- Department of Radiology, Shunde Hospital, Southern Medical University, Foshan, China
Background: The triglyceride–glucose (TyG) index had been proposed as a reliable surrogate marker of insulin resistance. We aimed to evaluate the association between TyG index and myocardial fibrosis, which was quantified by extracellular volume (ECV) fraction using cardiovascular magnetic resonance (CMR) examination, and their prognostic value in patients with heart failure (HF).
Methods: In this retrospective cohort study, 103 hospitalized HF patients were included. ECV fraction was calculated using CMR measurements and T1 mapping. TyG index was calculated using fasting triglyceride and blood glucose. The primary outcome events were defined as all-cause mortality and HF hospitalization during follow-up.
Results: During the median follow-up of 12.3 months, 39 patients (37.9%) experienced primary outcome events and had higher levels of TyG index, N-terminal pro–B-type natriuretic peptide (NT-proBNP), and ECV fraction compared with those without events. Multivariate linear regression analysis showed that the TyG index was the significant factor determined for ECV fraction (rpartial = 0.36, P = 0.01). In multivariate Cox regression analysis, presence of diabetes [hazard ratio (HR) = 1.28, 95% confidence interval (CI) = 1.01–1.62], higher TyG index (HR = 2.01, 95% CI = 1.03–4.01), ECV fraction (HR = 1.73, 95% CI = 1.04–2.88), and NT-proBNP (HR = 2.13, 95% CI = 1.08–4.20) were independent risk factors for the primary outcome events.
Conclusions: TyG index is a novel biomarker of myocardial fibrosis in HF patients and can be considered as a useful risk stratification metric in the management of HF.
Introduction
Epidemiological studies have shown that heart failure (HF) is a growing global public health burden, with prevalence up to 1 to 2% in the adult population (1). Detection of novel biomarkers and mechanisms would be of significant clinical importance for the prevention and treatment of HF (2, 3). It had been documented that metabolic disorders, including obesity, insulin resistance, and diabetes mellitus (DM) play an important role in the development and progression of HF (4). The triglyceride–glucose (TyG) index, a metric derived from fasting triglyceride and blood glucose levels, had been proposed as a reliable surrogate marker of insulin resistance (5). Previous studies had reported that a higher TyG index was associated with an increased risk of cardiovascular disease (CVD) (6, 7), chronic kidney disease (8), and diabetic retinopathy (9). It had also been reported that there is a positive correlation between the TyG index and the prognosis in patients with HF and type 2 DM (10). However, the underlying mechanisms were unexplored.
Cardiovascular magnetic resonance (CMR) imaging has emerged as a novel, non-invasive diagnostic tool to assess myocardial fibrosis (11), a key pathological process in HF (12). The importance of myocardial fibrosis to predict the prognosis in different cohorts of patients had been reported (13); however, there are limited clinical data on the interaction between insulin resistance and myocardial fibrosis in patients with HF.
This study investigated the association between TyG index and myocardial fibrosis among patients with advanced HF. We also determined the possibility that TyG index and myocardial fibrosis could serve as new biomarkers for the prognosis in patients with HF.
Methods
Study Design and Population
We retrospective reviewed hospitalized HF patients (aged ≥18 years) with CMR examination from January 2019 to December 2020. Patients with acute myocardial infarction, history of malignancy, sepsis, severe renal function failure [estimated glomerular filtration rate (eGFR) <30 mL/min per 1.73 m2 or under renal replacement therapy], severe anemia (Hb <60 g/L), autoimmune disease, heart transplantation, or severe hepatic disease or without data for calculating TyG index were excluded.
The study complied with the principles of the Declaration of Helsinki and was approved by the committee of the institutional review board at Shunde Hospital, Southern Medical University, China (no. 20200320). Because of the retrospective design of the current study, the patient informed consent form was waived by the institutional review board.
Baseline Characteristics, TyG Index Detection, and CMR Examination
We collected baseline characteristics, clinical data, and biochemistry tests from the hospital medical records. Hypertension was defined as systolic blood pressure ≥140 mm Hg and/or diastolic blood pressure ≥ 90 mm Hg or received antihypertensive treatment according to the Chinese guideline for the diagnostic and management of hypertension (14). DM was defined as fasting blood glucose (FBG) ≥7.0 mmol/L and/or hemoglobin A1c (HbA1c) ≥6.5% or received antihyperglycemic medications (15). We calculated the eGFR according to the Modification of Diet in Renal Disease equation adapted for Chinese patients (16).
