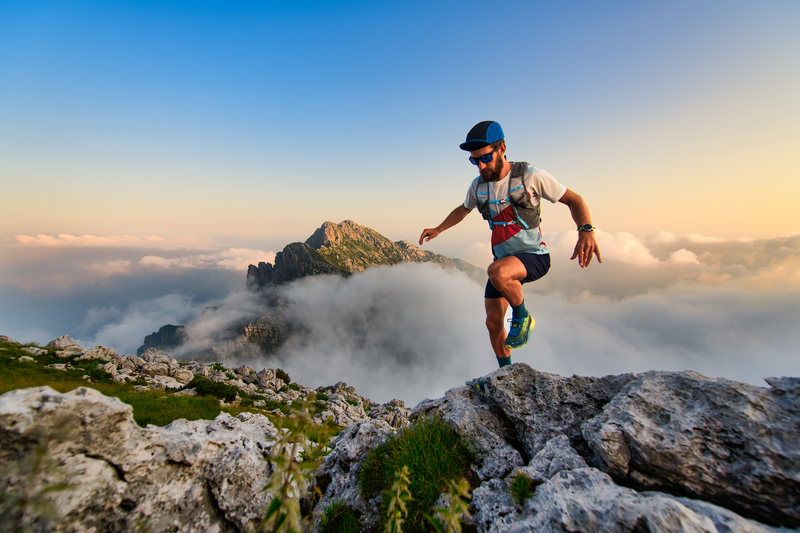
94% of researchers rate our articles as excellent or good
Learn more about the work of our research integrity team to safeguard the quality of each article we publish.
Find out more
REVIEW article
Front. Cardiovasc. Med. , 28 July 2021
Sec. Atherosclerosis and Vascular Medicine
Volume 8 - 2021 | https://doi.org/10.3389/fcvm.2021.681444
This article is part of the Research Topic Lipids and Inflammation in Health and Disease View all 26 articles
Despite great progress in the management of atherosclerosis (AS), its subsequent cardiovascular disease (CVD) remains the leading cause of morbidity and mortality. This is probably due to insufficient risk detection using routine lipid testing; thus, there is a need for more effective approaches relying on new biomarkers. Quantitative nuclear magnetic resonance (qNMR) metabolomics is able to phenotype holistic metabolic changes, with a unique advantage in regard to quantifying lipid-protein complexes. The rapidly increasing literature has indicated that qNMR-based lipoprotein particle number, particle size, lipid components, and some molecular metabolites can provide deeper insight into atherogenic diseases and could serve as novel promising determinants. Therefore, this article aims to offer an updated review of the qNMR biomarkers of AS and CVD found in epidemiological studies, with a special emphasis on lipoprotein-related parameters. As more researches are performed, we can envision more qNMR metabolite biomarkers being successfully translated into daily clinical practice to enhance the prevention, detection and intervention of atherosclerotic diseases.
Cardiovascular disease (CVD) remains the leading cause of morbidity and mortality around the world (1). Of the 55.95 million global deaths in 2017, 17.79 million (31.8%) were due to CVD (2). Atherosclerosis (AS), as the underlying pathogenesis, starts early in life and takes decades to develop into serious vascular occlusion, thus, effective detection and well-understanding of the diseases would be valuable for primary prevention (3).
Although definite mechanisms of atherogenic process continue to be intensively investigated, lipids or lipoproteins are known to play pivotal roles (4). Circulating low-density lipoprotein (LDL) and other ApoB-containing lipoproteins constitute a key driver of plaque formation through their interaction with monocyte-derived macrophages in the arterial intima. Due to the accumulation of retained lipoproteins, macrophage cells and extracellular matrix, the vascular lesions gradually enlarge and progress into more complex arterial plaques (5). Since dyslipidemia is a recognized risk factor for atherosclerosis, routine evaluation of lipoprotein level offers a valid contribution to stratify atherosclerotic risk (6). However, the standard lipid panel is not always sensitive and specific enough for the identification of patients at risk.
Low-density lipoprotein cholesterol (LDL-C) is a well-established risk factor and the primary intervention target (7–9). However, individuals who develop atherosclerotic diseases may have “optimal” LDL-C levels (10, 11), especially subjects with insulin resistance (12, 13). Additionally, patients who receive cholesterol-lowering treatment and reach satisfactory LDL-C targets still develop CVD (14). This is partly due to other nonlipid risk factors, such as hypertension, cigarette smoking, inactivity, obesity, and type 2 diabetes (T2DM). However, it is likely that other lipid or lipoprotein factors also play a part in the atherogenic process.
Many large epidemiological studies have supported that triglycerides (TGs) correlate with CVD risk (15–17), although the association is sometimes attenuated with adjustment for other traditional risk factors (18). However, increasing evidence now has highlighted the importance of triglyceride-rich lipoproteins (TRLs) and their remnants, both in fasting and nonfasting periods (19–22). Populations with mild-to-moderately elevated nonfasting TG levels have increased risks for ischemic stroke, myocardial infarction, ischemic heart disease, and all-cause mortality (23–25). Mendelian randomization studies also demonstrate that TRLs are causally associated with CVD and all-cause mortality (26). Thus, new insights strongly suggest that TRLs are independent CVD factors; however, the discrepancies in atherogenicity among their subclasses and lipid content are still an area of continuing investigation.
Additionally, there exists a widely held view that high-density lipoprotein cholesterol (HDL-C) levels causally relate to AS. Nevertheless, the EPOCH-JAPAN (Evidence for Cardiovascular Prevention from Observational Cohorts in Japan) study (27) and CANHEART (Cardiovascular Health in Ambulatory Care Research Team) study (28) both presented a U-shaped relationship between HDL-C and CVD mortality. In addition, elevated HDL-C from cholesterol ester transfer protein (CETP) inhibitors failed to lower CVD risk (29, 30), and gene variants altering HDL-C levels in Mendelian randomization studies were not necessarily associated with CVD (31). Instead, it has been proposed that indexes of HDL structure and function may better mediate the atheroprotective effects of HDL (32).
Hence, the standard metrics in routine lipid testing are only part of lipoprotein factors, which cannot account for all CVD risks. Lipoprotein is known to be a lipid-protein conglomerate possessing complex structure, composition and function (33). Chylomicron containing ApoB-48 is secreted from the intestine, whereas very low density lipoproteins (VLDL) containing ApoB-100 is synthesized in hepatocytes and then enters the systemic circulation. TGs in VLDL are hydrolyzed by lipoprotein lipase (LPL), resulting in small, cholesterol-enriched VLDL remnants and IDL (34). IDL particles can be further converted into LDL by hepatic lipase (HL), and both of them can be removed by the interaction of apolipoproteins with hepatic LDL receptor (LDLR) (35). The HDL metabolism begins with secretion of small discoidal protein-phospholipid complex mainly from liver. The nascent HDL removes cholesterol from peripheral tissues, and increasingly matures into a larger spherical HDL particle. In summary, the metabolic processes of lipoproteins are very complex, which involve dynamic changes of cholesterol, triglyceride, phospholipid and apolipoprotein in various lipoprotein subclasses (36). More detailed lipoprotein profiles based on advanced detection methods are necessary to identify new biomarkers of AS and its clinical manifestations (37–39).
Metabolomics is able to measure the global metabolic status in cells, tissues or biological fluids (40). Being downstream of biometabolic processes, the metabolome can filter out nonfunctional effects and greatly amplify small functional changes at the genetic or protein expression levels (41). Quantitative nuclear magnetic resonance (qNMR) metabolomics enables rapid, nondestructive, reproducible and high-throughput quantifications of lipoproteins, lipids and molecular metabolites with uncomplicated sample preparations (42). Since mass spectrometry (MS) cannot analyze protein-lipid complexes, qNMR metabolomics occupies a very important position in lipoprotein testing. It has also been demonstrated that qNMR lipoprotein detection is unaffected by frozen storage and multiple freeze-thaw cycles (43). Because of these advantages, qNMR metabolomics paves the way for large population-based studies and, therefore, provides a distinct perspective on atherosclerotic diseases (44, 45).
Over the past two decades, accumulating evidence has suggested that qNMR metabolomics can serve as a promising strategy to discover new biomarkers in atherosclerotic diseases (46, 47). The qNMR-based lipoproteins with differing sizes and densities have diverse vascular effects (48, 49). The profiling of metabolic status holds promise of unraveling the pathological mechanisms underlying atherosclerosis. In addition, qNMR metabolomics may better reflect atherogenesis than traditional lipid testing (48, 50, 51). The quantification of circulating metabolites identifies changes prior to the onset of overt disease, and hereby contributes to earlier and more accurate cardiovascular risk assessment.
Despite such a large number of studies in this field, no literature has systematically reviewed the qNMR-based metabolite biomarkers in detail. In this review, we intend to (1) describe the detection and analytical principle of qNMR metabolomics; (2) summarize the epidemiological findings that seek novel biomarkers for AS and CVD using qNMR metabolomics, focusing on lipoprotein and lipid; (3) discuss the significance of identifying qNMR biomarkers; (4) illustrate the current challenges and future directions in this field.
In 1983, Nicholson et al. (52, 53) pioneered the application of NMR spectroscopy to study multicomponent metabolic composition. NMR-based metabolomics identifies metabolites subjected to a magnetic field by characteristic chemical shifts in resonance frequency. Quantitative NMR metabolomics, also known as metabolite profiling, offers transparent biological information about metabolite identification and absolute quantitation (54). The most versatile and widely used method is 1H qNMR. Each molecule with hydrogen atoms gives a characteristic signal with a shape that is quantum mechanically distinctive, and the area is proportional to the concentration of the molecule (55, 56). Using standard equipment, qNMR can resolve the concentrations of target molecules down to the micromolar range. By enhancing or attenuating different signals with multiple pulse programs, qNMR is able to optimize large or small molecules (57). Individual molecular signals may overlap, but current linefitting methods relying on molecule-specific model lineshapes and regression modeling can robustly handle part of the overlapping information (58, 59).
It is also widely known that qNMR is suitable for detecting and quantifying lipoproteins because the chemical shifts fundamentally reflect the physical structure of the particle (60, 61). Lipoproteins give rise to a series of broad peaks in spectral regions that are superimposed on the very broad envelope from proteins such as albumin and the sharp peaks of small-molecule metabolites (62). Among them, the shapes of two peaks, i.e., the terminal CH3 group peak and the long-chain (CH2)n peak of the fatty acyl groups, can reflect the differences derived from lipid chemical heterogeneity and lipoprotein particle size (63). According to the size of the particle, the lipid methyl and methylene moieties in lipoproteins resonate at different frequencies, with smaller particles resonating at lower frequencies. Therefore, the concentrations of lipoproteins can be quantitated when decomposing the methyl and methylene signals of the core lipids into individual signals or using statistical methods to estimate the entire envelope (64).
