- 1Center for Cardiovascular Research and Heart Center, Nationwide Children's Hospital, Columbus, OH, United States
- 2Department of Pediatrics, Ohio State University, Columbus, OH, United States
- 3The Institute for Genomic Medicine, Nationwide Children's Hospital, Columbus, OH, United States
- 4Department of Molecular Genetics, Ohio State University, Columbus, OH, United States
Congenital heart disease (CHD) is the most common type of birth defect, affecting ~1% of all live births. Malformations of the cardiac outflow tract (OFT) account for ~30% of all CHD and include a range of CHDs from bicuspid aortic valve (BAV) to tetralogy of Fallot (TOF). We hypothesized that transcriptomic profiling of a mouse model of CHD would highlight disease-contributing genes implicated in congenital cardiac malformations in humans. To test this hypothesis, we utilized global transcriptional profiling differences from a mouse model of OFT malformations to prioritize damaging, de novo variants identified from exome sequencing datasets from published cohorts of CHD patients. Notch1+/−; Nos3−/− mice display a spectrum of cardiac OFT malformations ranging from BAV, semilunar valve (SLV) stenosis to TOF. Global transcriptional profiling of the E13.5 Notch1+/−; Nos3−/− mutant mouse OFTs and wildtype controls was performed by RNA sequencing (RNA-Seq). Analysis of the RNA-Seq dataset demonstrated genes belonging to the Hif1α, Tgf-β, Hippo, and Wnt signaling pathways were differentially expressed in the mutant OFT. Mouse to human comparative analysis was then performed to determine if patients with TOF and SLV stenosis display an increased burden of damaging, genetic variants in gene homologs that were dysregulated in Notch1+/−; Nos3−/− OFT. We found an enrichment of de novo variants in the TOF population among the 1,352 significantly differentially expressed genes in Notch1+/−; Nos3−/− mouse OFT but not the SLV population. This association was not significant when comparing only highly expressed genes in the murine OFT to de novo variants in the TOF population. These results suggest that transcriptomic datasets generated from the appropriate temporal, anatomic and cellular tissues from murine models of CHD may provide a novel approach for the prioritization of disease-contributing genes in patients with CHD.
Introduction
As the most common type of birth defect, congenital heart disease (CHD) affects nearly ~1% of all live births (1). Malformations of the cardiac outflow tract (OFT), which include incorrect positioning or septation of the major vessels (aorta and pulmonary artery) as well as anomalies of the aortic or pulmonic (semilunar) valves, account for an estimated 30% of CHD cases (1). These types of malformations range from the simple to the more complex such as bicuspid aortic valve (BAV) and tetralogy of Fallot, respectively. Bicuspid aortic valve (BAV), where the normal trileaflet structure is disrupted and two valve leaflets are instead observed, is the most common type of CHD, with an estimated population prevalence between 1–2% (2). BAV is frequently undiagnosed during infancy since it often does not impact cardiac function at an early age, however afflicted patients are at an increased risk of calcific aortic valve disease (CAVD) and resultant stenosis as adults (3, 4). Tetralogy of Fallot (TOF), is one of the more complex forms of CHD affecting the OFT in which abnormal positioning of the aorticopulmonary septum leads to pulmonic valve stenosis and a ventricular septal defect. TOF requires surgical intervention during infancy, lifelong medical monitoring, and often pulmonic valve replacement as an adult. Other less common cardiac OFT malformations, referred to as conotruncal heart defects, include truncus arteriosus, transposition of the great arteries and double outlet right ventricle. In total, conotruncal heart defects compose a significant and growing portion of adult CHD survivors, but the genetic contributors for the majority of cases have not been defined.
Conotruncal CHD can be traced back to the improper development of the transient yet critical common cardiac OFT (5). The common OFT contributes to the development of the great vessels and the semilunar valves following multiple morphological changes to this initial common structure. In mice, the common OFT is visually distinguishable by embryonic day (E) 9.5 as it is one of four major anatomical components in addition to the common atrium, atrioventricular canal, and common ventricle. At this stage, the OFT is best described as a cylinder of cells of anterior second heart field (SHF) origin that is lined with endothelial cells (6–10). This structure also receives important contributions from migrating cardiac neural crest cells (CNC) (11, 12). Septation of the OFT into the aorta and pulmonary artery is achieved by the migrating population of CNC towardz the SHF and muscularization of this tissue (13, 14). Endocardial cushions, which are the precursors of semilunar valves, form in the outflow region of the primitive heart tube (15). These primitive valve structures are composed of a layer of extracellular matrix that is interposed between the endothelial cells and the surrounding myocardium (15–17). Endothelial cells undergo endothelial to mesenchymal transition (EMT) and populate the endocardial cushions with newly transformed mesenchymal cells (18). This is followed by semilunar valve development and remodeling. Considering that the development of OFT-derived structures is dependent on the migration and differentiation of multiple cell lineages, it is unsurprising that the etiologies of OFT malformations are numerous and complex.
Previous human genetics analyses and mouse gene knockout studies have identified multiple genetic contributors to OFT development and disease. Among these, mutations in NOTCH1 were among the first implicated to contribute to semilunar valve and OFT malformations following linkage analysis of two kindreds affected with aortic valve disease consisting of BAV, aortic valve stenosis, CAVD along with one individual with TOF (19). A large cohort of patients with a left-ventricular outflow tract malformations were also screened for NOTCH1 mutations and were found to harbor a significant burden of inherited missense variants (20). Furthermore, a study of 428 probands with familial left-sided CHD demonstrated that those families having members with conotruncal heart disease often had pathogenic variants in NOTCH1 demonstrating a spectrum of phenotypes associated with NOTCH1 genetic variation (21). A genome-wide chromosomal analysis of TOF patients identified de novo copy number variations in loci known to encode NOTCH1 and JAG1, a ligand for the NOTCH1 receptor, that were absent in controls further implicating genes in the Notch signaling pathway as potential contributors of disease (22). Exome sequencing methods in TOF patient populations identified disease-contributing variants in NOTCH1 and also allowed for the characterization of other genes such as FLT4, which encodes for VEGFR-3 (23–25). Meanwhile, an inspection of cardiac phenotypes in transgenic mouse models deficient in Fgf, Bmp, Slit/Robo, N-Cadherin, and Wnt signaling have also identified malformations of OFT structures with varying degrees of penetrance (6, 26–36).
We have previously published that Notch1 haploinsufficient mice backcrossed into a Nos3-null background are a highly penetrant model of cardiac OFT malformations and semilunar valve disease (37, 38). At E18.5, these mice display a spectrum of phenotypes including thickened, malformed semilunar valves, BAV, and additional anomalies of the OFT including overriding aorta and ventricular septal defect, which are reminiscent of TOF. These cardiac phenotypes were observed in late gestation Notch1+/−; Nos3−/− embryos suggesting that they were the result of abnormal development at earlier timepoints. Deletion of Notch1 in the endothelial cell and the SHF lineages in this mouse model recapitulated the observed semilunar valve and OFT malformations indicating the importance of Notch1 in these cells and their derivatives.
Exome and genome sequencing approaches have greatly enhanced the ability to rapidly identify genetic variants in patients with CHD, but the prioritization of the rapidly growing number of variants in regard to pathogenicity has proven to be difficult. Here, we have utilized the gene expression profiling differences identified in a murine model of cardiac OFT malformations to prioritize and strengthen the genetic link between novel gene candidates identified in patients with conotruncal heart disease. First, we performed transcriptomic analysis of dissected OFTs obtained from E13.5 Notch1+/−; Nos3−/− embryonic hearts and identified genes with differential expression patterns when compared to wild-type controls. Single-cell RNA-Sequencing (scRNA-Seq) data generated from wildtype E12.5 cardiac OFTs was used to predict the cell-type specificity of dysregulated genes and genes expressed in non-contributing cell types were excluded. We cross-compared those genetic homologs dysregulated in the Notch1+/−; Nos3−/− mouse OFT to genetic variants identified in published patient cohorts with tetralogy of Fallot (TOF) and semilunar valve (SLV) disease. We identified a significant overlap between genes differentially expressed in the OFT of the Notch1+/−; Nos3−/− mouse model and genes with de novo variants in TOF but not SLV patients. Notably, no significant overlap was found when comparing the highest expressing genes in the mouse OFT to the de novo variant gene list from TOF patients. Together, this analysis pipeline provides an additional methodology to prioritize disease-causing genetic variants that are likely pathogenic contributors to CHD.
Materials and Methods
Experimental Mouse Models
Notch1 (Notch1+/−) and endothelial nitric oxide synthase 3 (Nos3−/−) knockout strains were generated as previously described and are publicly available at the Jackson Laboratory (#002797, and #002684) (39, 40). These mice were housed as live heterozygote colonies to ensure line maintenance and kept in a C57/BL6J background. All mice were maintained on a 12-h-light/dark cycle and fed a standard western diet. For timed breeding (Notch1+/−; Nos3+/− × Nos3+/−; Notch1+/− × wildtype), noon of the day of vaginal plug was observed is defined as embryonic day E0.5. Pregnant dams were anesthetized using inhalation of 3% isoflurane. Cervical dislocation and organ removal were used as a secondary method of euthanasia. Embryos were collected at E12.5 or E13.5 and wildtype littermates were used as controls. All animal experiments were approved by the Institutional Animal Care and Use Committee at the Research Institute at Nationwide Children's Hospital.
Isolation of RNA From the E13.5 Embryonic Cardiac Outflow Tract and Bulk RNA-Sequencing
E13.5 embryos were collected in an RNase-free environment. A blunt cut at the base of the OFT, below the conal cushions, was used to collect the OFT tissues from each embryo. The OFT were snap frozen until genotyping had been completed using tissue from the remaining embryo. Following genotyping of the embryos, three OFTs were pooled in 1 ml Trizol to form one biological replicate. Three biological replicates of the mutant embryos and three biological replicates of the wild type embryos were used for the final RNA-Seq analysis (i.e., 3 OFTs per sample submitted, 18 total OFTs). Total RNA was collected following tissue homogenization using TissueLyser II (Qiagen) and chloroform-isopropanol extraction and purification and isolated using the Total RNA Purification kit (Norgen Biotek Corp, 17200). RNA-Seq was performed at Ocean Ridge Biosciences as previously described (41). RNA TruSeq Stranded Total RNA LT with Ribo-Zero Gold Set A kit (Illumina, RS-122-2301) was used to generate libraries. Libraries were sequenced using Illumina HiSeq 2500 to generate paired-end 50 bp reads.
Bioinformatics Analysis of Bulk RNA-Sequencing
The raw FASTQ files were split into files containing 4,000,000 reads and checked for quality using the FASTX-Toolkit1 (version 0.0.14, http://hannonlab.cshl.edu/fastx_toolkit/). The reads were filtered (removing sequences that did not pass Illumina's quality filter) and trimmed based on the quality results (3 nucleotides at the left end of the R1 reads). Then, sequence alignment was performed using TopHat (v2.1.0) to mouse genome version mm10 (ftp://ftp.ccb.jhu.edu/pub/data/bowtie2_indexes/mm10) (42). Following the alignment to the mouse genome, BAM files were merged on a per-sample basis. Generation of BAM files was performed by Ocean Ridge Biosciences. Aligned BAM files are used to count the number of reads mapping to exons in each transcript using the GenomicAlignments (version 1.22.1) package in R to generate a gene-count matrix (43). Differential expression was evaluated from this gene-count matrix using the DESeq2 package (version 1.26.0) using the standard differential expression analysis pipeline (without log fold change shrinkage method) in R (44). Genes with ≤5 reads across all samples were excluded from the analysis. Genes that were differentially expressed were filtered using the cutoff: adjusted P-value from DESeq2 result <= 0.05. Genes passing this filtering cutoff were then used as input for a KEGG pathway enrichment analysis using DAVID V6.8 online tool (45, 46). Identified pathways were then classified as signaling pathways, metabolic pathways, and cardiovascular disease-related genes. A chord plot showing gene-pathway relationships in each of these classes was created using a modified GoChord function from the GOplot package in R (47).