Fasting venous blood was collected, and biochemistry tests were performed in the second morning after the patients were admitted. Hemoglobin, FBG, HbA1c, total cholesterol (TC), triglycerides, low-density lipoprotein-C (LDL-C), high-density lipoprotein cholesterol, serum creatinine, high-sensitivity C-reactive protein (hs-CRP), and N-terminal pro–B-type natriuretic peptide (NT-proBNP) levels were detected. LDL-C was calculated using the Friedewald equation (17, 18). TyG index was calculated using the following: Ln [fasting triglycerides (mg/dL) × FBG (mg/dL)/2] (5).
Two experienced radiologists performed CMR measurements and T1 mapping during the hospitalization of the patients. Quantification of extracellular volume (ECV) fraction by T1-mapping technique in CMR imaging was used to assess myocardial fibrosis. The detailed procedure had been reported in the previous study (19). Briefly, all patients were examined in the supine position using a 3.0-T scanner (Skrya; Siemens Medical Solutions, Erlangen, Germany). A total dose of 0.1 mmol/kg gadobutrol (Gadavist, Bayer Healthcare Leverkusen, Germany) was injected at a rate of 2.0 to 3.0 mL/s; 10–15 min after contrast injection, short- and long-axis two-dimensional inversion recovery late gadolinium enhancement images were acquired to evaluate focal myocardial fibrosis. Pre- and post-contrast myocardial T1 were measured in six regions of interest in the myocardium (anterior, anterolateral, inferolateral, inferior, inferoseptal, anteroseptal) and in the left ventricular blood pool. We calculated the ECV fraction using the following formula (20), in which R1 represents 1/T1, myo pre and myo post represent the precontrast and postcontrast myocardial T1 values, respectively, and blood pre and blood post represent the precontrast and postcontrast blood pool T1 values, respectively.
Follow-Up and Endpoint Ascertainment
The primary outcomes in the current study were defined as composite endpoints of all-cause death or HF rehospitalization (21). Patients were followed by reviewing the electronic medical record and/or telephone interview with the participants (or the family members if patients were deceased). The follow-up duration was up to February 28, 2021.
Statistical Analysis
All the included patients were categorized as with or without primary outcome events during follow-up. Categorical variables are presented as numbers and percentages. Continuous variables are presented as mean and standard deviation (SD), or median and interquartile range. Baseline characteristics of patients with or without primary outcome events were compared using the χ2 test for categorical variables, Wilcoxon rank-sum test for non–normally distributed continuous variables, and two-tailed t-test for normally distributed continuous variables.
Non-Gaussian data, including NT-proBNP level, left ventricular ejection fraction, eGFR, hs-CRP, ECV fraction, and cholesterol level, were log2-transformed. We used the Pearson product–moment correlation coefficient (r) as a measure of linear association between covariates and ECV fraction and performed multivariate linear regression analysis to identify factors associated with levels of ECV fraction.
Patients were divided into three groups according to tertiles of TyG index, ECV fraction, and NT-proBNP levels. Kaplan–Meier curves were employed to evaluate the study endpoints over time, and the log–rank test was used to assess differences in outcome events among different groups. Predictors of outcome were analyzed through univariate and multivariate adjusted Cox regression analyses, and the hazard ratios (HRs) and corresponding 95% confidence intervals (CIs) were presented. Risk factors with P < 0.10 in univariable analysis were included in the multivariable Cox regression model. Finally, receiver operating characteristic (ROC) curves and the area under the curve (AUC) were used to identify the potential relationships among TyG index, ECV fraction, and NT-proBNP levels and the primary outcomes. We used the method proposed by Delong et al. for the comparisons of the difference between two AUCs (22).
Statistical analyses were performed using SPSS version 20.0 (IBM Corp, Armonk, NY, USA). All P-values were two-tailed, and a P < 0.05 was considered statistically significant.
Results
Baseline Characteristics of the Included HF Patients
We screened 119 HF patients who had received CMR examination; 16 were excluded because of predefined exclusion criteria (Figure 1). Finally, 103 patients (mean age = 58.3 years) were included in the analysis. The demographic and clinical characteristics of the included patients with and without events are presented in Table 1. Compared with patients without primary adverse outcomes during follow-up, those with events had higher levels of TyG index, NT-proBNP, and ECV fraction (all P < 0.001).