To further determine lipoprotein parameters, research teams have developed various fitting models to cover different lipoprotein subfractions categorized by size or density. The research team led by Otvos first developed lipoprotein quantification in 1991 (65); they detected the particle numbers and sizes of large, medium, and small subclasses of VLDL, LDL, and HDL separately. The group then created a simplified LipoProfile® panel, which has been commercially available from LipoScience Inc. (Raleigh, North Carolina) since 1997 (66). The Finnish research team led by Ala-Korpela determined the particle numbers and sizes of 14 lipoproteins (CM, 5 VLDL, 1 IDL, 3 LDL, and 4 HDL) (67). A Spanish research team developed a Liposcale panel, which was reported to be more accurate than the LipoProfile (68). The spectrometer manufacturer company Bruker BioSpin also offers lipoprotein analysis. The latest method is capable of quantifying over 100 parameters, including 16 lipoprotein subclasses (5 VLDL, 1 IDL, 6 LDL, and 4 HDL) and their compositional components (total cholesterol, free cholesterol, triglyceride, phospholipid, apolipoprotein etc.) (62). It also reports 41 low-molecular-weight metabolites in units of mole, mildly extending the metabolic profiles.
Herein, drawing on discoveries in population-based studies, we describe the qNMR biomarkers from a perspective of three main categories of lipoproteins (i.e., LDL, VLDL, and HDL), along with some molecular metabolites. We provide a tabular overview of qNMR-based potential lipoprotein biomarkers (Table 1). We also summarize the epidemiological studies of biomarker discovery in subclinical atherosclerosis (Supplementary Table 1) and cardiovascular disease (Supplementary Table 2) using qNMR metabolomics in recent 5 years.
Table 1. Lipoprotein biomarkers of atherosclerotic diseases identified in epidemiological studies using quantitative NMR metabolomics.
The LDL-C in standard lipid panel is calculated from the Friedewald equation which is easily influenced by hypertriglyceridemia (69), but qNMR provides quantitative data directly. In addition, the LDL-C only refers to cholesterol in LDL, while qNMR detects various LDL-related measures such as particle number, subclasses, particle size, and lipid components.
There has been agreement that LDL particle number (LDL-P) is strongly related to atherosclerotic diseases. In 2,888 individuals from the EPIC (European Prospective Investigation into Cancer and Nutrition)-Norfolk study, LDL-P correlated with coronary artery disease (CAD), even after adjusting for the Framingham risk score and LDL-C (70). Toth et al. (71) showed that patients undergoing LDL-P measurement were more likely to receive intensive lipid-lowering therapy and had lower CVD risk than those in the LDL-C cohort. In fact, LDL-C and LDL-P may be discordant in some cases, exerting an additional risk (72–74). In the MESA (Multi-Ethnic Study of Atherosclerosis) study, Otvos et al. (72) identified 5,598 participants with discordant LDL-P and LDL-C. The carotid intima-media thickness (cIMT) was highest in subjects with raised LDL-P but normal LDL-C. Among 27,533 initially healthy women that were followed up for 17.2 years in another study (74), a similar result was observed, namely, coronary risk was underestimated for women with such discordance. Therefore, as described in the National Lipid Association (NLA) consensus statement (75), LDL-P is a “reasonable measure” in the estimation and management of CVD risk and may be a potential intervention target.
In regard to LDL subclasses, it is well-established that small dense LDLs (sdLDLs) are more atherogenic (76–79). In fact, the necessity of monitoring the sdLDL concentration has been stressed by many guidelines, such as the 2016 Chinese guidelines (80) and 2019 ESC/EAS guidelines (81). However, some researchers regard the large LDL subclass to be as important as sdLDL. Mora et al. (82) found that failure to adjust the strong inverse association between sdLDL and large LDL might conceal the atherogenic effect of large LDL. Likewise, Otvos et al. (83) demonstrated that both LDL subclasses were significantly related to coronary events once their correlation was taken into account. Moreover, it is worth noting that LDL particle size (LDL-S) in nanometers (nm) reflects similar but weaker information compared to the LDL subclass distribution. Specifically, although LDL-S was shown to be negatively associated with atherosclerotic diseases in some studies (84, 85), the relationship was not sustained in other studies or lost its statistical significance when adjusting for other lipid parameters (82, 86).
With regard to lipid components in LDL, most of the current studies targeting triglycerides in LDL (LDL-TG) use other methods, such as the enzyme-linked immunosorbent assay (ELISA) kits and automated homogeneous methods (87, 88). For example, Saeed et al. (89) examined plasma LDL-TG levels with automated homogeneity and discovered that LDL-TG independently correlated with the incidence of CVD events. In 4,381 patients with established CAD, high LDL-TG was associated with worse cardiovascular outcomes, suggesting its utility among secondary prevention populations (90). Likewise, Tzoulaki et al. (91) assessed metabolic profiling among 3,867 MESA participants based on qNMR metabolomics. The results indicated that TGs in total and large (density 1.019–1.031 kg/L) LDL were related to cIMT and CVD events (P < 0.005) when adjusting for other risk factors. Nevertheless, Albers et al. (92) failed to prove the correlation between LDL-TG and CVD events in the AIM-HIGH (Atherothrombosis Intervention in Metabolic Syndrome with Low HDL/High Triglycerides and Impact on Global Health Outcomes) trial. Thus, more studies using qNMR to precisely quantify LDL-TG levels are needed to explore its atherogenicity.
Although the mechanisms underlying the atherosclerotic process that are secondary to dyslipidemia remain largely unclear, the adverse effects of LDL have been partially identified. Circulating LDLs penetrate endothelial cells and enter the intimal space, especially in the context of hypercholesterolemia (93, 94). After being exposed to oxidative injury by free radical species, modified LDL can then be engulfed by monocyte-derived macrophages through receptor-mediated endocytosis and phagocytosis (95, 96). Macrophages subsequently turn into foam cells engorged with large amounts of cholesteryl esters, which give rise to the initiation of vascular lesions and the formation of fatty streaks (97). Regarding lipid components in LDL, it is interesting to note that TG can be catabolized by macrophages, which is different from cholesterol (98). LDL-TG may not directly lead to plaque formation, but may still potentiate atherogenesis. Elevated LDL-TG correlated with inflammatory markers such as C-reactive protein, white blood cell count, amyloid-A, fibrinogen, and interleukin-6 (89, 99) and was negatively associated with adiponectin levels, which have anti-inflammatory and cardioprotective effects (100). Therefore, chronic low-grade inflammation may be the link between LDL-TG and atherosclerosis, and more possible mechanisms remain under active investigation.
Increasing attentions have been focused on TG-rich lipoproteins, which consist of VLDL, IDL in the fasting state, and plus chylomicron (CM) in the postprandial state (101–103). Since CM particles may generate NMR spectral interference, qNMR detections usually avoid nonfasting serum or plasma, we thereby discuss the fasting TRLs, namely, VLDL and IDL in this part.
The first thing to mention is the concentrations of VLDL subclasses. Early in 1998, Freedman et al. (104) found that large (diameter 60–100 nm) VLDL was positively associated with CAD independent of age and standard lipid measurements. Four years later, Mackey et al. (105) reported that the level of large (diameter 60–200 nm) VLDL was associated with higher coronary calcification (CAC) after multivariable adjustments. However, studies performed in the JUPITER (Justification for the Use of Statins in Prevention) trial seemed to endorse the smaller VLDL subclasses. In 11,984 participants with a baseline LDL-C lower than 3.36 mmol/l (130 mg/dl), each standard deviation (SD) increase in the concentration of small (diameter 29–42 nm) VLDLs resulted in a 68% increase in residual CVD risk (106). However, statin-induced changes in large (diameter > 60 nm) or medium (diameter 42–60 nm) VLDL particles caused no risk reduction (107). In addition to the two opposite views, some studies considered all VLDL subclasses to have adverse effects. Mora et al. (108) found that total VLDL and its subclasses, as well as IDL, all independently predicted the incidence of CVD events in 27,673 women that were followed up for over 11 years. In 4,662 individuals from the CKB (China Kadoorie Biobank) cohort, the adjusted odds ratio (OR) for myocardial infarction ranged from 1.18 (1.07–1.29) for extremely large VLDL to 1.30 (1.19–1.44) for small VLDL, including IDL in the interval (109).
The second point that needs to be discussed is lipid components in VLDL and IDL. In recent years, cholesterol in TRL remnants (RLP-C), mainly small VLDL-C, has been proven to play a crucial role in residual CVD risk. Lawler et al. (107) observed a dose-dependent relationship between a decrease in VLDL-C and the residual risk reduction. In addition to VLDL-C, VLDL-TG is also an index of great concern but is under debate. In 25,480 Copenhagen subjects, Balling et al. (110) discovered that VLDL-TG did not account for MI risk, whereas VLDL-C explained 50% of the risk among ApoB-containing lipoproteins. However, for the same CVD endpoint, Holmes et al. (109) found that TG in all VLDL subclasses (diameter from 31.3 to >75 nm) had strong positive correlations with MI. Similarly, Tzoulaki et al. (91) demonstrated that nearly all VLDL-TG correlated with cIMT and TG in total and that the largest VLDL was related to CVD after multivariable adjustments (P < 0.005). Hence, VLDL-TG is very likely to take part in the atherogenic process and should be considered seriously in future studies.
Despite belonging to ApoB-containing lipoproteins, large VLDL, owing to the size limitation of transcytotic vesicles, cannot traverse the endothelium and exert atherogenic effects like LDL (111). However, some studies considered elevated large VLDL might reflect postprandial dyslipidemia, which is characterized by delayed chylomicron clearance and prolonged atherogenic lipoprotein retention (105). In addition, basic studies suggested that small RLP particles, which contain 5–20 times more cholesterol than LDL, had a greater affinity for subendothelial components. The TRL remnants could be taken up by macrophages directly without modification, thus promoting rapid cholesterol accumulation in lesional macrophages (22, 112). TRL remnants are also proposed to exacerbate the atherosclerotic process by inducing the secretion of TNF-α and IL-1β (113), activating the coagulation cascade (114), impairing endothelium-dependent vasodilation (115), and increasing oxidative stress (116). Except for its own pathogenicity, elevated VLDL under insulin resistance can also initiate lipoprotein destabilization (117). Specifically, excess VLDL particles undergo deficient TG lipolysis and poor hepatic uptake, which results in increased RLP particles. Afterwards, VLDL particles and remnants promote TG transfer to LDL and HDL by CETP. The latter two particles consequently become TG-enriched and can be further hydrolyzed into more atherogenic sdLDL and small TG-depleted, TC-depleted HDL (118). The whole pathological disturbances alter the lipoprotein profile towards an atherogenic form. In summary, TRL subclasses and their lipid composition promote endothelial dysfunction, but further research is still needed to identify the underlying mechanisms.
Since HDL is not simply a carrier of cholesterol but a complex particle with physiological heterogeneity, the structural, and compositional parameters measured by qNMR such as particle number, particle size, subclasses, and compositional components tend to better reflect the cardioprotective effects of HDL.
