- 1Department of Cardiothoracic Surgery, Stanford University, Stanford, CA, United States
- 2Department of Mechanical Engineering, Stanford University, Stanford, CA, United States
- 3Department of Bioengineering, Stanford University, Stanford, CA, United States
- 4University Department of Cardiac Surgery, Leipzig Heart Center, Leipzig, Germany
The field of heart valve biomechanics is a rapidly expanding, highly clinically relevant area of research. While most valvular pathologies are rooted in biomechanical changes, the technologies for studying these pathologies and identifying treatments have largely been limited. Nonetheless, significant advancements are underway to better understand the biomechanics of heart valves, pathologies, and interventional therapeutics, and these advancements have largely been driven by crucial in silico, ex vivo, and in vivo modeling technologies. These modalities represent cutting-edge abilities for generating novel insights regarding native, disease, and repair physiologies, and each has unique advantages and limitations for advancing study in this field. In particular, novel ex vivo modeling technologies represent an especially promising class of translatable research that leverages the advantages from both in silico and in vivo modeling to provide deep quantitative and qualitative insights on valvular biomechanics. The frontiers of this work are being discovered by innovative research groups that have used creative, interdisciplinary approaches toward recapitulating in vivo physiology, changing the landscape of clinical understanding and practice for cardiovascular surgery and medicine.
Introduction
Valvular heart disease is a significant cause of global morbidity and mortality, with a prognosis rivaling many types of cancer (1). While there are a wide variety of repair operations and devices, most of these strategies have been historically based upon anatomy and subjective visual appearance, and quantitative mechanical foundations have been limited as interventional insights have largely been driven by clinical outcomes. Moreover, many valvular pathologies are rooted in biomechanical changes, yet the technologies for studying these pathologies and identifying treatments have largely been limited. This disconnect is particularly evident in the lack of surgeon consensus regarding debates such as optimal repair techniques and mechanisms behind disease pathologies and operations (2–5). However, significant advancements have been made to better understand heart valves and have largely been driven by in silico, ex vivo, and in vivo
modeling technologies, which have provided the underlying platforms for generating many new analyses and insights. In particular, ex vivo modeling represents an especially promising class of translatable research that leverages the advantages from both in silico and in vivo modeling to provide deep quantitative and qualitative understandings of valvular biomechanics. Each of these modalities has unique advantages and disadvantages, and presented is a review of recent, impactful developments in the field, including the key outlooks and limitations of the technologies.
In Silico Modeling
Computational simulations have been a major driving force for generating greater intuition of heart valve mechanics. As imaging modalities and mechanical characterization of biological systems have improved, so have the relevance and realism of these models to better predict physiologic outcomes. A particular area of study that has seen major advancements from in silico, image-based modeling is that of MV dynamics. Pioneering the clinical application of new computational technologies, researchers have used real-time 3D echocardiography to generate patient-specific computational models of the MV (6–11). Using these models, publications have revealed pathological dynamics and generated useful quantifications based on valvular geometry. For example, by characterizing mitral annulus motion, researchers found that the cyclic, saddle-shape conformational changes of the annulus are important for efficient operation of the valve, reducing systolic strains on the posterior leaflet and peak leaflet stresses, potentially improving long-term durability (12, 13). These findings have large implications for informing clinical practice, as the annuloplasty procedure, which often results in fixing the mitral annulus, is the most common MV surgical intervention (6, 8). By correlating native mechanics to pathological annular biomechanical changes via image-based computational modeling, these studies have compared annuloplasty rings and identified areas of surgical intervention for improving care. Additional studies that model the MV have provided valuable insights describing the physiologic, pathologic, and repaired operation of the valve (14–21).
The study of prosthetic valvular replacements, particularly of the AV, is another area where in silico models are making great strides. Using computational flow or imaging-based models, researchers have created unique metrics that evaluate valvular replacement performance, addressing aspects such as leaflet thrombosis, abnormal flow patterns, blood damage, hinge design outcomes, paravalvular leakage, and left ventricular dynamics, while also creating novel preoperative, patient-specific planning protocols and prediction tools for surgical or transcatheter interventions (22–32). These models provide detailed views through unique quantifications, developing deeper intuition behind the valvular mechanics. They have been crucial for understanding complex phenomena, such as the value of a bi-leaflet prosthetic or the effect of neosinuses on the downstream aortic wall shear stresses and turbulence, and are providing useful new perspectives for comparing treatment options (22–24, 33).
