- Department of Cardiology, The Second People's Hospital of Foshan, Foshan, China
Background: The hemoglobin glycation index (HGI) has been proposed as a marker to quantify inter-individual variation in hemoglobin glycosylation. However, whether HGI is associated with an increased risk of diabetic complications independent of glycated hemoglobin (HbA1c) remains unclear. This meta-analysis aimed to determine the association between HGI and the risk of all cause mortality and composite cardiovascular disease (CVD).
Methods: PubMed, and EMBASE databases were searched for related studies up to March 31, 2021. Observational studies reported associations between HGI levels and composite CVD and all cause mortality were included for meta-analysis. A random effect model was used to calculate the hazard ratios (HRs) and 95% confidence intervals (CI) for higher HGI.
Results: A total of five studies, comprising 22,035 patients with type two diabetes mellitus were included for analysis. The median follow-up duration was 5.0 years. After adjusted for multiple conventional cardiovascular risk factors, an increased level of HGI was associated with a higher risk of composite CVD (per 1 SD increment: HR = 1.14, 95% CI = 1.04–1.26) and all cause mortality (per 1 SD increment: HR = 1.18, 95% CI = 1.05–1.32). However, when further adjusted for HbA1c, the association between HGI and risk of composite CVD (per 1 SD increment of HGI: HR = 1.01, 95% CI = 0.93–1.10) and all cause mortality (per 1 SD increment of HGI: HR = 1.03, 95% CI = 0.96–1.10) became insignificant.
Conclusions: High HGI was associated with an increased risk of composite CVD and all cause mortality after adjustment for multiple conventional cardiovascular risk factors. However, the association was mainly mediating by the level of HbA1c.
Introduction
Prevalence of type 2 diabetes mellitus (T2DM) has increased dramatically, with ~422 million adults diagnosed with T2DM worldwide (1). Cardiovascular disease (CVD) is a leading comorbidity of T2DM, contributing to the significantly increased mortality in patients with T2DM (2). Even in patients with prediabetes, the risk of all cause mortality and CVD was higher than those with normoglycemia (3, 4). Therefore, detection of biomarkers or metrics for risk stratification and guiding proper treatment in T2DM is of significant importance to relieve the global burden of T2DM (5).
Glycated hemoglobin (HbA1c) is an index that reflects the mean blood glucose (MBG) for the prior 2–3 months (6). HbA1c is considered the gold standard in evaluating of glycemic control in T2DM, broadly adopted in clinical practice and trials (7, 8). However, studies have shown considerable variation in the association between measured HbA1c and MBG. Only 60–80% of the variance in HbA1c levels could be explained by the MBG levels, while the rest is attributed to ethnic and biological factors involved in hemoglobin glycation (9, 10). To quantify such variation, the hemoglobin glycation index (HGI) was introduced by Hempe et al., which is defined as the measured HbA1c minus predicted HbA1c levels (11). The predicted HbA1c levels are calculated with equations from linear regression of HbA1c and fasting blood glucose. Therefore, individuals with high HGI values have higher measured HbA1c levels than predicted HbA1 levels from their blood glucose levels.
There has been contentious about adopting the HGI as a marker of risk stratification in patients with diabetes. It had been proposed that high HGI is associated with vascular complications in patients with and without diabetes (12–15). In a large sample cross-sectional study, high HGI was independently related to composite CVD, coronary artery disease, stroke, and peripheral artery disease after adjustment for other CVD risk factors, including HbA1c levels (16). However, the cross-sectional design limits its value to explore the causal relationship between HGI and the risk of CVD. Some prospective studies showed increased HGI was associated with an increased risk for CVD (17, 18), other studies have not found a similar association (19–21), especially after adjusting HbA1c levels. Based on these inconsistencies, we performed a meta-analysis of prospective studies to evaluate the associations between HGI and the risk of all cause mortality and CVD.
Methods
Search Strategy and Selection Criteria
According to the recommendations of the Meta-analysis of Observational Studies in Epidemiology group (22), we searched electronic databases, including PubMed, and EMBASE for studies up to March 31, 2021, using a combined MeSH heading and text search strategy with the following terms: “hemoglobin glycation index,” or “HGI,” and “cardiovascular disease,” “cardiovascular event,” “cardiocerebrovascular disease,” “coronary artery disease,” “coronary heart disease,” “ischemic heart disease,” “myocardial infarction,” “stroke,” “cerebral infarction,” “mortality,” or “death.” We also reviewed reference lists of included studies to identify other potential publications. The search was restricted to human studies, while without language restriction.