In the MESA cohort, HDL particle number (HDL-P) was protective against cIMT and CHD after adjusting for HDL-C, LDL-C, LDL-P, TG, and other confounders (119). In the Dallas Heart Study, when HDL-C was no longer related to CVD in multivariable analyses, HDL-P maintained a strong correlation (120). With no interaction with black race, HDL-P had consistency across diverse ethnicities (121). Moreover, among four HDL-related biomarkers in the JUPITER trial, HDL-P was consistently the strongest predictor of CVD at baseline or on statins (122). These studies all support that HDL-P might be a promising protective biomarker in atherosclerotic diseases.
The HDL subclass that best reflects the antiatherogenic features of HDL has been laden with controversy. Some studies describe large HDL as an effective form. A nested case-control study in the CKB cohort discovered that all HDL subclasses, except for small (diameter < 8.7 nm) HDL, were negatively associated with MI (109). In the Chicago Healthy Aging Study, large (diameter 8.8–13 nm) and medium (diameter 8.2–8.8 nm) HDL particle levels positively correlated with HDL cholesterol efflux capacity (CEC), which characterizes the key function of effluxing cellular cholesterol (123). Nevertheless, conflicting results have been reported. Ditah et al. (124) suggested that medium (diameter 8.2–9.4 nm) and small (diameter 7.2–8.2 nm) HDL were associated with CAC even after adjusting for HDL-C, while large (diameter 9.4–14 nm) HDL fell short of statistical significance. Silbernagel et al. (125) presented an inverse correlation of small (diameter 7.0–8.5 nm) HDL with CVD mortality, which could further improve the performance of the prediction model. Kim et al. (126) found that small and medium HDL-P was strongly associated with cardioprotective paraoxonase 1 (PON1) activity and that PON1 is a glycoprotein enzyme that prevents oxidation of LDL. These studies fuel the speculation that small HDL subclasses act as more accurate risk indicators.
HDL particle size (HDL-S) is usually inversely correlated with AS, but the negative relationship might be attenuated or abolished by other factors. In the Women's Health Study, the adjusted hazard ratio (HR) for HDL-S was 0.65 (0.51–0.81) (108). However, in the EPIC study, the association between HDL-S and CAD was diminished after adjusting for ApoB and TG levels (127). In the JUPITER trial, HDL-S underwent slight drug-induced changes and showed no association with CVD in fully adjusted models (128). As a result, in the long run, HDL-S seems to be a weaker predictor than the HDL subclass distribution.
In addition to HDL quantity, HDL quality has aroused growing attention. HDL functions are closely related to its compositional components. ApoA1 is the major protein on HDL that ensures structural stability and stimulates cholesterol efflux from cells to HDL (32). In the post-hoc analysis of the IDEAL (Incremental Decrease in End Points through Aggressive Lipid Lowering) trial and EPIC-Norfolk study, when HDL-C lost its inverse relationship with major coronary events after serial adjustments, ApoA1 exhibited a stable negative association in most models (129). Moreover, it also suggested that high levels of very large HDL particles not accompanied by high levels of ApoA1 were associated with increased but not decreased CVD risk (129). Therefore, ApoA1 should be taken into account more in risk assessment. Regarding lipid components in HDL, most cohort studies indicated that the cholesterol in small HDL subclasses primarily drove the inverse association with CVD events (130, 131). However, there is also a study that supports the idea that the cholesterol in large HDL inversely correlates with MI (109). Furthermore, despite the protective properties of HDL on the whole, HDL-TG has been reported to be positively correlated with arteriosclerotic diseases in many populations, such as the CKB cohort (109), MESA cohort (91) and T2DM or MS patients (132). Thus, it may be a potential pathogenic biomarker of CVD risk.
HDL particles demonstrate multiple antiatherogenic biological functions. The most widely known is reverse cholesterol transport (RCT) (133). Small HDL particles remove cholesterol from the arterial wall, primarily from the lipid-overloaded macrophages, and gradually expand into larger spherical HDL. These large HDL particles transfer their cholesterol esters to hepatocytes by scavenger receptor-B1 (SR-B1) or to ApoB-containing lipoproteins using CETP, regenerating a smaller HDL that repeats the process. Additional atheroprotective properties of HDL, particularly the small and medium HDL subclasses, include angiectatic, anti-inflammatory, antioxidant, and antiapoptotic actions. Specifically, HDL can stimulate the production of nitric oxide (NO) and prostacyclin to exert vasodilatory effects (134). HDL also suppresses the chronic inflammatory response by decreasing the generation of adhesion molecules, platelet-activating factor and Von Willebrand factor (135). By reducing the production of reactive oxygen species and contrasting the oxidation of LDL through paraoxonase or platelet activating factor acetyl hydrolase (136, 137), HDL is able to decrease intracellular oxidative stress. Furthermore, HDL particles exert cytoprotective actions, protecting both macrophages and endothelial cells from apoptosis (138). Taken together, small HDL particles seem to mainly represent the protective capacity of HDL, but more evidence from experimental studies in vitro and in vivo is needed.
The risk assessments of atherosclerotic diseases are traditionally based on lipoproteins and lipids. However, several qNMR platforms also enable the simultaneous detection of low-molecular-weight metabolites, offering multimetabolic signatures. In this review, we briefly introduce some typical qNMR-based molecular biomarkers.
Glycoprotein acetyls (GlycA) is a novel NMR biomarker of systemic inflammation that reflects the enzymatic glycosylation state of the main acute-phase reactants. Compared with hsCRP, GlycA was reported to have lower analytic imprecision and intraindividual variability (139). In the MESA cohort, GlycA was associated not only with subclinical atherosclerosis (140–142) but also with poorer CVD health independent of hsCRP, d-dimer, IL-6, and fibrinogen (143). However, it is not clear whether GlycA is a determinant or just an indicator of atherosclerotic progression.
The amino acid profile is also included in the qNMR metabolome, even though its overall picture may not be as clear as the lipoprotein profile. In the Taizhou Imaging Study, two branched-chain amino acids (leucine and isoleucine) were positively correlated with arterial stiffness (144). Additionally, phenylalanine showed an inverse association with CVD (91). In another multiqueue study, a high phenylalanine level was consistently associated with CVD death but was only associated with CVD events at a young age, except in elderly individuals over 60 years (145).
Lactate was inversely associated with cIMT in the Taizhou Imaging Study (144). But in another multi-racial study, lactate showed positive associations with CVD events (91). With respect to fatty acids, higher levels of monounsaturated fatty acid (MUFA) were positively correlated with CVD risk while higher levels of omega-6 fatty acids and docosahexaenoic acid (DHA) negatively related to CVD risk (145).
A statement by the American Heart Association addressed the potential impact of metabolomics on CVD health and disease (146). Indeed, studies looking for novel biomarkers using qNMR metabolomics, as described in the previous section, usually have three major, interlinked objectives, which will be discussed below.
Current CVD risk assessments, such as the Framingham Risk Score (FRS) (147) and the China-PAR tool (148), rely on traditional risk factors (TRFs). However, the first CVD event often originates in people classified as being at intermediate or low risk; hence, it is hoped that new qNMR-based metabolite biomarkers can complement or outperform the existing risk estimations.
The weighted metabolite score derived from 13 replicated signals was independently associated with CHD incidence. When adding age and sex to the score, the predictive performance paralleled that of TRFs (C-index 0.81 and 0.82) (149). The model using the calculated “VLDL extra-hepatic lipolysis indicator” and “VLDL hepatic turnover indicator” combined with LDL-C and HDL-C had better CVD risk prediction performance than that with only TRFs (AUROC 0.812 vs. 0.795) (150). In addition, in a meta-analysis across three cohorts, the prediction score incorporating phenylalanine, MUFAs, omega-6 fatty acids and DHA improved risk classification, particularly for people in the intermediate (5–10%) risk range (145).
Thus, qNMR metabolites not only offer additional risk information beyond traditional lipids but also have the clinical potential to reclassify the CVD risk stratification. In other words, qNMR metabolomics can be taken as an extension to routine analysis and, to a certain degree, may supersede lipid panels in the future.
qNMR metabolomics is expected to shed light on the “black box” mechanisms of AS and CVD. Biological pathways underlying the biomarkers can be characterized by searching metabolic pathway databases such as the Kyoto Encyclopedia of Genes and Genomes (KEGG; https://www.kegg.jp) and Human Metabolome Database (HMDB; https://www.hmdb.ca) (151). Clinical studies and basic research studies have shown that during AS progression, metabolic perturbations lie in lipid and fatty acid metabolism, BCAA and aromatic acid metabolism, the TCA cycle, glycolysis, urea metabolism, oxidative stress, and inflammatory and insulin pathways (152–154). Mapping the molecular interaction gene network can further depict interconnections between disturbed pathways to gain a more precise understanding (91).
Exposure to various environmental factors may bring about metabolic changes contributing to atherogenesis. A meta-analysis of 26,065 individuals from eight cohorts reported that age-specific metabolic profiles differed by sex, and the menopausal transition in females induced a proatherogenic lipoprotein profile along with amino acid changes (155). Additionally, the association between HDL-C and CVD events was attenuated in patients with chronic CAD or chronic kidney disease, probably owing to long-term inflammation, oxidative stress, and abnormal hormone secretion (38). In addition, a polymorphism of the APOA5 gene, which encodes a key regulator of TG levels, could modify lipoprotein distributions toward a more atherogenic pattern. This pattern was significantly associated with increased cIMT, especially in overweight or centrally obese patients, thus reflecting a “double hit” from gene-environment interactions (118).
The qNMR-based metabolic profile is capable of monitoring treatment efficacy. For example, among subjects treated with gemfibrozil, a fibric acid derivative, neither TG nor HDL-C predicted CHD events, but LDL-P and HDL-P could serve as independent predictive indexes (83). Therefore, the therapeutic benefit not reflected by conventional biochemical testing may be uncovered by qNMR metabolomics.
The profiling of metabolic changes also contributes to personalized intervention or optimal evaluation. Six metabolic patterns were identified in the early stage of AS (156). Among them, phenotype A held the highest risk (RR = 2.6); phenotype B presented less lipoprotein variation than A (RR = 2.4). Phenotype C held a relatively optimal profile, but the risk (RR = 1.8) was not accordingly desirable. For phenotypes D, E, and F, the relative risks were statistically similar. Thus, various metabolic phenotypes may involve varying risk levels. Proper classification of AS patients can help direct individualized treatment. Moreover, in the JUPITER trial that recruited subjects with low LDL-C, a small (diameter 29–42 nm) VLDL-P was responsible for residual CVD risk (106), and HDL-P was the strongest inverse predictor for CVD among several HDL-related indexes (122). Hence, qNMR metabolic profiles allow for the identification of potential targets for intervention and prognostic indicators for evaluation.
Although great progress has been made in regard to techniques, including automatic sample injection, spectral feature extraction, and efficient analytical tools, studies using qNMR metabolomics in AS and subsequent CVD still face a series of issues. In this section, we discuss several challenging points.
It is required to determine the “golden value” biomarkers for clinical applications. An eligible biomaker should fulfill some prerequisites, including valid and precise measurement, additional value beyond existing tests, and clinical benefit to subjects (157, 158).
