac-specific troponin I, troponin T, or the myocardial band isoenzyme of creatine kinase (CK–MB), there is still a lack of biomarkers that evaluate the inflammatory response mediated by neutrophils. In this study, we found two potential biomarkers, exosomal mRNAs ALPL and CXCR2, to be specifically enriched in circulating neutrophils. Results confirmed with the Human Protein Atlas (http://www.proteinatlas.org) (40).
ALPL has been shown to regulate cardiac fibrosis during myocardial infarction through TGF-β1/Smads and P53 signaling pathways (41). Similarly, an ALPL inhibitor was found to be a potential treatment for cardiovascular disease by attenuating arterial calcification in a non-chronic kidney disease context (42). The expression level of exosomal mRNA ALPL in the AMI groups was the greatest, followed by CAD group, with healthy individuals having the lowest. Whether exosomal mRNA ALPL increases during the progression of coronary plaque to acute myocardial infarction requires further study.
CXCR2 is an intriguing biomolecule that has cardio-protective effects and the capacity to reduce myocardial damage after myocardial ischemia-reperfusion injury (43, 44). Furthermore, CXCR2 may play a crucial cardio-protective role in myocardial infarction through enhanced myeloid progenitor production and upregulation of cardiac adhesion molecules (37, 45). One recent study reported a process of temporal neutrophil polarization in the ischemic heart, with N1 polarized pro-inflammatory neutrophils infiltrating the heart early after AMI, while the proportion of N2 polarized anti-inflammatory neutrophils increased (46). Another interestingly recent single-cell transcriptomics study investigating temporal neutrophil diversity in the blood and heart after murine myocardial infarction indicated that all neutrophils highly expressed CXCR2, and its surface level was slightly increased in SiglecFhi vs. SiglecFlow neutrophils at day 3 (47). Therefore, it is essential to investigate the involvement of biomarkers related to neutrophils. Our data show circulating exosomal mRNA CXCR2 to be a potential biomarker for AMI with high diagnostic efficiency and constituted a resource for further investigation of the functional implications of neutrophils in myocardial infarction. Further studies are required to understand the mechanistic basis for secretion of exosomal mRNA CXCR2.
Circulating plasma exosomes are known to interact with a variety of cell types and tissue. However, the exact physiological or purpose of plasma exosomal mRNAs is poorly understood. The functionality of exosomal mRNAs depends upon whether they are intact or in fragments. In fact, some exosomal mRNAs have been found to be full length and functional (48).
Multiple limitations of the present study should be acknowledged. First, the sample size of this study was relatively small. Further studies are required that assess a larger sample size in a validated and prospective clinical trial. Second, due to the small number of patients, the effect of confounding factors, especially patients' age and gender were difficult to exclude. Third, blood samples were collected immediately at admission before coronary angiography, however, the occurred time of AMI might varied from patient to patient. This could influence the results because the composition of exosomal exoLRs would change remarkablely after AMI in a time-dependent manner. Fourth, although the result of average diameter (Figure 1C) showed that the extracellular vesicles extracted from plasma were mainly composed of exosomes, other extracellular vesicles was still contaminated during the isolation of exosomes.
In conclusion, our study explored exoLRs in AMI patients and found an association with the acute inflammatory response mediated by neutrophils. Moreover, we found that exosomal mRNAs, ALPL and CXCR2, may serve as potential useful biomarkers of the acute inflammatory response mediated by neutrophils in AMI.
Data Availability Statement
The datasets presented in this study can be found in online repositories. The names of the repository/repositories and accession number(s) can be found below: https://www.ncbi.nlm.nih.gov/geo/ (GSE159657).
Ethics Statement
The studies involving human participants were reviewed and approved by Guangdong Provincial People's Hospital (No. 2018160A). The patients/participants provided their written informed consent to participate in this study.