Specifically regarding the AV, in silico modeling has enabled the simulation and study of many relevant mechanical parameters, leading to a detailed, new understanding of the native physiology as well as complex pathologies. Many innovative models have been developed and utilized to answer questions regarding, for example, hemodynamic anomalies and surgical repair techniques (34–36). In particular, a series of computational studies aimed at researching the bicuspid AV pathology has elucidated new insights such as the phenomena that specific bicuspid AV geometries induce regions of high stress concentrations in the leaflets, which may influence the mechanics of long-term degradation as well as leaflet calcification (37–39). These studies, coupled with hemodynamic simulations that can identify the temporal effects of blood flow on the AV leaflets, have generated powerful tools for comparing healthy and structural disease states with computationally generated and, in some cases, patient-specific models (40–42). This work highlights the power of in silico modeling: creating new connections between phenomena that would otherwise be unintuitive to understand and difficult to detect and setting a new benchmark of material and actionable mechanical data.
In silico models have many unique advantages for studying the heart. Specifically, by using computational models, researchers have generated unique comparisons, visualizations, and quantifications that would otherwise be very difficult to do. For example, using a lattice-Boltzmann method, a recent publication outlined the dynamics and surface shear stresses on a specific prosthetic valve hinge at an individual blood platelet level of granularity, generating a blood damage index based on a linear shear stress-exposure time model (23). Such metrics are almost impossible to quantify without computational modeling. However, in silico models require many unifying, homogenous assumptions, such as cellular response, parameter identification of tissue properties, and muscle fiber interactions, which can be quite far from the heterogeneous, patient-specific, in vivo physiology (43–45). While work is being done to incorporate neural networks for building accelerated, enhanced models, their unifying assumptions are often obstacles for generating accurate, relevant conclusions (46, 47). Regardless, in silico modeling provides a truly unique value proposition for greater biomechanical intuition of the heart, and these studies demonstrate the foundational research that has guided further experimentation and, ultimately, clinical practice.
Ex vivo Modeling
Ex vivo heart simulators allow researchers to study heart biomechanics with a high level of accuracy and control and are powerful tools for better understanding native physiology, pathologies, and treatments. This modality usually involves mounting explanted large animal valves in fluid filled chambers and/or flow loops, driving physiologic flows with a pulsatile linear piston pump (Figure 1). These simulators are often instrumented with flow and pressure sensors to measure hemodynamics, and valves can be imaged using echocardiography, videography, or MRI. Moreover, many of these systems have leveraged the use of a symbiotic, multimodal approach with computational strategies, which have provided critical biomechanical insights, particularly regarding deeper hemodynamic understanding, using technology such as particle image velocimetry (48–52). This comprehensive modeling approach has allowed for the use of more sophisticated computationally derived data, which when applied to ex vivo systems, provides more detailed quantitative analyses.
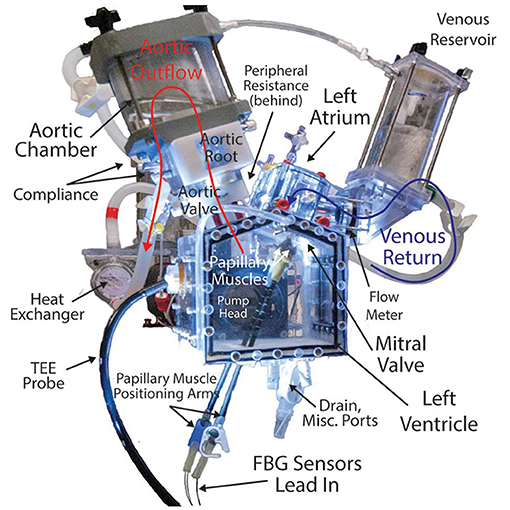
Figure 1. Detailed, labeled image of a left heart simulator. This system uses explanted large animal heart valves and a piston pump to generate and measure physiologic pressures and flows through the valves to study disease pathologies, repair techniques, and surgical devices. Simulators such as these provide useful platforms for modeling heart valve biomechanics and represent a promising new class of research in this field, directly informing clinical understandings and practice.