NMR metabolomics offers metabolic profiles in reproducible manners. The relative standard deviations (RSD) of metabolites derived from qNMR spectroscopy are much smaller (i.e., values are within less fluctuation range) than those detected by mass spectrometry (57). In this regard, qNMR metabolomics endows its biomarkers with some practicability.
Despite a plethora of biomarkers for AS and CVD that have been identified in epidemiological studies, very few successfully find their way into clinical use. Various biomarker panels comprise different lipoproteins and/or molecular metabolites, which bring great difficulties in comparison and validation. Another point that needs to be noted is the application scope. Although AS is a systemic disease, risk factors across differing arterial beds and atherosclerotic endpoints do not necessarily overlap (159, 160). Thus, owing to intergroup variability and disease complexity, few biomarker candidates have been translated into clinical practice, and further research is still required.
In addition, the cost-benefit needs to be considered. NMR metabolomics detects a suite of metabolites. Making a diagnosis based on so many metabolites would not be convenient or economical in clinical practice. Encouragingly, the simplified LipoProfile panel (including LDL particle number, NMR determined HDL-C, and TG) was approved by the U.S. Food and Drug Administration in 2008 (161) and was covered by some health insurance companies in America. However, the clinical utilization rates and practical benefits remain unclear. If the clinical benefits are very small or deficient, the additional costs may not be justified.
The lack of standardized and uniform settings has become one of the biggest barriers to putting biomarker candidates into practice. A variety of analytical methods have been developed to separate lipoprotein subfractions according to their size or density. However, the measurement units differ between qNMR platforms, which makes it difficult to compare or combine results from different studies.
However, on the upside, a series of consortia or programs have been established to overcome the heterogeneity. The Consortium of Metabolomics Studies (COMETS) was developed in 2014 (162). As the largest consortium worldwide, it builds on 47 prospective studies that include over 136,000 participants from Asia, Europe, North America, and South America as of April 2018. Furthermore, the Atheroflux consortium consists of two earlier EU consortia, AtheroRemo and RiskyCAD (157). The intention of these alliances is to combine multiple data and to discern more reliable targets for clinical treatment and scientific research. It can be anticipated that with more communication among researchers, uniform criteria or comparative methods across various platforms may be available in the future.
Despite the many advantages of qNMR, especially the unique ability to quantify lipoproteins, its sensitivity is relatively poor in contrast to that of mass spectrometry. It is difficult to identify molecules with concentrations much lower than the detection limit; thus, qNMR is currently less preferred than MS in lipidomics. Moreover, the high sample volume requirement makes it difficult to perform assays on specimens at trace levels. The overlapping resonances in spectral regions may correspond to multiple metabolites, posing a great challenge to metabolite annotation (45). By comparison, MS has a greater sensitivity and can identify complex mixtures, despite its suboptimal quality control and high cost of absolute quantification. Therefore, NMR and MS have become complementary technologies in the field of metabolomics.
It should be noted that qNMR metabolomics provides only a “snapshot” of metabolic profiles. To learn about the potential causal pathways and upstream changes, cooperation with genomics, transcriptomics, proteomics, and microbiomics is required to identify serial biomarkers (38, 158). Additionally, metabolomics studies may only function as hypothesis-generating to provide an initial framework for further research. Whether the identified biomarkers are pathogenic and through which signaling pathways the biomarkers play their role remain to be solved. Hence, to elucidate definitive pathological mechanisms underlying the atherogenic process, more scientific investigations need to be performed.
In the postgenomic era, qNMR metabolomics has been widely applied in atherosclerotic diseases, which allows for the rapid, accurate and high-throughput measurements of circulating lipoproteins, lipids, and some molecular metabolites. In this review, we summarized the recent qNMR metabolomics studies associated with AS and CVD, with a particular emphasis on lipoprotein biomarkers referring to particle number, particle size, and compositional components. Since various qNMR platforms have discordant categorization criteria, along with intraindividual variability, interlab heterogeneity, and confounding factor interference, few biomarker candidates have been ultimately accepted in routine clinical practice. In conclusion, more extensive exploration in large population-based cohorts and validation of clinical applications should be pursued in the future. To successfully translate the “golden value” metabolite biomarkers from bench to bedside, we still have a long way to go.
SM drafted the manuscript. MX and XG revised, researched, and discussed the article. All authors approved it for publication.
This work was supported by the Shanghai Municipal Science and Technology Major Project (2017SHZDZX01 to XG).
The authors declare that the research was conducted in the absence of any commercial or financial relationships that could be construed as a potential conflict of interest.
All claims expressed in this article are solely those of the authors and do not necessarily represent those of their affiliated organizations, or those of the publisher, the editors and the reviewers. Any product that may be evaluated in this article, or claim that may be made by its manufacturer, is not guaranteed or endorsed by the publisher.
The Supplementary Material for this article can be found online at: https://www.frontiersin.org/articles/10.3389/fcvm.2021.681444/full#supplementary-material
1. Virani SS, Alonso A, Aparicio HJ, Benjamin EJ, Bittencourt MS, Callaway CW, et al. Heart disease and stroke statistics-2021 update: a report from the American Heart Association. Circulation. (2021) 143:e254–e743. doi: 10.1161/CIR.0000000000000950
2. GBD 2017 Causes of Death Collaborators. Global, regional, and national age-sex-specific mortality for 282 causes of death in 195 countries and territories, 1980-2017: a systematic analysis for the Global Burden of Disease Study 2017. Lancet Lond Engl. (2018) 392:1736–88. doi: 10.1016/S0140-6736(18)32203-7
3. Libby P, Buring JE, Badimon L, Hansson GK, Deanfield J, Bittencourt MS, et al. Atherosclerosis. Nat Rev Dis Primer. (2019) 5:56. doi: 10.1038/s41572-019-0106-z
4. Song P, Fang Z, Wang H, Cai Y, Rahimi K, Zhu Y, et al. Global and regional prevalence, burden, and risk factors for carotid atherosclerosis: a systematic review, meta-analysis, and modelling study. Lancet Glob Health. (2020) 8:e721–e9. doi: 10.1016/S2214-109X(20)30117-0
5. Getz GS, Reardon CA. Atherosclerosis: cell biology and lipoproteins. Curr Opin Lipidol. (2020) 31:35–7. doi: 10.1097/MOL.0000000000000655
6. Timmis A, Townsend N, Gale CP, Torbica A, Lettino M, Petersen SE, et al. European society of cardiology: cardiovascular disease statistics 2019. Eur Heart J. (2020) 41:12–85. doi: 10.1093/eurheartj/ehz859
7. Dawber TR, Moore FE, Mann GV. Coronary heart disease in the Framingham study. Am J Public Health Nations Health. (1957) 47:4–24. doi: 10.2105/AJPH.47.4_Pt_2.4
8. Jellinger PS, Smith DA, Mehta AE, Ganda O, Handelsman Y, Rodbard HW, et al. American Association of Clinical Endocrinologists' Guidelines for management of dyslipidemia and prevention of atherosclerosis. Endocr Pract. (2012) 18(Suppl 1):1–78. doi: 10.4158/EP.18.S1.1
9. Grundy SM, Stone NJ, Bailey AL, Beam C, Birtcher KK, Blumenthal RS, et al. 2018 AHA/ACC/AACVPR/AAPA/ABC/ACPM/ADA/AGS/APhA/ASPC/NLA/ PCNA guideline on the management of blood cholesterol: a report of the American College of Cardiology/American Heart Association Task Force on Clinical Practice Guidelines. Circulation. (2019) 139:e1082–e143. doi: 10.1161/CIR.0000000000000698
10. Al Rifai M, Martin SS, McEvoy JW, Nasir K, Blankstein R, Yeboah J, et al. The prevalence and correlates of subclinical atherosclerosis among adults with low-density lipoprotein cholesterol <70 mg/dL: the Multi-Ethnic Study of Atherosclerosis (MESA) and Brazilian Longitudinal Study of Adult Health (ELSA-Brasil). Atherosclerosis. (2018) 274:61–6. doi: 10.1016/j.atherosclerosis.2018.04.021
11. Sachdeva A, Cannon CP, Deedwania PC, Labresh KA, Smith SC, Dai D, et al. Lipid levels in patients hospitalized with coronary artery disease: an analysis of 136,905 hospitalizations in Get With The Guidelines. Am Heart J. (2009) 157:111–7.e2. doi: 10.1016/j.ahj.2008.08.010
12. Ormazabal V, Nair S, Elfeky O, Aguayo C, Salomon C, Zuñiga FA. Association between insulin resistance and the development of cardiovascular disease. Cardiovasc Diabetol. (2018) 17:122. doi: 10.1186/s12933-018-0762-4
13. Zhou J, Gao Q, Wang J, Zhang M, Ma J, Wang C, et al. Comparison of coronary heart disease risk assessments among individuals with metabolic syndrome using three diagnostic definitions: a cross-sectional study from China. BMJ Open. (2018) 8:e022974. doi: 10.1136/bmjopen-2018-022974