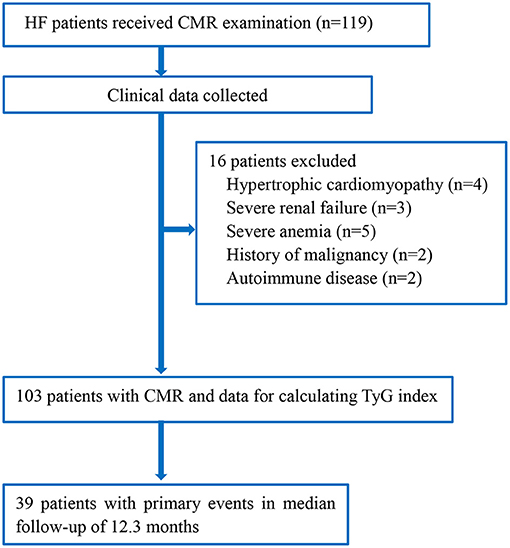
Figure 1. Flowchart of the study. CMR, cardiac magnetic resonance; HF, heart failure; TyG index, triglyceride–glucose index.
Associations of Clinical and Laboratory Variables With ECV Fraction
Pearson correlation analysis showed that Log2(TyG index) (r = 0.34, P = 0.01), Log2(hs-CRP) (r = 0.32, P = 0.02), and Log2(HbA1c) (r = 0.28, P = 0.04) were positively associated with ECV fraction (Table 2). However, in multivariate linear regression analysis, TyG index was the only significant factor determined for ECV fraction (rpartial = 0.36, P = 0.01). The R2 value of SFRP2 for ECV fraction was 0.13, which indicated that 13.0% of the total ECV fraction variation can be attributed to the TyG index.
Prognostic Value of Markers in HF Patients
During a mean follow-up period of 12.3 months (interquartile range = 9.2–16.9 months), 39 patients (37.9%) in the cohort experienced the composite primary outcome. Kaplan–Meier survival analysis showed that higher levels of TyG index (log–rank test for trend: P < 0.001), ECV fraction (log–rank test for trend: P < 0.001), and NT-proBNP (log–rank test for trend: P = 0.002) were significant predictors of composite primary outcomes (Figure 2). In multivariate Cox regression models, presence of diabetes (HR = 1.28, 95% CI = 1.01–1.62), higher TyG index (HR = 2.01, 95% CI = 1.03–4.01), ECV fraction (HR = 1.73, 95% CI = 1.04–2.88), and NT-proBNP (HR = 2.13, 95% CI = 1.08–4.20) were independent risk factors for the primary outcomes (Table 3).
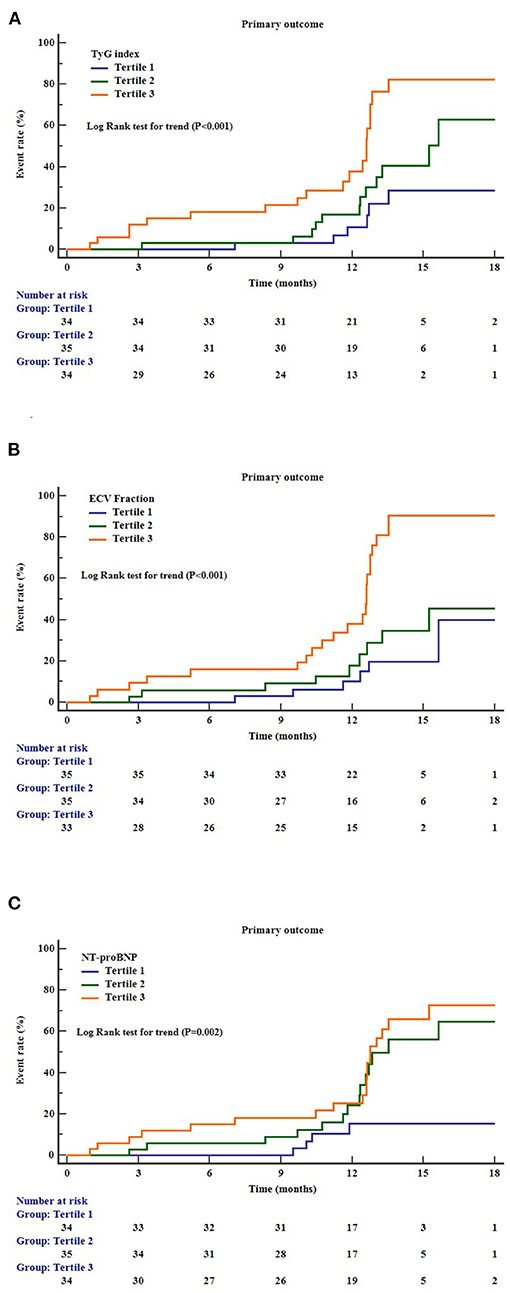
Figure 2. Cumulative incidence curves for the primary outcomes according to the different markers. (A) TyG index, triglyceride–glucose index; (B) ECV, extracellular volume; (C) NT-proBNP, N-terminal pro–B-type natriuretic peptide.