Single-Cell Preparation and Sequencing
E12.5 cardiac OFTs (both Notch1+/− and wildtype) were microdissected from five mouse embryos and pooled for single-cell RNA sequencing. To prepare single cells suspensions, pooled OFT tissues were incubated with 1 mg/ml Collagenase II (Worthington Biochemical Corporation# LS004176) for 15 min at 37°C with occasional stirring at every 5 min for complete dissociation. The digestion reaction was quenched immediately with 1 ml. DMEM supplemented with 10% FBS and pelleted down at 1,000 rpm for 5 min at 4°C. Pelleted cells were washed with cold PBS and resuspended in 0.04% BSA in PBS at concentration 9.74 × 105 cells/ml with 83% viability (8.03 × 105 live cells/ml).
A single-cell droplet library of the pooled Notch1+/−/wildtype OFTs samples was generated using the 10xGenomics Chromium controller from this suspension according to the manufacturer's instructions. The quality and integrity of the cDNA library was quantitated using the High Sensitivity D5000 and D1000 ScreenTape (Agilent# 5067-5592 and 5067-5584) on the Agilent-2200 TapeStation. The library was sequenced (150 bp paired-end) using the Illumina HiSeq 4000 platform at the Steve and Cindy Rasmussen Institute for Genomic Medicine at Nationwide Children's Hospital. A total of 337,081,925 paired end reads and a total of 2,116 cells were detected for the pooled sample. Thus, on average, the transcriptome of each cell was evaluated using 159,301 reads.
Analysis of scRNA-Seq Data
Illumina.bcl files were demultiplexed and converted into per-sample FASTQ files using the 10x Genomics cellranger “mkfastq” command. The FASTQ files were then used to create a gene-count matrix using the cellranger command “count” using the mm10 genome version index from 10X genomics (https://cf.10xgenomics.com/supp/cell-exp/refdata-gex-mm10-2020-A.tar.gz) modified to include Neomycin resistance sequence using “reform” (https://gencore.bio.nyu.edu/reform/; accessed 09/15/2020). The expression of Neomycin resistance gene (NeoR) was used to identify Notch1 heterozygote cells. The count output file was then imported into the Seurat (v 3.0) package in R (45). Cells with at least 1 read mapping to NeoR gene were removed and the rest of the cells were used for further analysis. These NeoR negative cells were filtered for number of expressed genes and percentage of mitochondrial gene expression, normalized, subject to principal component analysis using the highest variable genes, and scaled as described earlier (46). Following this, dimensional reduction was performed using the RunUMAP and RunTSNE functions of Seurat using the first 20 principal components, and cells were clustered using the Louvain algorithm using a resolution factor of 0.5. The clustered cells were renamed using markers described in literature into vascular smooth muscle cells (VSMC), mesenchymal cells (Mes), endothelial cells (EC), myocardial cells (Myo), epicardial cells (epi), blood and ectodermal cells (48). Blood and ectodermal cells were removed for further analysis and dimensional reduction and clustering was repeated for the mesodermal cells.
Using these clusters, we examined cell-type specific expression of genes. Cell type-specific expression was examined by comparing a gene in an individual cluster against all other cells in the dataset using the “FindAllMarkers” function in R. As changes in the number of cells expressing a gene as well as the changes in the average expression per cell can contribute to differential expression, we used a threshold for both of these attributes. For differential expression between a cluster and rest of the cells, log fold change threshold was set to 0.5 (logfc.threshold = 0.5) and minimal percentage of cells positive for a gene's expression within a cluster set to 25% (min.pct = 0.25) and Wald test was used to evaluate statistical significance. The genes that showed a significant differential expression between a cluster vs. rest of the cells (adj. P-value <= 0.05) were deemed to have tissue/cell type-specific expression patterns. Genes which were found to have a cell-type specific expression in blood or ectodermal clusters were removed from further analysis for comparison to congenital heart gene candidates. Heatmaps created using the pheatmap function in R was used to visualize the tissue-specific expression of various genes.
Histology and Immunohistochemistry
Whole E12.5 and E13.5 embryos were harvested, fixed in 4% paraformaldehyde (Electron Microscopy Sciences) overnight, washed briefly with 1X PBS, and processed using the Leica ASP2065 tissue processor and standard protocol. Tissues were embedded in paraffin and sections collected at a thickness of 6 μm. Staining was performed using Hematoxylin and Eosin (H&E) (Sigma Aldrich) according to the manufacturer's protocol. E13.5 embryos (n = 5) were used to examine β-Catenin expression by immunohistochemistry using anti-β-catenin antibody (1:200, Abcam, #ab16051) and anti-rabbit SignalStain Boost IHC Detection reagent (Cell Signaling Technology, 8114). Stained tissue was visualized using a Signal Stain DAB Substrate kit (Cell Signaling Technology, 8059). Sections were washed using TBS containing 0.1% Tween-20.
Bioinformatics and Statistical Analysis for Human CHD Association Studies
Exome sequencing data generated by the Pediatric Cardiac Genomics Consortium (PCGC) was previously analyzed and published by Jin et al. (21). A patient-specific table of genes with variants was created from the Supplementary data published by Jin et al. and is referenced in this publication. Variants, as reported in Jin et al. are already filtered based on population frequency. We removed synonymous variants from this list. From the remaining dataset, patients diagnosed with tetralogy of Fallot without additional syndromic features (n = 419) were examined for de novo variants. A list of genes with at least one de novo non-synonymous variant amongst the TOF patients was examined for a potential mouse homolog using the BioMart tool in Ensembl and the gene list was named tetralogy of Fallot, de novo variant gene with a mouse homolog (TOF-DN-MH) (49). Of the 327 genes with de novo variants in the TOF cohort, 270 of them had a mouse homolog that was identified to be expressed in OFT cell types (as determined in our single cell analysis).
We next wanted to determine if the list of 270 genes with de novo variants expressed in OFT cell types was enriched for genes that are differentially expressed in the Notch1+/−; Nos3−/− mouse model. Of the 1,352 DEGs from the RNA-Seq analysis of the Notch1+/−; Nos3−/− mouse model, 1,087 of them have human homolog. This list was named differentially expressed genes in Notch1+/−; Nos3−/− OFTs with a human homolog (DEG-HH). The DEH-HH genes were then compared back to the 270 TOF-DN-MH to determine how many genes were in common. A total of 29 genes were found to be in common, i.e., having a de novo variant in TOF patients and being differentially expressed in the Notch1+/−; Nos3−/− mouse model.
To determine if these 29 genes represented a significant over-representation (enrichment) of de novo variants in genes shown to be differentially expressed in the Notch1+/−; Nos3−/− mouse model, we utilized a test based on the hypergeometric distribution (equivalent to the one-tailed Fisher's exact test) as follows: the number of DEGs with a de novo variant represents the overlap (29; q); the total number of human genes with a de novo variant and a mouse homolog as group 1 (TOF-DN-MH = 270; m); the total number of known human genes with a mouse homolog represents the population size (16,536 – 270 = 16,266; n); the total genes that are differentially regulated in the mouse OFT data which also have a human homolog as group 2 (DEG-HH = 1,054; k). The cumulative probability (h) of getting equal or more genes in the overlap was calculated using the phyper function in R as follows:
We also examined if there is an increased burden of de novo missense and loss of function variants amongst these 30 genes using denovolyzeR (50). DenovolyzeR uses a theoretical rate of de novo variants estimated from the evolutionary changes between primate and human genomes to determine increased burden of de novo variants in patient cohorts. This allows us to examine if there is a significantly higher number of de novo loss of function and missense variants in genes in TOF patients compared to a theoretical estimate. We used overlapping genes as the genes to focus on for this analysis.
We also extracted variant information of patients with semilunar valve disease (n = 245) and patients diagnosed with hypoplastic left heart syndrome (n = 371) using the same parameters described above and named these lists SLV-DN-MH and HLHS-DN-MH, respectively. A total of 19 genes were seen in the SLV-DN-MH intersected with DEG-HH, while a total of 17 genes were seen in the HLHS-DN-MH intersected with DEG-HH. These overlaps were then evaluated using the hypergeometric distribution as described above.
Comparison of the DEG-HH genes with a second cohort of TOF-patients was done using data set from Page et al. (24). Page et al. reported non-synonymous variants filtered using cut-offs in population frequency and CADD scores with damaging effects. However, de novo variants and inherited variants were not separated in this dataset. Therefore, this analysis was different from the analysis of de novo variants in PCGC. To analyze the 829 TOF patient variants reported in Page et al., we used all the genes that had at least one variant in this dataset and a strong mouse homolog (n = 7,907) and named this gene list TOF variant genes (TOF-VAR-PAGE-MH). In order to be consistent between data sets, in parallel, we created a similar gene list with at least one variant (de novo and inherited) as reported in Jin et al. and named this list TOF-VAR-JIN-MH (n = 1,587). We compared these two patient lists to the DEG-HH list in two steps. In step 1, we examined the individual overlap between the DEG-HH list and TOF-VAR-PAGE-MH gene list and the TOF-VAR-JIN-MH gene list. Then in step 2, we identified common genes that appear in the overlap between these individual comparisons from step 1, focusing on those expressed in OFT cell types. Here, a hypergeometric distribution-based test was applied to calculate the probability of finding common genes amongst Step 1-overlapping genes. The population size was set to the number of DEG-HH genes.
To test whether high expressing genes are predictive of damaging genetic variation in patients with TOF, we selected the top 1,352 genes that showed the highest expression in the OFT RNA seq data in the wildtype embryos and the gene list was named highest expressing mouse outflow tract genes (HEM).This list was of equal number to the DEG gene list in the mouse as to minimize the number of variables between comparisons. The expression, in this case, was determined by calculating the average fragments per kilobase per million reads (FPKM) calculated for each gene in the wildtype dataset. We then overlapped the 270 genes with de novo variants identified in the TOF-DN-MH gene list with the HEM gene list and the overlap was then evaluated using a hypergeometric distribution as described above.
Results
Characterization of Notch1+/−; Nos3−/− Embryos Reveal Normal Endocardial Cushions
We previously reported a spectrum of cardiac OFT malformations including highly penetrant thickened, malformed semilunar valves and the partially penetrant phenotypes consisting of ventricular septal defects and an overriding aorta in Notch1+/−; Nos3−/− embryos (37, 38). Using the Notch1+/−; Nos3−/− mouse as a genetic tool, we wanted to determine if genes differentially expressed in cells required for the development of the cardiac outflow tract are predictive of damaging de novo variants in patients with OFT malformations (Figure 1).