We included studies for meta-analysis if they met the following criteria: (1) cohort study, nest case-control study or post-hoc analysis of randomized controlled trials (RCTs), evaluating the associations between HGI and risk of CVD or all cause mortality; (2) the risk of endpoints were reported in high HGI compared with low HGI patients (e.g., highest tertile vs. lowest tertile), or evaluated when HGI was defined as a continuous metric; (3) multivariable-adjusted relative risks (RRs), or hazard ratios (HRs) and 95% confidence intervals (CIs) were reported; (3) the follow up duration ≥1 years. We excluded studies if: (1) they were cross-sectional studies without follow-up evaluation; (2) the association between HGI and risk of endpoints was not adjusted for other cardiovascular risk factors, and (3) identical outcomes were derived from the same population.
Data Extraction and Quality Assessment
Two reviewers (JW and DL) screened the titles and abstracts of the retrieved reports, and reviewed the full copies of potentially relevant studies. Study information such as study design, ethnicity, participant's characteristics, sample size and proportion of female, follow-up duration, adjusted confounders, and endpoints assessment were recorded. Quality assessment of the included studies was conducted based on the Newcastle–Ottawa Quality Assessment Scale for cohort studies (23), in which an investigation was judged based on: selection (four items, one star each), comparability (one item, up to two stars), and exposure/outcome (three items, one star each), respectively. In this meta-analysis, we defined included studies as with good, fair, or poor quality if they were awarded ≥7, 4–6, or < 4 stars, respectively (24, 25).
Data Synthesis and Analysis
The primary outcomes were the risk of composite cardiovascular events and all-cause mortality associated with HGI. The associations between the HGI and risk of outcomes were reported in different ways in the included studies, such as per standard-deviation (SD) increment in the continuous trait; or per tertiles or quartiles in HGI levels. To enable a consistent approach to the meta-analysis, we calculated the HRs per 1 SD increment in the level of HGI. If the included studies did not report effect measures as per 1 SD change, we converted the results according to the previously reported methods (26, 27).
Adjusted HRs and 95% CI were extracted for the meta-analysis. If a study reported multiple results based on different statistical adjusted models, results adjusted for the most number of variables were extracted for analysis. If RRs, but not HRs were reported, the RRs were considered as approximate HRs and included for meta-analysis (28). To explore whether the endpoints associated with HGI were mediated by the level of HbA1c, studies with both estimates of HRs before and after adjustment of HbA1c were also extracted for meta-analysis, respectively. I2 statistics were used to test heterogeneity among studies, and an I2 > 50% was considered to be with significant heterogeneity. We used the inverse variance approach with a random-effects model to combine the log HRs and corresponding standard errors (SEs). We also performed a sensitivity analysis by omitting one study and recalculating the estimates of HRs at a time. Publication bias was evaluated by inspecting funnel plots for the primary outcomes in which the natural log HR was plotted against SEs, and further tested using Egger's and Begg's tests. Subgroup analyses were performed according to participant's age, follow-up duration, and baseline CVD.
Analyses were performed using Stata 12.0 (StataCorp LP, College Station, TX, USA) and RevMan 5.3 (The Cochrane Collaboration, Copenhagen, Denmark). A P < 0.05 was considered as with statistical significance.
Results
Studies Retrieved and Characteristics
A total of 121 manuscripts were retrieved from the electronic databases. After screening of the titles and abstracts, 12 qualified for a full review, and five studies were included for meta-analysis finally (Figure 1) (17–21). There were 22,035 patients involved in the meta-analysis, with a median follow-up duration of 5 years.