ROC analysis showed that levels of TyG index (AUC = 0.709, 95% CI = 0.611–0.794), ECV fraction (AUC 0.715, 95% CI = 0.618–0.80), and NT-proBNP (AUC 0.741, 95% CI = 0.646–0.823) had a significant predictive role on the primary outcomes (Figure 3). Pairwise comparisons of ROC curves showed that there were no significant differences in AUC among the three markers (all P > 0.63).
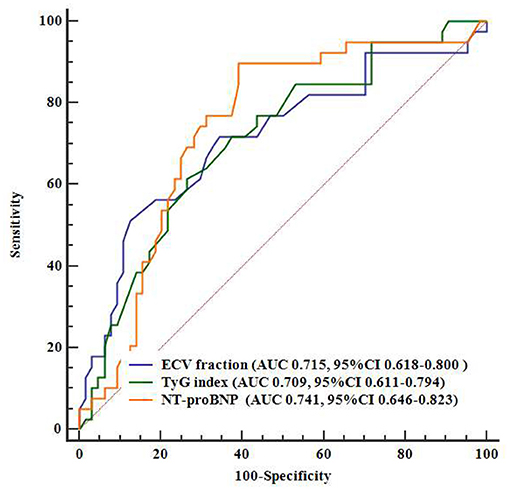
Figure 3. Comparisons between AUCs on ROCs. AUC, area under the curve; ECV, extracellular volume; NT-proBNP, N-terminal pro–B-type natriuretic peptide; ROC, receiver operating characteristics; TyG index, triglyceride–glucose index.
Discussion
To the best of our knowledge, this is the first study to report that the TyG index, a surrogate marker of insulin resistance, is positively associated with myocardial fibrosis detected by CMR. We also found that a higher TyG index was associated with poorer prognosis in HF patients after adjusting for other risk factors, including DM.
Myocardial fibrosis is a key pathological process and represents a potential therapeutic target for HF (23). The gold standard for evaluating myocardial fibrosis is myocardial biopsy. However, the invasive nature limits its clinical use. In the past decade, studies have demonstrated myocardial fibrosis estimated by CMR can provide important prognostic value (13, 23). In this study, we also found that a higher ECV fraction was associated with an increased adverse outcome in patients with HF, further supporting the notion that ECV fraction could be used as a non-invasive marker to evaluate myocardial fibrosis and risk stratification. Furthermore, we found that the TyG index was the only significant factor determined for ECV fraction, which provided the message that insulin resistance is an important risk factor for myocardial fibrosis. Our study was supported by another retrospective cohort study, which showed a positive correlation between the TyG index and the prognosis of patients with HF and type 2 DM (10). Several mechanisms involve the interaction among insulin resistance, myocardial fibrosis, and worse prognosis in HF. First, insulin resistance is associated with low-degree inflammation, which plays an important role in the pathogenesis of cardiomyocyte apoptosis and myocardial fibrosis (24). Second, insulin resistance has been associated with the increased sympathetic nervous system and renin–angiotensin–aldosterone system activity; both of them were involved in myocardial fibrosis and cardiac dysfunction (25, 26). Third, insulin resistance is related to the deposition of extracellular matrix deposition (27) and intramyocardial lipids, (28) resulting in subsequent myocardial fibrosis.