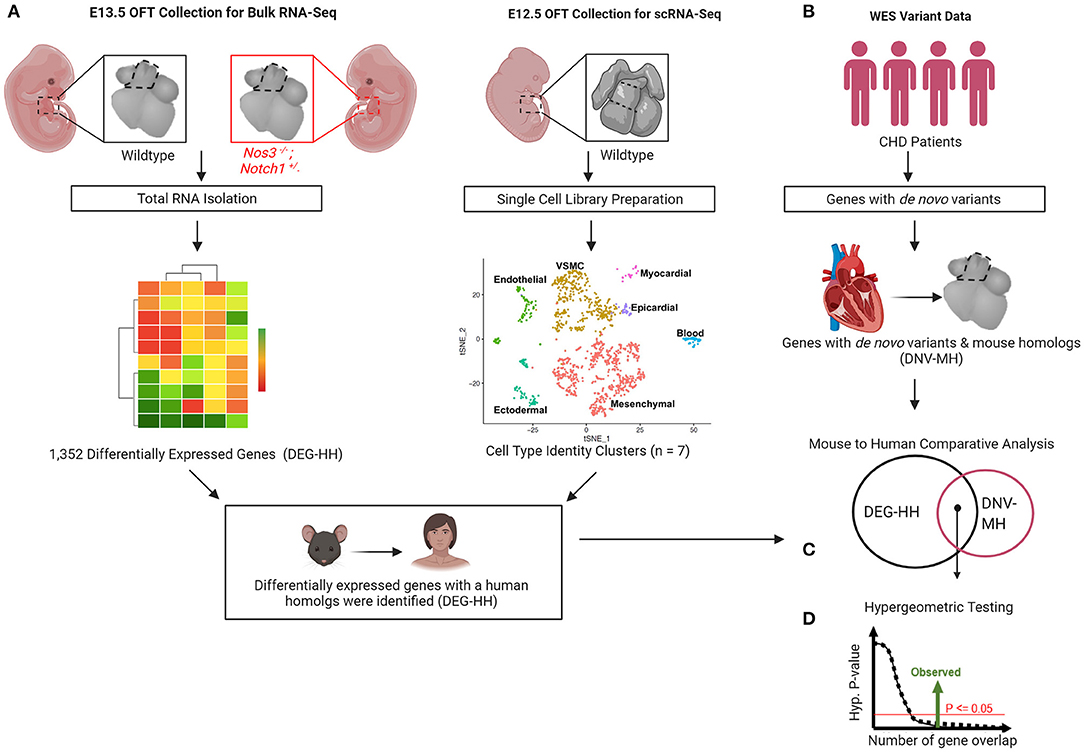
Figure 1. Gene discovery pipeline for human congenital heart disease (CHD) utilizing transcriptomic profiling from wildtype and mutant mouse embryos. (A) Differential gene expression by bulk RNA-Sequencing of E13.5 Notch1+/−; Nos3−/− mutant and wildtype outflow tracts (OFT) was performed. (B) scRNA-Sequencing transcriptomes derived from wildtype OFT was used to categorize cell identity clusters. (C) Differentially expressed genes (DEG) were categorized according to cell identity clusters and human homologs were identified. (D) De novo gene variants were extracted from WES databases of CHD patients and those with mouse homologs identified. (E) Mouse to human comparative analysis with hypergeometric testing was performed to determine statistical significance.
Semilunar valve defects are the most predominant phenotype observed in E15.5 and E18.5 compound mutant embryos. First, we compared E12.5 and E13.5 Notch1+/−; Nos3−/− compound mutant embryos to littermate controls (Nos3+/−) to determine the timing of disease onset. By gross examination, Notch1+/−; Nos3−/− compound mutant embryos at E12.5–E13.5 were grossly normal compared to littermate controls (Figure 2A). By histologic section, the developing outflow tract cushions in compound mutant embryos are indistinguishable from controls at E12.5 and E13.5 (Figure 2B). These results suggest that the onset of semilunar valve disease occurs later in development while the ventricular septal defect with overriding aorta phenotypes are likely the result of earlier developmental abnormalities.
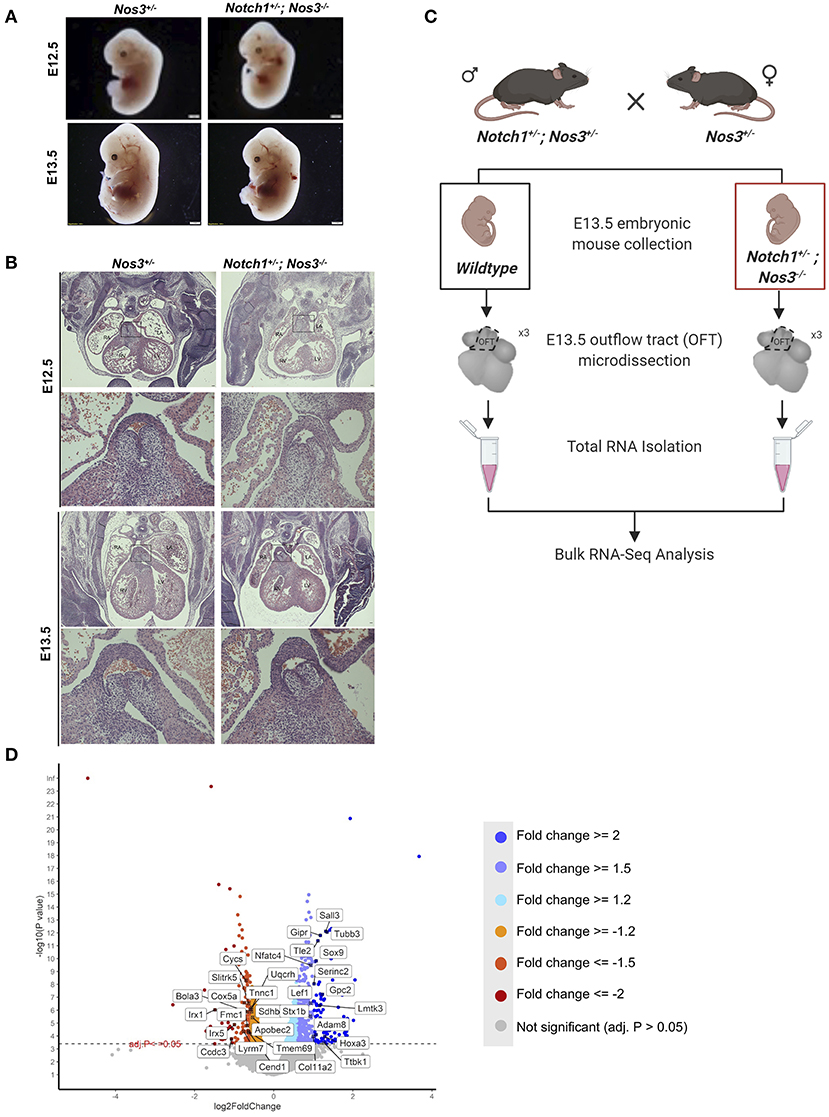
Figure 2. Histologic and RNA-Seq analysis of Notch1+/−; Nos3−/− embryos. (A) Notch1+/−; Nos3−/− compound mutant embryos do not show any size differences at E12.5 or E13.5 (n = 3). (B) Histological section (stained with hematoxylin and eosin) of E12.5 and E13.5 Notch1+/−; Nos3−/− embryonic hearts demonstrates no gross morphological differences. Outflow tract (boxed area in low magnification image) is shown in higher magnification (below) and no differences are noted when compared to Nos3+/− littermate controls (n = 3). (C) Schematic diagram outlining process of OFT collection and bulk RNA-Sequencing from E13.5 Notch1+/−; Nos3−/− and wildtype embryos. (D) Volcano plot of 1,352 differentially expressed genes sorted according to fold change and significance (adjusted p-value < = 0.05). Significantly downregulated genes with a fold change < −2, < −1.5, < −1.2 are labeled in orange, coral, and red, respectively. Significantly upregulated genes with a fold change > 2, > 1.5, > 1.2 are labeled in turquoise, light purple, and blue, respectively. Highlighted genes are top 15 upregulated and downregulated genes that also have a Notch1 ChIP peak according the previously published Notch1/Rbpjk in mouse ChIP-Sequencing dataset (51). Figure 2C was generated on www.Biorender.com.
Transcriptomic Profiling of the Notch1+/−; Nos3−/− OFT Reveals Dysregulation of Multiple Molecular Pathways
We hypothesized that molecular alterations in the OFT of E13.5 Notch1+/−; Nos3−/− compound mutant embryos contribute to the resultant outflow tract malformations found at later timepoints. Accordingly, Notch1+/−; Nos3+/− males were bred to Nos3+/− females to obtain E13.5 Notch1+/−; Nos3−/− compound mutants and wildtype (Notch1+/+; Nos3+/+) littermate controls. The embryonic OFT was micro-dissected from E13.5 mutant and wildtype embryos, and RNA was isolated for bulk RNA-Seq (Figure 2C). We found 1,352 differentially expressed genes (DEG) which are depicted in the volcano plot (Figure 2D; Supplementary Table 1). Network analysis and visualization using hierarchal heatmap and chord diagrams suggested that Hif1α, Tgf-β, Hippo, Wnt signaling amongst several others were affected in Notch1+/−; Nos3−/− as multiple dysregulated genes were predicted to participate in these pathways (Figures 3A,B) (52, 53). Quantitative RT-PCR of 5 highly DEG, including Nos3, Netrin-1, Bmp5, and Wnt2, was performed to validate transcriptomic profiling results (data not shown). Among these pathways, we further examined the Wnt signaling pathway as it is a known contributor to endocardial cushion development and myxomatous heart valve disease (54). The consequence of transcriptomic changes in Wnt signaling pathway members can be assessed by inspecting β-catenin protein levels. We found increased staining of β-catenin in the endocardium and endocardial cushions of E13.5 Notch1+/−; Nos3−/− embryos as compared to wildtype controls (Supplementary Figure 1A). Inspection of other disrupted signaling pathways within the Notch1+/−; Nos3+/− OFT is ongoing and beyond the scope of this work. Overall, these results suggest that global disruption of Notch and nitric oxide signaling has potential downstream effects on molecular pathways required for OFT development including Hif1α, Tgf-β, Hippo, and Wnt signaling.