Much of the early work of ex vivo modeling focused on mitral, aortic, and tricuspid valvular physiology and biomechanics (50, 53–60). Building on this technology, researchers have studied a wide variety of phenomena including coaptation area, mitral and tricuspid annular geometry, mechanisms behind regurgitation, and transcatheter prosthetic valve design and deployment strategies (61–78). Many of these publications have changed the understandings of key interactions, particularly those of MV annulus dynamics and the downstream effects on MR and annuloplasty repair. In a series of studies, researchers decoupled the effects of the mitral annulus and leaflet geometry, demonstrating that the D-shaped annulus allows for more efficient LV filling by minimizing energy dissipation (58, 61, 79, 80). Extrapolating further, a recent publication focused on creating a dynamically contracting mitral annulus to recapitulate the natural annular changes. With stereophotogrammetry, the authors tracked the anterior leaflet of a porcine MV, identifying increased leaflet strain when implanting a rigid annuloplasty ring (81).
Novel research is expanding the capabilities and applications of ex vivo modeling, focusing on generating immediately, clinically translatable insights for valvular treatment and surgical care. In a series of recent studies, researchers evaluated the biomechanical effects of apical and papillary neochord anchoring locations for MR repair, exploring the mechanical outcomes of novel percutaneous neochordoplasty technologies (82, 83). These studies were enabled by the development of optical fiber-based force-sensing neochordae, which provide minimally invasive, highly sensitive chordal strain measurements (84). It was determined that apical neochord anchoring increases the rate of loading on the surrounding chordae and neochord, suggesting increased stresses on and long-term durability concerns for the MV apparatus (83). This work was directly relevant for percutaneous neochordoplasty technologies, as the results provided mechanical bases that possibly corroborated early clinical results. A follow-up study proposed a new device design to alleviate these increased loading rates by introducing an elastic transapical artificial papillary muscle that shortens the length of the chord and dissipates sharp increases in force (85). Studies such as these demonstrate the transformative nature of ex vivo simulation, altering the clinical landscape by providing convincing new evidences for informing surgical and interventional care, which are driving how treatments are being developed and evaluated (86–90).
The strength of ex vivo experimentation can be seen in a series of AV studies that directly compares treatment techniques for VSARR replacement via their biomechanical outcomes (Figure 2). By experimenting with many different clinically used conduit configurations in a heart simulator, researchers were able to identify the subtle hemodynamic differences between repair strategies, bringing quantitative understanding to a topic that was previously, largely based on surgeon preference (91, 92). Specifically, using a combination of pressure, flow, echocardiographic, and high-speed videometric data, the researchers were able to derive a variety of biomechanical parameters including hemodynamics, graft compliance, leaflet kinematics (i.e., mean cusp opening and closing velocity and relative cusp opening and closing force), aortic root distensibility, and pulsed- and continuous-wave Doppler data. From these studies, it was concluded that straight grafts were associated with lower regurgitant fractions and more favorable leaflet mechanics, specifically lower cusp opening and closing velocities and relative forces (p ≤ 0.01 for each), most closely recapitulating native aortic root biomechanics. Additionally, work on novel AR models has led to detailed understandings behind disease mechanisms, such as cusp prolapse, bicuspid AV, and root aneurysm, and repairs, such as free margin suspension, free margin plication, and VSARR, which will lead to important insights on optimal repair strategies for AR (93–95). In these studies, while a wide variety of hemodynamic parameters were measured and extracted from pressure and flow data, regurgitation was specifically derived from flow measurements through the AV by integrating flow through the aorta during diastole. Moreover, pulsed- and continuous-wave Doppler, measured via echocardiography, provided further biomechanical data regarding regurgitation, peak velocity, peak gradient, and mean gradient. By combining ex vivo modeling with clinical guidance and expertise, research groups are leveraging physiologic heart simulators to biomechanically inform surgical practice, guiding optimal treatment strategies and exponentially advancing patient care.