Author Contributions
G-dH and Y-qH coordinated the testing of samples and performed the bioinformatics analyses. LL, J-yH, and KL performed the statistical analyses. Y-lY and C-lC collected the samples. BZ and Y-qF designed the original study and drafted the manuscript. All authors critically reviewed and approved the final manuscript.
Funding
This research was funded by Science and Technology Plan Program of Guangzhou (No. 201803040012), the Key Area R&D Program of Guangdong Province (No. 2019B020227005), Guangdong Provincial People's Hospital Clinical Research Fund (Y012018085), the Fundamental and Applied Basic Research Foundation Project of Guangdong Province (2020A1515010738), and the Climbing Plan of Guangdong Provincial People's Hospital (DFJH2020022).
Conflict of Interest
The authors declare that the research was conducted in the absence of any commercial or financial relationships that could be construed as a potential conflict of interest.
Publisher's Note
All claims expressed in this article are solely those of the authors and do not necessarily represent those of their affiliated organizations, or those of the publisher, the editors and the reviewers. Any product that may be evaluated in this article, or claim that may be made by its manufacturer, is not guaranteed or endorsed by the publisher.
Acknowledgments
We appreciate Guangzhou Epibiotek Co., Ltd., for excellent technical assistance.
Supplementary Material
The Supplementary Material for this article can be found online at: https://www.frontiersin.org/articles/10.3389/fcvm.2021.712061/full#supplementary-material
References
1. van Niel G, D'Angelo G, Raposo G. Shedding light on the cell biology of extracellular vesicles. Nat Rev Mol Cell Biol. (2018) 19:213–28. doi: 10.1038/nrm.2017.125
2. Wiklander OPB, Brennan M, Lötvall J, Breakefield XO, El Andaloussi S. Advances in therapeutic applications of extracellular vesicles. Sci Transl Med. (2019) 11:eaav8521. doi: 10.1126/scitranslmed.aav8521
3. Camarena V, Sant D, Mohseni M, Salerno T, Zaleski ML, Wang G, et al. Novel atherogenic pathways from the differential transcriptome analysis of diabetic epicardial adipose tissue. Nutr Metab Cardiovasc Dis. (2017) 27:739–50. doi: 10.1016/j.numecd.2017.05.010
4. Boulanger CM, Loyer X, Rautou PE, Amabile N. Extracellular vesicles in coronary artery disease. Nat Rev Cardiol. (2017) 14:259–72. doi: 10.1038/nrcardio.2017.7
5. Shah R, Patel T, Freedman JE. Circulating extracellular vesicles in human disease. N Engl J Med. (2018) 379:958–66. doi: 10.1056/NEJMra1704286
6. Barile L, Milano G, Vassalli G. Beneficial effects of exosomes secreted by cardiac-derived progenitor cells and other cell types in myocardial ischemia. Stem Cell Investig. (2017) 4:93. doi: 10.21037/sci.2017.11.06
7. Shanmuganathan M, Vughs J, Noseda M, Emanueli C. Exosomes: basic biology and technological advancements suggesting their potential as ischemic heart disease therapeutics. Front Physiol. (2018) 9:1159. doi: 10.3389/fphys.2018.01159
8. Zhang J, Li S, Li L, Li M, Guo C, Yao J, et al. Exosome and exosomal microRNA: trafficking, sorting, and function. Genomics Proteomics Bioinformatics. (2015) 13:17–24. doi: 10.1016/j.gpb.2015.02.001