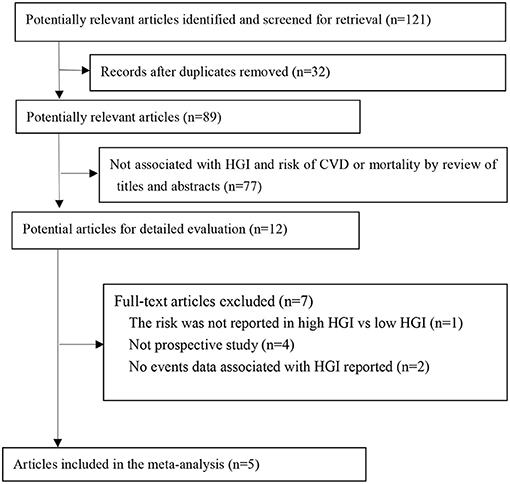
Figure 1. Flow of papers through review. CVD, cardiovascular disease; HGI, hemoglobin glycation index.
The key characteristics of the included studies are presented in Table 1. Two studies were from Asia, one from the Netherlands, and two post-hoc analyses of RCTs enrolled patients from multiple countries worldwide. All the studies included patients with T2DM, while differed in with/without CVD. According to quality assessment criteria, 4 studies were graded as good quality, and only one as fair (Online Supplementary File 1).
Association Between HGI and Prognosis in Patients With T2DM Before Adjusted for Hba1c
All the included five studies provided a multi-variable adjusted risk of composite CVD associated with HGI before adjusted for HbA1c. Significant heterogeneity among all studies was observed (I2 = 72%, P = 0.007). Random-effects models analyses showed that per 1 SD increment of HGI was associated with a 14% higher risk of composite CVD after adjusted for multiple cardiovascular risk factors (HR = 1.14, 95% CI = 1.04–1.26, P = 0.007) (Figure 2). We did not find any evidence of publication bias by visual inspection of the funnel plot (Online Supplementary File 2), or using Egger's test or Begg's (all P > 0.05).
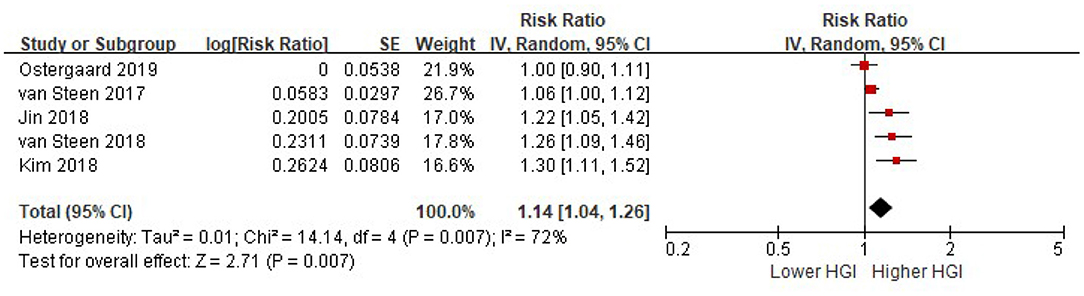
Figure 2. The association between HGI and the risk of composite CVD. Data were presented as the HR and 95% CI of composite CVD for per 1 SD increment of HGI, after adjusting for multiple cardiovascular risk factors (without HbA1c). CI, confidence interval; CVD, cardiovascular disease; HGI, hemoglobin glycation index.
Three studies provided a multi-variable adjusted risk of all cause mortality associated with HGI before adjusted for HbA1c. Random-effects models analyses showed that per 1 SD increment of HGI was associated with an 18% higher risk of all cause mortality after adjusted for multiple cardiovascular risk factors, while without adjusted for HbA1c (HR = 1.18, 95% CI = 1.05–1.32, P = 0.007) (Figure 3).
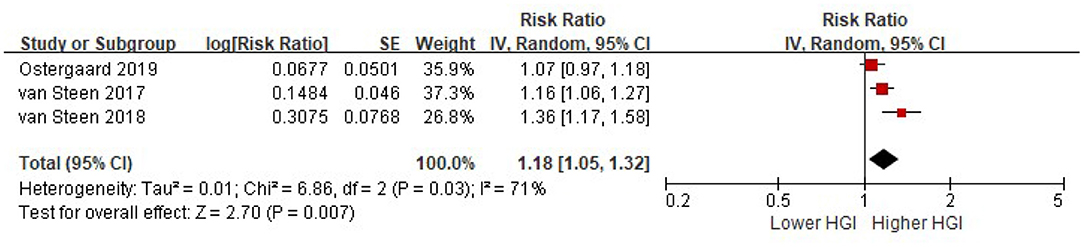
Figure 3. The association between HGI and the risk of all cause mortality. Data were presented as the HR and 95% CI of all cause mortality for per 1 SD increment of HGI, after adjusting for multiple cardiovascular risk factors (without HbA1c). CI, confidence interval; HGI, hemoglobin glycation index.