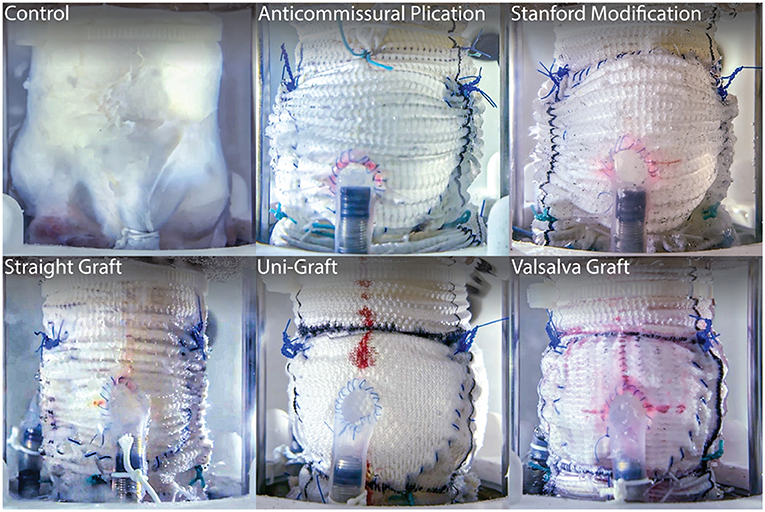
Figure 2. Long-axis view of five clinically used conduit configurations for VSARR, mounted within an ex vivo left heart simulator and attached to coronary circulation. Ex vivo experimentation allows for highly controlled, direct comparisons of surgical techniques, helping to identify optimal treatments and prostheses. Reprinted from Paulsen et al. (91).
While these technologies have provided incredible insights, ex vivo modeling is primarily limited by obstacles in physiologic accuracy and sensing capability. Since this modality uses explanted large animal tissues, fidelity is lost in not only the differences between animal and human hearts but also the inabilities to replicate entire systems and kinematics. An example that highlights this discrepancy is the phase mismatch limitation, which describes the condition where pulsatile piston pumps will initiate expansion of the tissues during systole, when in reality, heart tissue contracts. Regarding sensing capabilities, much work is being done to improve the quantitative metrics measured and derived from ex vivo systems. Examples include minimally invasive optical chordal force sensors and coaptation force distribution load sensors (84, 96). These technologies, adapted for heart simulation, can provide deeper insights and improved quantification. Moreover, expanding work has been spearheaded by trailblazing groups that have pushed the edge of capability in the effort to generate greater clinical and physiologic relevance of ex vivo simulation.
Two recent studies have pushed the frontiers, leading to a new level of realism for valvular modeling. A significant limitation of current MV simulation is that systems are unable to include the complex motion of the papillary muscles, which have been shown to translate and rotate with every heartbeat in vivo (97). These lost interactions crucially affect the motion and forces on the chordae tendineae and leaflets and play an important role for valve function and accurate ex vivo modeling. However, in a recent pioneering publication, researchers developed a biomimetic robotic system that captured and replicated this motion ex vivo (98). These robots use a six degree-of-freedom motion mechanism that allows for full tracking of the papillary muscle motion in three translation and three rotational axes, showing the effective capability to actuate any physical trajectory within its workspace. This system represents a major advancement in modeling the MV and provides a platform for closer simulation of true physiologic accuracy.
Another monumental achievement in heart simulation presented the development of an organosynthetic, biohybrid heart model that uses custom molded silicone and advanced tissue adhesion capabilities to fabricate a synthetic ventricular myocardium on an explanted porcine heart (99). Researchers implanted pneumatic actuators as the contractile elements in the synthetic myocardium to drive heart function. The model's fidelity was evaluated using echocardiography and 4D MRI and was found to have many geometric and hemodynamic similarities to in vivo images. This organosynthetic heart provides an advanced, highly accurate, contractile-based flow generation mechanism that can replace state-of-the-art piston pumps. Specifically, by introducing silicone-embedded contractile elements, this innovative model addresses the inverted phase limitation, one of the largest obstacles of current systems. While integrated sensors can provide hemodynamic data, the most exciting potential is the use of this technology with MRI imaging, as the pneumatically driven actuators allow for a metal-free system. This innovation enables access to a host of MRI-based biomechanical metrics such as high-fidelity 4D flow imaging and analysis, in a geometrically accurate and dynamic left ventricular chamber, leading the way for a new generation of hyper-realistic, ex vivo heart simulation technology.
In vivo Modeling
While in silico modeling and ex vivo modeling provide many valuable insights for developing fundamental and rapid understanding of the heart and valves, large-animal in vivo modeling remains the closest among these modalities at studying physiological truth. Large-animal modeling has provided a valuable platform in the valvular biomechanics research ecosystem, and while its primary, traditional use case has been informing surgical technique or device development, pioneering work with implanted, instrumented sensing has shown the potential for using these models to inform the fundamental biomechanics as well.