9. Kim KM, Abdelmohsen K, Mustapic M, Kapogiannis D, Gorospe M. RNA in extracellular vesicles. Wiley Interdiscip Rev RNA. (2017) 8:e1413. doi: 10.1002/wrna.1413
10. Godini R, Fallahi H, Ebrahimie E. Network analysis of inflammatory responses to sepsis by neutrophils and peripheral blood mononuclear cells. PLoS One. (2018) 13:e0201674. doi: 10.1371/journal.pone.0201674
11. Lv LL, Feng Y, Wen Y, Wu WJ, Ni HF, Li ZL, et al. Exosomal CCL2 from tubular epithelial cells is critical for albumin-induced tubulointerstitial inflammation. J Am Soc Nephrol. (2018) 29:919–35. doi: 10.1681/ASN.2017050523
12. Dong L, Lin W, Qi P, Xu MD, Wu X, Ni S, et al. Circulating long RNAs in serum extracellular vesicles: their characterization and potential application as biomarkers for diagnosis of colorectal cancer. Cancer Epidemiol Biomarkers Prev. (2016) 25:1158–66. doi: 10.1158/1055-9965.EPI-16-0006
13. Anderson JL, Morrow DA. Acute myocardial infarction. N Engl J Med. (2017) 376:2053–64. doi: 10.1056/NEJMra1606915
14. Montalescot G, Sechtem U, Achenbach S, Andreotti F, Arden C, Budaj A, et al. 2013 ESC guidelines on the management of stable coronary artery disease: the Task Force on the management of stable coronary artery disease of the European Society of Cardiology. Eur Heart J. (2013) 34:2949–3003. doi: 10.1093/eurheartj/eht296
15. Ibanez B, James S, Agewall S, Antunes MJ, Bucciarelli-Ducci C, Bueno H, et al. 2017 ESC Guidelines for the management of acute myocardial infarction in patients presenting with ST-segment elevation: the Task Force for the management of acute myocardial infarction in patients presenting with ST-segment elevation of the European Society of Cardiology (ESC). Eur Heart J. (2018) 39:119–77. doi: 10.1093/eurheartj/ehx393
16. Kim D, Langmead B, Salzberg SL. HISAT: a fast spliced aligner with low memory requirements. Nat Methods. (2015) 12:357–60. doi: 10.1038/nmeth.3317
17. You X, Conrad TO. Acfs: accurate circRNA identification and quantification from RNA-Seq data. Sci Rep. (2016) 6:38820. doi: 10.1038/srep38820
18. Newman AM, Liu CL, Green MR, Gentles AJ, Feng W, Xu Y, et al. Robust enumeration of cell subsets from tissue expression profiles. Nat Methods. (2015) 12:453–7. doi: 10.1038/nmeth.3337
19. Zhou Y, Zhou B, Pache L, Chang M, Khodabakhshi AH, Tanaseichuk O, et al. Metascape provides a biologist-oriented resource for the analysis of systems-level datasets. Nat Commun. (2019) 10:1523. doi: 10.1038/s41467-019-09234-6
20. Yu G, Wang LG, Han Y, He QY. clusterProfiler: an R package for comparing biological themes among gene clusters. Omics. (2012) 16:284–7. doi: 10.1089/omi.2011.0118
21. Langfelder P, Horvath S. WGCNA: an R package for weighted correlation network analysis. BMC Bioinformatics. (2008) 9:559. doi: 10.1186/1471-2105-9-559
22. Otasek D, Morris JH, Bouças J, Pico AR, Demchak B. Cytoscape Automation: empowering workflow-based network analysis. Genome Biol. (2019) 20:185. doi: 10.1186/s13059-019-1758-4
23. Parikshak NN, Swarup V, Belgard TG, Irimia M, Ramaswami G, Gandal MJ, et al. Genome-wide changes in lncRNA, splicing, and regional gene expression patterns in autism. Nature. (2016) 540:423–7. doi: 10.1038/nature20612