14. Taguchi I, Iimuro S, Iwata H, Takashima H, Abe M, Amiya E, et al. High-dose versus low-dose pitavastatin in japanese patients with stable coronary artery disease (REAL-CAD): a randomized superiority trial. Circulation. (2018) 137:1997–2009. doi: 10.1161/CIRCULATIONAHA.117.032615
15. Nordestgaard LT, Christoffersen M, Afzal S, Nordestgaard BG, Tybjærg-Hansen A, Frikke-Schmidt R. Triglycerides as a shared risk factor between dementia and atherosclerotic cardiovascular disease: a study of 125 727 individuals. Clin Chem. (2021) 67:245–55. doi: 10.1093/clinchem/hvaa269
16. Koo BK, Park S, Han K-D, Moon MK. Hypertriglyceridemia is an independent risk factor for cardiovascular diseases in korean adults aged 30-49 years: a Nationwide Population-Based Study. J Lipid Atheroscler. (2021) 10:88–98. doi: 10.12997/jla.2021.10.1.88
17. Sarwar N, Danesh J, Eiriksdottir G, Sigurdsson G, Wareham N, Bingham S, et al. Triglycerides and the risk of coronary heart disease: 10,158 incident cases among 262,525 participants in 29 Western prospective studies. Circulation. (2007) 115:450–8. doi: 10.1161/CIRCULATIONAHA.106.637793
18. Malhotra G, Sethi A, Arora R. Hypertriglyceridemia and cardiovascular outcomes. Am J Ther. (2016) 23:e862–70. doi: 10.1097/MJT.0000000000000116
19. Nordestgaard BG. Triglyceride-rich lipoproteins and atherosclerotic cardiovascular disease: new insights from epidemiology, genetics, and biology. Circ Res. (2016) 118:547–63. doi: 10.1161/CIRCRESAHA.115.306249
20. Langsted A, Nordestgaard BG. Nonfasting versus fasting lipid profile for cardiovascular risk prediction. Pathology (Phila). (2019) 51:131–41. doi: 10.1016/j.pathol.2018.09.062
21. Langsted A, Madsen CM, Nordestgaard BG. Contribution of remnant cholesterol to cardiovascular risk. J Intern Med. (2020) 288:116–27. doi: 10.1111/joim.13059
22. Basu D, Bornfeldt KE. Hypertriglyceridemia and atherosclerosis: using human research to guide mechanistic studies in animal models. Front Endocrinol. (2020) 11:504. doi: 10.3389/fendo.2020.00504
23. Langsted A, Freiberg JJ, Tybjaerg-Hansen A, Schnohr P, Jensen GB, Nordestgaard BG. Nonfasting cholesterol and triglycerides and association with risk of myocardial infarction and total mortality: the Copenhagen City Heart Study with 31 years of follow-up. J Intern Med. (2011) 270:65–75. doi: 10.1111/j.1365-2796.2010.02333.x
24. Freiberg JJ, Tybjaerg-Hansen A, Jensen JS, Nordestgaard BG. Nonfasting triglycerides and risk of ischemic stroke in the general population. JAMA. (2008) 300:2142–52. doi: 10.1001/jama.2008.621
25. Nordestgaard BG, Benn M, Schnohr P, Tybjaerg-Hansen A. Nonfasting triglycerides and risk of myocardial infarction, ischemic heart disease, and death in men and women. JAMA. (2007) 298:299–308. doi: 10.1001/jama.298.3.299
26. Thomsen M, Varbo A, Tybjærg-Hansen A, Nordestgaard BG. Low nonfasting triglycerides and reduced all-cause mortality: a mendelian randomization study. Clin Chem. (2014) 60:737–46. doi: 10.1373/clinchem.2013.219881
27. Hirata A, Sugiyama D, Watanabe M, Tamakoshi A, Iso H, Kotani K, et al. Association of extremely high levels of high-density lipoprotein cholesterol with cardiovascular mortality in a pooled analysis of 9 cohort studies including 43,407 individuals: the EPOCH-JAPAN study. J Clin Lipidol. (2018) 12:674–84. doi: 10.1016/j.jacl.2018.01.014
28. Ko DT, Alter DA, Guo H, Koh M, Lau G, Austin PC, et al. High-density lipoprotein cholesterol and cause-specific mortality in individuals. J Am Coll Cardiol. (2016) 68:2073–83. doi: 10.1016/j.jacc.2016.08.038
29. Cannon CP, Shah S, Dansky HM, Davidson M, Brinton EA, Gotto AM, et al. Safety of anacetrapib in patients with or at high risk for coronary heart disease. N Engl J Med. (2010) 363:2406–15. doi: 10.1056/NEJMoa1009744
30. Schwartz GG, Olsson AG, Abt M, Ballantyne CM, Barter PJ, Brumm J, et al. Effects of dalcetrapib in patients with a recent acute coronary syndrome. N Engl J Med. (2012) 367:2089–99. doi: 10.1056/NEJMoa1206797
31. Zanoni P, Khetarpal SA, Larach DB, Hancock-Cerutti WF, Millar JS, Cuchel M, et al. Rare variant in scavenger receptor BI raises HDL cholesterol and increases risk of coronary heart disease. Science. (2016) 351:1166–71. doi: 10.1126/science.aad3517
32. Pappa E, Elisaf MS, Kostara C, Bairaktari E, Tsimihodimos VK. Cardioprotective properties of HDL: structural and functional considerations. Curr Med Chem. (2020) 27:2964–78. doi: 10.2174/0929867326666190201142321
33. Arnao V, Tuttolomondo A, Daidone M, Pinto A. Lipoproteins in atherosclerosis process. Curr Med Chem. (2019) 26:1525–43. doi: 10.2174/0929867326666190516103953
34. Kockx M, Kritharides L. Triglyceride-rich lipoproteins. Cardiol Clin. (2018) 36:265–75. doi: 10.1016/j.ccl.2017.12.008
35. Diffenderfer MR, Schaefer EJ. The composition and metabolism of large and small LDL. Curr Opin Lipidol. (2014) 25:221–6. doi: 10.1097/MOL.0000000000000067
36. Woudberg NJ, Pedretti S, Lecour S, Schulz R, Vuilleumier N, James RW, et al. Pharmacological intervention to modulate HDL: what do we target? Front Pharmacol. (2017) 8:989. doi: 10.3389/fphar.2017.00989
37. Patel KV, Pandey A, de Lemos JA. Conceptual framework for addressing residual atherosclerotic cardiovascular disease risk in the era of precision medicine. Circulation. (2018) 137:2551–3. doi: 10.1161/CIRCULATIONAHA.118.035289
38. Hoefer IE, Steffens S, Ala-Korpela M, Bäck M, Badimon L, Bochaton-Piallat M-L, et al. Novel methodologies for biomarker discovery in atherosclerosis. Eur Heart J. (2015) 36:2635–42. doi: 10.1093/eurheartj/ehv236
39. Tibaut M, Caprnda M, Kubatka P, Sinkovič A, Valentova V, Filipova S, et al. Markers of atherosclerosis: part 1 - serological markers. Heart Lung Circ. (2019) 28:667–77. doi: 10.1016/j.hlc.2018.06.1057
40. Wishart DS. Emerging applications of metabolomics in drug discovery and precision medicine. Nat Rev Drug Discov. (2016) 15:473–84. doi: 10.1038/nrd.2016.32
41. Nicholson JK, Lindon JC, Holmes E. “Metabonomics”: understanding the metabolic responses of living systems to pathophysiological stimuli via multivariate statistical analysis of biological NMR spectroscopic data. Xenobiotica Fate Foreign Compd Biol Syst. (1999) 29:1181–9. doi: 10.1080/004982599238047
42. Iida M, Harada S, Takebayashi T. Application of metabolomics to epidemiological studies of atherosclerosis and cardiovascular disease. J Atheroscler Thromb. (2019) 26:747–57. doi: 10.5551/jat.RV17036
43. Wang F, Debik J, Andreassen T, Euceda LR, Haukaas TH, Cannet C, et al. Effect of repeated freeze-thaw cycles on NMR-measured lipoproteins and metabolites in biofluids. J Proteome Res. (2019) 18:3681–8. doi: 10.1021/acs.jproteome.9b00343
44. Martinez-Pinna R, Barbas C, Blanco-Colio LM, Tunon J, Ramos-Mozo P, Lopez JA, et al. Proteomic and metabolomic profiles in atherothrombotic vascular disease. Curr Atheroscler Rep. (2010) 12:202–8. doi: 10.1007/s11883-010-0102-y
45. Soininen P, Kangas AJ, Würtz P, Suna T, Ala-Korpela M. Quantitative serum nuclear magnetic resonance metabolomics in cardiovascular epidemiology and genetics. Circ Cardiovasc Genet. (2015) 8:192–206. doi: 10.1161/CIRCGENETICS.114.000216
46. Blake GJ, Otvos JD, Rifai N, Ridker PM. Low-density lipoprotein particle concentration and size as determined by nuclear magnetic resonance spectroscopy as predictors of cardiovascular disease in women. Circulation. (2002) 106:1930–7. doi: 10.1161/01.CIR.0000033222.75187.B9
47. Juonala M, Ellul S, Lawlor DA, Santos Ferreira DL, Carlin JB, Cheung M, et al. A cross-cohort study examining the associations of metabolomic profile and subclinical atherosclerosis in children and their parents: the child health checkpoint study and avon longitudinal study of parents and children. J Am Heart Assoc. (2019) 8:e011852. doi: 10.1161/JAHA.118.011852
48. Pallarés-Carratalá V, Quesada JA, Orozco-Beltrán D, Amigó-Grau N, Lopez-Pineda A, Fernández Giménez A, et al. Analysis of LDL and HDL size and number by nuclear magnetic resonance in a healthy working population: the LipoLab Study. Int J Clin Pract. (2021) 75:e13610. doi: 10.1111/ijcp.13610
49. Deelen J, Kettunen J, Fischer K, van der Spek A, Trompet S, Kastenmüller G, et al. A metabolic profile of all-cause mortality risk identified in an observational study of 44,168 individuals. Nat Commun. (2019) 10:3346. doi: 10.1038/s41467-019-11311-9
50. Amor AJ, Castelblanco E, Hernández M, Gimenez M, Granado-Casas M, Blanco J, et al. Advanced lipoprotein profile disturbances in type 1 diabetes mellitus: a focus on LDL particles. Cardiovasc Diabetol. (2020) 19:126. doi: 10.1186/s12933-020-01099-0
51. Virani SS, Pompeii L, Lincoln AE, Dunn RE, Tucker AM, Nambi V, et al. Association between traditional cholesterol parameters, lipoprotein particle concentration, novel biomarkers and carotid plaques in retired National Football League players. Atherosclerosis. (2012) 222:551–6. doi: 10.1016/j.atherosclerosis.2012.03.031
52. Nicholson JK, Sadler PJ, Cain K, Holt DE, Webb M, Hawkes GE. 88MHz 113Cd-n.m.r. studies of native rat liver metallothioneins. Biochem J. (1983) 211:251–5. doi: 10.1042/bj2110251
53. Nicholson JK, Buckingham MJ, Sadler PJ. High resolution 1H n.m.r. studies of vertebrate blood and plasma. Biochem J. (1983) 211:605–15. doi: 10.1042/bj2110605
54. Barding GA, Salditos R, Larive CK. Quantitative NMR for bioanalysis and metabolomics. Anal Bioanal Chem. (2012) 404:1165–79. doi: 10.1007/s00216-012-6188-z
55. Rankin NJ, Preiss D, Welsh P, Burgess KEV, Nelson SM, Lawlor DA, et al. The emergence of proton nuclear magnetic resonance metabolomics in the cardiovascular arena as viewed from a clinical perspective. Atherosclerosis. (2014) 237:287–300. doi: 10.1016/j.atherosclerosis.2014.09.024
56. Otvos JD. Measurement of lipoprotein subclass profiles by nuclear magnetic resonance spectroscopy. Clin Lab. (2002) 48:171–80.
