- 1Department of Cardiovascular and Metabolic Health, Norwich Medical School, University of East Anglia, Norwich, United Kingdom
- 2Department of Cardiology, Norfolk and Norwich University Hospital, Norwich, United Kingdom
- 3Department of Infection, Immunity and Cardiovascular Disease, University of Sheffield, Sheffield, United Kingdom
- 4Multidisciplinary Cardiovascular Research Centre & Division of Biomedical Imaging, Leeds Institute of Cardiovascular and Metabolic Medicine, University of Leeds, Leeds, United Kingdom
- 5Bristol Heart Institute and Translational Biomedical Research Centre, Faculty of Health Science, University of Bristol, Bristol, United Kingdom
- 6Cardiothoracic Imaging Unit, Hospital San Juan De Dios, Ressalta, HT Medica, Córdoba, Spain
Cardiovascular magnetic resonance (CMR) imaging is a versatile tool that has established itself as the reference method for functional assessment and tissue characterisation. CMR helps to diagnose, monitor disease course and sub-phenotype disease states. Several emerging CMR methods have the potential to offer a personalised medicine approach to treatment. CMR tissue characterisation is used to assess myocardial oedema, inflammation or thrombus in various disease conditions. CMR derived scar maps have the potential to inform ablation therapy—both in atrial and ventricular arrhythmias. Quantitative CMR is pushing boundaries with motion corrections in tissue characterisation and first-pass perfusion. Advanced tissue characterisation by imaging the myocardial fibre orientation using diffusion tensor imaging (DTI), has also demonstrated novel insights in patients with cardiomyopathies. Enhanced flow assessment using four-dimensional flow (4D flow) CMR, where time is the fourth dimension, allows quantification of transvalvular flow to a high degree of accuracy for all four-valves within the same cardiac cycle. This review discusses these emerging methods and others in detail and gives the reader a foresight of how CMR will evolve into a powerful clinical tool in offering a precision medicine approach to treatment, diagnosis, and detection of disease.
Introduction
Non-invasive imaging plays a fundamental role in the assessment of cardiovascular disease. Cardiovascular magnetic resonance (CMR) is now considered the gold standard imaging technique for the assessment of myocardial anatomy, regional and global function, and viability. More recently, novel methods of CMR are pushing the boundaries of diagnosis and allowing CMR to guide treatment whilst also further sub-phenotyping cardiovascular diseases.
Myocardial tissue characterisation has undoubtedly been proven to be an invaluable tool for clinicians as it often provides the diagnosis, therefore enabling the most appropriate treatment option. Established techniques, including native T1-mapping, extracellular volume (ECV) quantification, and T2-mapping, are used in clinical practise routinely not only to differentiate cardiomyopathies but also to provide information on the extent of myocardial disease as evidenced by oedema and/or fibrosis encountered in several disease processes that affect the myocardium. CMR also allows individualised planning of invasive management strategies against arrhythmias. More specifically, localisation of the scar tissue can provide invaluable information for targeted ablation treatment strategies.
More advanced techniques, such as quantitative perfusion CMR and diffusion tensor imaging (DTI), have shown promising advancements that further support the transition of their use from research to the clinical practise. Moreover, novel methods such as the four-dimensional (4D) flow CMR have revolutionised the field as it allows direct evaluation of the flow in all three directions. The information provided by 4D flow can be vital in the assessment of conditions such as complex valvular lesions or congenital heart diseases, in which the haemodynamic patterns and effects have been difficult to visualise with other imaging modalities.
CMR therefore plays an important role in several aspects of clinical practise having significant impact on the diagnostic, prognostic and treatment pathways in patient care. In this comprehensive review, we first discuss established methods of CMR and then describe important emerging methods which have the potential for clinical impact and are likely to influence the future role of CMR.
Tissue Characterisation
CMR is now established for myocardial tissue characterisation and allows the assessment of myocardial oedema, microvascular obstruction, thrombus, and scar (1). Clinical use includes—T2-weighted STIR (short tau inversion recovery) imaging for myocardial oedema and intra-myocardial haemorrhage post-acute myocardial infarction (MI), early gadolinium enhancement (EGE) for thrombus and microvascular obstruction and late gadolinium enhancement (LGE) for the myocardial scar. The pattern of the myocardial scar on LGE can sub-phenotype the disease process (2). In addition, a combination of the aforementioned methods can further differentiate acute from chronic MI (3), cause of heart failure (HF) or cardiomyopathy (4, 5). Importantly, visual identification of LGE has been associated with worse prognosis across a wide series of pathologies, including aortic stenosis, cardiomyopathies, and congenital heart disease (6–8). Furthermore, myocardial and liver iron content quantification by T2* magnetic resonance has been well-established in the management of conditions with iron-overload and particularly in the serial follow-up of patients with thalassaemia (9). These standard methods have some limitations—they are predominantly non-quantitative methods, which might limit their standardisation and utilisation as endpoints in clinical trials. Whilst quantification of LGE is being investigated in the research arena, and higher LGE mass has been associated with worse prognosis, this is not being routinely utilised (10, 11). Also, these are two-dimensional acquisitions which limit full left ventricular (LV) coverage for either diagnosis or therapeutic reasons, for example, in ventricular tachycardia (VT) ablation (12). Here we describe emerging CMR tissue characterisation methods that have the potential for improving diagnosis, informing treatment, and outcomes.
Scar Mapping by LGE Imaging for Arrhythmic Ablation Therapy
The presence of myocardial scar and its characteristics define the arrhythmogenic substrate (13, 14). In particular, the partially infarcted myocardial tissue, named the grey zone, has been shown to be an important predictor of adverse cardiac events, outperforming traditional functional parameters such as left ventricular ejection fraction (15, 16). More recently, the deep channels, islets of alive myocytes sandwiched between two discrete infarcted regions have been identified as areas of late potential, which can be targeted for ablation (Figure 1) (17). Translational studies have already demonstrated that the arrhythmogenic deep channel ablation, identified by using LGE imaging, can reduce arrhythmic burden and recurrence over electrical mapping techniques. In addition, scar localisation (epicardial vs. subendocardial) allows planning for either an endocardial or epicardial approach for ablation. For example, for ablation of interventricular septum ventricular tachycardia, epicardial access is typically not required. This has the huge potential to save time in the catheter laboratories, reduce patient risk and discomfort and overall demonstrate promising outcome results. To map the core scar, grey zone, and the deep channels, a three-dimensional (3D) coverage of the left ventricle or the atrium is necessary. A proposed solution is to acquire short-axis and long-axis stacks of the region of interest and generate a high-resolution 3D stack using this (18). With the emergence of novel acceleration methods, including compressed sensing, it is now possible to do 3D LGE imaging with full coverage of the LV or left atrium (LA) for informing ablation therapy. CMR-aided scar de-channelling results in a lower need for radio-frequency delivery, higher rates of non-inducibility after substrate ablation, and VT-recurrence-free survival (17). However, integrated software solutions are needed to push this emerging method into routine clinical practice.
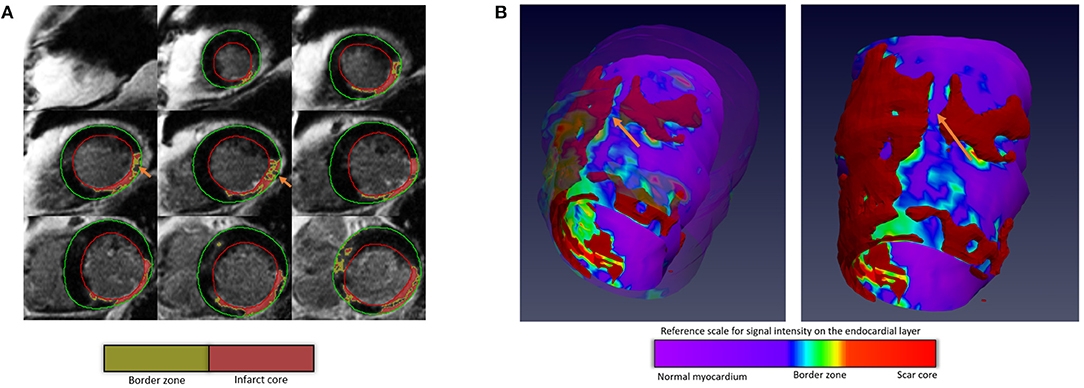
Figure 1. Scar mapping. (A) Short-axis, two-dimensional segmentation of scar and border zone. (B) These are high-resolution signal intensity maps generated using two 2D LGE acquisitions. Full-width half max threshold of 60% has been applied to identify the infarct core—the red areas. The orange arrow demonstrates the presence of deep channel with late action potential which was ablated for this patient.
Quantitative Tissue Characterisation
In the last 5-years, several CMR relaxometry-based quantitative techniques have translated into routine clinical practice to inform diagnosis and guide treatment (19, 20). The most common ones are native T1-mapping, extracellular volume (ECV) quantification using native/post-contrast T1-mapping methods, T2-mapping, and T2*-mapping. These techniques are now established to sub-phenotype cardiomyopathies and differentiate the aetiology of myocardial infarction with non-obstructive coronary arteries (MINOCA) in an acute setting (Figure 2) (21). A case example of Takotsubo cardiomyopathy diagnosed acutely on multi-parametric CMR is demonstrated in Figure 3. In addition, native-T1 and ECV mapping allow quantifying infarct size, area at risk, and myocardial salvageable index (MSI) in an acute infarct setting (22, 23). In patients who have contraindications for gadolinium-based contrast agents, native-T1 allows making some degree of infarct assessment; however, as it is less specific, it is not always possible to infer infarct using this method alone. Diagnostic strengths of native T1 vs. ECV are shown in Figure 4.
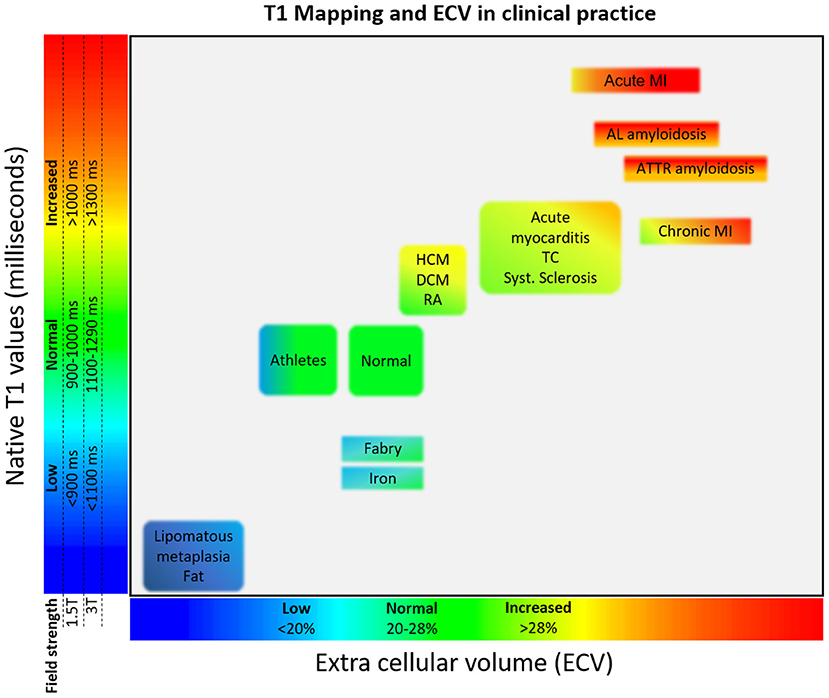
Figure 2. Tissue characterisation using native T1 and ECV. Absolute values for native T1 depend greatly on field strength (1.5 or 3 T), pulse sequence (MOLLI or ShMOLLI), scanner manufacturer and rules of measurements. For the purpose of comparability, only studies using MOLLI sequences were considered in this figure. AL, amyloid light chain; ATTR, amyloid transthyretin; DCM, dilated cardiomyopathy; ECV, extracellular volume; HCM, hypertrophic cardiomyopathy; MI, myocardial infarction; RA, rheumatoid arthritis; TC, takotsubo cardiomyopathy.