The homeostatic model assessment of insulin resistance (HOMA-IR) is another marker broadly used for defining insulin resistance. Because of the retrospective design, HOMA-IR data were not available in the current study. However, several studies aimed to compare the predictive effect of the TyG index and HOMA-IR for cardiovascular risk. In a cross-sectional study, the TyG index was still significantly associated with coronary artery stenosis in patients with type 2 DM (29). It had been reported that the TyG index was independently associated with arterial stiffness and 10-year CVD risk in a Chinese cohort, while in the same cohort, the association of the HOMA-IR and the 10-year CVD risk was absent when adjusting for multiple risk factors (6). Other studies also showed that the TyG index was more independently associated with increased arterial stiffness and coronary artery calcification than HOMA-IR in Korean (30, 31). Nowadays, the detection of metrics for calculating the TyG index (including fasting triglycerides and FBG) is convenient and affordable worldwide. However, the calculation for HOMA-IR was based on fasting insulin, which was much expensive than triglycerides and not available in most clinical laboratories. Therefore, we proposed that the TyG index could be considered as a more convenient marker of insulin resistance and regarded as a useful predictor of adverse prognosis in HF.
Some limitations to this study should be noted. First, the retrospective design of the current research would cause recall bias, and residual confounders could not be totally avoided. Prospective cohort studies would be useful to further ascertain the association between TyG index and myocardial fibrosis. Second, our study had a relatively small sample size, which limits us to further perform analysis based on patients with and without DM. However, in the multivariable Cox regression analysis, the association between TyG index and primary outcome events was still significant after adjusting for DM, which indicated that the higher TyG index was associated with a worse prognosis of HF independent of DM. Third, the TyG index was available only at baseline, not during follow-up. Thus, any changes in the TyG index that may have occurred in response to treatment of HF are unknown and require further exploration. Finally, the ECV fraction is a surrogate marker, not the gold standard of myocardial fibrosis. Therefore, the association between TyG index and ECV fraction is only an indirect evidence of myocardial fibrosis.
Conclusions
TyG index is a novel biomarker of myocardial fibrosis in HF patients. We also demonstrated that a higher TyG index was significantly associated with a worse prognosis in HF, which can be considered as a useful risk stratification metric in the management of HF.
Data Availability Statement
The raw data supporting the conclusions of this article will be made available by the authors, without undue reservation.
Ethics Statement
The studies involving human participants were reviewed and approved by Institutional review board at Shunde Hospital, Southern Medical University, China. Written informed consent for participation was not required for this study in accordance with the national legislation and the institutional requirements.
Author Contributions
SY, YD, and HC: conception, design, and administrative support. SY, YD, ZL, and RZ: data analysis and interpretation. SY, YD, ZL, and XL: manuscript writing. All authors provision of study materials or patients, collection and assembly of data, and final approval of manuscript.
Funding
The project was supported by Guangdong Provincial Administration of Traditional Chinese Medicine, China (Grant No. 20201344).
Conflict of Interest
The authors declare that the research was conducted in the absence of any commercial or financial relationships that could be construed as a potential conflict of interest.
References
1. Ziaeian B, Fonarow GC. Epidemiology and aetiology of heart failure. Nat Rev Cardiol. (2016) 13:368–78. doi: 10.1038/nrcardio.2016.25
2. Wu J, Zheng H, Liu X, Chen P, Zhang Y, Luo J, et al. Prognostic value of secreted frizzled-related protein 5 in heart failure patients with and without type 2 diabetes mellitus. Circ Heart Fail. (2020) 13:e7054. doi: 10.1161/CIRCHEARTFAILURE.120.007054
3. Li W, Huang A, Zhu H, Liu X, Huang X, Huang Y, et al. Gut microbiota-derived trimethylamine N-oxide is associated with poor prognosis in patients with heart failure. Med J Aust. (2020) 213:374–9. doi: 10.5694/mja2.50781
4. Cai X, Liu X, Sun L, He Y, Zheng S, Zhang Y, et al. Prediabetes and the risk of heart failure: a meta-analysis. Diabetes Obes Metab. (2021). doi: 10.1111/dom.14388. [Epub ahead of print].