57. Ussher JR, Elmariah S, Gerszten RE, Dyck JRB. The emerging role of metabolomics in the diagnosis and prognosis of cardiovascular disease. J Am Coll Cardiol. (2016) 68:2850–70. doi: 10.1016/j.jacc.2016.09.972
58. Mihaleva VV, van Schalkwijk DB, de Graaf AA, van Duynhoven J, van Dorsten FA, Vervoort J, et al. A systematic approach to obtain validated partial least square models for predicting lipoprotein subclasses from serum NMR spectra. Anal Chem. (2014) 86:543–50. doi: 10.1021/ac402571z
59. Mihaleva VV, Korhonen S-P, van Duynhoven J, Niemitz M, Vervoort J, Jacobs DM. Automated quantum mechanical total line shape fitting model for quantitative NMR-based profiling of human serum metabolites. Anal Bioanal Chem. (2014) 406:3091–102. doi: 10.1007/s00216-014-7752-5
60. Ala-Korpela M, Korhonen A, Keisala J, Hörkkö S, Korpi P, Ingman LP, et al. 1H NMR-based absolute quantitation of human lipoproteins and their lipid contents directly from plasma. J Lipid Res. (1994) 35:2292–304. doi: 10.1016/S0022-2275(20)39935-1
61. Flote VG, Vettukattil R, Bathen TF, Egeland T, McTiernan A, Frydenberg H, et al. Lipoprotein subfractions by nuclear magnetic resonance are associated with tumor characteristics in breast cancer. Lipids Health Dis. (2016) 15:56. doi: 10.1186/s12944-016-0225-4
62. Jiménez B, Holmes E, Heude C, Tolson RF, Harvey N, Lodge SL, et al. Quantitative lipoprotein subclass and low molecular weight metabolite analysis in human serum and plasma by 1H NMR spectroscopy in a multilaboratory trial. Anal Chem. (2018) 90:11962–71. doi: 10.1021/acs.analchem.8b02412
63. Lounila J, Ala-Korpela M, Jokisaari J, Savolainen MJ, Kesäniemi YA. Effects of orientational order and particle size on the NMR line positions of lipoproteins. Phys Rev Lett. (1994) 72:4049–52. doi: 10.1103/PhysRevLett.72.4049
64. Crook AA, Powers R. Quantitative NMR-based biomedical metabolomics: current status and applications. Mol Basel Switz. (2020) 25:5128. doi: 10.3390/molecules25215128
65. Otvos JD, Jeyarajah EJ, Bennett DW. Quantification of plasma lipoproteins by proton nuclear magnetic resonance spectroscopy. Clin Chem. (1991) 37:377–86. doi: 10.1093/clinchem/37.3.377
66. Jeyarajah EJ, Cromwell WC, Otvos JD. Lipoprotein particle analysis by nuclear magnetic resonance spectroscopy. Clin Lab Med. (2006) 26:847–70. doi: 10.1016/j.cll.2006.07.006
67. Hiltunen Y, Ala-Korpela M, Jokisaari J, Eskelinen S, Kiviniitty K, Savolainen M, et al. A lineshape fitting model for 1H NMR spectra of human blood plasma. Magn Reson Med. (1991) 21:222–32. doi: 10.1002/mrm.1910210207
68. Mallol R, Amigó N, Rodríguez MA, Heras M, Vinaixa M, Plana N, et al. Liposcale: a novel advanced lipoprotein test based on 2D diffusion-ordered 1H NMR spectroscopy. J Lipid Res. (2015) 56:737–46. doi: 10.1194/jlr.D050120
69. Martin SS, Blaha MJ, Elshazly MB, Toth PP, Kwiterovich PO, Blumenthal RS, et al. Comparison of a novel method vs the Friedewald equation for estimating low-density lipoprotein cholesterol levels from the standard lipid profile. JAMA. (2013) 310:2061–8. doi: 10.1001/jama.2013.280532
70. El Harchaoui K, van der Steeg WA, Stroes ESG, Kuivenhoven JA, Otvos JD, Wareham NJ, et al. Value of low-density lipoprotein particle number and size as predictors of coronary artery disease in apparently healthy men and women: the EPIC-Norfolk Prospective Population Study. J Am Coll Cardiol. (2007) 49:547–53. doi: 10.1016/j.jacc.2006.09.043
71. Toth PP, Grabner M, Punekar RS, Quimbo RA, Cziraky MJ, Jacobson TA. Cardiovascular risk in patients achieving low-density lipoprotein cholesterol and particle targets. Atherosclerosis. (2014) 235:585–91. doi: 10.1016/j.atherosclerosis.2014.05.914
72. Otvos JD, Mora S, Shalaurova I, Greenland P, Mackey RH, Goff DC. Clinical implications of discordance between low-density lipoprotein cholesterol and particle number. J Clin Lipidol. (2011) 5:105–13. doi: 10.1016/j.jacl.2011.02.001
73. Lawler PR, Akinkuolie AO, Ridker PM, Sniderman AD, Buring JE, Glynn RJ, et al. Discordance between circulating atherogenic cholesterol mass and lipoprotein particle concentration in relation to future coronary events in women. Clin Chem. (2017) 63:870–9. doi: 10.1373/clinchem.2016.264515
74. Mora S, Buring JE, Ridker PM. Discordance of low-density lipoprotein (LDL) cholesterol with alternative LDL-related measures and future coronary events. Circulation. (2014) 129:553–61. doi: 10.1161/CIRCULATIONAHA.113.005873
75. Bays HE, Jones PH, Orringer CE, Brown WV, Jacobson TA. National lipid association annual summary of clinical lipidology 2016. J Clin Lipidol. (2016) 10:S1–43. doi: 10.1016/j.jacl.2015.08.002
76. Qi Y, Liu J, Wang W, Wang M, Zhao F, Sun J, et al. High sdLDL cholesterol can be used to reclassify individuals with low cardiovascular risk for early intervention: findings from the Chinese multi-provincial cohort study. J Atheroscler Thromb. (2020) 27:695–710. doi: 10.5551/jat.49841
77. Pichler G, Amigo N, Tellez-Plaza M, Pardo-Cea MA, Dominguez-Lucas A, Marrachelli VG, et al. LDL particle size and composition and incident cardiovascular disease in a South-European population: the Hortega-Liposcale follow-up study. Int J Cardiol. (2018) 264:172–8. doi: 10.1016/j.ijcard.2018.03.128
78. Mora S, Caulfield MP, Wohlgemuth J, Chen Z, Superko HR, Rowland CM, et al. Atherogenic lipoprotein subfractions determined by ion mobility and first cardiovascular events after random allocation to high-intensity statin or placebo: the justification for the use of statins in prevention: an intervention trial evaluating rosuvastatin (JUPITER) trial. Circulation. (2015) 132:2220–9. doi: 10.1161/CIRCULATIONAHA.115.016857
79. Williams PT, Zhao X-Q, Marcovina SM, Otvos JD, Brown BG, Krauss RM. Comparison of four methods of analysis of lipoprotein particle subfractions for their association with angiographic progression of coronary artery disease. Atherosclerosis. (2014) 233:713–20. doi: 10.1016/j.atherosclerosis.2014.01.034
80. Joint committee for guideline revision. 2016 Chinese guidelines for the management of dyslipidemia in adults. J Geriatr Cardiol JGC. (2018) 15:1–29. doi: 10.11909/j.issn.1671-5411.2018.01.011
81. Mach F, Baigent C, Catapano AL, Koskinas KC, Casula M, Badimon L, et al. 2019 ESC/EAS Guidelines for the management of dyslipidaemias: lipid modification to reduce cardiovascular risk. Eur Heart J. (2020) 41:111–88. doi: 10.15829/1560-4071-2020-3826
82. Mora S, Szklo M, Otvos JD, Greenland P, Psaty BM, Goff DC, et al. LDL particle subclasses, LDL particle size, and carotid atherosclerosis in the Multi-Ethnic Study of Atherosclerosis (MESA). Atherosclerosis. (2007) 192:211–7. doi: 10.1016/j.atherosclerosis.2006.05.007
83. Otvos JD, Collins D, Freedman DS, Shalaurova I, Schaefer EJ, McNamara JR, et al. Low-density lipoprotein and high-density lipoprotein particle subclasses predict coronary events and are favorably changed by gemfibrozil therapy in the Veterans Affairs High-Density Lipoprotein Intervention Trial. Circulation. (2006) 113:1556–63. doi: 10.1161/CIRCULATIONAHA.105.565135
84. Urbina E, McCoy C, Gao Z, Khoury P, Shah A, Dolan L, et al. Lipoprotein particle number & size predict vascular structure & function better than traditional lipids in adolescents & young adults. J Clin Lipidol. (2017) 11:1023–31. doi: 10.1016/j.jacl.2017.05.011
85. Mason AE, Saslow LR, Moran PJ, Kim S, Abousleiman H, Richler R, et al. Lipid findings from the Diabetes Education to Lower Insulin, Sugars, and Hunger (DELISH) Study. Nutr Metab. (2019) 16:58. doi: 10.1186/s12986-019-0416-x
86. Rodríguez-Borjabad C, Ibarretxe D, Girona J, Ferré R, Feliu A, Amigó N, et al. Lipoprotein profile assessed by 2D-1H-NMR and subclinical atherosclerosis in children with familial hypercholesterolaemia. Atherosclerosis. (2018) 270:117–22. doi: 10.1016/j.atherosclerosis.2018.01.040
87. Tonouchi R, Okada T, Abe Y, Kazama M, Kuromori Y, Yoshino Y, et al. Subclass distribution of low-density lipoprotein triglyceride and the clustering of metabolic syndrome components in Japanese children. Pediatr Int. (2021) 63:664–70. doi: 10.1111/ped.14490
88. Ding X-H, Ye P, Wang X-N, Cao R-H, Yang X, Xiao W-K, et al. The predictive value of baseline LDL-TG level on major adverse cardiovascular events in a followed up cohort population. Eur Rev Med Pharmacol Sci. (2017) 21:1060–4.