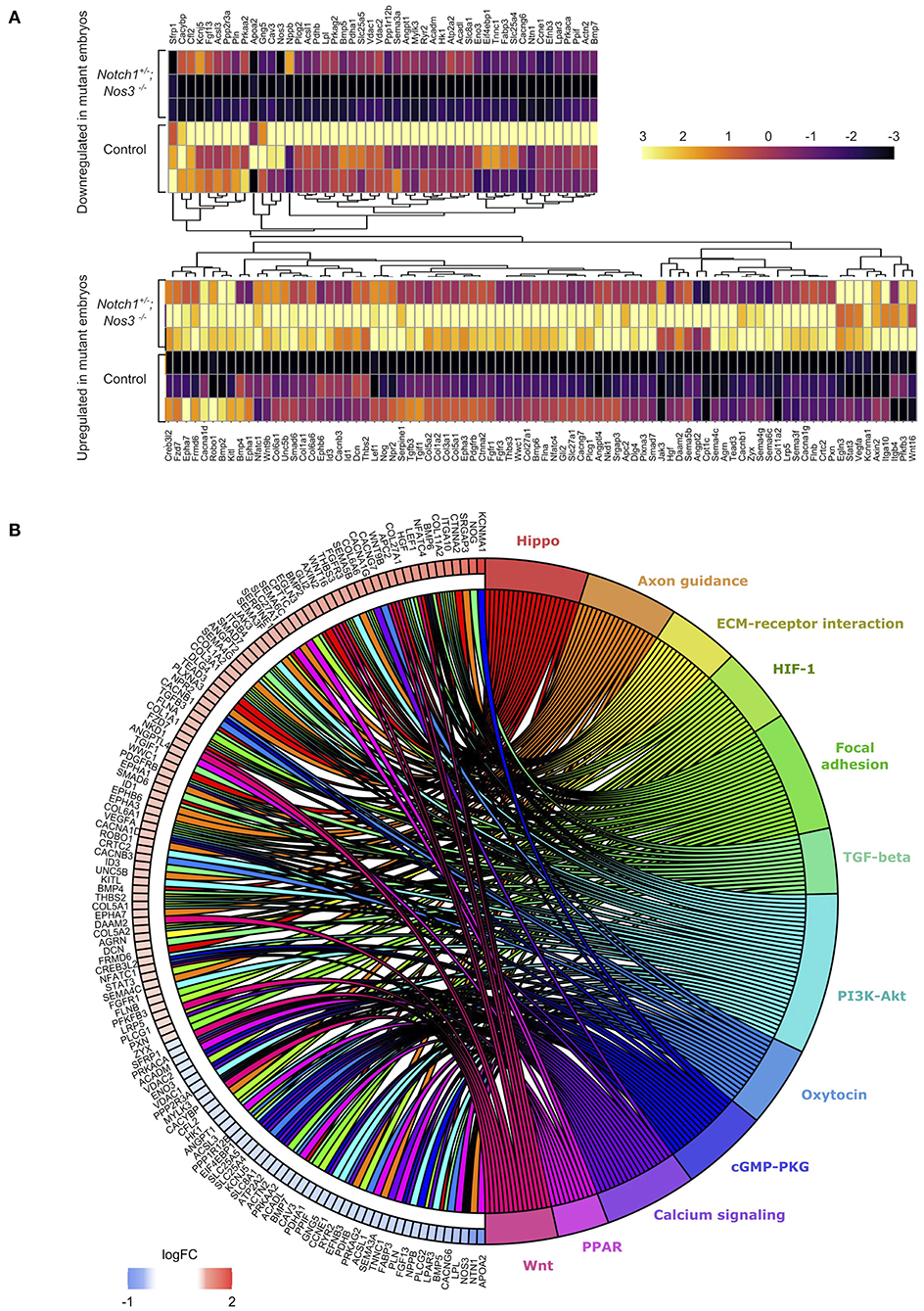
Figure 3. Differential gene expression analysis (DESeq2) shows significant gene expression changes between Notch1+/−; Nos3−/− compound mutants and wildtype (WT) littermate controls. (A) Split hierarchical heatmap cluster show differential gene expression among biological replicates obtained from transcriptomic profiling of Notch1+/−; Nos3−/− compound mutants and wildtype littermate controls for a subset of genes (n = 3 for each condition). (B) Chord plot shows 132 differentially expressed genes identified in bulk RNA-Seq analysis and predicted signaling pathway associations.
Classification of Bulk Transcriptomic Data Into Seven Cell Identity Clusters by Single-Cell RNA Sequencing (scRNA-Seq)
The cardiac OFT is composed of multiple cell types, and in order to determine molecular and cellular pathways which were disrupted in the E13.5 Notch1+/−; Nos3−/− RNA-Seq datasets, we performed scRNA-Seq of the embryonic OFT. scRNA-Seq data generated from pooled E12.5 cardiac OFT was readily available to us and was used for subsequent analysis. Following pre-processing steps, which revealed 1,354 cells were captured within the sample, tSNE visualization was performed and clustering analysis found nine distinct groups within the wildtype E12.5 OFT (Figure 4A). Clustering annotation was performed by finding the gene signature of each cluster using marker genes that delineate cell identities (55–59). The clusters were reduced and classified as mesenchymal, vascular smooth muscle, endothelial, ectodermal, epicardial, myocardial, and blood cells (Figure 4B; Supplementary Figure 1B). Next, we re-analyzed the 1,352 gene transcripts identified in the bulk RNA-Seq to be DEG according to the scRNA-Seq gene cluster identities in which they are predominantly expressed (Figure 4C). The DEG transcripts (1,039) with a human homolog (1,054) were categorized by cell type, due to mouse transcripts mapping to multiple homologous human genes (Figure 4D). In parallel, we examined the tissue-specific expression of the 1,352 DEG genes. We found 431 of these mouse genes preferentially expressed amongst the seven cell identity clusters. Those that were also differentially expressed in Notch1+/−; Nos3−/− compound mutant embryos are referred henceforth as DEG mouse gene list (Supplementary Tables 1, 2). This analysis demonstrated disruption of molecular pathways in each of these cell types (myocardial, mesenchymal, VSMC, endothelial) that populate the developing OFT.
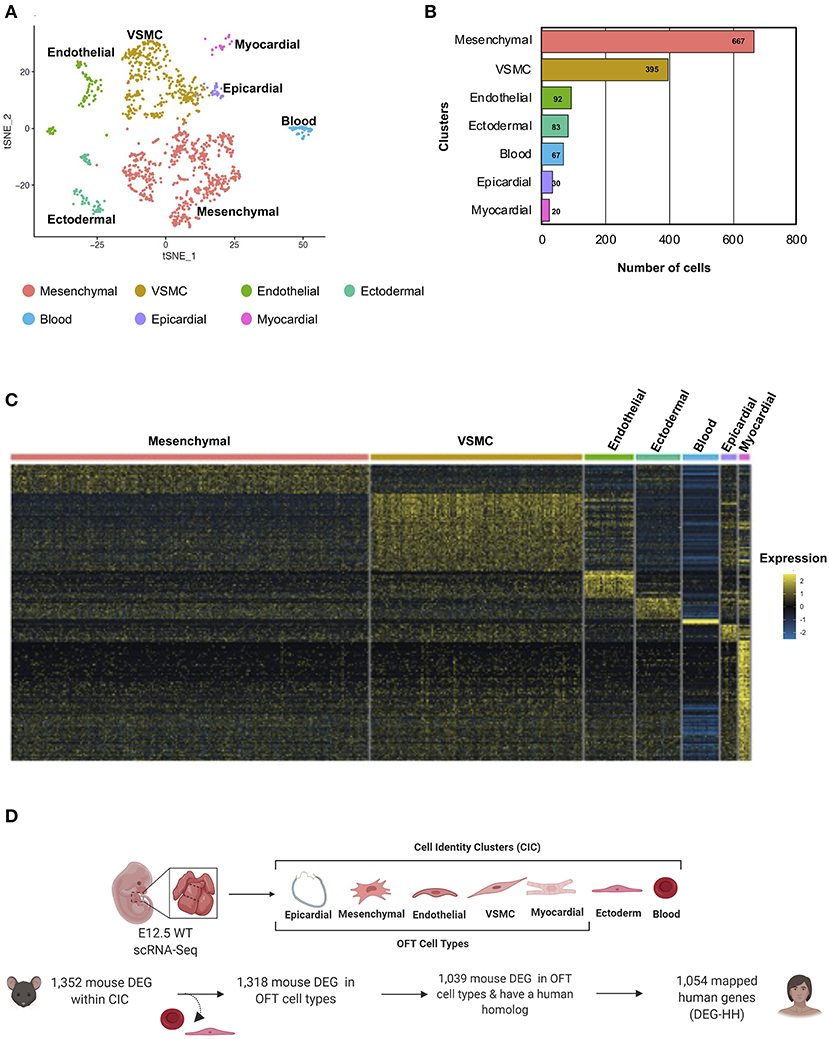
Figure 4. Cellular classification of gene transcripts identified in bulk RNA-Seq utilizing scRNA-Seq analysis of the embryonic cardiac outflow tract. (A) tSNE plot of cells captured via scRNA-Seq is shown and classified according to cell identity markers. The identified subclusters are mesenchymal, vascular smooth muscle cells (VSMC), endothelial, ectodermal, blood, epicardial, and myocardial cells. (B) Raw count of cells within each cluster indicated that 1,354 cells were captured from the cardiac outflow tract. (C) Wildtype gene transcripts identified via bulk RNA-Seq were classified according to scRNA-Seq cell cluster profile; heatmap of overlapping genes is shown. (D) Schematic of how the DEG-HH gene list was generated. Differentially expressed genes that were also found to be expressed in the scRNA-Seq dataset were selected. These were filtered based on cell-type specificity and whether they possess a human homolog to generate a candidate list of 1,054 human genes. Figure 4D was generated on www.Biorender.com.
Differentially Expressed Genes in Notch1+/−; Nos3−/− Mouse OFT Are Enriched as de novo Variants in TOF Patient Cohorts
A large number of patients with CHD have undergone genomic sequencing and numerous potential genetic contributors have been reported (60). We asked if differentially expressed genes in a mouse model of cardiac OFT malformations overlapped with identified putative disease-causing variants reported from large cohort studies. We selected 419 patients from the Pediatric Genomics Consortium (PCGC) published in Jin et al. with a primary diagnosis of TOF (Supplementary Table 3A). We examined the rare de novo variants (population frequency < = 0.05) found in these 419 TOF patients (23). To compare this data to the mouse model, we retained only those genes with a de novo variant and a mouse homolog (TOF-DN-MH), which numbered 271. This was filtered further to 270 by selecting against genes that are predominantly expressed in ectoderm and blood as developing ectoderm and blood cells are not known to contribute to development of the OFT or TOF (Supplementary Table 1). From the mouse model, we selected 1,352 significantly impacted (adjusted P-values < = 0.05) transcripts from the Notch1+/−; Nos3−/− OFTs and identified its human homolog (DEG-HH, n = 1,054) expressed in OFT cell types (Figure 4D). By assessing genes expressed in OFT cell types (myocardial, epicardial, mesenchymal, endothelial, and VSMC) for genetic homologs, we found an enrichment of de novo gene variants in the TOF-DN-MH population and the DEG-HH gene lists as according to statistical analysis (hypergeometric p-value = 0.0042). Ultimately, we found 29 genes with de novo variants that are likely contributors of TOF considering they are dysregulated in a mouse model of OFT-disease, present as damaging, de novo variant carrying genes in this CHD genomic dataset and predominantly expressed in OFT cell types (Figure 5A; Table 1).
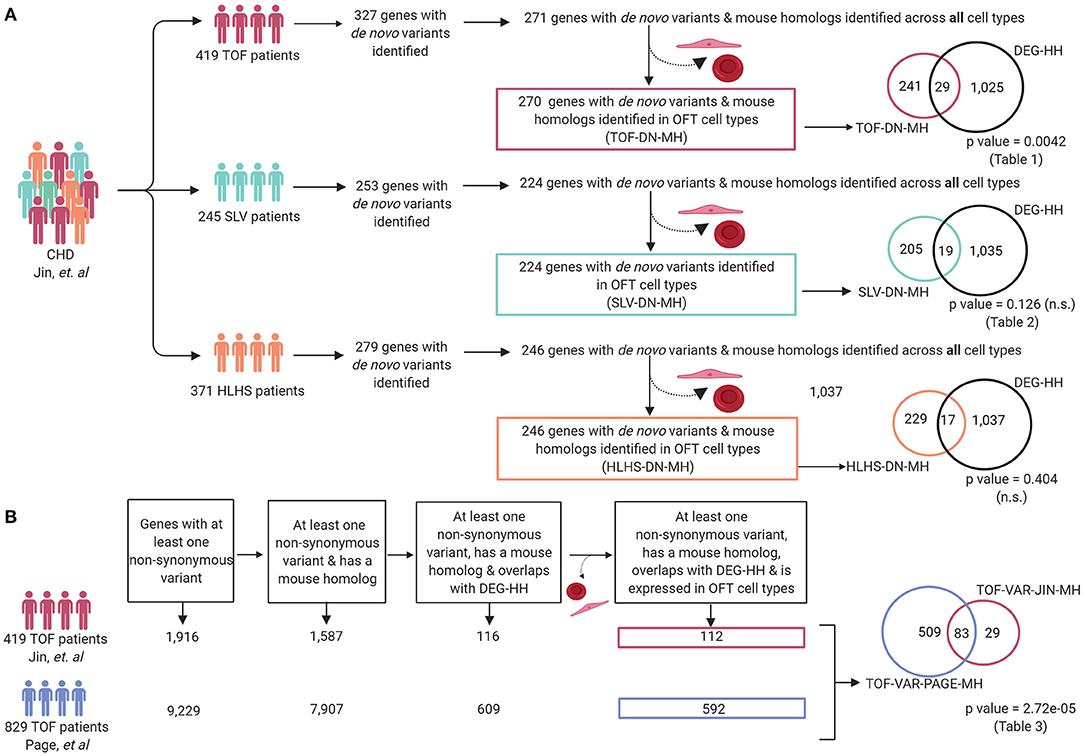
Figure 5. Differentially expressed genes in Notch1+/−; Nos3−/− compound mutants are enriched within reported genetic variants from TOF but not SLV and HLHS patients. (A) Patients with a primary TOF and SLV diagnosis and their associated de novo variant information were extracted from Jin et al. and compiled for analysis (TOF-DN-MH and SLV-DN-MH, respectively). Overlapping of TOF-DN-MH and SLV-DN-MH with DEG-HH demonstrated a significant enrichment of genes between TOF-DN-MH and DEG-HH but not SLV-DN-MH (p-value = 0.0042 and p-value = 0.126, respectively). Overlapping of the HLHS-DN-MH cohort with DEG-HH failed to reach statistical significance (p-value = 0.404). (B) Damaging, non-synonymous variants specific to TOF patients were extracted from Page et al. and Jin et al. and assessed for mouse gene homologs and compiled for analysis (TOF-VAR-PAGE-MH & TOF-VAR-JIN-MH, respectively). After overlapping with differentially expressed genes in the Notch1+/−; Nos3−/− compound mutants and assessing those genes found in OFT cell types, we found 83 shared genes among the 112 (TOF-VAR-JIN-MH) and 592 (TOF-VAR-PAGE-MH) genes in each cohort (p-value = 2.72e-05). Figure was generated on www.Biorender.com.