5. Simental-Mendia LE, Rodriguez-Moran M, Guerrero-Romero F. The product of fasting glucose and triglycerides as surrogate for identifying insulin resistance in apparently healthy subjects. Metab Syndr Relat Disord. (2008) 6:299–304. doi: 10.1089/met.2008.0034
6. Guo W, Zhu W, Wu J, Li X, Lu J, Qin P, et al. Triglyceride glucose index is associated with arterial stiffness and 10-year cardiovascular disease risk in a Chinese population. Front Cardiovasc Med. (2021) 8:585776. doi: 10.3389/fcvm.2021.585776
7. Ding X, Wang X, Wu J, Zhang M, Cui M. Triglyceride-glucose index and the incidence of atherosclerotic cardiovascular diseases: a meta-analysis of cohort studies. Cardiovasc Diabetol. (2021) 20:76. doi: 10.1186/s12933-021-01268-9
8. Fritz J, Brozek W, Concin H, Nagel G, Kerschbaum J, Lhotta K, et al. The triglyceride-glucose index and obesity-related risk of end-stage kidney disease in Austrian adults. JAMA Netw Open. (2021) 4:e212612. doi: 10.1001/jamanetworkopen.2021.2612
9. Yao L, Wang X, Zhong Y, Wang Y, Wu J, Geng J, et al. the triglyceride-glucose index is associated with diabetic retinopathy in chinese patients with type 2 diabetes: a hospital-based, nested, case-control study. Diabetes Metab Syndr Obes. (2021) 14:1547–55. doi: 10.2147/DMSO.S294408
10. Guo W, Zhao L, Mo F, Peng C, Wang L, Xu Y, et al. The prognostic value of the triglyceride glucose index in patients with chronic heart failure and type 2 diabetes: a retrospective cohort study. Diabetes Res Clin Pract. (2021). doi: 10.1016/j.diabres.2021.108786. [Epub ahead of print].
11. Ramchand J, Desai MY. Clinical dilemmas in predicting the progression of pre-clinical hypertrophic cardiomyopathy-is MRI strain the solution? Ann Transl Med. (2019) 7 (Suppl 6):S177. doi: 10.21037/atm.2019.07.82
12. Kang SC, Sohn EH, Lee SR. Hydrogen sulfide as a potential alternative for the treatment of myocardial fibrosis. Oxid Med Cell Longev. (2020) 2020:4105382. doi: 10.1155/2020/4105382
13. Roy C, Slimani A, de Meester C, Amzulescu M, Pasquet A, Vancraeynest D, et al. Associations and prognostic significance of diffuse myocardial fibrosis by cardiovascular magnetic resonance in heart failure with preserved ejection fraction. J Cardiovasc Magn Reson. (2018) 20:55. doi: 10.1186/s12968-018-0477-4
14. Joint Committee for Guideline Revision. 2018 Chinese guidelines for prevention and treatment of hypertension-a report of the revision committee of Chinese guidelines for prevention and treatment of hypertension. J Geriatr Cardiol. (2019) 16:182–241. doi: 10.11909/j.issn.1671-5411.2019.03.014
15. Expert Committee on the Diagnosis and Classification of Diabetes Mellitus. Report of the expert committee on the diagnosis and classification of diabetes mellitus. Diabetes Care. (2003) 26 (Suppl. 1):S5–20. doi: 10.2337/diacare.26.2007.S5
16. Ma YC, Zuo L, Chen JH, Luo Q, Yu XQ, Li Y, et al. Modified glomerular filtration rate estimating equation for Chinese patients with chronic kidney disease. J Am Soc Nephrol. (2006) 17:2937–44. doi: 10.1681/ASN.2006040368
17. Martins J, Olorunju SA, Murray LM, Pillay TS. Comparison of equations for the calculation of LDL-cholesterol in hospitalized patients. Clin Chim Acta. (2015) 444:137–42. doi: 10.1016/j.cca.2015.01.037
18. Huang Y, Luo J, Liu X, Wu Y, Yang Y, Li W, et al. Gamma-Glutamyltransferase and risk of acute coronary syndrome in young chinese patients: a case-control study. Dis Markers. (2018) 2018:2429160. doi: 10.1155/2018/2429160
19. Yang S, Chen H, Tan K, Cai F, Du Y, Lv W, et al. Secreted frizzled-related protein 2 and extracellular volume fraction in patients with heart failure. Oxid Med Cell Longev. (2020) 2020:2563508. doi: 10.1155/2020/2563508