Early work using large animals primarily centered around ischemic modeling, as coronary vasculature ligation or percutaneous ethanol injection provides straightforward mechanisms for inducing targeted infarction and subsequent ischemia (100–104). In studying ischemic valvular biomechanics, researchers have used these models to analyze a wide variety of phenomena such as ischemic remodeling of MV annular dynamics, surgical techniques, such as posterior leaflet augmentation and chordal cutting, the relationship between ischemic MR and ventricular remodeling, and the effects of annuloplasty ring selection and sizing for ischemic MR repair (102, 103, 105–113). While studies such as these have shown the power of in vivo modeling capabilities, there are some key limitations with this line of research. Primarily, working with large-animal models comes with much greater financial costs, limited sample sizes due to administrative humane animal care protocols, and surgical coordination required for developing animal models and conducting in vivo experiments, and as such there are few laboratories in the world equipped to execute these kinds of studies. Moreover, the usefulness of in vivo experimentation is greatly limited by the number of reliable valvular large-animal disease models, and unfortunately, not many exist (114). However, other works have targeted the expansion of these models including an annular dilation-based, non-ischemic MR model using multiple annular incisions and a mitral leaflet flail model using chordal transection to induce leaflet prolapse and subsequent acute MR (104, 115). Outside of the MV, researchers have worked on a variety of different in vivo techniques including pacing-induced tachycardia to generate dilated cardiomyopathy and subsequent valvular regurgitation, valvular lesions to generate aortic constriction, and surgically altered leaflets to generate coaptation failure and AR (114, 116–121).
While much progress is being made to develop new in vivo models, biomechanics-focused work aims to generate implantable instrumentation for improved quantification and analysis of underlying phenomena. In a series of studies, researchers developed and utilized a mitral annulus force transducer that measures in-plane radial forces resulting from annular contraction (122). This has led to several lines of experimentation aimed at characterizing mitral annular dynamics in native, pathological, and repair scenarios (123–129). Another instrumented, in vivo biomechanics study translates the fiber Bragg grating neochordae, which directly measures chordal forces, for in vivo use. These sensors were implanted in a rare ovine case of functional MR, and chordal force profiles were generated for pathological and restored conditions post-annuloplasty. This was the first in vivo study to show that mitral ring annuloplasty reduces elevated chordal forces associated with chronic functional MR (130). Publications such as these reveal the potential for implantable, in vivo instrumentation to inform valvular biomechanics and surgical repair.
Comparison of the Modeling Modalities
In silico modeling, ex vivo modeling, and in vivo modeling represent the major capabilities for better understanding and engineering for valvular heart disease. These three modalities comprise the modern ecosystem driving valvular biomechanics research. While each has its own strengths and weaknesses, used in a collaborative, comprehensive manner, modeling technologies can allow for dramatic expansion of this field. The strength of in silico modeling lies in the ability to generate numerous new insights and ideas based on a finite element level approach and unique and intuitive quantifications and visualizations, supported by a plethora of data and mathematically derived biomechanical metrics. While these models face challenges, specifically regarding compounding inaccuracies due to homogenized, unifying assumptions that can result in weaker physiologic relevance, the types of questions and hypotheses that can be evaluated in silico are distinctively expansive and can provide the basis for deeper investigations. On the other hand, in vivo modeling sits on the opposite end of the spectrum in many regards. While these models are almost unparalleled in their physiologic accuracy, they are largely limited by cost, smaller sample sizes, surgical expertise requirements, availability of collaborative interdisciplinary engineering and surgical infrastructure, and the number of reproducible disease models. However, ex vivo modeling has the potential to bridge the translational gap for research in this field, leveraging the advantages of both in silico and in vivo modalities to create an unprecedented level of cost-effective, rapidly iterable, physiologic accuracy. These models have proven to be instrumental to modern research of valvular biomechanics, especially in their ability to immediately inform and improve clinical care.
Conclusions
The field of heart valve biomechanics is a rapidly expanding, highly clinically relevant area of research that has been driven by crucial in silico, ex vivo, and in vivo modeling technologies. These modalities represent cutting-edge abilities for generating novel insights regarding native, disease, and repair physiologies, and each has unique advantages and limitations for advancing study in this field. However, leveraged in a collaborative, comprehensive manner, modeling technologies can allow researchers to expand the holistic understanding of heart valves from a wide variety of perspectives, developing critical intuition of these complex systems. Moreover, research in valvular biomechanics has a particularly direct link to clinical translation, as new findings are often immediately and directly manifested and implemented in surgical practice, altering patient outcomes.