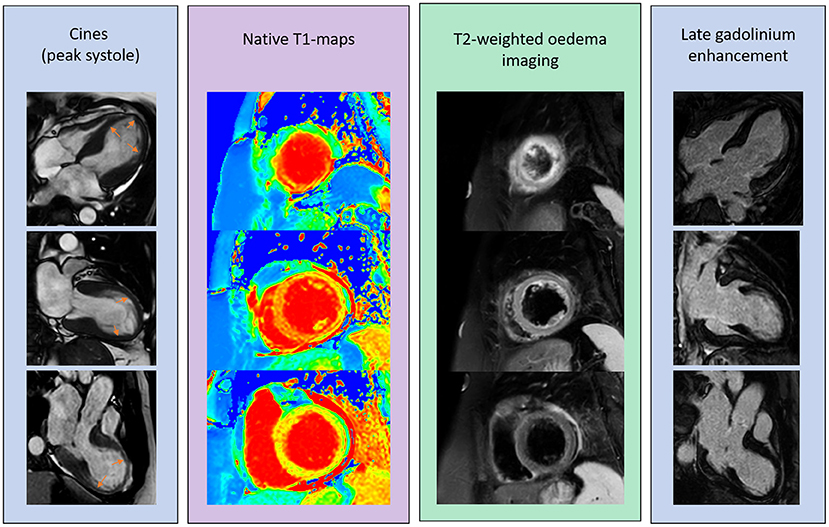
Figure 3. Multi-parametric tissue characterisation of the myocardium to aid diagnosis. This is a case of a 57 year old lady who presents with acute chest pain, ST elevation, and raised troponin biomarkers. Her coronary angiogram was normal. An acute CMR revealed apical ballooning demonstrated in the 4CH view by orange arrows, rise in myocardial oedema on T2-weigthed imaging and confirmed on quantitative native T1-maps (white arrows). There was no evidence of scar on late gadolinium enhancement imaging and overall the extracellular volume was relatively not high as T1-values. This is suggestive of more intra-cellular swelling than extracellular expansion in an acute setting.
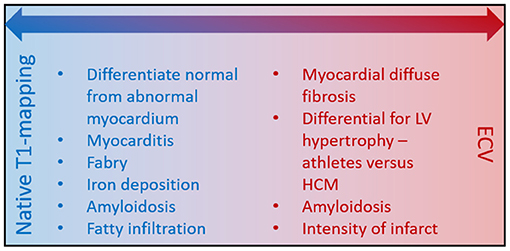
Figure 4. Diagnostic complementary utility of native T1-mapping vs. ECV. The main advantage of ECV is that it takes gadolinium flow kinetics into consideration to improve the precision of diagnosis.
In recent years, the focus of the assessment and risk stratification of patients with valvular lesions has shifted towards the myocardium. For example, in the case of aortic stenosis, there remains a major uncertainty regarding the timing of intervention in asymptomatic patients. Myocardial fibrosis in aortic stenosis is mainly a result of chronically elevated left ventricular afterload, which is associated with several pathological changes, including cellular hypertrophy, expansion of the extracellular matrix (or volume), and ischaemia due to demand-supply mismatch (24). Several studies have shown that once replacement fibrosis ensues, it progresses rapidly and is unlikely to improve following aortic valve replacement (25, 26), diffuse fibrosis indexed for the left ventricular volume measured by the indexed ECV (iECV) regress after aortic valve intervention (25, 26). Following correction of the afterload issue by valve intervention, the left ventricular mass decreases with regression of both cellular and extracellular mass, but cellular mass regresses more, hence resulting in relative increase in ECV% as the ratio of matrix to total mass is increased. However, the iECV decreases as it represents the extracellular matrix as a total volume, rather than a percentage which is therefore in keeping with the potential for reversal of diffuse fibrosis. Clinical trials, including the EVOLVED trial, are investigating the role of myocardial fibrosis assessment as a trigger for intervention (27). Quantitative tools (T1-mapping and ECV) for mapping diffuse fibrosis are of particular interest in aortic stenosis. There is some evidence that myocardial fibrosis mapped using these tools is associated with histology in aortic stenosis (28, 29); they can detect ventricular decompensation (24), further risk stratification, and predict adverse outcomes offering incremental value to LGE (30, 31).
In the case of the mitral valve, the role of diffuse myocardial fibrosis assessment by mapping techniques is less established. A study by Edward et al. (32) demonstrated the ECV in patients with asymptomatic primary mitral regurgitation to be raised. In addition, ECV was associated with two previously defined important prognosis markers—end-systolic volume and left atrial volume, and with peak VO2 max (r-0.51, P < 0.05). Furthermore, the impact of diffuse fibrosis on patients symptoms and ventricular response following surgery is being addressed by the FINDER study (33).
Quantitative Myocardial Perfusion
Vasodilator stress perfusion CMR imaging has evolved into a recognised form of assessment for patients with known or suspected cardiac chest pain for the identification of myocardial ischaemia. Visual assessment involves identification of delayed first-pass wash-in of gadolinium-based contrast agent, from epicardial to endocardial layers in one or more myocardial segments. By comparison with single photon emission computed tomography (SPECT), perfusion CMR allows better assessment of transmural perfusion due to its high spatial resolution and has been shown to be superior in the evaluation of left main stenosis (34) and in assessment of women with suspected ischaemia (35).
First-pass perfusion images are usually acquired by a dynamic T1-weighted sequence; this is meant to generate a contrast between zones of stress-induced hyperaemic myocardium and zones of relatively reduced perfusion, based on the distinct speed of inflow of blood and gadolinium over time. Typically stress images are performed under vasodilator (adenosine, dipyridamole, or regadenoson) stress followed by rest images, which comprise the same sequence without the vasodilator effect, usually as three short axis (basal, mid, and apex) slices. Automated inline quantification enables the user to obtain myocardial blood flow (MBF) at stress and rest. Using the values of stress and rest MBF, a ratio known as the myocardial perfusion can be obtained.
Despite the more widespread availability of CMR in recent years, quantitative perfusion CMR has remained largely a research tool, and perfusion CMR is assessed visually in the clinical setting. Clinical perfusion CMR compares well to invasive angiography (36, 37), fractional flow reserve (FFR) (38–42), and single positron emission computed tomography (SPECT) (43–45), and has demonstrated good prognostic value (46–48).
Over the past three decades, quantitative perfusion CMR has been described (47, 48), refined, and subsequently validated against FFR (40, 49), microspheres (50), and more recently against PET (51, 52). Though PET remains the non-invasive reference method for perfusion quantification, it involves the use of ionising radiation and requires an on-site cyclotron.
The aim of a quantitative approach is to enable user-independent and reproducible measurements of myocardial perfusion. This is especially pertinent in cases where perfusion abnormalities are diffuse as visual assessment can be challenging. In the case of multi-vessel disease, a quantitative approach has been shown to be superior to visual assessment (53). Similarly, in an observational registry, the use of a quantitative approach proved it could provide incremental prognostic benefit over a visual approach (54).
Lack of standardisation appears to be the limiting factor to more widespread implementation of quantitative perfusion CMR particularly in regards to acquisition and dosing protocols, analysis methods, and the availability of software for post-processing across centres. The last few years, however, have seen significant steps towards more user-friendly quantitative approaches to drive towards clinical translation. Using a dual-sequence approach, Kellman et al. have achieved inline myocardial perfusion quantification allowing fast results with minimal user interaction (Figure 5). This approach has been validated in healthy volunteers (55, 56), in patients with known or suspected coronary artery disease (CAD) against angiography (56) and in patients against PET (57). Other recent studies have explored the use of perfusion mapping against angiography (58), deep learning-based processing of perfusion data (59), and most recently, artificial intelligence quantification of perfusion mapping was shown to be a strong, independent predictor of adverse cardiovascular outcome (60).
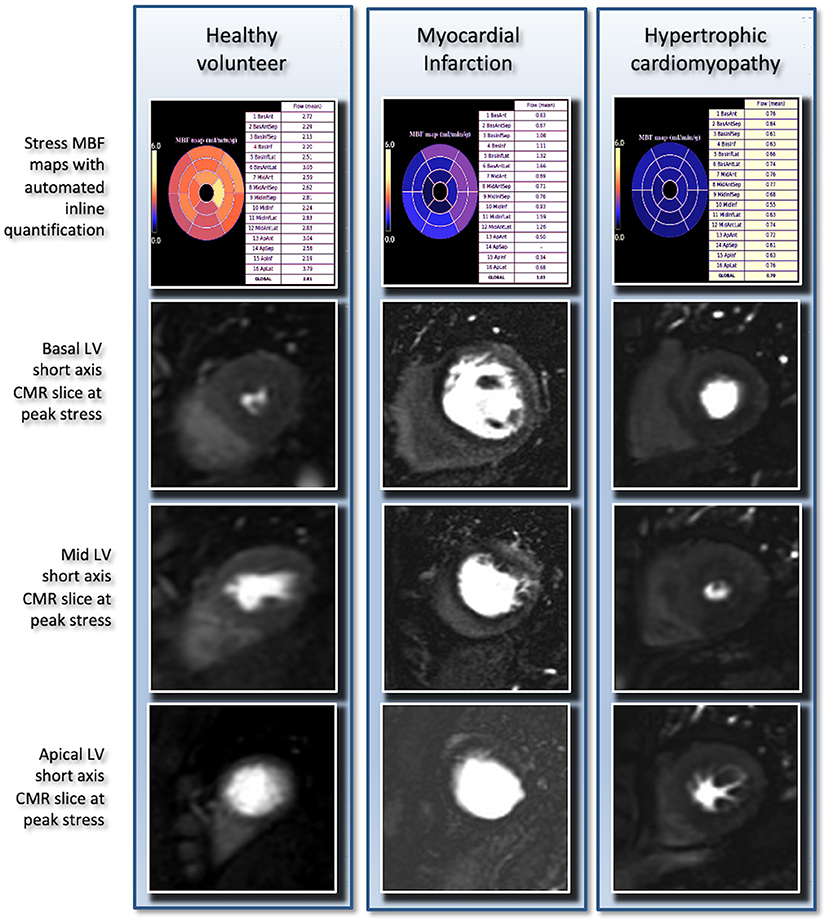
Figure 5. Stress perfusion CMR images obtained by automated in line quantification as well as the basal, mid, and apical slices that were obtained in a healthy volunteer showing no inducible ischaemia, in a patient with a myocardial infarction showing inducible ischaemia in the basal to apical septal and inferior segments and in a patient with hypertrophic cardiomyopathy demonstrating globally reduced myocardial blood flow secondary to severe microvascular disease.
Perfusion quantification is based on the indicator-dilution principle (61). A recognised amount of an indicator is injected into a fluid flowing at an unknown rate. This is through a system of unknown volume. The fluid is monitored at different points downstream from the plane of introduction and the concentration of indicator, diluted by the parent fluid, is measured as a function of time. In first-pass perfusion, the “indicator” is usually a gadolinium-based contrast agent introduced via a peripheral vein. Because the concentration of contrast at the ostium of the coronary arteries is not known, the quantity is estimated by measuring the arterial first-pass time-intensity curve (arterial input function, AIF) of the left ventricle. Finally, myocardial blood flow is observed as a function of the quantified myocardial time-intensity curve (62).
Here lies one of the main hurdles in quantification of perfusion CMR—at lower doses, the dose-signal relationship is linear. However, at higher doses, there is saturation of the gadolinium signal curves, which, if uncorrected, can lead to elevated and non-physiological perfusion estimates. As a result, the bulk of the study on quantitative perfusion research has focused on optimisation of approaches to overcome this challenge by addressing various dosing regimes, acquisition protocols, manipulation of magnetic signal, and complex mathematical processing.
Early studies simply used lower doses of gadolinium to avoid saturation effects in combination with strongly T1 weighted sequences—this overcame saturation at higher doses, but however resulted in low myocardial contrast-to-noise ratio, and poor visualisation of perfusion abnormalities (63–65).