Association Between HGI and Risk of All-Cause Mortality and CVD After Adjusted for HbA1c
Four studies reported the risk of composite CVD associated with HGI after adjusted for multiple cardiovascular risk factors plus HbA1c. There was significant heterogeneity among studies (I2 = 51%, P = 0.10). Random-effects models analyses showed that when adjusted for multiple cardiovascular risk factors and HbA1c, the association between HGI and risk of composite CVD became insignificant (per 1 SD increment of HGI: HR = 1.01, 95% CI = 0.93–1.10, P = 0. 79) (Figure 4). Similarly, there was no association between HGI and risk of all cause mortality after adjustment with HbA1c (per 1 SD increment of HGI: HR = 1.03, 95% CI = 0.96–1.10, P = 0.37) (Figure 5).
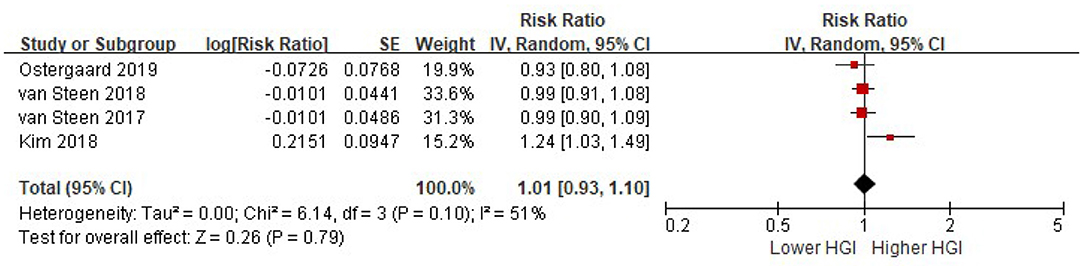
Figure 4. The association between HGI and the risk of composite CVD further adjusting for HbA1c. Data were presented as the HR and 95% CI of composite CVD for per 1 SD increment of HGI, after adjusting for multiple cardiovascular risk factors and plus HbA1c. CI, confidence interval; CVD, cardiovascular disease; HGI, hemoglobin glycation index.
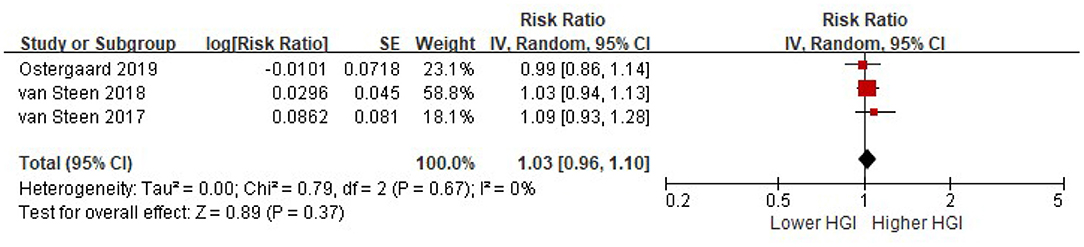
Figure 5. The association between HGI and the risk of all cause mortality further adjusting for HbA1c. Data were presented as the HR and 95% CI of composite CVD for per 1 SD increment of HGI, after adjusting for multiple cardiovascular risk factors and plus HbA1c. CI, confidence interval; HGI, hemoglobin glycation index.
Sensitivity Analyses and Subgroup Analyses
We performed several sensitivity analyses and confirmed that the results were not influenced by the use of fixed-effects models compared with random-effects models, or recalculating the HRs by omitting one study at a time.