In particular, novel ex vivo modeling technologies represent an especially promising class of translatable research, providing unique quantitative and qualitative insights on valvular biomechanics. Research that aims to develop new technology adapted for ex vivo heart simulation has expanded the capabilities and usefulness of these systems, boosting the clinical and physiologic relevance of this modality while maintaining enough control and measurement ability to generate insightful new quantifications. Overall, the frontiers of this work are being driven by innovative research groups that have used creative, interdisciplinary approaches toward recapitulating in vivo physiology, changing the landscape of many related disciplines and positively affecting the lives of patients worldwide.
Author Contributions
All authors listed have made a substantial, direct, and intellectual contribution to the work and approved it for publication.
Funding
This research was supported by the National Institutes of Health (NIH R01 HL089315-01 and NIH R01 HL152155, YW), American Heart Association (17POST33410497, MJP and 18POST3399022, HW), the Thoracic Surgery Foundation Resident Research Fellowship (YZ), and the National Science Foundation Graduate Research Fellowship Program (AI-M).
Conflict of Interest
The authors declare that the research was conducted in the absence of any commercial or financial relationships that could be construed as a potential conflict of interest.
Abbreviations
MV, mitral valve; AV, aortic valve; LV, left ventricle; MR, mitral regurgitation; AR, aortic regurgitation; VSARR, valve-sparing aortic root replacement; MRI, magnetic resonance imaging.
References
1. Nkomo VT, Gardin JM, Skelton TN, Gottdiener JS, Scott CG, Enriquez-Sarano M. Burden of valvular heart diseases: a population-based study. Lancet. (2006) 368:1005–11. doi: 10.1016/S0140-6736(06)69208-8
2. Kassab GS, An G, Sander EA, Miga MI, Guccione JM, Ji S, et al. augmenting surgery via multi-scale modeling and translational systems biology in the era of precision medicine: a multidisciplinary perspective. Ann Biomed Eng. (2016) 44:2611–25. doi: 10.1007/s10439-016-1596-4
3. Ayoub S, Ferrari G, Gorman RC, Gorman JH, Schoen FJ, Sacks MS. Heart valve biomechanics and underlying mechanobiology. Compr Physiol. (2016) 6:1743–80. doi: 10.1002/cphy.c150048
4. Sacks MS, David Merryman W, Schmidt DE. On the biomechanics of heart valve function. J Biomech. (2009) 42:1804–24. doi: 10.1016/j.jbiomech.2009.05.015
5. Sacks MS, Yoganathan AP. Heart valve function: a biomechanical perspective. Philos Trans R Soc Lond B Biol Sci. (2007) 362:1369–91. doi: 10.1098/rstb.2007.2122
6. Levack MM, Jassar AS, Shang EK, Vergnat M, Woo YJ, Acker MA, et al. Three-dimensional echocardiographic analysis of mitral annular dynamics: implication for annuloplasty selection. Circulation. (2012) 126:S183–8. doi: 10.1161/CIRCULATIONAHA.111.084483
7. Wijdh-den Hamer IJ, Bouma W, Lai EK, Levack MM, Shang EK, Pouch AM, et al. The value of preoperative 3-dimensional over 2-dimensional valve analysis in predicting recurrent ischemic mitral regurgitation after mitral annuloplasty. J Thorac Cardiovasc Surg. (2016) 152:847–59. doi: 10.1016/j.jtcvs.2016.06.040
8. Bouma W, Lai EK, Levack MM, Shang EK, Pouch AM, Eperjesi TJ, et al. Preoperative three-dimensional valve analysis predicts recurrent ischemic mitral regurgitation after mitral annuloplasty. Ann Thorac Surg. (2016) 101:567–75. doi: 10.1016/j.athoracsur.2015.09.076
9. Meijerink F, Wijdh-den Hamer IJ, Bouma W, Pouch AM, Aly AH, Lai EK, et al. Intraoperative post-annuloplasty three-dimensional valve analysis does not predict recurrent ischemic mitral regurgitation. J Cardiothorac Surg. (2020) 15:161. doi: 10.1186/s13019-020-01138-7