There are currently two main approaches to obtaining perfusion-imaging data that can be both visually assessed and quantified; the dual bolus and dual sequence approaches. The dual-bolus method is a combination of a low-high dosage approach, using two consecutive injections of gadolinium contrast (66–69). In short, this is attained by using a combination of a body weight-adjusted and high-dose main bolus of contrast agent preceded by the injection of a low dose pre-bolus having the same volume of the bolus but only 10% of the concentration of gadolinium. The pre-bolus low dosage injection allows the acquisition of a non-saturated AIF. The main bolus injection produces a desired myocardial signal response for both visual and quantitative assessment. The dual-sequence method is a more recently described alternative to the dual-bolus approach, which has gained momentum in recent years (70–72). In this approach, for each cardiac cycle a low-resolution image, with a short saturation- recovery time after the R-wave is acquired to measure the AIF. This avoids any AIF saturation. Low-resolution acquisition is followed by high-resolution acquisition with longer saturation-recovery time to measure the myocardial signal with higher contrast. There are pros and cons associated with both methods; the dual-bolus protocols are feasible in the majority of centres and do not require new software or hardware, but the preparation of the scan is more complex. Conversely, the dual-sequence protocols are more straightforward to implement but widespread use is limited by availability across different scanner vendors.
Following the acquisition of suitable data by either dual bolus or dual-sequence methods, the data must then be analysed to provide stress and rest perfusion estimates and then in turn myocardial perfusion reserve. Myocardial perfusion reserve is arguably more robust as inherent errors within the mathematical modelling are cancelled out by using a ratio between stress and rest. The mathematical approaches proposed are beyond the scope of this review but all rely upon model-dependent or independent deconvolution processing of the AIF and myocardial signals to determine myocardial perfusion (63, 73–84).
Data can be analysed on both a segmental or voxel-wise basis. When segmental quantitative analysis is performed from high-resolution images, the spatial resolution of the images is only partially used, as the signal intensity is sampled transmurally. Hence, time-intensity curves from subendocardial ischaemic areas are averaged. This results in partial-volume effects and possibly reduced sensitivity. High-resolution, voxel-wise myocardial perfusion assessment offers additional information on the heterogeneity of myocardial perfusion (50, 80). This combination of absolute quantification and voxel-level resolution has the potential for calculation of the true ischaemic burden. Hence voxel perfusion maps limit partial volume effects and the influence of other factors such as the LV wall thickness, LV remodeling, and presence of previous infarction.
High-resolution, voxel-wise perfusion is therefore likely to be the more robust method for assessment of ischaemic burden, especially when coupled with full LV coverage enabled by newer 3D perfusion sequences. Though not widely employed, some have demonstrated that 3D quantitative perfusion analysis is feasible with good diagnostic accuracy for CAD (85–88).
Hautvast et al. (89) proposed an alternative approach to exploit and quantify high-resolution perfusion CMR data for the assessment of transmural gradients. This is based on the quantification of perfusion gradients between endocardial and epicardial myocardial layers. The transmural perfusion gradient (TPG) is defined as the percentage of transmural redistribution of myocardial blood flow between layers. The optimal diagnostic threshold for TPG is 20%, which identifies the presence of haemodynamically significant ischaemia in comparison with FFR (90) with high sensitivity, specificity, and diagnostic accuracy. There are several advantages to transmural perfusion gradient analysis compared to other methods of quantitative analysis. Firstly, administration of a diluted pre-bolus is not required nor is the acquisition of rest perfusion images. The method is also more robust to image homogeneity due to variations in the B1 field, different coil configurations, different schemes of contrast agent administration, field strength, and the acquisition pulse sequence (89, 90).
More recently, another approach, the perfusion dyssynchrony analysis, has been proposed (91). This examines both the spatial and temporal dispersion of myocardial signals. The concept rests on the knowledge that in normal myocardium with preserved vasodilatory reserve, the myocardium is perfused uniformly across all segments. The presence of one or more coronary stenoses influences not only the peak but also the time to peak myocardial signal. Specifically, in cases of multi-vessel CAD, the temporal dispersion has been shown to increase in relation to the number of diseased vessels, using FFR as a correlate for haemodynamic significance.
In summary, robust quantification of perfusion has been a goal of many investigators for over two decades. Significant advances in image acquisition, dosing regimens, and analysis methods, particularly in recent years, along with robust validation against a number of techniques, have significantly advanced the field towards clinical translation.
Diffusion Tensor Imaging
DTI, also known as diffusion tractography, is an advanced CMR technique which allows the study of 3-dimensional whole-organ tissue microstructure, it was initially developed for static organs such as neurological tracts in the brain (92, 93). In more recent years cardiac DTI has become a reality (94). The myocardium has a complex microarchitecture. This consists of a three-dimensional functional syncytium of branching and inter-connecting myocytes. These myocytes are embedded in a collagen matrix. Myocytes are grouped in layers of 5–10, separated by collagen sheetlets which interconnect with neighbouring layers (95, 96). In the left ventricle, the overall direction of myocyte aggregates follows a helical arrangement running in opposite directions at subendocardial and subepicardial levels; the helix is right-handed (positive angulation) at the subendocardium and transitions to being left-handed (negative angulation) at the subepicardum with myofibre aggregates in the mid LV layer being orientated transversely (97). The structure and interplay of the myocytes are intrinsically linked to cardiac contractility and efficiency and therefore the opportunity to perform cardiac DTI offers the potential for deeper understanding of cardiac mechanics in health and disease.
The base principle of DTI involves the imaging of the diffusion of water. In brief, when water is not bound to tissue, it will diffuse at the same rate in all directions—this can be visualised as a sphere. Whereas in a tissue, water will diffuse predominantly in one direction more than in others—this is known as the phenomenon of anisotropy. This results in a different diffusion characteristic that can be seen to progress in different directions and this diffusion property of water can be used to create a map of diffusion. This can be applied to a region of interest—the displacement probability of diffusing water molecules within the myocardium can be measured and the arrangement of the mean direction of water diffusion can be reconstructed. In cardiac imaging, DTI is acquired as a series of two-dimensional short axis ventricular slices which are then reconstructed in 3D form. The use of diffusion-sensitising sequences allows calculation of a set of three eigenvectors with water diffusing more readily along the myofiber, here the average local myocyte orientation within the voxel is represented by the first, largest eigenvector (E1). The second largest eigenvector (E2) corresponds to the average local sheetlet direction. The mean helix angle is represented by E1A. E1A is the angle relative to the local wall tangent plane. E2A represents the mean intra-voxel sheetlet angle. The “third” eigenvector is the sheet-normal that is perpendicular to the helix and sheet plane (also described as the transverse angle). Scalar metrics such as mean diffusivity (MD) and fractional anisotropy (FA) allow phenotyping the structural integrity by registering the degree of free diffusion in a tissue as a general measure. The “diffusivity” is described as the average of the sum of eigenvalues whereas “fractional anisotropy” is described as an index which reflects the degree of anisotropy within a voxel (the variation by which the tissue diffusion contrasts to an idealised sphere).
Early ex-vivo cardiac DTI work was demonstrated that cardiac DTI was feasible without destruction of the myocardial architecture and also confirmed that the first and second eigenvectors correlated with histological orientation of the myofibres and sheetlet direction, respectively (98–100). Early pilot studies also explored changes in fibre architecture in pathologies such as myocardial infarction (101, 102). There are several limitations associated with ex-vivo cardiac DTI regarding the method/ duration of fixation of the tissue, the cardiac phase in which the heart was fixed (i.e., in a systolic or diastolic state), and the absence of normal loading conditions. Ex-vivo work suggested a dynamic rearrangement of the myocytes during contraction, but in recent years, the development of in-vivo dual-phase cardiac DTI has built on this. Recent work has shown that macroscopic LV hypertrophy is associated with sheetlet angle during contraction (103, 104).
The application of DTI to a moving tissue, in particular the cardiovascular system, is more challenging than in a static organ. The relaxation time of the myocardium is shorter than in the cerebral tissue, which imposes significant limitations on the echo time that can be used. Moreover, inhomogeneity of the B0-field is increased in the thoracic cavity, particularly at higher field strengths in comparison with the brain. This results in a higher incidence of artefacts, which can affect the diffusion-weighted images. Furthermore, DTI in a beating heart with respiratory motion and displacement of the myocardium during contraction is challenging in that the diffusion of water is several orders of magnitude smaller than bulk motion. The sequences that are used for ex-vivo imaging are highly motion-sensitive and therefore are not necessarily suitable for in-vivo imaging. A number of other novel DTI sequences have been proposed. The most broadly used technique is the dual-gated stimulated echo acquisition mode (STEAM) sequence. In brief, these involve three excitation pulses applied over two successive heartbeats. This STEAM sequence is relatively insensitive to cardiac motion however it is not robust in arrhythmia. Cardiac DTI is also affected by cardiac strain. The strain produced by myocardial deformation has an impact on diffusion measurements. Proposed approaches to circumvent this issue include imaging at the so-called “sweet-spot” which is a time point, individual for a subject, where the temporal mean of strain approaches zero, thereby eliminating the effect of strain, typically at the mid-systolic or mid-diastolic phase of the cardiac cycle (105, 106). Post-processing methods also allow to correct for the effects of strain (107).
In vivo cardiac DTI has reported reproducibility and normal ranges of helix and sheetlet angles in healthy hearts (94, 108–111), and has since been used to explore a variety of pathologies including myocardial infarction, cardiac amyloidosis, and both dilated and hypertrophic cardiomyopathy (112–117). These more recent studies have underlined the potential for DTI to investigate architecture-related changes in disease in relation to biomechanics and over time. Data from in-vivo dual-phase cardiac DTI in patients with DCM combined with tagging data and biomechanical modelling explored the relationship between geometry and helix and sheetlet angle, concluding that helix and sheetlet angle changes are likely to be maladaptive rather than compensatory (117). A fascinating and elaborate study by Nielles-Vallespin et al. (113) shed more detailed light on changes in architecture in DCM and HCM; the authors were able to conclude that the myocardium in hypertrophic cardiomyopathy is permanently in a more systolic, contracted state whereas in dilated cardiomyopathy the myocardium is in a more diastolic state. This may potentially account for the predominant diastolic impairment seen in hypertrophic cardiomyopathy compared with the more prevalent systolic impairment seen in dilated cardiomyopathy. Recent studies have also investigated the changes in infarcted tissue comparing DTI with late gadolinium enhancement imaging demonstrating changes in infarcted tissue, suggesting there may be an opportunity to use DTI to evaluate myocardial scar in place of using a gadolinium-based contrast agent (118), and suggested that cardiac DTI may even provide complementary information on remodelling post-myocardial infarction (119). Technical developments in cardiac DTI are ongoing with recent studies exploring the use of cardiac DTI at 7-Tesla (120).
There is no doubt that there is a crucial interplay between the cardiac microstructure, and the biomechanical and electrical functions of cardiac function and that cardiac DTI holds promise in developing a deeper understanding of this relationship. Recent years have seen significant developments in the cardiac DTI, allowing a greater understanding of cardiac structure and function in both health and disease. Though cardiac DTI remains a research tool with ongoing work in optimisation of the technique, in due course this advanced technology may play a part in identifying novel biomarkers or response to new therapies.
Four-Dimensional Flow
Intracardiac blood flow and its velocities have played a very important role in cardiovascular assessment. However, novel imaging methods like four-dimensional flow CMR (4D flow CMR) imaging are resulting in a paradigm shift as they inform us about flow in all three-directions (Figure 6) (121). We already know that the blood flow is complex, dynamic, and has a diverse 3D profile (122). The fourth dimension in 4D flow CMR imaging is time. More recently, 4D flow CMR has seen significant development allowing for whole-heart cross-sectional flow imaging in under 10 min (123–125).
Visualisation
One method to visualise 4D flow is to co-register the velocity coded 2-dimensional flow over the cine planes. This provides an instant and quick assessment of flow vectors depending on the cine visualised (Figure 7). For example, a four-chamber or two-chamber cine can then be used to visualise mitral regurgitation jets using 4D flow CMR. Furthermore, 4D flow CMR enables the visualisation of flow accelerations through stenotic valvular or vascular lesions and can provide additional information in challenging cases such as congenital heart disease where haemodynamic effects of anatomical abnormalities are not always clear. 4D flow CMR visualisation can be integrated into routine CMR reporting and can add diagnostic advantage. Valvular stenotic flow accelerations and pathological eccentricity of the flow can be determined by visualisation of 2-directional velocity vectors. This becomes clinically relevant, for example, an eccentric aortic ejection flow direction would raise the suspicion of bicuspid aortic valve or aortic stenosis (126, 127). Routine visualisation of 2-directional vectors on four-chamber cine can enhance detection of septal defects, flow acceleration in outflow tracts, and valvular regurgitation (Figure 8).