20. Bissell LA, Dumitru RB, Erhayiem B, Abignano G, Fent G, Kidambi A, et al. Incidental significant arrhythmia in scleroderma associates with cardiac magnetic resonance measure of fibrosis and hs-TnI and NT-proBNP. Rheumatology. (2019) 58:1221–6. doi: 10.1093/rheumatology/key430
21. Ng LL, Squire IB, Jones DJL, Cao TH, Chan DCS, Sandhu JK, et al. Proenkephalin, renal dysfunction, and prognosis in patients with acute heart failure: a great network study. J Am Coll Cardiol. (2017) 69:56–69. doi: 10.1016/j.jacc.2016.10.038
22. DeLong ER, DeLong DM, Clarke-Pearson DL. Comparing the areas under two or more correlated receiver operating characteristic curves: a nonparametric approach. Biometrics. (1988) 44:837–45. doi: 10.2307/2531595
23. Treibel TA, Fridman Y, Bering P, Sayeed A, Maanja M, Frojdh F, et al. Extracellular volume associates with outcomes more strongly than native or post-contrast myocardial T1. JACC Cardiovasc Imaging. (2020) 13 (1 Pt. 1):44–54. doi: 10.1016/j.jcmg.2019.03.017
24. Nishida K, Otsu K. Inflammation and metabolic cardiomyopathy. Cardiovasc Res. (2017) 113:389–98. doi: 10.1093/cvr/cvx012
25. Zhou MS, Schulman IH, Zeng Q. Link between the renin-angiotensin system and insulin resistance: implications for cardiovascular disease. Vasc Med. (2012) 17:330–41. doi: 10.1177/1358863X12450094
26. Fu Q. Sex differences in sympathetic activity in obesity and its related hypertension. Ann N Y Acad Sci. (2019) 1454:31–41. doi: 10.1111/nyas.14095
27. Sartori M, Ceolotto G, Papparella I, Baritono E, Ciccariello L, Calò L, et al. Effects of angiotensin II and insulin on ERK1/2 activation in fibroblasts from hypertensive patients. Am J Hypertens. (2004) 17:604–10. doi: 10.1016/j.amjhyper.2004.02.017
28. Lopaschuk GD, Ussher JR, Folmes CD, Jaswal JS, Stanley WC. Myocardial fatty acid metabolism in health and disease. Physiol Rev. (2010) 90:207–58. doi: 10.1152/physrev.00015.2009
29. Thai PV, Tien HA, Van Minh H, Valensi P. Triglyceride glucose index for the detection of asymptomatic coronary artery stenosis in patients with type 2 diabetes. Cardiovasc Diabetol. (2020) 19:137. doi: 10.1186/s12933-020-01108-2
30. Lee SB, Ahn CW, Lee BK, Kang S, Nam JS, You JH, et al. Association between triglyceride glucose index and arterial stiffness in Korean adults. Cardiovasc Diabetol. (2018) 17:41. doi: 10.1186/s12933-018-0692-1
Keywords: triglyceride-glucose index, heart failure, insulin resistance, biomarkers, myocardial fibrosis
Citation: Yang S, Du Y, Liu Z, Zhang R, Lin X, Ouyang Y and Chen H (2021) Triglyceride–Glucose Index and Extracellular Volume Fraction in Patients With Heart Failure. Front. Cardiovasc. Med. 8:704462. doi: 10.3389/fcvm.2021.704462
Received: 03 May 2021; Accepted: 17 May 2021;
Published: 17 June 2021.
Edited by:
Xiongfei Pan, Vanderbilt University Medical Center, United StatesReviewed by:
Zuheng Liu, First Affiliated Hospital of Xiamen University, ChinaFedorov Alexandr, International University in Moscow, Russia
Copyright © 2021 Yang, Du, Liu, Zhang, Lin, Ouyang and Chen. This is an open-access article distributed under the terms of the Creative Commons Attribution License (CC BY). The use, distribution or reproduction in other forums is permitted, provided the original author(s) and the copyright owner(s) are credited and that the original publication in this journal is cited, in accordance with accepted academic practice. No use, distribution or reproduction is permitted which does not comply with these terms.
*Correspondence: Haixiong Chen, 13825553451@139.com
†These authors have contributed equally to this work