The results of subgroup analyses for the risk of composite CVD and HGI were presented in Table 2. A high level of HGI was associated with an increased risk of composite CVD in patients without baseline CVD (HR = 1.22, 95% CI = 1.04–1.43), even after adjustment for HbA1c. However, there was no significant association observed between HGI and composite CVD in patients with a history of CVD (HR = 0.96, 95% CI = 0.88–1.06) (P for subgroup heterogeneity = 0.01, I2 = 84.5%). There were no significant associations for HGI and composite CVD risk among all other subgroup analyses.
Discussion
In this study, we included five observational studies, including more than 22,000 T2DM patients with a median follow-up duration of 5 years for meta-analysis. We found that high HGI was associated with an increased risk of composite CVD, and all cause mortality after adjustment of multiple cardiovascular risk factors. However, when further adjusted for levels of HbA1c, the association between HGI and poor prognosis was disappeared.
Although still with controversies, several mechanisms had been proposed to explain the association between HGI and the risk of CVD. First, patients with high HGI had a longer duration of diabetes and combined cardiovascular risk factors (15). Second, high HGI had been reported to be associated with subclinical target organ damage, such as coronary artery calcification (12), and carotid atherosclerosis (14). Third, high HGI is associated with activated inflammation status, which played an important role in the development of CVD (29). However, our results raised concern on these proposed mechanisms, because after adjusting for HbA1c, the association between HGI and poor prognosis was disappeared. These results indicated that the impact of HGI on CVD and all cause mortality was mainly mediating by the level of HbA1c. These finding were supported by data from the Diabetes Control and Complications Trial (DCCT), which showed that among type 1 diabetic patients, HGI was not an independent risk factor for microvascular complications, and that the effect of the HGI on risk of microvascular complications was wholly explained by the associated level of HbA1c (30).
Recently, Zhang et al. had reported a meta-analysis, which showed that for each 1-unit increment in HGI, there was a 20 and 13% increase of composite CVD and all cause mortality, respectively (31). Our analysis had several different findings compared with Zhang's report. First, we calculated the risk estimate before and after adjustingd for HbA1c, respectively, but not lumped them together, which was useful for interpreting the mediating effect of HbA1c on the association between HGI and poor prognosis. Second, we set up more strict inclusion criteria to avoid incorrect inclusion. For example, we did not include a study that reported a U-shaped association between HGI and the prognosis in patients with stroke, in which the data cannot be transformed into a continuous increment of HGI (32). We also did not include the study by Hempe et al., in which the association between HGI and risk of composite CVD or all cause mortality was not reported (33). Third, we also find that a high level of HGI was associated with an increased risk of composite CVD in patients without baseline CVD, even after adjustment for HbA1c. However, there was no significant association observed between HGI and composite CVD in patients with a history of CVD. This interesting finding raised a hypothesis that in T2DM without a history of CVD, detection of HGI may provide further information for risk stratification. However, due to the limited number of studies available, further studies are urgently needed.
Our study has several limitations. First, this is a meta-analysis based on the study level. We did not have individual participant data. Therefore, residual confounders could not be avoided. However, all the included studies had adjusted for multiple conventional cardiovascular risk factors. Second, the calculation of HGI differed among the included studies, which would cause significant heterogeneity among the studies. Third, it had been proposed that the variance in HbA1c was different in Asians and Westerns (34). However, due to the limited studies available, we did not perform subgroups analysis according to race. Further studies are needed to explore whether the associations between HGI and poor prognosis are different among different ethnicities.
Conclusions
Our results showed that although high HGI was associated with an increased risk of composite CVD and all cause mortality after adjustment for multiple conventional cardiovascular risk factors. However, the association was mainly mediating by the level of HbA1c. Considering that HbA1c can be easily evaluated in most laboratories worldwide, and the calculation of the HGI is complicated, clinical use of HGI in T2DM cannot be routinely recommended.
Data Availability Statement
The raw data supporting the conclusions of this article will be made available by the authors, without undue reservation.
Author Contributions
J-dW, D-lL, YX, M-yC, and H-hC: research idea and study design. J-dW, D-lL, DS, and H-qH: data acquisition. J-dW and D-lL: data analysis and interpretation. J-dW and YX: statistical analysis. J-dW: supervision and mentorship. All authors contributed important intellectual content during manuscript drafting or revision and accept accountability for the overall work by ensuring that questions pertaining to the accuracy or integrity of any portion of the work are appropriately investigated and resolved.