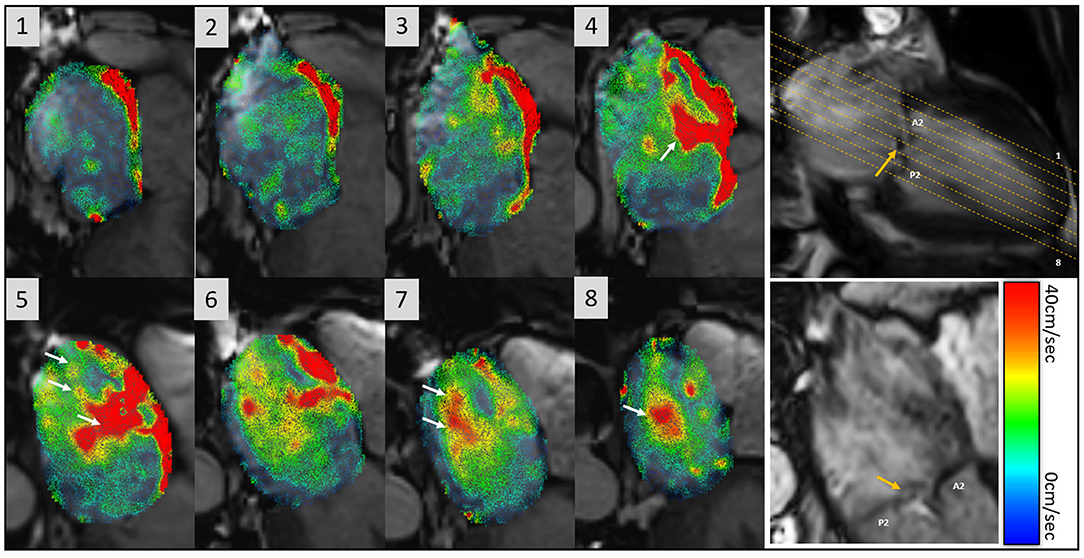
Figure 7. Overview of flow visualisation in the left atrium in a patient with mitral regurgitation secondary to P2 prolapse (orange arrow). 4D flow data is co-registered with cines to demonstrate 2-directional vectors, which have speed encoded colour coding (0–40 cm/s). There is eccentric mitral regurgitation that is directed towards the intra-atrial septum and swirls back into the left atrium (white arrows in panels 4–8). The mitral regurgitation jet almost fills the entire left atrium.
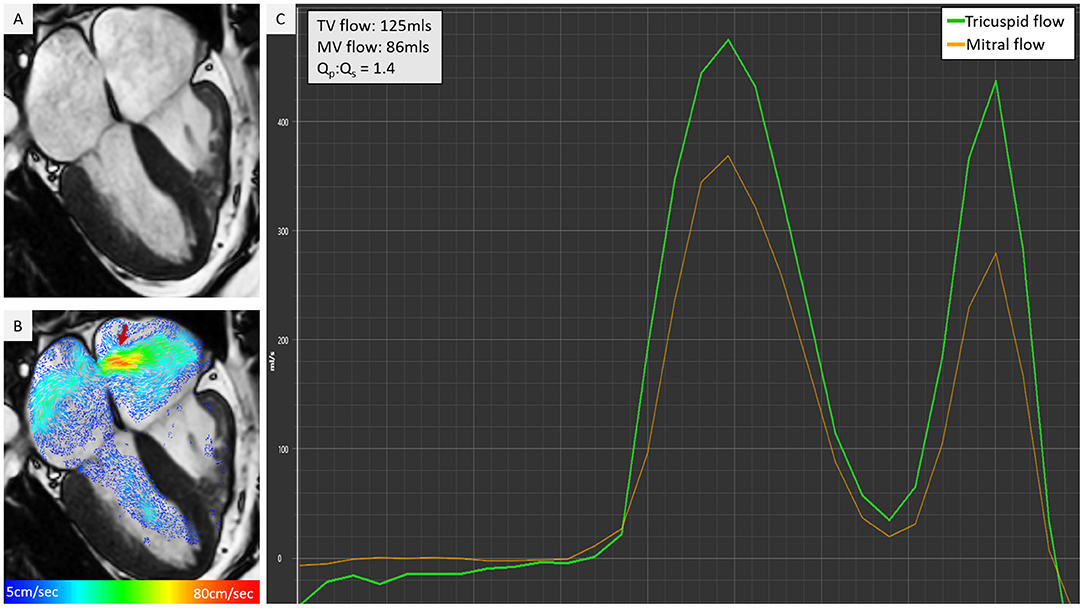
Figure 8. Cine co-registered 4D flow visualisation can offer instant understanding of intra-cardiac shunts and other similar cardiac pathologies. In this figure, there is atrial septal defect that was not clearly recognised on cine (A), however, with 2-directional vector visualisation in (B), there was an obvious left to right shunt. This was quantified by retrospective valve tracking method and significant Qp, Qs was noted (C).
Vortex Imaging
We are now developing a better understanding of blood flow behaviour inside the ventricles using 4D flow CMR. Vortices are formed in almost all chambers of the heart and major vessels. Vortices can be due to optimum physiological flow (128), pathological flow due to dilatation (129), or raised pressures (130). Vortical flow in the main pulmonary artery is an example of pathological flow and is associated with elevated main pulmonary arterial pressure (131). Vortical blood flow in the main pulmonary artery tends to be pathological and a threshold >14.3% of the cardiac interval is associated with pulmonary hypertension (sensitivity: 97%; specificity: 96%). Similarly, in aortic root dilation, more helical flows with vortices have been demonstrated as pathological (132). Another study also showed that flow abnormalities may be a major contributor to aortic dilation in patients with bicuspid aortic valve disease (133).
Quantification of 4D Flow
4D flow CMR not only helps to visualise but also allows to quantify vascular, valvular, and intra-ventricular flow. These include transvalvular flow, intra-cavity velocity or kinetic energy (KE) assessment, vortex quantification, blood flow component analysis, and haemodynamic forces quantification (134). From the above-mentioned methods, valvular flow quantification using retrospective valve tracking is ready for clinical adoption as it has been validated and has demonstrated superior accuracy to standard 2D phase-contrast methods (134–136). The other method which shows promising intra-/inter-observer reliability is intra-cavity blood flow KE mapping (137). Most of the other techniques remain research tools, which have offered mechanistic insight into several disease processes and are currently under investigation of direct clinical applications.
Quantification of Transvalvular Flow
4D flow CMR in the context of retrospective valve tracking allows quantification of flow through all four heart valves for the same averaged cardiac cycle, whilst factoring in valve motion by tracking the valve (138) (Figure 9). This valve tracking method overcomes the limitations of conventional 2D phase-contrast velocity encoded imaging or Doppler ultrasound-based through-plane motion. Therefore, 4D flow CMR flow quantification methods can be considered to be the non-invasive “gold-standard” for intracardiac flow quantification (139–141). In patients with limited apical ultrasound views, 4D flow CMR may provide an alternative to assess peak E and A velocities for LV diastolic function assessment. Moreover, 4D flow CMR can be used to directly quantify regurgitant volumes by generating a reformatted plane in the 4D volume data set that is exactly perpendicular to the regurgitant jet (142). Software solutions with automated valve tracking procedures reduce post-processing time significantly and are becoming more readily available (143).
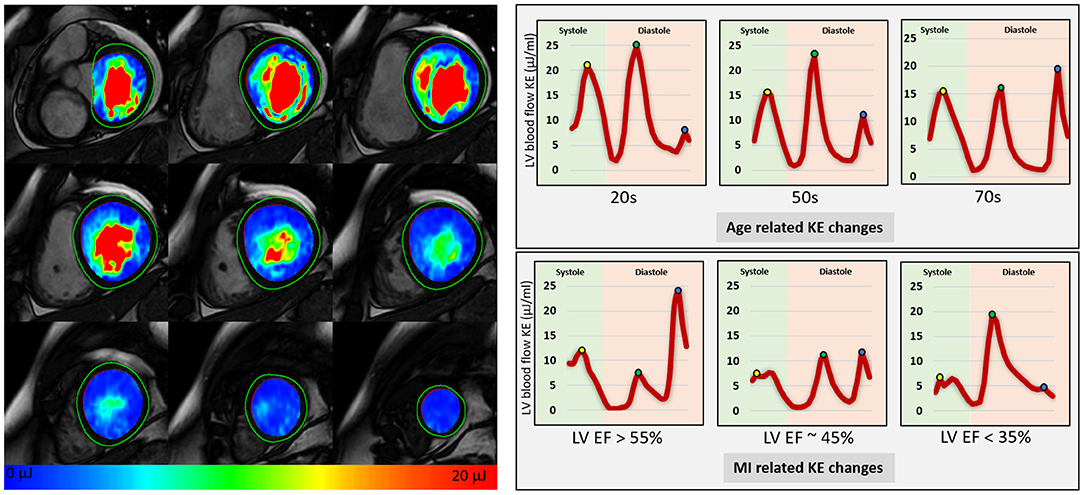
Figure 9. Left ventricular blood flow kinetic energy mapping in healthy, ageing, and in patients with myocardial infarction.
Quantification of Blood Flow Kinetic Energy
4D flow CMR also allows us to quantify the KE of blood flow through a chamber of the heart for the complete cardiac cycle. The KE of a moving blood flow with mass (m) and velocity (v) is KE = ½m × v2. In this equation mass is (blood viscosity) × (voxel size). Using the KE formula, the whole left ventricular blood flow KE can be quantified by summing the KE of each individual voxel within the ventricular cavity. This is achieved by superimposing the time-resolved endocardial contours defined from cine images onto the 4D flow data to extract velocity information. The analysis of KE is predominantly automated. As this technique uses endocardial contours which have established high reproducibility, a key strength of KE quantification is its excellent intra-/inter-observer agreement and almost instant haemodynamic assessment without needing any additional post-processing (137). As LV blood flow KE takes into consideration complete intra-cavity flow, it is a better marker of diastolic function when compared to standard diastolic parameters (Figure 9) (137). Furthermore, studies have shown how it is an independent predictor of adverse remodelling post-myocardial infarction (144) and even LV thrombus formation (145). This emergent tool holds great potential for phenotyping cardiac haemodynamics, informing diagnosis, and potentially predicting disease course. Future trials are needed to evaluate its value in informing treatment outcomes in cardiovascular diseases.
Future Perspectives
The role of CMR and its inclusion in multiple guidelines across the breadth of cardiology has been expanding steadily in recent years. Whilst conventional techniques provide without doubt the gold standard assessment for anatomy, function, and tissue characterisation in a non-invasive radiation-free method, the emergence of novel methods of CMR will allow incremental value not only in the diagnosis, but also in the management of more patients with cardiac pathology. It is only a matter of time before the described techniques become more broadly available to the cardiovascular imaging community and further prognostic studies validate the utility of these techniques for clinical translation.
Conclusion
CMR has a role of paramount importance in the assessment and diagnosis of cardiovascular disease. We reviewed the conventional CMR methods currently used in clinical practice but also described novel methods likely to enter the clinical arena in the future. With these novel methods, CMR can successfully guide clinical management further by revealing clinically important clues related to cardiac anatomy and function. Technical developments, advanced methods of image acquisition, and a better understanding of cardiac haemodynamics provide the foundation for further research and validation of the newly emerging tools and techniques that are being proved to be of extreme clinical importance.
Author Contributions
SP, VT, HA, and BM: original draft preparation, writing - review and editing, reference management, and quality checks. PG, AC, PS, AS, and EL: conceptualisation, data curation, and project administration. PG, AC, ES, AD, JC, and JR: figures, illustrations, and content. JS, AR, CS, RG, PM, DH, VV, and PG: critical appraisal, editing, and draft final version. All authors contributed to the article and approved the submitted version.