Among the identified genes, several have been previously implicated to contribute to outflow tract development in mice. Not surprisingly, members of the Notch signaling pathway, specifically Notch1 and Jag2, were identified to be differentially expressed in mice and identified to possess damaging, de novo variants in TOF patients (38, 39, 72, 86). Genes required for endocardial cushion development in mice Snai1, Vcan, and Smad6 were also identified as strong candidates (7, 80, 82). Genes not previously implicated in the development of the cardiac outflow tract yet recognized to contribute to other aspects of heart development were identified including Myh6, Pkp2, Sema3a, Fat4, Hdac7, Ddr2, Med13l, and Acsl1 (66, 67, 70, 71, 75, 77–79, 87–89). Literature review of the remaining gene candidates found no CHD phenotypes having been described in existing mouse models.
Considering Notch1+/−; Nos3−/− mice display a wide range of SLV malformations, we repeated the previously outlined analysis on 245 patients from PCGC with CHD affecting the semilunar valves, aorta and aortic arch arteries (encompassed the following phenotypes: aortic and pulmonary valve stenosis, patent ductus arteriosus, aortic arch artery malformations, bicuspid, and unicuspid aortic valve) (Figure 5A; Supplementary Table 3B). In repeating the analysis outlined previously for TOF patients, we identified 224 genes with de novo variants present in SLV disease populations in OFT cell types, 19 of which overlapped with the DEG-HH list. This overlap did not reach statistical significance (hypergeometric p-value = 0.126) (Figure 5A; Table 2).
To determine the utility of our mouse to human comparative analysis pipeline, we performed a secondary analysis using a distinct cohort consisting of 829 non-syndromic TOF patients described by Page et al. as described previously with minor modifications (Figure 5B; Supplementary Table 3D) (24). As the inheritance of these variants was not available, we generated a list of genes that were reported to possess at least one non-synonymous variant and also had a mouse homolog (TOF-VAR-PAGE-MH). Then, we overlapped the TOF-VAR-PAGE-MH gene list with the DEG-HH list and to generate a filtered list of 592 candidates that were identified to have non-synonymous variants in patients with TOF, possess a mouse homolog, be differentially expressed in Notch1+/−; Nos3−/− mouse OFT and expressed in OFT cell types. In parallel, we generated a candidate gene list using TOF patient data derived from Jin et al. that included genes all non-synonymous variants (de novo and inherited) that also possessed a mouse homolog (TOF-VAR-JIN-MH). We overlapped the TOF-VAR-JIN-MH gene list with the DEG-HH and assessed for expression in OFT cell types as described previously to generate a filtered list of 112 candidates. We then examined whether there was a significant overlap of genes with all non-synonymous variants, regardless of inheritance, between both TOF cohorts (TOF-VAR-PAGE-MH vs. TOF-VAR-JIN-MH) that were also identified to be differentially expressed in Notch1+/−; Nos3−/− mouse OFT. In doing so, we identified 83 shared genes which reached statistical significance (2.72 × 10−5) (Table 3; Figure 5B). Of these identified genes, 13 were associated with a cardiovascular phenotype as determined by the Online Mendelian Inheritance in Man compendium which includes ABCC9, CENPF, HADHA, HSPA9, MED13L, MIPEP, MYH6, MYPN, NEXN, NOS3, NOTCH1, SMAD6, and TNNT2. Thirty-six of these genes are not yet linked to human disease; 18 of which were previously linked to cardiovascular development in various animal modeling systems.
To test the validity of our gene prioritization pipeline, we performed two separate bioinformatic analyses. First, we repeated mouse to human comparative analysis in a non-OFT malformation population (Figure 5A; Supplementary Table 3C). Patients with hypoplastic left heart syndrome (HLHS) are characterized by a hypoplastic left ventricle, aorta, and mitral valve. HLHS is recognized to be an oligogenic and genetically heterogeneous type of CHD therefore, we hypothesized there would be minimal to no overlap between genes with de novo or inherited variants and the DEG-HH gene lists generated by our OFT-disease model than expected by chance (55). As expected, there was no more overlap of genes (n = 17) between these gene lists than by chance suggested by our statistical test (hyper p-value = 0.39).
Previous research identified enrichment of damaging de novo mutations in patients with multiple CHD diagnoses in genes considered to be highly expressed in the developing heart as this was used by the PCGC (105). Using the bulk RNA-Seq transcriptome, we selected the top 1,352 genes with the highest average RPKM across all three sequenced wild-type OFT samples, identified their genetic homolog, and determined whether there was the overlap of genes with de novo mutations in the TOF-DN-MH variant list and genes highly expressed in mouse (HEM) OFT (Figure 6; Supplementary Table 4). We were unable to identify any more genes with de novo mutations identified in this comparative analysis than what was expected by chance (n = 10). Removal of genes expressed primarily in ectodermal, and blood cells from the HEM OFT gene lists did not improve this association. Overall, our results demonstrate that mouse to human comparative analysis can identify with statistical significance genes with de novo variation in distinct CHD populations.
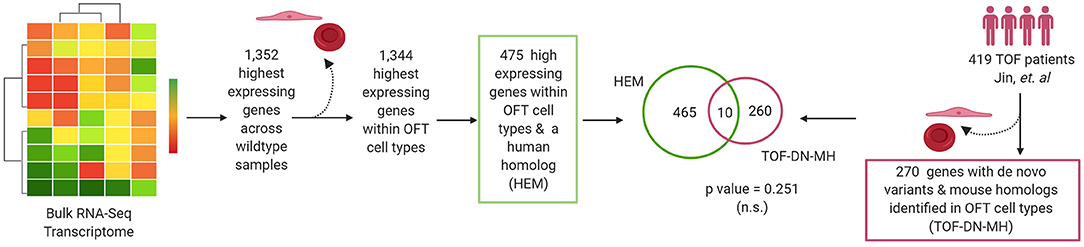
Figure 6. Highest expressed genes in cardiac outflow tract identified by RNA-Seq are not predictors of de novo variants in tetralogy of Fallot (TOF) cohorts. The highest expressing genes (n = 1,352) across all three wildtype mouse outflow tract (OFT) samples were selected. Of these (n = 475) were expressed with OFT cell types and also possessed a human homolog. We assessed the overlapped the TOF-DN-MH gene list with the HEM gene list in OFT cell types and determined no enrichment of genes between these datasets (p-value = 0.251). Figure was generated on www.Biorender.com.
Discussion
By using the differentially expressed transcriptome from a mouse model of CHD, we demonstrate a methodology that may allow for improved classification of potential genetic contributors that are generated from exome sequencing of patients with CHD. Notch1+/−; Nos3−/− mice are a highly penetrant model of cardiac OFT malformations. Differential gene expression and network analysis of E13.5 Notch1+/−; Nos3−/− OFT showed that multiple genes and pathways were disrupted downstream of Notch1 and Nos3 including the Hif1α, Tgf-β, Hippo, and Wnt signaling pathways. We reclassified wildtype gene transcripts identified in the bulk RNA-Seq according to cellular identity clusters generated from wildtype scRNA-Seq data. In doing so, we generated a list of candidate genes expressed in known cellular contributors of the developing OFT. We found that genes that were differentially expressed in E13.5 Notch1+/−; Nos3−/− OFT (DEG-HH) are present as damaging, de novo variants in patients diagnosed with TOF. There was specificity of our pipeline as it failed to detect a significant enrichment of genes with de novo variants in a HLHS population. We were also unable to detect an enrichment of genes with de novo mutations in the TOF-DN-MH variant list when compared to gene lists generated from the highest expressing genes in the mouse OFT. In summary, these findings highlight the value of utilizing transcriptomic profiling datasets from highly penetrant mouse models of disease when attempting to determine the clinical significance of genetic variants identified by large scale sequencing efforts of patients with CHD (Figure 1).
Our current understanding of definitive genetic contributors of OFT-derived malformations is limited to familial inheritance studies, sequencing of large populations of affected individuals, and mouse modeling approaches. TOF can occur in isolation (non-syndromic) as well in combination with non-cardiac anomalies (syndromic). Syndromic TOF accounts for ~20% of cases (e.g., 22q11.2 deletion syndrome) while the genetic contributors of non-syndromic TOF are not entirely elucidated. Single-gene knockout studies in mice have been instrumental in describing in detail the morphological changes that occur in the developing OFT following gene disruption. Oligogenic disruptions are also known to contribute to heart disease as noted by exome sequencing of trios and compound mouse mutant modeling (106). Through this publication, we have been able to demonstrate the benefit of a mouse to human comparative analysis to prioritize genes candidates previously understudied in the development of non-syndromic TOF. Global and conditional deletion mouse strains are available for many of the prioritized gene candidates identified in this publication. However, many of these candidates have not been studied in the context of cardiac OFT development which hinders our understanding of their functional role in the heart. Similarly, while exome sequencing approaches have identified potential damaging contributors of high statistical significance, these studies lack in vivo validation using animal models which limits the application of these findings in a clinical setting. We propose that mouse models of disease, such as the Notch1+/−; Nos3−/− compound mutant mouse line, are instrumental tools as they provide much needed in vivo evidence of gene candidates identified through large-scale sequencing screens.