Funding
J-dW was supported by the Guangdong Basic and Applied Basic Research Fund (Key project of Guangdong-Foshan Joint Fund) (2019B1515120044). The funders had no role in study design, data collection, data analysis, data interpretation, or writing of the report.
Conflict of Interest
The authors declare that the research was conducted in the absence of any commercial or financial relationships that could be construed as a potential conflict of interest.
Supplementary Material
The Supplementary Material for this article can be found online at: https://www.frontiersin.org/articles/10.3389/fcvm.2021.690689/full#supplementary-material
References
1. Shirai Y, Imai T, Sezaki A, Miyamoto K, Kawase F, Abe C, et al. Trends in age-standardised prevalence of type 2 diabetes mellitus according to country from 1990 to 2017 and their association with socioeconomic, lifestyle and health indicators: an ecological study. J Glob Health. (2021) 11:4005. doi: 10.7189/jogh.11.04005
2. Pearson-Stuttard J, Bennett J, Cheng YJ, Vamos EP, Cross AJ, Ezzati M, et al. Trends in predominant causes of death in individuals with and without diabetes in England from 2001 to 2018: an epidemiological analysis of linked primary care records. Lancet Diabetes Endocrinol. (2021) 9:165–73. doi: 10.1016/S2213-8587(20)30431-9
3. Huang Y, Cai X, Mai W, Li M, Hu Y. Association between prediabetes and risk of cardiovascular disease and all cause mortality: systematic review and meta-analysis. BMJ. (2016) 355:i5953. doi: 10.1136/bmj.i5953
4. Cai X, Liu X, Sun L, He Y, Zheng S, Zhang Y, et al. Prediabetes and risk of heart failure: a meta-analysis. Diabetes Obes Metab. (2021). doi: 10.1111/dom.14388. [Epub ahead of print].
5. Wu J, Zheng H, Liu X, Chen P, Zhang Y, Luo J, et al. Prognostic value of secreted frizzled-related protein 5 in heart failure patients with and without type 2 diabetes mellitus. Circ Heart Fail. (2020) 13:e7054. doi: 10.1161/CIRCHEARTFAILURE.120.007054
6. Cavagnolli G, Pimentel AL, Freitas PA, Gross JL, Camargo JL. Factors affecting A1C in non-diabetic individuals: review and meta-analysis. Clin Chim Acta. (2015) 445:107–14. doi: 10.1016/j.cca.2015.03.024
7. Cai X, Zhang Y, Li M, Wu JH, Mai L, Li J, et al. Association between prediabetes and risk of all cause mortality and cardiovascular disease: updated meta-analysis. BMJ. (2020) 370:m2297. doi: 10.1136/bmj.m2297
8. Langholz PL, Wilsgaard T, Njolstad I, Jorde R, Hopstock LA. Trends in known and undiagnosed diabetes, HbA1c levels, cardiometabolic risk factors and diabetes treatment target achievement in repeated cross-sectional surveys: the population-based Tromso Study 1994-2016. Bmj Open. (2021) 11:e41846. doi: 10.1136/bmjopen-2020-041846
9. Nagarathna R, Kaur N, Anand A, Sharma K, Dada R, Sridhar P, et al. Distribution of glycated haemoglobin and its determinants in Indian young adults. Diabetes Res Clin Pract. (2020) 159:107982. doi: 10.1016/j.diabres.2019.107982
10. Seo JY, Hwang SS, Kim JH, Lee YA, Lee SY, Shin CH, et al. Distribution of glycated haemoglobin and its determinants in Korean youth and young adults: a nationwide population-based study. Sci Rep. (2018) 8:1962. doi: 10.1038/s41598-018-20274-8
11. Hempe JM, Gomez R, McCarter RJ, Chalew SA. High and low hemoglobin glycation phenotypes in type 1 diabetes: a challenge for interpretation of glycemic control. J Diabetes Complications. (2002) 16:313–320. doi: 10.1016/S1056-8727(01)00227-6