Funding
This work was funded in part by the Wellcome Trust [215799/Z/19/Z] and [205188/Z/16/Z]. For the purpose of Open Access, the author has applied a CC BY public copyright licence to any Author Accepted Manuscript version arising from this submission.
Conflict of Interest
PG is an advisor for Pie Medical Imaging and Medis Medical Imaging.
The remaining authors declare that the research was conducted in the absence of any commercial or financial relationships that could be construed as a potential conflict of interest.
References
1. Garg P, Saunders LC, Swift AJ, Wild JM, Plein S. Role of cardiac T1 mapping and extracellular volume (ECV) in the assessment of myocardial infarction. Anatol J Cardiol. (2018) 19:404–11. doi: 10.14744/AnatolJCardiol.2018.39586
2. Satoh H, Sano M, Suwa K, Saitoh T, Nobuhara M, Saotome M, et al. Distribution of late gadolinium enhancement in various types of cardiomyopathies: significance in differential diagnosis, clinical features and prognosis. World J Cardiol. (2014) 6:585–601. doi: 10.4330/wjc.v6.i7.585
3. Smulders MW, Bekkers SCAM, Kim HW, Van Assche LMR, Parker MA, Kim RJ. Performance of CMR methods for differentiating acute from chronic MI. JACC Cardiovasc Imaging. (2015) 8:669–79. doi: 10.1016/j.jcmg.2014.12.030
4. Karamitsos TD, Francis JM, Myerson S, Selvanayagam JB, Neubauer S. The role of cardiovascular magnetic resonance imaging in heart failure. J Am Coll Cardiol. (2009) 54:1407–24. doi: 10.1016/j.jacc.2009.04.094
5. Karamitsos TD, Neubauer S. Cardiovascular magnetic resonance in heart failure. Curr Cardiol Rep. (2011) 13:210–9. doi: 10.1007/s11886-011-0177-2
6. Vassiliou VS, Perperoglou A, Raphael CE, Joshi S, Malley T, Everett R, et al. Midwall fibrosis and 5-year outcome in moderate and severe aortic stenosis. J Am Coll Cardiol. (2017) 69:1755–6. doi: 10.1016/j.jacc.2017.01.034
7. Halliday BP, Gulati A, Ali A, Guha K, Newsome S, Arzanauskaite M, et al. Association between midwall late gadolinium enhancement and sudden cardiac death in patients with dilated cardiomyopathy and mild and moderate left ventricular systolic dysfunction. Circulation. (2017) 135:2106–15. doi: 10.1161/CIRCULATIONAHA.116.026910
8. Babu-Narayan SV, Kilner PJ, Li W, Moon JC, Goktekin O, Davlouros PA, et al. Ventricular fibrosis suggested by cardiovascular magnetic resonance in adults with repaired tetralogy of fallot and its relationship to adverse markers of clinical outcome. Circulation. (2006) 113:405–13. doi: 10.1161/CIRCULATIONAHA.105.548727
9. Alam MH, Auger D, McGill L-A, Smith GC, He T, Izgi C, et al. Comparison of 3 T and 1.5 T for T2* magnetic resonance of tissue iron. J Cardiovasc Magn Reson. (2016) 18:40. doi: 10.1186/s12968-016-0259-9
10. Halliday BP, Baksi AJ, Gulati A, Ali A, Newsome S, Izgi C, et al. Outcome in dilated cardiomyopathy related to the extent, location, and pattern of late gadolinium enhancement. JACC Cardiovasc Imaging. (2019) 12(8 Pt 2):1645–55. doi: 10.1016/j.jcmg.2018.07.015
11. Raphael CE, Liew AC, Mitchell F, Kanaganayagam GS, Pietro ED, Newsome S, et al. Predictors and mechanisms of atrial fibrillation in patients with hypertrophic cardiomyopathy. Am J Cardiol. (2020) 136:140–8. doi: 10.1016/j.amjcard.2020.09.006
12. Nelson T, Garg P, Clayton RH, Lee J. The role of cardiac MRI in the management of ventricular arrhythmias in ischaemic and non-ischaemic dilated cardiomyopathy. Arrhythmia Electrophysiol Rev. (2019) 8:191–201. doi: 10.15420/aer.2019.5.1
13. Arevalo HJ, Vadakkumpadan F, Guallar E, Jebb A, Malamas P, Wu KC, et al. Arrhythmia risk stratification of patients after myocardial infarction using personalized heart models. Nat Commun. (2016) 7:11437. doi: 10.1038/ncomms11437
14. Linhart M, Alarcon F, Borràs R, Benito EM, Chipa F, Cozzari J, et al. Delayed gadolinium enhancement magnetic resonance imaging detected anatomic gap length in wide circumferential pulmonary vein ablation lesions is associated with recurrence of atrial fibrillation. Circ Arrhythm Electrophysiol. (2018) 11:e006659. doi: 10.1161/CIRCEP.118.006659
15. Yan AT, Shayne AJ, Brown KA, Gupta SN, Chan CW, Luu TM, et al. Characterization of the peri-infarct zone by contrast-enhanced cardiac magnetic resonance imaging is a powerful predictor of post-myocardial infarction mortality. Circulation. (2006) 114:32–9. doi: 10.1161/CIRCULATIONAHA.106.613414
16. Roes SD, Borleffs CJW, van der Geest RJ, Westenberg JJM, Marsan NA, Kaandorp TAM, et al. Infarct tissue heterogeneity assessed with contrast-enhanced MRI predicts spontaneous ventricular arrhythmia in patients with ischemic cardiomyopathy and implantable cardioverter-defibrillator. Circ Cardiovasc Imaging. (2009) 2:183–90. doi: 10.1161/CIRCIMAGING.108.826529
17. Andreu D, Penela D, Acosta J, Fernández-Armenta J, Perea RJ, Soto-Iglesias D, et al. Cardiac magnetic resonance-aided scar dechanneling: influence on acute and long-term outcomes. Heart Rhythm. (2017) 14:1121–8. doi: 10.1016/j.hrthm.2017.05.018
18. Dzyubachyk O, Tao Q, Poot DHJ, Lamb HJ, Zeppenfeld K, Lelieveldt BPF, et al. Super-resolution reconstruction of late gadolinium-enhanced MRI for improved myocardial scar assessment. J Magn Reson Imaging JMRI. (2015) 42:160–7. doi: 10.1002/jmri.24759
19. Messroghli DR, Moon JC, Ferreira VM, Grosse-Wortmann L, He T, Kellman P, et al. Clinical recommendations for cardiovascular magnetic resonance mapping of T1, T2, T2* and extracellular volume: a consensus statement by the Society for Cardiovascular Magnetic Resonance (SCMR) endorsed by the European Association for Cardiovascular Imaging (EACVI). J Cardiovasc Magn Reson. (2017) 19:75. doi: 10.1186/s12968-017-0389-8
20. Haaf P, Garg P, Messroghli DR, Broadbent DA, Greenwood JP, Plein S. Cardiac T1 mapping and extracellular volume (ECV) in clinical practice: a comprehensive review. J Cardiovasc Magn Reson. (2016) 18:89. doi: 10.1186/s12968-016-0308-4
21. Dastidar AG, Baritussio A, De Garate E, Drobni Z, Biglino G, Singhal P, et al. Prognostic role of CMR and conventional risk factors in myocardial infarction with nonobstructed coronary arteries. JACC Cardiovasc Imaging. (2019) 12:1973–82. doi: 10.1016/j.jcmg.2018.12.023
22. Liu D, Borlotti A, Viliani D, Jerosch-Herold M, Alkhalil M, De Maria GL, et al. CMR native T1 mapping allows differentiation of reversible versus irreversible myocardial damage in ST-segment-elevation myocardial infarction CLINICAL PERSPECTIVE. Circ Cardiovasc Imaging. (2017) 10:e005986. doi: 10.1161/CIRCIMAGING.116.005986
23. Garg P, Broadbent DA, Swoboda PP, Foley JRJ, Fent GJ, Musa TA, et al. Acute infarct extracellular volume mapping to quantify myocardial area at risk and chronic infarct size on cardiovascular magnetic resonance imaging. Circ Cardiovasc Imaging. (2017) 10:e006182. doi: 10.1161/CIRCIMAGING.117.006182
24. Chin CWL, Everett RJ, Kwiecinski J, Vesey AT, Yeung E, Esson G, et al. Myocardial fibrosis and cardiac decompensation in aortic stenosis. JACC Cardiovasc Imaging. (2017) 10:1320–33. doi: 10.1016/j.jcmg.2016.10.007
25. Everett RJ, Tastet L, Clavel MA, Chin CWL, Capoulade R, Vassiliou VS, et al. Progression of hypertrophy and myocardial fibrosis in aortic stenosis. Circ Cardiovasc Imaging. (2018) 11:e007451. doi: 10.1161/CIRCIMAGING.117.007451
26. Treibel TA, Kozor R, Schofield R, Benedetti G, Fontana M, Bhuva AN, et al. Reverse myocardial remodeling following valve replacement in patients with aortic stenosis. J Am Coll Cardiol. (2018) 71:860–71. doi: 10.1016/j.jacc.2017.12.035
27. Bing R, Everett RJ, Tuck C, Semple S, Lewis S, Harkess R, et al. Rationale and design of the randomized, controlled Early Valve Replacement Guided by Biomarkers of Left Ventricular Decompensation in Asymptomatic Patients with Severe Aortic Stenosis (EVOLVED) trial. Am Heart J. (2019) 212:91–100. doi: 10.1016/j.ahj.2019.02.018
28. Park S-J, Cho SW, Kim SM, Ahn J, Carriere K, Jeong DS, et al. Assessment of myocardial fibrosis using multimodality imaging in severe aortic stenosis: comparison with histologic fibrosis. JACC Cardiovasc Imaging. (2019) 12:109–19. doi: 10.1016/j.jcmg.2018.05.028
29. Vassiliou VS, Wassilew K, Cameron D, Heng EL, Nyktari E, Asimakopoulos G, et al. Identification of myocardial diffuse fibrosis by 11 heartbeat MOLLI T1 mapping: averaging to improve precision and correlation with collagen volume fraction. Magma N Y N. (2018) 31:101–13. doi: 10.1007/s10334-017-0630-3
30. Lee H, Park J-B, Yoon YE, Park E-A, Kim H-K, Lee W, et al. Noncontrast myocardial T1 mapping by cardiac magnetic resonance predicts outcome in patients with aortic stenosis. JACC Cardiovasc Imaging. (2018) 11:974–83. doi: 10.1016/j.jcmg.2017.09.005
31. Everett RJ, Treibel TA, Fukui M, Lee H, Rigolli M, Singh A, et al. Extracellular myocardial volume in patients with aortic stenosis. J Am Coll Cardiol. (2020) 75:304–16. doi: 10.1016/j.jacc.2019.11.032
32. Edwards NC, Moody WE, Yuan M, Weale P, Neal D, Townend JN, et al. Quantification of left ventricular interstitial fibrosis in asymptomatic chronic primary degenerative mitral regurgitation. Circ Cardiovasc Imaging. (2014) 7:946–53. doi: 10.1161/CIRCIMAGING.114.002397
33. Liu B, Edwards NC, Neal DAH, Weston C, Nash G, Nikolaidis N, et al. A prospective study examining the role of myocardial Fibrosis in outcome following mitral valve repair IN DEgenerative mitral Regurgitation: rationale and design of the mitral FINDER study. BMC Cardiovasc Disord. (2017) 17:282. doi: 10.1186/s12872-017-0715-y
34. Foley JRJ, Kidambi A, Biglands JD, Maredia N, Dickinson CJ, Plein S, et al. A comparison of cardiovascular magnetic resonance and single photon emission computed tomography (SPECT) perfusion imaging in left main stem or equivalent coronary artery disease: a CE-MARC substudy. J Cardiovasc Magn Reson. (2017) 19:84. doi: 10.1186/s12968-017-0398-7
35. Greenwood JP, Motwani M, Maredia N, Brown JM, Everett CC, Nixon J, et al. Comparison of cardiovascular magnetic resonance and single-photon emission computed tomography in women with suspected coronary artery disease from the Clinical Evaluation of Magnetic Resonance Imaging in Coronary Heart Disease (CE-MARC) Trial. Circulation. (2014) 129:1129–38. doi: 10.1161/CIRCULATIONAHA.112.000071