We recognize that there are limitations to our work that hinder our ability to make more definitive and potentially more clinically relevant conclusions. First, although Notch1+/−; Nos3−/− animals are recognized to be a highly penetrant model of OFT malformations, the phenotype observed is quite variable. The predominant phenotype observed is SLV stenosis however TOF-like phenotypes are also observed at a lower rate. Therefore, we suspected that transcriptomic profiles generated from E13.5 Notch1+/−; Nos3−/− OFTs would differ between sequenced samples. Accordingly, we did note that one of the three samples sequenced was substantially different from the other two. In lieu of removing available RNA-sequencing data, we decided it was best to proceed with n = 3 datasets despite the variability observed as one could argue that disease observed between CHD patients harboring the same genetic mutation is also highly variable. To our knowledge, there is no perfect animal model for OFT malformations, and we argue that the identification of dysregulated genes in murine models of CHD could provide sufficient functional evidence to better assess variants of unknown significance identified by genetic sequencing of affected populations. Furthermore, these type of analyses offer initial observations to stimulate the study novel genetic contributors of OFT development as compared to making use of transcriptomic data derived from unaffected animals with normal hearts. Second, we recognize that we may have selected too late of a time point in the development of the cardiac OFT to investigate potential contributors of TOF. At E13.5 the location of the great vessels has already been established; one of the hallmarks of TOF is a displacement of the aorta. Similarly, E13.5 SLV has already undergone EMT, although valve remodeling and elongation has not yet occurred. It is possible that by performing transcriptomic profiling of E13.5 Notch1+/−; Nos3−/− OFTs we only detected the tail-end expression of critical developmental pathways required for the development of the great vessels and EMT of the semilunar valve, which may affect our downstream analysis. However, we were able to detect several genes in patients with TOF that were also differentially expressed in mouse OFT which suggests our findings are of clinical relevance. Future studies using cross-species analysis should take into consideration the developmental milestones that occur prior to the onset of disease before performing a transcriptomic analysis of mouse models. Another limitation was the use of an scRNA-seq E12.5 OFT library as opposed to an E13.5 timepoint. Currently, there is no publicly available single-cell RNA-seq data set of the E13.5 OFT. Developmentally, the E12.5 and E13.5 OFT are similar considering EMT is still underway at both timepoints and the contributions of the cardiac neural crest and second heart field to OFT septation are complete. Therefore, while not the ideal we do not believe the classification of transcripts is significantly affected by this process nor does it impact downstream analysis. Finally, considering we selected variant information derived from publicly available databases we are limited to the information presented in these reports and unable to validate the bioinformatic conclusions we generated here. However, given the size of the patient populations selected and the filtering criteria used to call damaging genetic variation we are confident in our analysis as a method to identify causative genes involved in OFT malformations. Future work must focus on validating the expression and functionality of these gene products in developing hearts.
With the growing availability of exome and genome sequencing technologies, there is expected to be an increase in the use of this method of genetic testing in CHD populations. However, sequence variants identified in these patients are often classified as variants of uncertain significance (VUS) as opposed to being deemed pathogenic due to limited availability of relevant biological data. Barring very few examples, where the specific variants identified in human OFT malformation have been modeled in mice, there has been limited evaluation of the effect of the identified human sequence variant on the development of the OFT (41, 107). This has not only hampered the classification of novel variants as pathogenic or benign but also limits the establishment of clear links between CHDs and novel candidate genes identified in large patient cohort studies. One way to prioritize and strengthen the genetic link between novel candidate genes and CHDs is to determine if their expression is altered during the development of OFT in an animal model of the disease. As clinical databases become more enriched with patient sequencing information, transcriptomic profiling of disease models has the potential to provide additional data to assist in the classification of sequence variants. Our work demonstrates the utility of using a disease specific model to generate transcriptomic profiling data for the purposes of identifying genes of clinical significance in patients with TOF. Accordingly, we describe a pipeline that may improve the analysis of genetic sequencing data from human patient cohorts and overlay pathological relevance from disease models to purely bioinformatic findings.
Data Availability Statement
The original contributions presented in the study are included in the article/Supplementary Material. Single cell RNA-Sequencing data has been deposited in GEO under accession number GSE171239. Further inquiries can be directed to the corresponding author.
Ethics Statement
The animal study was reviewed and approved by Institutional Animal Care and Use Committee at Nationwide Children's Hospital.
Author Contributions
The experimental data was collected by AM-N and UM. Bioinformatics data analysis was performed by SM. Data analysis was reviewed and evaluated by AM-N, UM, SM, KM, PW, and VG. Original draft of the manuscript was generated by AM-N with contributions from SM and VG. All authors contributed to the designing of the experiments, drawing conclusions from the data, and editing of the manuscript.
Funding
Research reported in this publication was supported by the National Heart, Lung, and Blood Institute of the National Institutes of Health Award Number T32HL134616 (AM-N), Award Number T32HL098039 (SM) and Award Numbers R01-HL121797 and R01-HL132801 (VG).
Author Disclaimer
The content is solely the responsibility of the authors and does not necessarily represent the official views of the National Institutes of Health.
Conflict of Interest
The authors declare that the research was conducted in the absence of any commercial or financial relationships that could be construed as a potential conflict of interest.
Publisher's Note
All claims expressed in this article are solely those of the authors and do not necessarily represent those of their affiliated organizations, or those of the publisher, the editors and the reviewers. Any product that may be evaluated in this article, or claim that may be made by its manufacturer, is not guaranteed or endorsed by the publisher.
Acknowledgments
The authors thank Dr. Jianli Bi, for his expertise in preparation of 10x Genomics library for scRNA-seq. We are grateful to Emily M. Cameron, MS and Sara Adamczak, MS for their expert animal care and technical assistance.
Supplementary Material
The Supplementary Material for this article can be found online at: https://www.frontiersin.org/articles/10.3389/fcvm.2021.683074/full#supplementary-material
References
1. Benjamin EJ, Muntner P, Alonso A, Bittencourt MS, Callaway CW, Carson AP, et al. Heart disease and stroke statistics-2019 update: a report from the American Heart Association. Circulation. (2019) 139:e56–528. doi: 10.1161/CIR.0000000000000659
2. Verma S, Siu SC. Aortic dilatation in patients with bicuspid aortic valve. N Engl J Med. (2014) 370:1920–9. doi: 10.1056/NEJMra1207059
3. Ward C. Clinical significance of the bicuspid aortic valve. Heart. (2000) 83:81–5. doi: 10.1136/heart.83.1.81
4. Michelena HI, Prakash SK, Della Corte A, Bissell MM, Anavekar N, Mathieu P, et al. Bicuspid aortic valve: identifying knowledge gaps and rising to the challenge from the International Bicuspid Aortic Valve Consortium (BAVCon). Circulation. (2014) 129:2691–704. doi: 10.1161/CIRCULATIONAHA.113.007851
5. Meilhac SM, Buckingham ME. The deployment of cell lineages that form the mammalian heart. Nat Rev Cardiol. (2018) 15:705–24. doi: 10.1038/s41569-018-0086-9
6. Waldo KL, Kumiski DH, Wallis KT, Stadt HA, Hutson MR, Platt DH, et al. Conotruncal myocardium arises from a secondary heart field. Development. (2001) 128:3179–88. doi: 10.1242/dev.128.16.3179
7. Mjaatvedt CH, Nakaoka T, Moreno-Rodriguez R, Norris RA, Kern MJ, Eisenberg CA, et al. The outflow tract of the heart is recruited from a novel heart-forming field. Dev Biol. (2001) 238:97–109. doi: 10.1006/dbio.2001.0409
8. Zaffran S, Kelly RG, Meilhac SM, Buckingham ME, Brown NA. Right ventricular myocardium derives from the anterior heart field. Circ Res. (2004) 95:261–8. doi: 10.1161/01.RES.0000136815.73623.BE
9. Verzi MP, McCulley DJ, De Val S, Dodou E, Black BL. The right ventricle, outflow tract, and ventricular septum comprise a restricted expression domain within the secondary/anterior heart field. Dev Biol. (2005) 287:134–45. doi: 10.1016/j.ydbio.2005.08.041
10. Waldo KL, Hutson MR, Ward CC, Zdanowicz M, Stadt HA, Kumiski D, et al. Secondary heart field contributes myocardium and smooth muscle to the arterial pole of the developing heart. Dev Biol. (2005) 281:78–90. doi: 10.1016/j.ydbio.2005.02.012
11. Kirby ML, Gale TF, Stewart DE. Neural crest cells contribute to normal aorticopulmonary septation. Science. (1983) 220:1059–61. doi: 10.1126/science.6844926
12. Kirby ML, Waldo KL. Neural crest and cardiovascular patterning. Circ Res. (1995) 77:211–5. doi: 10.1161/01.RES.77.2.211
13. Jiang X, Rowitch DH, Soriano P, McMahon AP, Sucov HM. Fate of the mammalian cardiac neural crest. Development. (2000) 127:1607–16. doi: 10.1242/dev.127.8.1607
14. Epstein JA, Li J, Lang D, Chen F, Brown CB, Jin F, et al. Migration of cardiac neural crest cells in Splotch embryos. Development. (2000) 127:1869–78. doi: 10.1242/dev.127.9.1869
15. Person AD, Klewer SE, Runyan RB. Cell biology of cardiac cushion development. Int Rev Cytol. (2005) 243:287–335. doi: 10.1016/S0074-7696(05)43005-3