24. Pan BT, Teng K, Wu C, Adam M, Johnstone RM. Electron microscopic evidence for externalization of the transferrin receptor in vesicular form in sheep reticulocytes. J Cell Biol. (1985) 101:942–8. doi: 10.1083/jcb.101.3.942
25. Skog J, Würdinger T, van Rijn S, Meijer DH, Gainche L, Sena-Esteves M, et al. Glioblastoma microvesicles transport RNA and proteins that promote tumour growth and provide diagnostic biomarkers. Nat Cell Biol. (2008) 10:1470–6. doi: 10.1038/ncb1800
26. Li S, Li Y, Chen B, Zhao J, Yu S, Tang Y, et al. exoRBase: a database of circRNA, lncRNA and mRNA in human blood exosomes. Nucleic Acids Res. (2018) 46:D106–D12. doi: 10.1093/nar/gkx891
27. Zhou R, Chen KK, Zhang J, Xiao B, Huang Z, Ju C, et al. The decade of exosomal long RNA species: an emerging cancer antagonist. Mol Cancer. (2018) 17:75. doi: 10.1186/s12943-018-0823-z
28. GlaŽar P, Papavasileiou P, Rajewsky N. circBase: a database for circular RNAs. Rna. (2014) 20:1666–70. doi: 10.1261/rna.043687.113
29. Momen-Heravi F, Bala S. Emerging role of non-coding RNA in oral cancer. Cell Signal. (2018) 42:134–43. doi: 10.1016/j.cellsig.2017.10.009
30. Yang L, Peng X, Li Y, Zhang X, Ma Y, Wu C, et al. Long non-coding RNA HOTAIR promotes exosome secretion by regulating RAB35 and SNAP23 in hepatocellular carcinoma. Mol Cancer. (2019) 18:78. doi: 10.1186/s12943-019-0990-6
31. Alibhai FJ, Lim F, Yeganeh A, DiStefano PV, Binesh-Marvasti T, Belfiore A, et al. Cellular senescence contributes to age-dependent changes in circulating extracellular vesicle cargo and function. Aging Cell. (2020) 19:e13103. doi: 10.1111/acel.13103
32. Borras C, Mas-Bargues C, Sanz-Ros J, Román-Domínguez A, Gimeno-Mallench L, Inglés M, et al. Extracellular vesicles and redox modulation in aging. Free Radic Biol Med. (2020) 149:44–50. doi: 10.1016/j.freeradbiomed.2019.11.032
33. Chistiakov DA, Orekhov AN, Bobryshev YV. Cardiac extracellular vesicles in normal and infarcted heart. Int J Mol Sci. (2016) 17:63. doi: 10.3390/ijms17010063
34. Toldo S, Abbate A. The NLRP3 inflammasome in acute myocardial infarction. Nat Rev Cardiol. (2018) 15:203–14. doi: 10.1038/nrcardio.2017.161
35. Vieira JM, Norman S, Villa Del Campo C, Cahill TJ, Barnette DN, Gunadasa-Rohling M, et al. The cardiac lymphatic system stimulates resolution of inflammation following myocardial infarction. J Clin Invest. (2018) 128:3402–12. doi: 10.1172/JCI97192
36. Michel JB, Ho-Tin-Noé B. Thrombi and neutrophils. Circ Res. (2015) 116:1107–8. doi: 10.1161/CIRCRESAHA.115.306050
37. Schloss MJ, Horckmans M, Nitz K, Duchene J, Drechsler M, Bidzhekov K, et al. The time-of-day of myocardial infarction onset affects healing through oscillations in cardiac neutrophil recruitment. EMBO Mol Med. (2016) 8:937–48. doi: 10.15252/emmm.201506083
38. Kubota A, Suto A, Suzuki K, Kobayashi Y, Nakajima H. Matrix metalloproteinase-12 produced by Ly6C(low) macrophages prolongs the survival after myocardial infarction by preventing neutrophil influx. J Mol Cell Cardiol. (2019) 131:41–52. doi: 10.1016/j.yjmcc.2019.04.007
39. Silvestre-Roig C, Braster Q, Ortega-Gomez A, Soehnlein O. Neutrophils as regulators of cardiovascular inflammation. Nat Rev Cardiol. (2020) 17:327–40. doi: 10.1038/s41569-019-0326-7