89. Saeed A, Feofanova EV, Yu B, Sun W, Virani SS, Nambi V, et al. Remnant-like particle cholesterol, low-density lipoprotein triglycerides, and incident cardiovascular disease. J Am Coll Cardiol. (2018) 72:156–69. doi: 10.1016/j.jacc.2018.04.050
90. Jin J-L, Zhang H-W, Cao Y-X, Liu H-H, Hua Q, Li Y-F, et al. Long-term prognostic utility of low-density lipoprotein (LDL) triglyceride in real-world patients with coronary artery disease and diabetes or prediabetes. Cardiovasc Diabetol. (2020) 19:152. doi: 10.1186/s12933-020-01125-1
91. Tzoulaki I, Castagné R, Boulangé CL, Karaman I, Chekmeneva E, Evangelou E, et al. Serum metabolic signatures of coronary and carotid atherosclerosis and subsequent cardiovascular disease. Eur Heart J. (2019) 40:2883–96. doi: 10.1093/eurheartj/ehz235
92. Albers JJ, Slee A, Fleg JL, O'Brien KD, Marcovina SM. Relationship of baseline HDL subclasses, small dense LDL and LDL triglyceride to cardiovascular events in the AIM-HIGH clinical trial. Atherosclerosis. (2016) 251:454–9. doi: 10.1016/j.atherosclerosis.2016.06.019
93. Skålén K, Gustafsson M, Rydberg EK, Hultén LM, Wiklund O, Innerarity TL, et al. Subendothelial retention of atherogenic lipoproteins in early atherosclerosis. Nature. (2002) 417:750–4. doi: 10.1038/nature00804
94. Morita S. Metabolism and modification of apolipoprotein B-containing lipoproteins involved in dyslipidemia and atherosclerosis. Biol Pharm Bull. (2016) 39:1–24. doi: 10.1248/bpb.b15-00716
95. Ylä-Herttuala S, Palinski W, Rosenfeld ME, Parthasarathy S, Carew TE, Butler S, et al. Evidence for the presence of oxidatively modified low density lipoprotein in atherosclerotic lesions of rabbit and man. J Clin Invest. (1989) 84:1086–95. doi: 10.1172/JCI114271
96. Steinbrecher UP. Receptors for oxidized low density lipoprotein. Biochim Biophys Acta. (1999) 1436:279–98. doi: 10.1016/S0005-2760(98)00127-1
97. Allahverdian S, Pannu PS, Francis GA. Contribution of monocyte-derived macrophages and smooth muscle cells to arterial foam cell formation. Cardiovasc Res. (2012) 95:165–72. doi: 10.1093/cvr/cvs094
98. Sandesara PB, Virani SS, Fazio S, Shapiro MD. The forgotten lipids: triglycerides, remnant cholesterol, and atherosclerotic cardiovascular disease risk. Endocr Rev. (2019) 40:537–57. doi: 10.1210/er.2018-00184
99. März W, Scharnagl H, Winkler K, Tiran A, Nauck M, Boehm BO, et al. Low-density lipoprotein triglycerides associated with low-grade systemic inflammation, adhesion molecules, and angiographic coronary artery disease: the Ludwigshafen Risk and Cardiovascular Health study. Circulation. (2004) 110:3068–74. doi: 10.1161/01.CIR.0000146898.06923.80
100. Okada T, Saito E, Kuromori Y, Miyashita M, Iwata F, Hara M, et al. Relationship between serum adiponectin level and lipid composition in each lipoprotein fraction in adolescent children. Atherosclerosis. (2006) 188:179–83. doi: 10.1016/j.atherosclerosis.2005.10.030
101. Nordestgaard BG, Varbo A. Triglycerides and cardiovascular disease. Lancet Lond Engl. (2014) 384:626–35. doi: 10.1016/S0140-6736(14)61177-6
102. Varbo A, Nordestgaard BG. Remnant cholesterol and triglyceride-rich lipoproteins in atherosclerosis progression and cardiovascular disease. Arterioscler Thromb Vasc Biol. (2016) 36:2133–5. doi: 10.1161/ATVBAHA.116.308305
103. Castañer O, Pintó X, Subirana I, Amor AJ, Ros E, Hernáez Á, et al. Remnant Cholesterol, Not LDL cholesterol, is associated with incident cardiovascular disease. J Am Coll Cardiol. (2020) 76:2712–24. doi: 10.1016/j.jacc.2020.10.008
104. Freedman DS, Otvos JD, Jeyarajah EJ, Barboriak JJ, Anderson AJ, Walker JA. Relation of lipoprotein subclasses as measured by proton nuclear magnetic resonance spectroscopy to coronary artery disease. Arterioscler Thromb Vasc Biol. (1998) 18:1046–53. doi: 10.1161/01.ATV.18.7.1046
105. Mackey RH, Kuller LH, Sutton-Tyrrell K, Evans RW, Holubkov R, Matthews KA. Lipoprotein subclasses and coronary artery calcium in postmenopausal women from the healthy women study. Am J Cardiol. (2002) 90:71i−6i. doi: 10.1016/S0002-9149(02)02636-X
106. Lawler PR, Akinkuolie AO, Chu AY, Shah SH, Kraus WE, Craig D, et al. Atherogenic lipoprotein determinants of cardiovascular disease and residual risk among individuals with low low-density lipoprotein cholesterol. J Am Heart Assoc Cardiovasc Cerebrovasc Dis. (2017) 6:e005549. doi: 10.1161/JAHA.117.005549
107. Lawler PR, Akinkuolie AO, Harada P, Glynn RJ, Chasman DI, Ridker PM, et al. Residual risk of atherosclerotic cardiovascular events in relation to reductions in very-low-density lipoproteins. J Am Heart Assoc Cardiovasc Cerebrovasc Dis. (2017) 6:e007402. doi: 10.1161/JAHA.117.007402
108. Mora S, Otvos JD, Rifai N, Rosenson RS, Buring JE, Ridker PM. Lipoprotein particle profiles by nuclear magnetic resonance compared with standard lipids and apolipoproteins in predicting incident cardiovascular disease in women. Circulation. (2009) 119:931–9. doi: 10.1161/CIRCULATIONAHA.108.816181
109. Holmes MV, Millwood IY, Kartsonaki C, Hill MR, Bennett DA, Boxall R, et al. Lipids, lipoproteins, and metabolites and risk of myocardial infarction and stroke. J Am Coll Cardiol. (2018) 71:620–32. doi: 10.1016/j.jacc.2017.12.006
110. Balling M, Afzal S, Varbo A, Langsted A, Davey Smith G, Nordestgaard BG. VLDL cholesterol accounts for one-half of the risk of myocardial infarction associated with apoB-containing lipoproteins. J Am Coll Cardiol. (2020) 76:2725–35. doi: 10.1016/j.jacc.2020.09.610
111. Nordestgaard BG, Wootton R, Lewis B. Selective retention of VLDL, IDL, and LDL in the arterial intima of genetically hyperlipidemic rabbits in vivo. Molecular size as a determinant of fractional loss from the intima-inner media. Arterioscler Thromb Vasc Biol. (1995) 15:534–42. doi: 10.1161/01.ATV.15.4.534
112. Rosenson RS, Davidson MH, Hirsh BJ, Kathiresan S, Gaudet D. Genetics and causality of triglyceride-rich lipoproteins in atherosclerotic cardiovascular disease. J Am Coll Cardiol. (2014) 64:2525–40. doi: 10.1016/j.jacc.2014.09.042
113. Shin HK, Kim YK, Kim KY, Lee JH, Hong KW. Remnant lipoprotein particles induce apoptosis in endothelial cells by NAD(P)H oxidase-mediated production of superoxide and cytokines via lectin-like oxidized low-density lipoprotein receptor-1 activation: prevention by cilostazol. Circulation. (2004) 109:1022–8. doi: 10.1161/01.CIR.0000117403.64398.53
114. Doi H, Kugiyama K, Oka H, Sugiyama S, Ogata N, Koide SI, et al. Remnant lipoproteins induce proatherothrombogenic molecules in endothelial cells through a redox-sensitive mechanism. Circulation. (2000) 102:670–6. doi: 10.1161/01.CIR.102.6.670
115. Steinberg HO, Tarshoby M, Monestel R, Hook G, Cronin J, Johnson A, et al. Elevated circulating free fatty acid levels impair endothelium-dependent vasodilation. J Clin Invest. (1997) 100:1230–9. doi: 10.1172/JCI119636
116. Anderson RA, Evans ML, Ellis GR, Graham J, Morris K, Jackson SK, et al. The relationships between post-prandial lipaemia, endothelial function and oxidative stress in healthy individuals and patients with type 2 diabetes. Atherosclerosis. (2001) 154:475–83. doi: 10.1016/S0021-9150(00)00499-8
117. Meex RCR, Blaak EE, van Loon LJC. Lipotoxicity plays a key role in the development of both insulin resistance and muscle atrophy in patients with type 2 diabetes. Obes Rev. (2019) 20:1205–17. doi: 10.1111/obr.12862
118. Guardiola M, Cofán M, de Castro-Oros I, Cenarro A, Plana N, Talmud PJ, et al. APOA5 variants predispose hyperlipidemic patients to atherogenic dyslipidemia and subclinical atherosclerosis. Atherosclerosis. (2015) 240:98–104. doi: 10.1016/j.atherosclerosis.2015.03.008
119. Mackey RH, Greenland P, Goff DC, Lloyd-Jones D, Sibley CT, Mora S. High-density lipoprotein cholesterol and particle concentrations, carotid atherosclerosis, and coronary events: MESA (multi-ethnic study of atherosclerosis). J Am Coll Cardiol. (2012) 60:508–16. doi: 10.1016/j.jacc.2012.03.060
120. Rohatgi A, Khera A, Berry JD, Givens EG, Ayers CR, Wedin KE, et al. HDL cholesterol efflux capacity and incident cardiovascular events. N Engl J Med. (2014) 371:2383–93. doi: 10.1056/NEJMoa1409065
121. Chandra A, Neeland IJ, Das SR, Khera A, Turer AT, Ayers CR, et al. Relation of black race between high density lipoprotein cholesterol content, high density lipoprotein particles and coronary events (from the Dallas Heart Study). Am J Cardiol. (2015) 115:890–4. doi: 10.1016/j.amjcard.2015.01.015
122. Khera AV, Demler OV, Adelman SJ, Collins HL, Glynn RJ, Ridker PM, et al. Cholesterol efflux capacity, high-density lipoprotein particle number, and incident cardiovascular events: an analysis from the JUPITER Trial (Justification for the Use of Statins in Prevention: An Intervention Trial Evaluating Rosuvastatin). Circulation. (2017) 135:2494–504. doi: 10.1161/CIRCULATIONAHA.116.025678
123. Mutharasan RK, Thaxton CS, Berry J, Daviglus ML, Yuan C, Sun J, et al. HDL efflux capacity, HDL particle size, and high-risk carotid atherosclerosis in a cohort of asymptomatic older adults: the Chicago Healthy Aging Study. J Lipid Res. (2017) 58:600–6. doi: 10.1194/jlr.P069039
124. Ditah C, Otvos J, Nassar H, Shaham D, Sinnreich R, Kark JD. Small and medium sized HDL particles are protectively associated with coronary calcification in a cross-sectional population-based sample. Atherosclerosis. (2016) 251:124–31. doi: 10.1016/j.atherosclerosis.2016.06.010
125. Silbernagel G, Pagel P, Pfahlert V, Genser B, Scharnagl H, Kleber ME, et al. High-density lipoprotein subclasses, coronary artery disease, and cardiovascular mortality. Clin Chem. (2017) 63:1886–96. doi: 10.1373/clinchem.2017.275636
126. Kim DS, Li YK, Bell GA, Burt AA, Vaisar T, Hutchins PM, et al. Concentration of smaller high-density lipoprotein particle (HDL-P) is inversely correlated with carotid intima media thickening after confounder adjustment: the multi ethnic study of atherosclerosis (MESA). J Am Heart Assoc Cardiovasc Cerebrovasc Dis. (2016) 5:e002977. doi: 10.1161/JAHA.115.002977
127. El Harchaoui K, Arsenault BJ, Franssen R, Després J-P, Hovingh GK, Stroes ESG, et al. High-density lipoprotein particle size and concentration and coronary risk. Ann Intern Med. (2009) 150:84–93. doi: 10.7326/0003-4819-150-2-200901200-00006