16. Gross L, Kugel MA. Topographic anatomy and histology of the valves in the human heart. Am J Pathol. (1931) 7:445–74.
17. Hinton RB Jr, Lincoln J, Deutsch GH, Osinska H, Manning PB, et al. Extracellular matrix remodeling and organization in developing and diseased aortic valves. Circ Res. (2006) 98:1431–8. doi: 10.1161/01.RES.0000224114.65109.4e
18. Markwald RR, Fitzharris TP, Manasek FJ. Structural development of endocardial cushions. Am J Anat. (1977) 148:85–119. doi: 10.1002/aja.1001480108
19. Garg V, Muth AN, Ransom JF, Schluterman MK, Barnes R, King IN, et al. Mutations in NOTCH1 cause aortic valve disease. Nature. (2005) 437:270–4. doi: 10.1038/nature03940
20. McBride KL, Riley MF, Zender GA, Fitzgerald-Butt SM, Towbin JA, Belmont JW, et al. NOTCH1 mutations in individuals with left ventricular outflow tract malformations reduce ligand-induced signaling. Hum Mol Genet. (2008) 17:2886–93. doi: 10.1093/hmg/ddn187
21. Kerstjens-Frederikse WS, van de Laar IM, Vos YJ, Verhagen JM, Berger RM, Lichtenbelt KD, et al. Cardiovascular malformations caused by NOTCH1 mutations do not keep left: data on 428 probands with left-sided CHD and their families. Genet Med. (2016) 18:914–23. doi: 10.1038/gim.2015.193
22. Greenway SC, Pereira AC, Lin JC, DePalma SR, Israel SJ, Mesquita SM, et al. De novo copy number variants identify new genes and loci in isolated sporadic tetralogy of Fallot. Nat Genet. (2009) 41:931–5. doi: 10.1038/ng.415
23. Jin SC, Homsy J, Zaidi S, Lu Q, Morton S, DePalma SR, et al. Contribution of rare inherited and de novo variants in 2,871 congenital heart disease probands. Nat Genet. (2017) 49:1593–601. doi: 10.1038/ng.3970
24. Page DJ, Miossec MJ, Williams SG, Monaghan RM, Fotiou E, Cordell HJ, et al. Whole exome sequencing reveals the major genetic contributors to nonsyndromic tetralogy of fallot. Circ Res. (2019) 124:553–63. doi: 10.1136/heartjnl-2019-BCS.136
25. Reuter MS, Jobling R, Chaturvedi RR, Manshaei R, Costain G, Heung T, et al. Haploinsufficiency of vascular endothelial growth factor related signaling genes is associated with tetralogy of Fallot. Genet Med. (2019) 21:1001–7. doi: 10.1038/s41436-018-0260-9
26. Zhang J, Chang JY, Huang Y, Lin X, Luo Y, Schwartz RJ, et al. The FGF-BMP signaling axis regulates outflow tract valve primordium formation by promoting cushion neural crest cell differentiation. Circ Res. (2010) 107:1209–19. doi: 10.1161/CIRCRESAHA.110.225318
27. Zhang J, Lin Y, Zhang Y, Lan Y, Lin C, Moon AM, et al. Frs2alpha-deficiency in cardiac progenitors disrupts a subset of FGF signals required for outflow tract morphogenesis. Development. (2008) 135:3611–22. doi: 10.1242/dev.025361
28. Park EJ, Watanabe Y, Smyth G, Miyagawa-Tomita S, Meyers E, Klingensmith J, et al. An FGF autocrine loop initiated in second heart field mesoderm regulates morphogenesis at the arterial pole of the heart. Development. (2008) 135:3599–610. doi: 10.1242/dev.025437
29. Delot EC, Bahamonde ME, Zhao M, Lyons KM. BMP signaling is required for septation of the outflow tract of the mammalian heart. Development. (2003) 130:209–20. doi: 10.1242/dev.00181
30. McCulley DJ, Kang JO, Martin JF, Black BL. BMP4 is required in the anterior heart field and its derivatives for endocardial cushion remodeling, outflow tract septation, and semilunar valve development. Dev Dyn. (2008) 237:3200–9. doi: 10.1002/dvdy.21743
31. Mommersteeg MT, Yeh ML, Parnavelas JG, Andrews WD. Disrupted Slit-Robo signalling results in membranous ventricular septum defects and bicuspid aortic valves. Cardiovasc Res. (2015) 106:55–66. doi: 10.1093/cvr/cvv040
32. Luo Y, High FA, Epstein JA, Radice GL. N-cadherin is required for neural crest remodeling of the cardiac outflow tract. Dev Biol. (2006) 299:517–28. doi: 10.1016/j.ydbio.2006.09.003
33. Taneyhill LA. To adhere or not to adhere: the role of Cadherins in neural crest development. Cell Adh Migr. (2008) 2:223–30. doi: 10.4161/cam.2.4.6835
34. Hurlstone AF, Haramis AP, Wienholds E, Begthel H, Korving J, Van Eeden F, et al. The Wnt/beta-catenin pathway regulates cardiac valve formation. Nature. (2003) 425:633–7. doi: 10.1038/nature02028
35. Abu-Issa R, Smyth G, Smoak I, Yamamura K, Meyers EN. Fgf8 is required for pharyngeal arch and cardiovascular development in the mouse. Development. (2002) 129:4613–25. doi: 10.1242/dev.129.19.4613
36. Jia Q, McDill BW, Li SZ, Deng C, Chang CP, Chen F. Smad signaling in the neural crest regulates cardiac outflow tract remodeling through cell autonomous and non-cell autonomous effects. Dev Biol. (2007) 311:172–84. doi: 10.1016/j.ydbio.2007.08.044
37. Bosse K, Hans CP, Zhao N, Koenig SN, Huang N, Guggilam A, et al. Corrigendum to “Endothelial nitric oxide signaling regulates Notch1 in aortic valve disease” [J. Mol. Cell. Cardiol. 60. (2013) 27-35]. J Mol Cell Cardiol. (2018) 121:307. doi: 10.1016/j.yjmcc.2018.04.013
38. Koenig SN, Bosse K, Majumdar U, Bonachea EM, Radtke F, Garg V. Endothelial notch1 is required for proper development of the semilunar valves and cardiac outflow tract. J Am Heart Assoc. (2016) 5:3075. doi: 10.1161/JAHA.115.003075
39. Conlon RA, Reaume AG, Rossant J. Notch1 is required for the coordinate segmentation of somites. Development. (1995) 121:1533–45. doi: 10.1242/dev.121.5.1533
40. Shesely EG, Maeda N, Kim HS, Desai KM, Krege JH, Laubach VE, et al. Elevated blood pressures in mice lacking endothelial nitric oxide synthase. Proc Natl Acad Sci USA. (1996) 93:13176–81. doi: 10.1073/pnas.93.23.13176
41. LaHaye S, Majumdar U, Yasuhara J, Koenig SN, Matos-Nieves A, Kumar R, et al. Developmental origins for semilunar valve stenosis identified in mice harboring congenital heart disease-associated GATA4 mutation. Dis Model Mech. (2019) 12:36764. doi: 10.1242/dmm.036764
42. Kim D, Pertea G, Trapnell C, Pimentel H, Kelley R, Salzberg SL. TopHat2: accurate alignment of transcriptomes in the presence of insertions, deletions and gene fusions. Genome Biol. (2013) 14:R36. doi: 10.1186/gb-2013-14-4-r36
43. Lawrence M, Huber W, Pagès H, Aboyoun P, Carlson M, Gentleman R, et al. Software for computing and annotating genomic ranges. PLoS Comput Biol. (2013) 9:e1003118. doi: 10.1371/journal.pcbi.1003118
44. Love MI, Huber W, Anders S. Moderated estimation of fold change and dispersion for RNA-seq data with DESeq2. Genome Biol. (2014) 15:550. doi: 10.1186/s13059-014-0550-8
45. Huang da W, Sherman BT, Lempicki RA. Systematic and integrative analysis of large gene lists using DAVID bioinformatics resources. Nat Protoc. (2009) 4:44–57. doi: 10.1038/nprot.2008.211
46. Huang da W, Sherman BT, Lempicki RA. Bioinformatics enrichment tools: paths toward the comprehensive functional analysis of large gene lists. Nucleic Acids Res. (2009) 37:1–13. doi: 10.1093/nar/gkn923
47. Walter W, Sánchez-Cabo F, Ricote M. GOplot: an R package for visually combining expression data with functional analysis. Bioinformatics. (2015) 31:2912–4. doi: 10.1093/bioinformatics/btv300
48. Manivannan S, Garg V. Natian and Ryabhatta-graphical user interfaces to create, analyze and visualize single-cell transcriptomic datasets. bioRxiv. (2021) 2021.06.17.448424. doi: 10.1101/2021.06.17.448424
49. Herrero J, Muffato M, Beal K, Fitzgerald S, Gordon L, Pignatelli M, et al. Ensembl comparative genomics resources. Database (Oxford). (2016) 2016:baw053. doi: 10.1093/database/baw053
50. Ware JS, Samocha KE, Homsy J, Daly MJ. Interpreting de novo variation in human disease using denovolyzer. Curr Protoc Hum Genet. (2015) 87:7.25.1–15. doi: 10.1002/0471142905.hg0725s87
51. Li Y, Hibbs MA, Gard AL, Shylo NA, Yun K. Genome-wide analysis of N1ICD/RBPJ targets in vivo reveals direct transcriptional regulation of Wnt, SHH, and hippo pathway effectors by Notch1. Stem Cells. (2012) 30:741–52. doi: 10.1002/stem.1030
52. Ma M, Li P, Shen H, Estrada KD, Xu J, Kumar SR, et al. Dysregulated endocardial TGFbeta signaling and mesenchymal transformation result in heart outflow tract septation failure. Dev Biol. (2016) 409:272–6. doi: 10.1016/j.ydbio.2015.09.021
53. Heallen T, Zhang M, Wang J, Bonilla-Claudio M, Klysik E, Johnson RL, et al. Hippo pathway inhibits Wnt signaling to restrain cardiomyocyte proliferation and heart size. Science. (2011) 332:458–61. doi: 10.1126/science.1199010
54. Hulin A, Moore V, James JM, Yutzey KE. Loss of Axin2 results in impaired heart valve maturation and subsequent myxomatous valve disease. Cardiovasc Res. (2017) 113:40–51. doi: 10.1093/cvr/cvw229
55. Liu X, Yagi H, Saeed S, Bais AS, Gabriel GC, Chen Z, et al. The complex genetics of hypoplastic left heart syndrome. Nat Genet. (2017) 49:1152–9. doi: 10.1038/ng.3870
56. de Soysa TY, Ranade SS, Okawa S, Ravichandran S, Huang Y, Salunga HT, et al. Single-cell analysis of cardiogenesis reveals basis for organ-level developmental defects. Nature. (2019) 572:120–4. doi: 10.1038/s41586-019-1414-x
57. Liu X, Chen W, Li W, Li Y, Priest JR, Zhou B, et al. Single-Cell RNA-Seq of the developing cardiac outflow tract reveals convergent development of the vascular smooth muscle cells. Cell Rep. (2019) 28:1346–61. doi: 10.1016/j.celrep.2019.06.092
58. Xiao Y, Hill MC, Zhang M, Martin TJ, Morikawa Y, Wang S, et al. Hippo signaling plays an essential role in cell state transitions during cardiac fibroblast development. Dev Cell. (2018) 45:153–69. doi: 10.1016/j.devcel.2018.03.019
59. Stankunas K, Shang C, Twu KY, Kao SC, Jenkins NA, Copeland NG, et al. Pbx/Meis deficiencies demonstrate multigenetic origins of congenital heart disease. Circ Res. (2008) 103:702–9. doi: 10.1161/CIRCRESAHA.108.175489
60. Pierpont ME, Brueckner M, Chung WK, Garg V, Lacro RV, McGuire AL, et al. Genetic basis for congenital heart disease: revisited: a scientific statement from the American Heart Association. Circulation. (2018) 138:e653–e711. doi: 10.1161/CIR.0000000000000631
61. Ellis JM, Mentock SM, Depetrillo MA, Koves TR, Sen S, Watkins SM, et al. Mouse cardiac acyl coenzyme a synthetase 1 deficiency impairs Fatty Acid oxidation and induces cardiac hypertrophy. Mol Cell Biol. (2011) 31:1252–62. doi: 10.1128/MCB.01085-10
62. Clark H, Carling D, Saggerson D. Covalent activation of heart AMP-activated protein kinase in response to physiological concentrations of long-chain fatty acids. Eur J Biochem. (2004) 271:2215–24. doi: 10.1111/j.1432-1033.2004.04151.x
63. Davis J, Davis LC, Correll RN, Makarewich CA, Schwanekamp JA, Moussavi-Harami F, et al. A tension-based model distinguishes hypertrophic versus dilated cardiomyopathy. Cell. (2016) 165:1147–59. doi: 10.1016/j.cell.2016.04.002
64. Skarnes WC, Rosen B, West AP, Koutsourakis M, Bushell W, Iyer V, et al. A conditional knockout resource for the genome-wide study of mouse gene function. Nature. (2011) 474:337–42. doi: 10.1038/nature10163