36. Nandalur KR, Dwamena BA, Choudhri AF, Nandalur MR, Carlos RC. Diagnostic performance of stress cardiac magnetic resonance imaging in the detection of coronary artery disease: a meta-analysis. J Am Coll Cardiol. (2007) 50:1343–53. doi: 10.1016/j.jacc.2007.06.030
37. Hamon M, Fau G, Née G, Ehtisham J, Morello R, Hamon M. Meta-analysis of the diagnostic performance of stress perfusion cardiovascular magnetic resonance for detection of coronary artery disease. J Cardiovasc Magn Reson. (2010) 12:29. doi: 10.1186/1532-429X-12-29
38. Rieber J, Huber A, Erhard I, Mueller S, Schweyer M, Koenig A, et al. Cardiac magnetic resonance perfusion imaging for the functional assessment of coronary artery disease: a comparison with coronary angiography and fractional flow reserve. Eur Heart J. (2006) 27:1465–71. doi: 10.1093/eurheartj/ehl039
39. Nagel E, Greenwood JP, McCann GP, Bettencourt N, Shah AM, Hussain ST, et al. Magnetic resonance perfusion or fractional flow reserve in coronary disease. N Engl J Med. (2019) 380:2418–28. doi: 10.1056/NEJMoa1716734
40. Lockie T, Ishida M, Perera D, Chiribiri A, De Silva K, Kozerke S, et al. High-resolution magnetic resonance myocardial perfusion imaging at 3.0-Tesla to detect hemodynamically significant coronary stenoses as determined by fractional flow reserve. J Am Coll Cardiol. (2011) 57:70–5. doi: 10.1016/j.jacc.2010.09.019
41. Bettencourt N, Chiribiri A, Schuster A, Ferreira N, Sampaio F, Duarte R, et al. Cardiac magnetic resonance myocardial perfusion imaging for detection of functionally significant obstructive coronary artery disease: a prospective study. Int J Cardiol. (2013) 168:765–73. doi: 10.1016/j.ijcard.2012.09.231
42. Bettencourt N, Chiribiri A, Schuster A, Ferreira N, Sampaio F, Pires-Morais G, et al. Direct comparison of cardiac magnetic resonance and multidetector computed tomography stress-rest perfusion imaging for detection of coronary artery disease. J Am Coll Cardiol. (2013) 61:1099–107. doi: 10.1016/j.jacc.2012.12.020
43. Schwitter J, Wacker CM, van Rossum AC, Lombardi M, Al-Saadi N, Ahlstrom H, et al. MR-IMPACT: comparison of perfusion-cardiac magnetic resonance with single-photon emission computed tomography for the detection of coronary artery disease in a multicentre, multivendor, randomized trial. Eur Heart J. (2008) 29:480–9. doi: 10.1093/eurheartj/ehm617
44. Schwitter J, Wacker CM, Wilke N, Al-Saadi N, Sauer E, Huettle K, et al. MR-IMPACT II: magnetic resonance imaging for myocardial perfusion assessment in coronary artery disease trial: perfusion-cardiac magnetic resonance vs. single-photon emission computed tomography for the detection of coronary artery disease: a comparative multicentre, multivendor trial. Eur Heart J. (2013) 34:775–81. doi: 10.1093/eurheartj/ehs022
45. Greenwood JP, Maredia N, Younger JF, Brown JM, Nixon J, Everett CC, et al. Cardiovascular magnetic resonance and single-photon emission computed tomography for diagnosis of coronary heart disease (CE-MARC): a prospective trial. Lancet Lond Engl. (2012) 379:453–60. doi: 10.1016/S0140-6736(11)61335-4
46. Lipinski MJ, McVey CM, Berger JS, Kramer CM, Salerno M. Prognostic value of stress cardiac magnetic resonance imaging in patients with known or suspected coronary artery disease: a systematic review and meta-analysis. J Am Coll Cardiol. (2013) 62:826–38. doi: 10.1016/S0735-1097(13)60809-8
47. Freed BH, Narang A, Bhave NM, Czobor P, Mor-Avi V, Zaran ER, et al. Prognostic value of normal regadenoson stress perfusion cardiovascular magnetic resonance. J Cardiovasc Magn Reson. (2013) 15:108. doi: 10.1186/1532-429X-15-108
48. Gargiulo P, Dellegrottaglie S, Bruzzese D, Savarese G, Scala O, Ruggiero D, et al. The prognostic value of normal stress cardiac magnetic resonance in patients with known or suspected coronary artery disease. Circ Cardiovasc Imaging. (2013) 6:574–82. doi: 10.1161/CIRCIMAGING.113.000035
49. Costa MA, Shoemaker S, Futamatsu H, Klassen C, Angiolillo DJ, Nguyen M, et al. Quantitative magnetic resonance perfusion imaging detects anatomic and physiologic coronary artery disease as measured by coronary angiography and fractional flow reserve. J Am Coll Cardiol. (2007) 50:514–22. doi: 10.1016/j.jacc.2007.04.053
50. Hsu L-Y, Groves DW, Aletras AH, Kellman P, Arai AE. A quantitative pixel-wise measurement of myocardial blood flow by contrast-enhanced first-pass CMR perfusion imaging: microsphere validation in dogs and feasibility study in humans. JACC Cardiovasc Imaging. (2012) 5:154–66. doi: 10.1016/j.jcmg.2011.07.013
51. Schwitter J, Nanz D, Kneifel S, Bertschinger K, Büchi M, Knüsel PR, et al. Assessment of myocardial perfusion in coronary artery disease by magnetic resonance: a comparison with positron emission tomography and coronary angiography. Circulation. (2001) 103:2230–5. doi: 10.1161/01.CIR.103.18.2230
52. Morton G, Chiribiri A, Ishida M, Hussain ST, Schuster A, Indermuehle A, et al. Quantification of absolute myocardial perfusion in patients with coronary artery disease: comparison between cardiovascular magnetic resonance and positron emission tomography. J Am Coll Cardiol. (2012) 60:1546–55. doi: 10.1016/S0735-1097(12)61065-1
53. Patel AR, Antkowiak PF, Nandalur KR, West AM, Salerno M, Arora V, et al. Assessment of advanced coronary artery disease: advantages of quantitative cardiac magnetic resonance perfusion analysis. J Am Coll Cardiol. (2010) 56:561–9. doi: 10.1016/j.jacc.2010.02.061
54. Sammut EC, Villa ADM, Di Giovine G, Dancy L, Bosio F, Gibbs T, et al. Prognostic value of quantitative stress perfusion cardiac magnetic resonance. JACC Cardiovasc Imaging. (2018) 11:686–94. doi: 10.1016/j.jcmg.2017.07.022
55. Brown LAE, Onciul SC, Broadbent DA, Johnson K, Fent GJ, Foley JRJ, et al. Fully automated, inline quantification of myocardial blood flow with cardiovascular magnetic resonance: repeatability of measurements in healthy subjects. J Cardiovasc Magn Reson. (2018) 20:48. doi: 10.1186/s12968-018-0462-y
56. Hsu L-Y, Jacobs M, Benovoy M, Ta AD, Conn HM, Winkler S, et al. Diagnostic performance of fully automated pixel-wise quantitative myocardial perfusion imaging by cardiovascular magnetic resonance. JACC Cardiovasc Imaging. (2018) 11:697–707. doi: 10.1016/j.jcmg.2018.01.005
57. Engblom H, Tufvesson J, Jablonowski R, Carlsson M, Aletras AH, Hoffmann P, et al. A new automatic algorithm for quantification of myocardial infarction imaged by late gadolinium enhancement cardiovascular magnetic resonance: experimental validation and comparison to expert delineations in multi-center, multi-vendor patient data. J Cardiovasc Magn Reson. (2016) 18:27. doi: 10.1186/s12968-016-0242-5
58. Knott KD, Camaioni C, Ramasamy A, Augusto JA, Bhuva AN, Xue H, et al. Quantitative myocardial perfusion in coronary artery disease: a perfusion mapping study. J Magn Reson Imaging JMRI. (2019) 50:756–62. doi: 10.1002/jmri.26668
59. Scannell CM, Veta M, Villa ADM, Sammut EC, Lee J, Breeuwer M, et al. Deep-learning-based preprocessing for quantitative myocardial perfusion MRI. J Magn Reson Imaging JMRI. (2020) 51:1689–96. doi: 10.1002/jmri.26983
60. Knott KD, Seraphim A, Augusto JB, Xue H, Chacko L, Aung N, et al. The prognostic significance of quantitative myocardial perfusion. Circulation. (2020) 141:1282–91. doi: 10.1161/CIRCULATIONAHA.119.044666
61. Zierler KL. Theoretical basis of indicator-dilution methods for measuring flow and volume. Circ Res. (1962) 10:393–407. doi: 10.1161/01.RES.10.3.393
62. Sammut E, Zarinabad N, Vianello PF, Chiribiri A. Quantitative assessment of perfusion - where are we now? Curr Cardiovasc Imaging Rep. (2014) 7:9278. doi: 10.1007/s12410-014-9278-9
63. Jerosch-Herold M, Wilke N, Stillman AE. Magnetic resonance quantification of the myocardial perfusion reserve with a Fermi function model for constrained deconvolution. Med Phys. (1998) 25:73–84. doi: 10.1118/1.598163
64. Kroll K, Wilke N, Jerosch-Herold M, Wang Y, Zhang Y, Bache RJ, et al. Modeling regional myocardial flows from residue functions of an intravascular indicator. Am J Physiol-Heart Circ Physiol. (1996) 271:H1643–55. doi: 10.1152/ajpheart.1996.271.4.H1643
65. Hsu L-Y, Kellman P, Arai AE. Nonlinear myocardial signal intensity correction improves quantification of contrast-enhanced first-pass MR perfusion in humans. J Magn Reson Imaging JMRI. (2008) 27:793–801. doi: 10.1002/jmri.21286
66. Hsu L-Y, Rhoads KL, Holly JE, Kellman P, Aletras AH, Arai AE. Quantitative myocardial perfusion analysis with a dual-bolus contrast-enhanced first-pass MRI technique in humans. J Magn Reson Imaging JMRI. (2006) 23:315–22. doi: 10.1002/jmri.20502
67. Utz W, Greiser A, Niendorf T, Dietz R, Schulz-Menger J. Single- or dual-bolus approach for the assessment of myocardial perfusion reserve in quantitative MR perfusion imaging. Magn Reson Med. (2008) 59:1373–7. doi: 10.1002/mrm.21611
68. Köstler H, Ritter C, Lipp M, Beer M, Hahn D, Sandstede J. Prebolus quantitative MR heart perfusion imaging. Magn Reson Med. (2004) 52:296–9. doi: 10.1002/mrm.20160
69. Ishida M, Schuster A, Morton G, Chiribiri A, Hussain S, Paul M, et al. Development of a universal dual-bolus injection scheme for the quantitative assessment of myocardial perfusion cardiovascular magnetic resonance. J Cardiovasc Magn Reson. (2011) 13:28. doi: 10.1186/1532-429X-13-28
70. Gatehouse PD, Elkington AG, Ablitt NA, Yang G-Z, Pennell DJ, Firmin DN. Accurate assessment of the arterial input function during high-dose myocardial perfusion cardiovascular magnetic resonance. J Magn Reson Imaging JMRI. (2004) 20:39–45. doi: 10.1002/jmri.20054
71. Kim D, Axel L. Multislice, dual-imaging sequence for increasing the dynamic range of the contrast-enhanced blood signal and CNR of myocardial enhancement at 3T. J Magn Reson Imaging. (2006) 23:81–6. doi: 10.1002/jmri.20471
72. Gatehouse P, Lyne J, Smith G, Pennell D, Firmin D. T2* effects in the dual-sequence method for high-dose first-pass myocardial perfusion. J Magn Reson Imaging. (2006) 24:1168–71. doi: 10.1002/jmri.20746