40. Pontén F, Jirström K, Uhlen M. The Human Protein Atlas–a tool for pathology. J Pathol. (2008) 216:387–93. doi: 10.1002/path.2440
41. Gao L, Wang LY, Liu ZQ, Jiang D, Wu SY, Guo YQ, et al. TNAP inhibition attenuates cardiac fibrosis induced by myocardial infarction through deactivating TGF-β1/Smads and activating P53 signaling pathways. Cell Death Dis. (2020) 11:44. doi: 10.1038/s41419-020-2243-4
42. Opdebeeck B, D'Haese PC, Verhulst A. Inhibition of tissue non-specific alkaline phosphatase; a novel therapy against arterial media calcification? J Pathol. (2020) 250:248–50. doi: 10.1002/path.5377
43. Tarzami ST, Miao W, Mani K, Lopez L, Factor SM, Berman JW, et al. Opposing effects mediated by the chemokine receptor CXCR2 on myocardial ischemia-reperfusion injury: recruitment of potentially damaging neutrophils and direct myocardial protection. Circulation. (2003) 108:2387–92. doi: 10.1161/01.CIR.0000093192.72099.9A
44. Liehn EA, Kanzler I, Konschalla S, Kroh A, Simsekyilmaz S, Sönmez TT, et al. Compartmentalized protective and detrimental effects of endogenous macrophage migration-inhibitory factor mediated by CXCR2 in a mouse model of myocardial ischemia/reperfusion. Arterioscler Thromb Vasc Biol. (2013) 33:2180–6. doi: 10.1161/ATVBAHA.113.301633
45. Adrover JM, Del Fresno C, Crainiciuc G, Cuartero MI, Casanova-Acebes M, Weiss LA, et al. A neutrophil timer coordinates immune defense and vascular protection. Immunity. (2019) 50:390–402.e310. doi: 10.1016/j.immuni.2019.01.002
46. Ma Y, Yabluchanskiy A, Iyer RP, Cannon PL, Flynn ER, Jung M, et al. Temporal neutrophil polarization following myocardial infarction. Cardiovasc Res. (2016) 110:51–61. doi: 10.1093/cvr/cvw024
47. Vafadarnejad E, Rizzo G, Krampert L, Arampatzi P, Arias-Loza AP, Nazzal Y, et al. Dynamics of cardiac neutrophil diversity in murine myocardial infarction. Circ Res. (2020) 127:e232–e49. doi: 10.1161/CIRCRESAHA.120.317200
Keywords: acute myocardial infarction, exosomes, mRNAs, acute inflammatory response, neutrophil, WCGNA
Citation: He G-d, Huang Y-q, Liu L, Huang J-y, Lo K, Yu Y-l, Chen C-l, Zhang B and Feng Y-q (2021) Association of Circulating, Inflammatory-Response Exosomal mRNAs With Acute Myocardial Infarction. Front. Cardiovasc. Med. 8:712061. doi: 10.3389/fcvm.2021.712061
Received: 19 May 2021; Accepted: 29 July 2021;
Published: 19 August 2021.
Edited by:
Tomas G. Neilan, Massachusetts General Hospital and Harvard Medical School, United StatesReviewed by:
Jun-ichiro Koga, Kyushu University, JapanTimothy P. Fitzgibbons, University of Massachusetts Medical School, United States
Copyright © 2021 He, Huang, Liu, Huang, Lo, Yu, Chen, Zhang and Feng. This is an open-access article distributed under the terms of the Creative Commons Attribution License (CC BY). The use, distribution or reproduction in other forums is permitted, provided the original author(s) and the copyright owner(s) are credited and that the original publication in this journal is cited, in accordance with accepted academic practice. No use, distribution or reproduction is permitted which does not comply with these terms.
*Correspondence: Ying-qing Feng, NjUxNzkyMjA5JiN4MDAwNDA7cXEuY29t; Bin Zhang, MzQxODk4OTM1MCYjeDAwMDQwO3FxLmNvbQ==
†These authors have contributed equally to this work