128. Mora S, Glynn RJ, Ridker PM. High-density lipoprotein cholesterol, size, particle number, and residual vascular risk after potent statin therapy. Circulation. (2013) 128:1189–97. doi: 10.1161/CIRCULATIONAHA.113.002671
129. van der Steeg WA, Holme I, Boekholdt SM, Larsen ML, Lindahl C, Stroes ESG, et al. High-density lipoprotein cholesterol, high-density lipoprotein particle size, and apolipoprotein A-I: significance for cardiovascular risk: the IDEAL and EPIC-Norfolk studies. J Am Coll Cardiol. (2008) 51:634–42. doi: 10.1016/j.jacc.2007.09.060
130. Joshi PH, Toth PP, Lirette ST, Griswold ME, Massaro JM, Martin SS, et al. Association of high-density lipoprotein subclasses and incident coronary heart disease: the Jackson Heart and Framingham Offspring Cohort Studies. Eur J Prev Cardiol. (2016) 23:41–9. doi: 10.1177/2047487314543890
131. Martin SS, Khokhar AA, May HT, Kulkarni KR, Blaha MJ, Joshi PH, et al. HDL cholesterol subclasses, myocardial infarction, and mortality in secondary prevention: the Lipoprotein Investigators Collaborative. Eur Heart J. (2015) 36:22–30. doi: 10.1093/eurheartj/ehu264
132. Girona J, Amigó N, Ibarretxe D, Plana N, Rodríguez-Borjabad C, Heras M, et al. HDL triglycerides: a new marker of metabolic and cardiovascular risk. Int J Mol Sci. (2019) 20:3151. doi: 10.3390/ijms20133151
133. Feig JE, Feig JL, Dangas GD. The role of HDL in plaque stabilization and regression: basic mechanisms and clinical implications. Coron Artery Dis. (2016) 27:592–603. doi: 10.1097/MCA.0000000000000408
134. Nofer J-R, van der Giet M, Tölle M, Wolinska I, von Wnuck Lipinski K, Baba HA, et al. HDL induces NO-dependent vasorelaxation via the lysophospholipid receptor S1P3. J Clin Invest. (2004) 113:569–81. doi: 10.1172/JCI200418004
135. Norata GD, Catapano AL. Molecular mechanisms responsible for the antiinflammatory and protective effect of HDL on the endothelium. Vasc Health Risk Manag. (2005) 1:119–29. doi: 10.2147/vhrm.1.2.119.64083
136. Robbesyn F, Garcia V, Auge N, Vieira O, Frisach M-F, Salvayre R, et al. HDL counterbalance the proinflammatory effect of oxidized LDL by inhibiting intracellular reactive oxygen species rise, proteasome activation, and subsequent NF-kappaB activation in smooth muscle cells. FASEB J. (2003) 17:743–5. doi: 10.1096/fj.02-0240fje
137. Yvan-Charvet L, Pagler TA, Seimon TA, Thorp E, Welch CL, Witztum JL, et al. ABCA1 and ABCG1 protect against oxidative stress-induced macrophage apoptosis during efferocytosis. Circ Res. (2010) 106:1861–9. doi: 10.1161/CIRCRESAHA.110.217281
138. Negre-Salvayre A, Dousset N, Ferretti G, Bacchetti T, Curatola G, Salvayre R. Antioxidant and cytoprotective properties of high-density lipoproteins in vascular cells. Free Radic Biol Med. (2006) 41:1031–40. doi: 10.1016/j.freeradbiomed.2006.07.006
139. Otvos JD, Shalaurova I, Wolak-Dinsmore J, Connelly MA, Mackey RH, Stein JH, et al. GlycA: a composite nuclear magnetic resonance biomarker of systemic inflammation. Clin Chem. (2015) 61:714–23. doi: 10.1373/clinchem.2014.232918
140. Tibuakuu M, Fashanu OE, Zhao D, Otvos JD, Brown TT, Haberlen SA, et al. GlycA, a novel inflammatory marker, is associated with subclinical coronary disease. AIDS Lond Engl. (2019) 33:547–57. doi: 10.1097/QAD.0000000000002079
141. Fashanu OE, Oyenuga AO, Zhao D, Tibuakuu M, Mora S, Otvos JD, et al. GlycA, a novel inflammatory marker and its association with peripheral arterial disease and carotid plaque: the Multi-Ethnic Study of Atherosclerosis. Angiology. (2019) 70:737–46. doi: 10.1177/0003319719845185
142. Ezeigwe A, Fashanu OE, Zhao D, Budoff MJ, Otvos JD, Thomas IC, et al. The novel inflammatory marker GlycA and the prevalence and progression of valvular and thoracic aortic calcification: the Multi-Ethnic Study of Atherosclerosis. Atherosclerosis. (2019) 282:91–9. doi: 10.1016/j.atherosclerosis.2019.01.011
143. Benson E-MA, Tibuakuu M, Zhao D, Akinkuolie AO, Otvos JD, Duprez DA, et al. Associations of ideal cardiovascular health with GlycA, a novel inflammatory marker: the Multi-Ethnic Study of Atherosclerosis. Clin Cardiol. (2018) 41:1439–45. doi: 10.1002/clc.23069
144. Jiang Y, Zhang K, Zhu Z, Cui M, An Y, Wang Y, et al. Associations between serum metabolites and subclinical atherosclerosis in a Chinese population: the Taizhou Imaging Study. Aging. (2020) 12:15302–13. doi: 10.18632/aging.103456
145. Würtz P, Havulinna AS, Soininen P, Tynkkynen T, Prieto-Merino D, Tillin T, et al. Metabolite profiling and cardiovascular event risk: a prospective study of three population-based cohorts. Circulation. (2015) 131:774–85. doi: 10.1161/CIRCULATIONAHA.114.013116
146. Cheng S, Shah SH, Corwin EJ, Fiehn O, Fitzgerald RL, Gerszten RE, et al. Potential impact and study considerations of metabolomics in cardiovascular health and disease: a scientific statement from the American Heart Association. Circ Cardiovasc Genet. (2017) 10:e000032. doi: 10.1161/HCG.0000000000000032
147. Wilson PW, D'Agostino RB, Levy D, Belanger AM, Silbershatz H, Kannel WB. Prediction of coronary heart disease using risk factor categories. Circulation. (1998) 97:1837–47. doi: 10.1161/01.CIR.97.18.1837
148. Yang X, Li J, Hu D, Chen J, Li Y, Huang J, et al. Predicting the 10-year risks of atherosclerotic cardiovascular disease in Chinese Population: the China-PAR Project (Prediction for ASCVD Risk in China). Circulation. (2016) 134:1430–40. doi: 10.1161/CIRCULATIONAHA.116.022367
149. Vaarhorst AAM, Verhoeven A, Weller CM, Böhringer S, Göraler S, Meissner A, et al. A metabolomic profile is associated with the risk of incident coronary heart disease. Am Heart J. (2014) 168:45–52.e7. doi: 10.1016/j.ahj.2014.01.019
150. van Schalkwijk DB, de Graaf AA, Tsivtsivadze E, Parnell LD, van der Werff-van der Vat BJC, van Ommen B, et al. Lipoprotein metabolism indicators improve cardiovascular risk prediction. PLoS One. (2014) 9:e92840. doi: 10.1371/journal.pone.0092840
151. Du F, Virtue A, Wang H, Yang X-F. Metabolomic analyses for atherosclerosis, diabetes, and obesity. Biomark Res. (2013) 1:17. doi: 10.1186/2050-7771-1-17
152. Teul J, Rupérez FJ, Garcia A, Vaysse J, Balayssac S, Gilard V, et al. Improving metabolite knowledge in stable atherosclerosis patients by association and correlation of GC-MS and 1H NMR fingerprints. J Proteome Res. (2009) 8:5580–9. doi: 10.1021/pr900668v
153. Zabek A, Paslawski R, Paslawska U, Wojtowicz W, Drozdz K, Polakof S, et al. The influence of different diets on metabolism and atherosclerosis processes-A porcine model: blood serum, urine and tissues 1H NMR metabolomics targeted analysis. PLoS One. (2017) 12:e0184798. doi: 10.1371/journal.pone.0184798
154. Guo W, Jiang C, Yang L, Li T, Liu X, Jin M, et al. Quantitative metabolomic profiling of plasma, urine, and liver extracts by 1H NMR spectroscopy characterizes different stages of atherosclerosis in hamsters. J Proteome Res. (2016) 15:3500–10. doi: 10.1021/acs.jproteome.6b00179
155. Auro K, Joensuu A, Fischer K, Kettunen J, Salo P, Mattsson H, et al. A metabolic view on menopause and ageing. Nat Commun. (2014) 5:4708. doi: 10.1038/ncomms5708
156. Würtz P, Soininen P, Kangas AJ, Mäkinen V-P, Groop P-H, Savolainen MJ, et al. Characterization of systemic metabolic phenotypes associated with subclinical atherosclerosis. Mol Biosyst. (2011) 7:385–93. doi: 10.1039/C0MB00066C
157. Laaksonen R, Ekroos K, Sysi-Aho M, Hilvo M, Vihervaara T, Kauhanen D, et al. Plasma ceramides predict cardiovascular death in patients with stable coronary artery disease and acute coronary syndromes beyond LDL-cholesterol. Eur Heart J. (2016) 37:1967–76. doi: 10.1093/eurheartj/ehw148
158. Wu D-N, Guan L, Jiang Y-X, Ma S-H, Sun Y-N, Lei H-T, et al. Microbiome and metabonomics study of quercetin for the treatment of atherosclerosis. Cardiovasc Diagn Ther. (2019) 9:545–60. doi: 10.21037/cdt.2019.12.04
159. Lind L. A detailed lipoprotein profile in relation to intima-media thickness and echogenicity of three major arteries. Clin Physiol Funct Imaging. (2019) 39:415–21. doi: 10.1111/cpf.12594
160. Vojinovic D, van der Lee SJ, van Duijn CM, Vernooij MW, Kavousi M, Amin N, et al. Metabolic profiling of intra- and extracranial carotid artery atherosclerosis. Atherosclerosis. (2018) 272:60–5. doi: 10.1016/j.atherosclerosis.2018.03.015
161. US Food and Drug Administration Office of In Vitro Diagnostic Device Eval uation and Safety. K063841 NMR Profiler and NMR Lipo Profile Test. (2008). Available online at: http://www.accessdata.fda.gov/cdrh_docs/pdf6/K063841.pdf (accessed July 08, 2008).
Keywords: quantitative nuclear magnetic resonance, metabolomics, biomarker, lipoprotein, atherosclerosis, cardiovascular disease
Citation: Ma S, Xia M and Gao X (2021) Biomarker Discovery in Atherosclerotic Diseases Using Quantitative Nuclear Magnetic Resonance Metabolomics. Front. Cardiovasc. Med. 8:681444. doi: 10.3389/fcvm.2021.681444
Received: 16 March 2021; Accepted: 02 July 2021;
Published: 28 July 2021.
Edited by:
Vasily Sukhorukov, Research Institute of Human Morphology, RussiaReviewed by:
Raul Maranhão, Universidade de São Paulo, BrazilCopyright © 2021 Ma, Xia and Gao. This is an open-access article distributed under the terms of the Creative Commons Attribution License (CC BY). The use, distribution or reproduction in other forums is permitted, provided the original author(s) and the copyright owner(s) are credited and that the original publication in this journal is cited, in accordance with accepted academic practice. No use, distribution or reproduction is permitted which does not comply with these terms.
*Correspondence: Xin Gao, aGFwcHkyMDA2MTIwOEAxMjYuY29t; emhvbmdzaGFuX2VuZG9AMTI2LmNvbQ==
Disclaimer: All claims expressed in this article are solely those of the authors and do not necessarily represent those of their affiliated organizations, or those of the publisher, the editors and the reviewers. Any product that may be evaluated in this article or claim that may be made by its manufacturer is not guaranteed or endorsed by the publisher.
Research integrity at Frontiers
Learn more about the work of our research integrity team to safeguard the quality of each article we publish.