73. Sourbron SP, Buckley DL. Tracer kinetic modelling in MRI: estimating perfusion and capillary permeability. Phys Med Biol. (2012) 57:R1–R33. doi: 10.1088/0031-9155/57/2/R1
74. Rutland MD. Origin of the Patlak-Rutland plot. Nucl Med Commun. (1996) 17:441. doi: 10.1097/00006231-199605000-00015
75. Ichihara T, Ishida M, Kitagawa K, Ichikawa Y, Natsume T, Yamaki N, et al. Quantitative analysis of first-pass contrast-enhanced myocardial perfusion MRI using a Patlak plot method and blood saturation correction. Magn Reson Med. (2009) 62:373–83. doi: 10.1002/mrm.22018
76. Tong CY, Prato FS, Wisenberg G, Lee TY, Carroll E, Sandler D, et al. Measurement of the extraction efficiency and distribution volume for Gd-DTPA in normal and diseased canine myocardium. Magn Reson Med. (1993) 30:337–46. doi: 10.1002/mrm.1910300310
77. Tong CY, Prato FS, Wisenberg G, Lee TY, Carroll E, Sandler D, et al. Techniques for the measurement of the local myocardial extraction efficiency for inert diffusible contrast agents such as gadopentate dimeglumine. Magn Reson Med. (1993) 30:332–6. doi: 10.1002/mrm.1910300309
78. Diesbourg LD, Prato FS, Wisenberg G, Drost DJ, Marshall TP, Carroll SE, et al. Quantification of myocardial blood flow and extracellular volumes using a bolus injection of Gd-DTPA: kinetic modeling in canine ischemic disease. Magn Reson Med. (1992) 23:239–53. doi: 10.1002/mrm.1910230205
79. Pack NA, DiBella EVR. Comparison of myocardial perfusion estimates from dynamic contrast-enhanced magnetic resonance imaging with four quantitative analysis methods. Magn Reson Med. (2010) 64:125–37. doi: 10.1002/mrm.22282
80. Zarinabad N, Chiribiri A, Hautvast GLTF, Ishida M, Schuster A, Cvetkovic Z, et al. Voxel-wise quantification of myocardial perfusion by cardiac magnetic resonance. Feasibility and methods comparison. Magn Reson Med. (2012) 68:1994–2004. doi: 10.1002/mrm.24195
81. Wilke N, Jerosch-Herold M, Wang Y, Huang Y, Christensen BV, Stillman AE, et al. Myocardial perfusion reserve: assessment with multisection, quantitative, first-pass MR imaging. Radiology. (1997) 204:373–84. doi: 10.1148/radiology.204.2.9240523
82. Jerosch-Herold M, Swingen C, Seethamraju RT. Myocardial blood flow quantification with MRI by model-independent deconvolution. Med Phys. (2002) 29:886–97. doi: 10.1118/1.1473135
83. Keeling SL, Kogler T, Stollberger R. Deconvolution for DCE-MRI using an exponential approximation basis. Med Image Anal. (2009) 13:80–90. doi: 10.1016/j.media.2008.06.011
84. Hautvast G, Chiribiri A, Zarinabad N, Schuster A, Breeuwer M, Nagel E. Myocardial blood flow quantification from MRI by deconvolution using an exponential approximation basis. IEEE Trans Biomed Eng. (2012) 59:2060–7. doi: 10.1109/TBME.2012.2197620
85. McDiarmid AK, Ripley DP, Mohee K, Kozerke S, Greenwood JP, Plein S, et al. Three-dimensional whole-heart vs. two-dimensional high-resolution perfusion-CMR: a pilot study comparing myocardial ischaemic burden. Eur Heart J Cardiovasc Imaging. (2016) 17:900–8. doi: 10.1093/ehjci/jev231
86. Motwani M, Kidambi A, Sourbron S, Fairbairn TA, Uddin A, Kozerke S, et al. Quantitative three-dimensional cardiovascular magnetic resonance myocardial perfusion imaging in systole and diastole. J Cardiovasc Magn Reson. (2014) 16:19. doi: 10.1186/1532-429X-16-19
87. Jogiya R, Kozerke S, Morton G, Silva KD, Redwood S, Perera D, et al. Validation of dynamic 3-dimensional whole heart magnetic resonance myocardial perfusion imaging against fractional flow reserve for the detection of significant coronary artery disease. J Am Coll Cardiol. (2012) 60:756–65. doi: 10.1016/j.jacc.2012.02.075
88. Wissmann L, Niemann M, Gotschy A, Manka R, Kozerke S. Quantitative three-dimensional myocardial perfusion cardiovascular magnetic resonance with accurate two-dimensional arterial input function assessment. J Cardiovasc Magn Reson. (2015) 17:108. doi: 10.1186/s12968-015-0212-3
89. Hautvast GLTF, Chiribiri A, Lockie T, Breeuwer M, Nagel E, Plein S. Quantitative analysis of transmural gradients in myocardial perfusion magnetic resonance images. Magn Reson Med. (2011) 66:1477–87. doi: 10.1002/mrm.22930
90. Chiribiri A, Hautvast GLTF, Lockie T, Schuster A, Bigalke B, Olivotti L, et al. Assessment of coronary artery stenosis severity and location: quantitative analysis of transmural perfusion gradients by high-resolution MRI versus FFR. JACC Cardiovasc Imaging. (2013) 6:600–9. doi: 10.1016/j.jcmg.2012.09.019
91. Chiribiri A, Villa ADM, Sammut E, Breeuwer M, Nagel E. Perfusion dyssynchrony analysis. Eur Heart J Cardiovasc Imaging. (2016) 17:1414–23. doi: 10.1093/ehjci/jev326
92. Mori S, Frederiksen K, van Zijl PCM, Stieltjes B, Kraut MA, Solaiyappan M, et al. Brain white matter anatomy of tumor patients evaluated with diffusion tensor imaging. Ann Neurol. (2002) 51:377–80. doi: 10.1002/ana.10137
93. Parmar H, Sitoh Y-Y, Yeo TT. Combined magnetic resonance tractography and functional magnetic resonance imaging in evaluation of brain tumors involving the motor system. J Comput Assist Tomogr. (2004) 28:551–6. doi: 10.1097/00004728-200407000-00019
94. McGill L-A, Ismail TF, Nielles-Vallespin S, Ferreira P, Scott AD, Roughton M, et al. Reproducibility of in-vivo diffusion tensor cardiovascular magnetic resonance in hypertrophic cardiomyopathy. J Cardiovasc Magn Reson. (2012) 14:86. doi: 10.1186/1532-429X-14-86
95. Grant RP. NOTES ON THE MUSCULAR ARCHITECTURE OF THE LEFT VENTRICLE. Circulation. (1965) 32:301–8. doi: 10.1161/01.CIR.32.2.301
96. Smerup M, Nielsen E, Agger P, Frandsen J, Vestergaard-Poulsen P, Andersen J, et al. The three-dimensional arrangement of the myocytes aggregated together within the mammalian ventricular myocardium. Anat Rec Hoboken NJ. (2007) (2009) 292:1–11. doi: 10.1002/ar.20798
97. Streeter DD Jr, Spotnitz HM, Patel DP, Ross J Jr, Sonnenblick EH. Fiber orientation in the canine left ventricle during diastole and systole. Circ Res. (1969) 24:339–47. doi: 10.1161/01.RES.24.3.339
98. Hales PW, Schneider JE, Burton RAB, Wright BJ, Bollensdorff C, Kohl P. Histo-anatomical structure of the living isolated rat heart in two contraction states assessed by diffusion tensor MRI. Prog Biophys Mol Biol. (2012) 110:319–30. doi: 10.1016/j.pbiomolbio.2012.07.014
99. Hsu EW, Muzikant AL, Matulevicius SA, Penland RC, Henriquez CS. Magnetic resonance myocardial fiber-orientation mapping with direct histological correlation. Am J Physiol. (1998) 274:H1627–H34. doi: 10.1152/ajpheart.1998.274.5.H1627
100. Schmid P, Jaermann T, Boesiger P, Niederer PF, Lunkenheimer PP, Cryer CW, et al. Ventricular myocardial architecture as visualised in postmortem swine hearts using magnetic resonance diffusion tensor imaging. Eur J Cardiothorac Surg. (2005) 27:468–72. doi: 10.1016/j.ejcts.2004.11.036
101. Mekkaoui C, Huang S, Chen HH, Dai G, Reese TG, Kostis WJ, et al. Fiber architecture in remodeled myocardium revealed with a quantitative diffusion CMR tractography framework and histological validation. J Cardiovasc Magn Reson. (2012) 14:70. doi: 10.1186/1532-429X-14-70
102. Chen J, Song S-K, Liu W, McLean M, Allen JS, Tan J, et al. Remodeling of cardiac fiber structure after infarction in rats quantified with diffusion tensor MRI. Am J Physiol Heart Circ Physiol. (2003) 285:H946–954. doi: 10.1152/ajpheart.00889.2002
103. Chen J, Liu W, Zhang H, Lacy L, Yang X, Song S-K, et al. Regional ventricular wall thickening reflects changes in cardiac fiber and sheet structure during contraction: quantification with diffusion tensor MRI. Am J Physiol-Heart Circ Physiol. (2005) 289:H1898–907. doi: 10.1152/ajpheart.00041.2005
104. Costa KD, Takayama Y, McCulloch AD, Covell JW. Laminar fiber architecture and three-dimensional systolic mechanics in canine ventricular myocardium. Am J Physiol. (1999) 276:H595–607. doi: 10.1152/ajpheart.1999.276.2.H595
105. Dou J, Reese TG, Tseng W-YI, Wedeen VJ. Cardiac diffusion MRI without motion effects. Magn Reson Med. (2002) 48:105–14. doi: 10.1002/mrm.10188
106. Tseng WY, Reese TG, Weisskoff RM, Wedeen VJ. Cardiac diffusion tensor MRI in vivo without strain correction. Magn Reson Med. (1999) 42:393–403. doi: 10.1002/(SICI)1522-2594(199908)42:2<393::AID-MRM22>3.0.CO;2-F
107. Stoeck CT, Kalinowska A, Deuster C von, Harmer J, Chan RW, Niemann M, et al. Dual-phase cardiac diffusion tensor imaging with strain correction. PLoS One. (2014) 9:e107159. doi: 10.1371/journal.pone.0107159
108. McGill L-A, Scott AD, Ferreira PF, Nielles-Vallespin S, Ismail T, Kilner PJ, et al. Heterogeneity of fractional anisotropy and mean diffusivity measurements by in vivo diffusion tensor imaging in normal human hearts. PLOS ONE. (2015) 10:e0132360. doi: 10.1371/journal.pone.0132360
109. Reese TG, Weisskoff RM, Smith RN, Rosen BR, Dinsmore RE, Wedeen VJ. Imaging myocardial fiber architecture in vivo with magnetic resonance. Magn Reson Med. (1995) 34:786–91. doi: 10.1002/mrm.1910340603
110. Gamper U, Boesiger P, Kozerke S. Diffusion imaging of the in vivo heart using spin echoes–considerations on bulk motion sensitivity. Magn Reson Med. (2007) 57:331–7. doi: 10.1002/mrm.21127
111. Nielles-Vallespin S, Mekkaoui C, Gatehouse P, Reese TG, Keegan J, Ferreira PF, et al. In vivo diffusion tensor MRI of the human heart: reproducibility of breath-hold and navigator-based approaches. Magn Reson Med. (2013) 70:454–65. doi: 10.1002/mrm.24488
112. Ferreira PF, Kilner PJ, McGill L-A, Nielles-Vallespin S, Scott AD, Ho SY, et al. In vivo cardiovascular magnetic resonance diffusion tensor imaging shows evidence of abnormal myocardial laminar orientations and mobility in hypertrophic cardiomyopathy. J Cardiovasc Magn Reson. (2014) 16:87. doi: 10.1186/s12968-014-0087-8
113. Nielles-Vallespin S, Khalique Z, Ferreira PF, de Silva R, Scott AD, Kilner P, et al. Assessment of myocardial microstructural dynamics by in vivo diffusion tensor cardiac magnetic resonance. J Am Coll Cardiol. (2017) 69:661–76. doi: 10.1016/j.jacc.2016.11.051