12. Rhee EJ, Cho JH, Kwon H, Park SE, Park CY, Oh KW, et al. Association between coronary artery calcification and the hemoglobin glycation index: the kangbuk samsung health study. J Clin Endocrinol Metab. (2017) 102:4634–41. doi: 10.1210/jc.2017-01723
13. Cheng PC, Hsu SR, Cheng YC, Liu YH. Relationship between hemoglobin glycation index and extent of coronary heart disease in individuals with type 2 diabetes mellitus: a cross-sectional study. Peerj. (2017) 5:e3875. doi: 10.7717/peerj.3875
14. Marini MA, Fiorentino TV, Succurro E, Pedace E, Andreozzi F, Sciacqua A, et al. Association between hemoglobin glycation index with insulin resistance and carotid atherosclerosis in non-diabetic individuals. PLoS ONE. (2017) 12:e175547. doi: 10.1371/journal.pone.0175547
15. Lee B, Heo YJ, Lee YA, Lee J, Kim JH, Lee SY, et al. Association between hemoglobin glycation index and cardiometabolic risk factors in Korean pediatric nondiabetic population. Ann Pediatr Endocrinol Metab. (2018) 23:196–203. doi: 10.6065/apem.2018.23.4.196
16. Ahn CH, Min SH, Lee DH, Oh TJ, Kim KM, Moon JH, et al. Hemoglobin glycation index is associated with cardiovascular diseases in people with impaired glucose metabolism. J Clin Endocrinol Metab. (2017) 102:2905–13. doi: 10.1210/jc.2017-00191
17. Jin JL, Sun D, Cao YX, Guo YL, Wu NQ, Zhu CG, et al. Triglyceride glucose and haemoglobin glycation index for predicting outcomes in diabetes patients with new-onset, stable coronary artery disease: a nested case-control study. Ann Med. (2018) 50:576–86. doi: 10.1080/07853890.2018.1523549
18. Kim MK, Jeong JS, Yun JS, Kwon HS, Baek KH, Song KH, et al. Hemoglobin glycation index predicts cardiovascular disease in people with type 2 diabetes mellitus: A 10-year longitudinal cohort study. J Diabetes Complications. (2018) 32:906–10. doi: 10.1016/j.jdiacomp.2018.08.007
19. Ostergaard HB, Mandrup-Poulsen T, Berkelmans G, van der Graaf Y, Visseren F, Westerink J. Limited benefit of haemoglobin glycation index as risk factor for cardiovascular disease in type 2 diabetes patients. Diabetes Metab. (2019) 45:254–60. doi: 10.1016/j.diabet.2018.04.006
20. van Steen SC, Woodward M, Chalmers J, Li Q, Marre M, Cooper ME, et al. Haemoglobin glycation index and risk for diabetes-related complications in the Action in Diabetes and Vascular Disease: Preterax and Diamicron Modified Release Controlled Evaluation (ADVANCE) trial. Diabetologia. (2018) 61:780–9. doi: 10.1007/s00125-017-4539-1
21. van Steen SC, Schrieks IC, Hoekstra JB, Lincoff AM, Tardif JC, Mellbin LG, et al. The haemoglobin glycation index as predictor of diabetes-related complications in the AleCardio trial. Eur J Prev Cardiol. (2017) 24:858–66. doi: 10.1177/2047487317692664
22. Stroup DF, Berlin JA, Morton SC, Olkin I, Williamson GD, Rennie D, et al. Meta-analysis of observational studies in epidemiology: a proposal for reporting. Meta-analysis Of Observational Studies in Epidemiology (MOOSE) group. JAMA. (2000) 283:2008–12. doi: 10.1001/jama.283.15.2008
23. GA Wells, B Shea, D O'Connell, J Peterson, V Welch, M Losos, et al. The Newcastle-Ottawa Scale (NOS) for Assessing the Quality of Nonrandomised Studies in Meta-Analyses. (2008) Available online at: http://www.ohri.ca/programs/clinical_epidemiology/oxford.asp (accessed January 1, 2008).