65. Koizumi H, Tanaka T, Gleeson JG. Doublecortin-like kinase functions with doublecortin to mediate fiber tract decussation and neuronal migration. Neuron. (2006) 49:55–66. doi: 10.1016/j.neuron.2005.10.040
66. Labrador JP, Azcoitia V, Tuckermann J, Lin C, Olaso E, Mañes S, et al. The collagen receptor DDR2 regulates proliferation and its elimination leads to dwarfism. EMBO Rep. (2001) 2:446–52. doi: 10.1093/embo-reports/kve094
67. Saburi S, Hester I, Fischer E, Pontoglio M, Eremina V, Gessler M, et al. Loss of Fat4 disrupts PCP signaling and oriented cell division and leads to cystic kidney disease. Nat Genet. (2008) 40:1010–5. doi: 10.1038/ng.179
68. Arteaga-Solis E, Gayraud B, Lee SY, Shum L, Sakai L, Ramirez F. Regulation of limb patterning by extracellular microfibrils. J Cell Biol. (2001) 154:275–81. doi: 10.1083/jcb.200105046
69. Ding J, Allen E, Wang W, Valle A, Wu C, Nardine T, et al. Gene targeting of GAN in mouse causes a toxic accumulation of microtubule-associated protein 8 and impaired retrograde axonal transport. Hum Mol Genet. (2006) 15:1451–63. doi: 10.1093/hmg/ddl069
70. Hsu A, Duan Q, McMahon S, Huang Y, Wood SA, Gray NS, et al. Salt-inducible kinase 1 maintains HDAC7 stability to promote pathologic cardiac remodeling. J Clin Invest. (2020) 130:2966–77. doi: 10.1172/JCI133753
71. Chang S, Young BD, Li S, Qi X, Richardson JA, Olson EN. Histone deacetylase 7 maintains vascular integrity by repressing matrix metalloproteinase 10. Cell. (2006) 126:321–34. doi: 10.1016/j.cell.2006.05.040
72. Xu J, Krebs LT, Gridley T. Generation of mice with a conditional null allele of the Jagged2 gene. Genesis. (2010) 48:390–3. doi: 10.1002/dvg.20626
73. Nakajima K, Yin X, Takei Y, Seog DH, Homma N, Hirokawa N. Molecular motor KIF5A is essential for GABA(A) receptor transport, and KIF5A deletion causes epilepsy. Neuron. (2012) 76:945–61. doi: 10.1016/j.neuron.2012.10.012
74. Iwanaga A, Sato T, Sugihara K, Hirao A, Takakura N, Okamoto H, et al. Neural-specific ablation of the scaffold protein JSAP1 in mice causes neonatal death. Neurosci Lett. (2007) 429:43–8. doi: 10.1016/j.neulet.2007.09.057
75. Grueter CE, van Rooij E, Johnson BA, DeLeon SM, Sutherland LB, Qi X, et al. A cardiac microRNA governs systemic energy homeostasis by regulation of MED13. Cell. (2012) 149:671–83. doi: 10.1016/j.cell.2012.03.029
76. Rangrez AY, Pott J, Kluge A, Frauen R, Stiebeling K, Hoppe P, et al. Myeloid leukemia factor-1 is a novel modulator of neonatal rat cardiomyocyte proliferation. Biochim Biophys Acta Mol Cell Res. (2017) 1864:634–44. doi: 10.1016/j.bbamcr.2017.01.004
77. Jones WK, Grupp IL, Doetschman T, Grupp G, Osinska H, Hewett TE, et al. Ablation of the murine alpha myosin heavy chain gene leads to dosage effects and functional deficits in the heart. J Clin Invest. (1996) 98:1906–17. doi: 10.1172/JCI118992
78. Grossmann KS, Grund C, Huelsken J, Behrend M, Erdmann B, Franke WW, et al. Requirement of plakophilin 2 for heart morphogenesis and cardiac junction formation. J Cell Biol. (2004) 167:149–60. doi: 10.1083/jcb.200402096
79. Behar O, Golden JA, Mashimo H, Schoen FJ, Fishman MC. Semaphorin III is needed for normal patterning and growth of nerves, bones and heart. Nature. (1996) 383:525–8. doi: 10.1038/383525a0
80. Galvin KM, Donovan MJ, Lynch CA, Meyer RI, Paul RJ, Lorenz JN, et al. A role for smad6 in development and homeostasis of the cardiovascular system. Nat Genet. (2000) 24:171–4. doi: 10.1038/72835
81. Wylie LA, Mouillesseaux KP, Chong DC, Bautch VL. Developmental SMAD6 loss leads to blood vessel hemorrhage and disrupted endothelial cell junctions. Dev Biol. (2018) 442:199–209. doi: 10.1016/j.ydbio.2018.07.027
82. Carver EA, Jiang R, Lan Y, Oram KF, Gridley T. The mouse snail gene encodes a key regulator of the epithelial-mesenchymal transition. Mol Cell Biol. (2001) 21:8184–8. doi: 10.1128/MCB.21.23.8184-8188.2001
83. Kyriakides TR, Zhu YH, Smith LT, Bain SD, Yang Z, Lin MT, et al. Mice that lack thrombospondin 2 display connective tissue abnormalities that are associated with disordered collagen fibrillogenesis, an increased vascular density, and a bleeding diathesis. J Cell Biol. (1998) 140:419–30. doi: 10.1083/jcb.140.2.419
84. Witt CC, Witt SH, Lerche S, Labeit D, Back W, Labeit S. Cooperative control of striated muscle mass and metabolism by MuRF1 and MuRF2. Embo J. (2008) 27:350–60. doi: 10.1038/sj.emboj.7601952
85. Mjaatvedt CH, Yamamura H, Capehart AA, Turner D, Markwald RR. The Cspg2 gene, disrupted in the hdf mutant, is required for right cardiac chamber and endocardial cushion formation. Dev Biol. (1998) 202:56–66. doi: 10.1006/dbio.1998.9001
86. D'Amato G, Luxan G, del Monte-Nieto G, Martinez-Poveda B, Torroja C, Walter W, et al. Sequential Notch activation regulates ventricular chamber development. Nat Cell Biol. (2016) 18:7–20. doi: 10.1038/ncb3280
87. Ieda M, Kanazawa H, Kimura K, Hattori F, Ieda Y, Taniguchi M, et al. Sema3a maintains normal heart rhythm through sympathetic innervation patterning. Nat Med. (2007) 13:604–12. doi: 10.1038/nm1570
88. Ragni CV, Diguet N, Le Garrec JF, Novotova M, Resende TP, Pop S, et al. Amotl1 mediates sequestration of the Hippo effector Yap1 downstream of Fat4 to restrict heart growth. Nat Commun. (2017) 8:14582. doi: 10.1038/ncomms14582
89. Cowling RT, Yeo SJ, Kim IJ, Park JI, Gu Y, Dalton ND, et al. Discoidin domain receptor 2 germline gene deletion leads to altered heart structure and function in the mouse. Am J Physiol Heart Circ Physiol. (2014) 307:H773–81. doi: 10.1152/ajpheart.00142.2014
90. Huang XF, Xiang L, Fang XL, Liu WQ, Zhuang YY, Chen ZJ, et al. Functional characterization of CEP250 variant identified in nonsyndromic retinitis pigmentosa. Hum Mutat. (2019) 40:1039–45. doi: 10.1002/humu.23759
91. Wickman K, Nemec J, Gendler SJ, Clapham DE. Abnormal heart rate regulation in GIRK4 knockout mice. Neuron. (1998) 20:103–14. doi: 10.1016/S0896-6273(00)80438-9
92. Herz J, Clouthier DE, Hammer RE. LDL receptor-related protein internalizes and degrades uPA-PAI-1 complexes and is essential for embryo implantation. Cell. (1992) 71:411–21. doi: 10.1016/0092-8674(92)90511-A
93. Morano I, Chai GX, Baltas LG, Lamounier-Zepter V, Lutsch G, Kott M, et al. Smooth-muscle contraction without smooth-muscle myosin. Nat Cell Biol. (2000) 2:371–5. doi: 10.1038/35014065
94. Doherty KR, Cave A, Davis DB, Delmonte AJ, Posey A, Earley JU, et al. Normal myoblast fusion requires myoferlin. Development. (2005) 132:5565–75. doi: 10.1242/dev.02155
95. Schneider M, Zimmermann AG, Roberts RA, Zhang L, Swanson KV, Wen H, et al. The innate immune sensor NLRC3 attenuates Toll-like receptor signaling via modification of the signaling adaptor TRAF6 and transcription factor NF-kappaB. Nat Immunol. (2012) 13:823–31. doi: 10.1038/ni.2378
96. McCright B, Lozier J, Gridley T. Generation of new Notch2 mutant alleles. Genesis. (2006) 44:29–33. doi: 10.1002/gene.20181
97. Bergmann C, Fliegauf M, Bruchle NO, Frank V, Olbrich H, Kirschner J, et al. Loss of nephrocystin-3 function can cause embryonic lethality, Meckel-Gruber-like syndrome, situs inversus, and renal-hepatic-pancreatic dysplasia. Am J Hum Genet. (2008) 82:959–70. doi: 10.1016/j.ajhg.2008.02.017
98. Szondy Z, Mastroberardino PG, Varadi J, Farrace MG, Nagy N, Bak I, et al. Tissue transglutaminase (TG2) protects cardiomyocytes against ischemia/reperfusion injury by regulating ATP synthesis. Cell Death Differ. (2006) 13:1827–9. doi: 10.1038/sj.cdd.4401889
99. De Laurenzi V, Melino G. Gene disruption of tissue transglutaminase. Mol Cell Biol. (2001) 21:148–55. doi: 10.1128/MCB.21.1.148-155.2001
100. Shan W, Li J, Xu W, Li H, Zuo Z. Critical role of UQCRC1 in embryo survival, brain ischemic tolerance and normal cognition in mice. Cell Mol Life Sci. (2019) 76:1381–96. doi: 10.1007/s00018-019-03007-6
101. Takeshima H, Komazaki S, Hirose K, Nishi M, Noda T, Iino M. Embryonic lethality and abnormal cardiac myocytes in mice lacking ryanodine receptor type 2. Embo J. (1998) 17:3309–16. doi: 10.1093/emboj/17.12.3309
102. Loaiza R, Benkusky NA, Powers PP, Hacker T, Noujaim S, Ackerman MJ, et al. Heterogeneity of ryanodine receptor dysfunction in a mouse model of catecholaminergic polymorphic ventricular tachycardia. Circ Res. (2013) 112:298–308. doi: 10.1161/CIRCRESAHA.112.274803
103. Liu Y, Wang R, Sun B, Mi T, Zhang J, Mu Y, et al. Generation and characterization of a mouse model harboring the exon-3 deletion in the cardiac ryanodine receptor. PLoS ONE. (2014) 9:e95615. doi: 10.1371/journal.pone.0095615
104. Lu X, Le Noble F, Yuan L, Jiang Q, De Lafarge B, Sugiyama D, et al. The netrin receptor UNC5B mediates guidance events controlling morphogenesis of the vascular system. Nature. (2004) 432:179–86. doi: 10.1038/nature03080
105. Homsy J, Zaidi S, Shen Y, Ware JS, Samocha KE, Karczewski KJ, et al. De novo mutations in congenital heart disease with neurodevelopmental and other congenital anomalies. Science. (2015) 350:1262–6. doi: 10.1126/science.aac9396
106. Gifford CA, Ranade SS, Samarakoon R, Salunga HT, de Soysa TY, Huang Y, et al. Oligogenic inheritance of a human heart disease involving a genetic modifier. Science. (2019) 364:865–70. doi: 10.1126/science.aat5056
Keywords: heart development, congenital heart disease, mouse model, tetralogy of Fallot, human genetics
Citation: Matos-Nieves A, Manivannan S, Majumdar U, McBride KL, White P and Garg V (2021) A Multi-Omics Approach Using a Mouse Model of Cardiac Malformations for Prioritization of Human Congenital Heart Disease Contributing Genes. Front. Cardiovasc. Med. 8:683074. doi: 10.3389/fcvm.2021.683074
Received: 19 March 2021; Accepted: 22 July 2021;
Published: 24 August 2021.
Edited by:
Christoph Dieterich, Heidelberg University, GermanyReviewed by:
José Luis De La Pompa, Spanish National Center for Cardiovascular Research, SpainMiguel A. Aon, National Institute on Aging, National Institutes of Health (NIH), United States
Tobias Jakobi, University of Arizona, United States
Copyright © 2021 Matos-Nieves, Manivannan, Majumdar, McBride, White and Garg. This is an open-access article distributed under the terms of the Creative Commons Attribution License (CC BY). The use, distribution or reproduction in other forums is permitted, provided the original author(s) and the copyright owner(s) are credited and that the original publication in this journal is cited, in accordance with accepted academic practice. No use, distribution or reproduction is permitted which does not comply with these terms.
*Correspondence: Vidu Garg, vidu.garg@nationwidechildrens.org
†These authors share first authorship