114. Gotschy A, von Deuster C, van Gorkum RJH, Gastl M, Vintschger E, Schwotzer R, et al. Characterizing cardiac involvement in amyloidosis using cardiovascular magnetic resonance diffusion tensor imaging. J Cardiovasc Magn Reson. (2019) 21:56. doi: 10.1186/s12968-019-0563-2
115. Wu M-T, Tseng W-YI, Su M-YM, Liu C-P, Chiou K-R, Wedeen VJ, et al. Diffusion tensor magnetic resonance imaging mapping the fiber architecture remodeling in human myocardium after infarction: correlation with viability and wall motion. Circulation. (2006) 114:1036–45. doi: 10.1161/CIRCULATIONAHA.105.545863
116. Tseng W-YI, Dou J, Reese TG, Wedeen VJ. Imaging myocardial fiber disarray and intramural strain hypokinesis in hypertrophic cardiomyopathy with MRI. J Magn Reson Imaging JMRI. (2006) 23:1–8. doi: 10.1002/jmri.20473
117. von Deuster Constantin, Sammut Eva, Asner Liya, Nordsletten David, Lamata Pablo, Stoeck Christian T., et al. Studying dynamic myofiber aggregate reorientation in dilated cardiomyopathy using in vivo magnetic resonance diffusion tensor imaging. Circ Cardiovasc Imaging. (2016) 9:e005018. doi: 10.1161/CIRCIMAGING.116.005018
118. Mekkaoui C, Jackowski MP, Kostis WJ, Stoeck CT, Thiagalingam A, Reese TG, et al. Myocardial scar delineation using diffusion tensor magnetic resonance tractography. J Am Heart Assoc. (2018) 7:e007834. doi: 10.1161/JAHA.117.007834
119. Pashakhanloo F, Herzka DA, Mori S, Zviman M, Halperin H, Gai N, et al. Submillimeter diffusion tensor imaging and late gadolinium enhancement cardiovascular magnetic resonance of chronic myocardial infarction. J Cardiovasc Magn Reson. (2017) 19:9. doi: 10.1186/s12968-016-0317-3
120. Lohr D, Terekhov M, Weng AM, Schroeder A, Walles H, Schreiber LM. Spin echo based cardiac diffusion imaging at 7T: an ex vivo study of the porcine heart at 7T and 3T. PLoS One. (2019) 14:e0213994. doi: 10.1371/journal.pone.0213994
121. van der Geest RJ, Garg P. Advanced analysis techniques for intra-cardiac flow evaluation from 4D flow MRI. Curr Radiol Rep. (2016) 4:38. doi: 10.1007/s40134-016-0167-7
122. Taylor TW, Yamaguchi T. Flow patterns in three-dimensional left ventricular systolic and diastolic flows determined from computational fluid dynamics. Biorheology. (1995) 32:61–71. doi: 10.3233/BIR-1995-32105
123. Garg P, Westenberg JJM, van den Boogaard PJ, Swoboda PP, Aziz R, Foley JRJ, et al. Comparison of fast acquisition strategies in whole-heart four-dimensional flow cardiac MR: two-center, 1.5 Tesla, phantom and in vivo validation study. J Magn Reson Imaging. (2017) 47:272–81. doi: 10.1002/jmri.25746
124. Zhang J-M, Tan RS, Zhang S, Geest R van der, Garg P, Leong BR, et al. Comparison of image acquisition techniques in four-dimensional flow cardiovascular MR on 3 tesla in volunteers and tetralogy of fallot patients. Annu Int Conf IEEE Eng Med Biol Soc. (2018) 2018:1115–8. doi: 10.1109/EMBC.2018.8512412
125. Bock J, Töger J, Bidhult S, Markenroth Bloch K, Arvidsson P, Kanski M, et al. Validation and reproducibility of cardiovascular 4D-flow MRI from two vendors using 2 × 2 parallel imaging acceleration in pulsatile flow phantom and in vivo with and without respiratory gating. Acta Radiol Stockh Swed. (1987) (2019) 60:327–37. doi: 10.1177/0284185118784981
126. Hope MD, Hope TA, Meadows AK, Ordovas KG, Urbania TH, Alley MT, et al. Bicuspid aortic valve: four-dimensional MR evaluation of ascending aortic systolic flow patterns. Radiology. (2010) 255:53–61. doi: 10.1148/radiol.09091437
127. Rodríguez-Palomares JF, Dux-Santoy L, Guala A, Kale R, Maldonado G, Teixidó-Turà G, et al. Aortic flow patterns and wall shear stress maps by 4D-flow cardiovascular magnetic resonance in the assessment of aortic dilatation in bicuspid aortic valve disease. J Cardiovasc Magn Reson. (2018) 20:28. doi: 10.1186/s12968-018-0451-1
128. Elbaz MSM, Calkoen EE, Westenberg JJM, Lelieveldt BPF, Roest AAW, van der Geest RJ. Vortex flow during early and late left ventricular filling in normal subjects: quantitative characterization using retrospectively-gated 4D flow cardiovascular magnetic resonance and three-dimensional vortex core analysis. J Cardiovasc Magn Reson. (2014) 16:78. doi: 10.1186/s12968-014-0078-9
129. Calkoen EE, Elbaz MSM, Westenberg JJM, Kroft LJM, Hazekamp MG, Roest AAW, et al. Altered left ventricular vortex ring formation by 4-dimensional flow magnetic resonance imaging after repair of atrioventricular septal defects. J Thorac Cardiovasc Surg. (2015) 150:1233–40.e1. doi: 10.1016/j.jtcvs.2015.07.048
130. Schäfer M, Barker AJ, Kheyfets V, Stenmark KR, Crapo J, Yeager ME, et al. Helicity and vorticity of pulmonary arterial flow in patients with pulmonary hypertension: quantitative analysis of flow formations. J Am Heart Assoc. (2017) 6:e007010. doi: 10.1161/JAHA.117.007010
131. Reiter G, Reiter U, Kovacs G, Kainz B, Schmidt K, Maier R, et al. Magnetic resonance-derived 3-dimensional blood flow patterns in the main pulmonary artery as a marker of pulmonary hypertension and a measure of elevated mean pulmonary arterial pressure. Circ Cardiovasc Imaging. (2008) 1:23–30. doi: 10.1161/CIRCIMAGING.108.780247
132. Bürk J, Blanke P, Stankovic Z, Barker A, Russe M, Geiger J, et al. Evaluation of 3D blood flow patterns and wall shear stress in the normal and dilated thoracic aorta using flow-sensitive 4D CMR. J Cardiovasc Magn Reson. (2012) 14:84. doi: 10.1186/1532-429X-14-84
133. Bissell MM, Hess AT, Biasiolli L, Glaze SJ, Loudon M, Pitcher A, et al. Aortic dilation in bicuspid aortic valve disease: flow pattern is a major contributor and differs with valve fusion type. Circ Cardiovasc Imaging. (2013) 6:499–507. doi: 10.1161/CIRCIMAGING.113.000528
134. Crandon S, Elbaz MSM, Westenberg JJM, van der Geest RJ, Plein S, Garg P. Clinical applications of intra-cardiac four-dimensional flow cardiovascular magnetic resonance: a systematic review. Int J Cardiol. (2017) 249:486–93. doi: 10.1016/j.ijcard.2017.07.023
135. Barker N, Fidock B, Johns CS, Kaur H, Archer G, Rajaram S, et al. A systematic review of right ventricular diastolic assessment by 4D flow CMR. BioMed Res Int. (2019) 2019:6074984. doi: 10.1155/2019/6074984
136. Fidock B, Barker N, Balasubramanian N, Archer G, Fent G, Al-Mohammad A, et al. A systematic review of 4D-flow MRI derived mitral regurgitation quantification methods. Front Cardiovasc Med. (2019) 6:103. doi: 10.3389/fcvm.2019.00103
137. Crandon S, Westenberg JJM, Swoboda PP, Fent GJ, Foley JRJ, Chew PG, et al. Impact of age and diastolic function on novel, 4D flow CMR biomarkers of left ventricular blood flow kinetic energy. Sci Rep. (2018) 8:14436. doi: 10.1038/s41598-018-32707-5
138. Kamphuis VP, van der Palen RLF, de Koning PJH, Elbaz MSM, van der Geest RJ, de Roos A, et al. In-scan and scan-rescan assessment of LV in- and outflow volumes by 4D flow MRI versus 2D planimetry: reproducibility of LV in- and outflow from MRI. J Magn Reson Imaging. (2018) 47:511–22. doi: 10.1002/jmri.25792
139. Dyverfeldt P, Bissell M, Barker AJ, Bolger AF, Carlhäll C-J, Ebbers T, et al. 4D flow cardiovascular magnetic resonance consensus statement. J Cardiovasc Magn Reson. (2015) 17:72. doi: 10.1186/s12968-015-0174-5
140. Zhong L, Schrauben EM, Garcia J, Uribe S, Grieve SM, Elbaz MSM, et al. Intracardiac 4D flow MRI in congenital heart disease: recommendations on behalf of the ISMRM flow & motion study group. J Magn Reson Imaging JMRI. (2019) 50:677–81. doi: 10.1002/jmri.26893
141. Garg P, Swift AJ, Zhong L, Carlhäll C-J, Ebbers T, Westenberg J, et al. Assessment of mitral valve regurgitation by cardiovascular magnetic resonance imaging. Nat Rev Cardiol. (2019) 17:298–312. doi: 10.1038/s41569-019-0305-z
142. Feneis JF, Kyubwa E, Atianzar K, Cheng JY, Alley MT, Vasanawala SS, et al. 4D flow MRI quantification of mitral tricuspid regurgitation: reproducibility consistency relative to conventional MRI. J Magn Reson Imaging JMRI. (2018) 48:1147–58. doi: 10.1002/jmri.26040
143. Kamphuis VP, Roest AAW, Ajmone Marsan N, van den Boogaard PJ, Kroft LJM, Aben J-P, et al. Automated cardiac valve tracking for flow quantification with four-dimensional flow MRI. Radiology. (2019) 290:70–8. doi: 10.1148/radiol.2018180807
144. Garg P, Crandon S, Swoboda PP, Fent GJ, Foley JRJ, Chew PG, et al. Left ventricular blood flow kinetic energy after myocardial infarction - insights from 4D flow cardiovascular magnetic resonance. J Cardiovasc Magn Reson. (2018) 20:61. doi: 10.1186/s12968-018-0483-6
Keywords: cardiovascular magnetic resonance, diffusion tensor imaging, tissue characterisation, myocardial fibrosis, four-dimensional flow imaging
Citation: Paddock S, Tsampasian V, Assadi H, Mota BC, Swift AJ, Chowdhary A, Swoboda P, Levelt E, Sammut E, Dastidar A, Broncano Cabrero J, Del Val JR, Malcolm P, Sun J, Ryding A, Sawh C, Greenwood R, Hewson D, Vassiliou V and Garg P (2021) Clinical Translation of Three-Dimensional Scar, Diffusion Tensor Imaging, Four-Dimensional Flow, and Quantitative Perfusion in Cardiac MRI: A Comprehensive Review. Front. Cardiovasc. Med. 8:682027. doi: 10.3389/fcvm.2021.682027
Received: 17 March 2021; Accepted: 04 June 2021;
Published: 07 July 2021.
Edited by:
Reza Nezafat, Harvard University, United StatesReviewed by:
Shiro Nakamori, Mie University, JapanEmmanuel Androulakis, Royal Brompton and Harefield NHS Foundation Trust, United Kingdom
Copyright © 2021 Paddock, Tsampasian, Assadi, Mota, Swift, Chowdhary, Swoboda, Levelt, Sammut, Dastidar, Broncano Cabrero, Del Val, Malcolm, Sun, Ryding, Sawh, Greenwood, Hewson, Vassiliou and Garg. This is an open-access article distributed under the terms of the Creative Commons Attribution License (CC BY). The use, distribution or reproduction in other forums is permitted, provided the original author(s) and the copyright owner(s) are credited and that the original publication in this journal is cited, in accordance with accepted academic practice. No use, distribution or reproduction is permitted which does not comply with these terms.
*Correspondence: Pankaj Garg, cC5nYXJnJiN4MDAwNDA7dWVhLmFjLnVr
†These authors share co-first authorship