24. Huang Y, Huang W, Mai W, Cai X, An D, Liu Z, et al. White-coat hypertension is a risk factor for cardiovascular diseases and total mortality. J Hypertens. (2017) 35:677–88. doi: 10.1097/HJH.0000000000001226
25. Cai X, Zheng S, Liu Y, Zhang Y, Lu J, Huang Y. Nonalcoholic fatty liver disease is associated with increased risk of atrial fibrillation. Liver Int. (2020) 40:1594–600. doi: 10.1111/liv.14461
26. Chowdhury R, Stevens S, Gorman D, Pan A, Warnakula S, Chowdhury S, et al. Association between fish consumption, long chain omega 3 fatty acids, and risk of cerebrovascular disease: systematic review and meta-analysis. BMJ. (2012) 345:e6698. doi: 10.1136/bmj.e6698
27. Li W, Huang A, Zhu H, Liu X, Huang X, Huang Y, et al. Gut microbiota-derived trimethylamine N-oxide is associated with poor prognosis in patients with heart failure. Med J Aust. (2020) 213:374–9. doi: 10.5694/mja2.50781
28. Yang Y, Li W, Zhu H, Pan XF, Hu Y, Arnott C, et al. Prognosis of unrecognised myocardial infarction determined by electrocardiography or cardiac magnetic resonance imaging: systematic review and meta-analysis. BMJ. (2020) 369:m1184. doi: 10.1136/bmj.m1184
29. Liu S, Hempe JM, McCarter RJ, Li S, Fonseca VA. Association between inflammation and biological variation in hemoglobin A1c in U.S. Nondiabetic Adults. J Clin Endocrinol Metab. (2015) 100:2364–71. doi: 10.1210/jc.2014-4454
30. Lachin JM, Genuth S, Nathan DM, Rutledge BN. The hemoglobin glycation index is not an independent predictor of the risk of microvascular complications in the Diabetes Control and Complications Trial. Diabetes. (2007) 56:1913–21. doi: 10.2337/db07-0028
31. Zhang L, Wang M, Zhang R, Zhong Y, Fan H, Wang M, et al. Hemoglobin glycation index in relationship to the risk of cardiovascular complication in patients with type 2 diabetes: a systematic review and meta-analysis. J Diabetes Complications. (2020) 34:107673. doi: 10.1016/j.jdiacomp.2020.107673
32. Pan Y, Jing J, Wang Y, Liu L, Wang Y, He Y. Association of hemoglobin glycation index with outcomes of acute ischemic stroke in type 2 diabetic patients. Neurol Res. (2018) 40:573–80. doi: 10.1080/01616412.2018.1453991
33. Hempe JM, Liu S, Myers L, McCarter RJ, Buse JB, Fonseca V. The hemoglobin glycation index identifies subpopulations with harms or benefits from intensive treatment in the ACCORD trial. Diabetes Care. (2015) 38:1067–74. doi: 10.2337/dc14-1844
34. Sarnowski C, Leong A, Raffield LM, Wu P, de Vries PS, DiCorpo D, et al. Impact of rare and common genetic variants on diabetes diagnosis by hemoglobin a1c in multi-ancestry cohorts: the trans-omics for precision medicine program. Am J Hum Genet. (2019) 105:706–18. doi: 10.1016/j.ajhg.2019.08.010
Keywords: hemoglobin glycation index, glycated hemoglobin, all-cause mortality, cardiovascular disease, risk
Citation: Wu J-d, Liang D-l, Xie Y, Chen M-y, Chen H-h, Sun D and Hu H-q (2021) Association Between Hemoglobin Glycation Index and Risk of Cardiovascular Disease and All Cause Mortality in Type 2 Diabetic Patients: A Meta-Analysis. Front. Cardiovasc. Med. 8:690689. doi: 10.3389/fcvm.2021.690689
Received: 03 April 2021; Accepted: 05 May 2021;
Published: 28 May 2021.
Edited by:
Yuli Huang, Southern Medical University, ChinaReviewed by:
Fedorov Alexandr, International University in Moscow, RussiaCopyright © 2021 Wu, Liang, Xie, Chen, Chen, Sun and Hu. This is an open-access article distributed under the terms of the Creative Commons Attribution License (CC BY). The use, distribution or reproduction in other forums is permitted, provided the original author(s) and the copyright owner(s) are credited and that the original publication in this journal is cited, in accordance with accepted academic practice. No use, distribution or reproduction is permitted which does not comply with these terms.
*Correspondence: Jian-di Wu, fsdrwu@126.com
†These authors have contributed equally to this work