10. Siefert AW, Rabbah J-PM, Saikrishnan N, Kunzelman KS, Yoganathan AP. Isolated effect of geometry on mitral valve function for in silico model development. Comput Methods Biomech Biomed Engin. (2015) 18:618–27. doi: 10.1080/10255842.2013.822490
11. Grbic S, Easley TF, Mansi T, Bloodworth CH, Pierce EL, Voigt I, et al. Personalized mitral valve closure computation and uncertainty analysis from 3D echocardiography. Med Image Anal. (2017) 35:238–49. doi: 10.1016/j.media.2016.03.011
12. Padala M, Hutchison RA, Croft LR, Jimenez JH, Gorman RC, Gorman JH, et al. Saddle shape of the mitral annulus reduces systolic strains on the P2 segment of the posterior mitral leaflet. Ann Thorac Surg. (2009) 88:1499–504. doi: 10.1016/j.athoracsur.2009.06.042
13. Salgo IS Gorman JH Gorman RC Jackson BM Bowen FW Plappert T. Effect of annular shape on leaflet curvature in reducing mitral leaflet stress. Circulation. (2002) 106:711–7. doi: 10.1161/01.cir.0000025426.39426.83
14. Siefert AW, Icenogle DA, Rabbah J-PM, Saikrishnan N, Rossignac J, Lerakis S, et al. Accuracy of a mitral valve segmentation method using J-splines for real-time 3D echocardiography data. Ann Biomed Eng. (2013) 41:1258–68. doi: 10.1007/s10439-013-0784-8
15. Herrmann TA, Siefert AW, Pressman GS, Gollin HR, Touchton SA, Saikrishnan N, et al. In vitro comparison of Doppler and catheter-measured pressure gradients in 3D models of mitral valve calcification. J Biomech Eng. (2013) 135:94502. doi: 10.1115/1.4024579
16. Toma M, Einstein DR, Kohli K, Caroll SL, Bloodworth CH, Cochran RP, et al. Effect of edge-to-edge mitral valve repair on chordal strain: fluid-structure interaction simulations. Biology. (2020) 9:173. doi: 10.3390/biology9070173
17. Ryan L, Jackson B, Parish L, Sakamoto H, Plappert T, Sutton MSJ, et al. Quantification and localization of mitral valve tenting in ischemic mitral regurgitation using real-time three-dimensional echocardiography. Eur J Cardiothorac Surg. (2007) 31:839–4. doi: 10.1016/j.ejcts.2007.01.050
18. Pierce EL, Bloodworth CH, Naran A, Easley TF, Jensen MO, Yoganathan AP. Novel method to track soft tissue deformation by micro-computed tomography: application to the mitral valve. Ann Biomed Eng. (2016) 44:2273–81. doi: 10.1007/s10439-015-1499-9
19. Yap C-H, Thiele K, Wei Q, Santhanakrishnan A, Khiabani R, Cardinale M, et al. Novel method of measuring valvular regurgitation using three-dimensional nonlinear curve fitting of Doppler signals within the flow convergence zone. IEEE Trans Ultrason Ferroelectr Freq Control. (2013) 60:1295–311. doi: 10.1109/TUFFC.2013.2704
20. Pierce EL, Rabbah JPM, Thiele K, Wei Q, Vidakovic B, Jensen MO, et al. Three-dimensional field optimization method: gold-standard validation of a novel color doppler method for quantifying mitral regurgitation. J Am Soc Echocardiogr. (2016) 29:917–25. doi: 10.1016/j.echo.2016.05.009
21. Noack T, Janietz M, Lurz P, Kiefer P, Sieg F, Marin-Cuartas M, et al. Dynamic mitral valve geometry in patients with primary and secondary mitral regurgitation: implications for mitral valve repair†. Eur J Cardiothorac Surg. (2019) 56:983–92. doi: 10.1093/ejcts/ezz096
22. Midha PA, Raghav V, Sharma R, Condado JF, Okafor IU, Rami T, et al. The fluid mechanics of transcatheter heart valve leaflet thrombosis in the neosinus. Circulation. (2017) 136:1598–609. doi: 10.1161/CIRCULATIONAHA.117.029479
23. Yun BM, Wu J, Simon HA, Arjunon S, Sotiropoulos F, Aidun CK, et al. A numerical investigation of blood damage in the hinge area of aortic bileaflet mechanical heart valves during the leakage phase. Ann Biomed Eng. (2012) 40:1468–85. doi: 10.1007/s10439-011-0502-3
24. Simon HA, Ge L, Borazjani I, Sotiropoulos F, Yoganathan AP. Simulation of the three-dimensional hinge flow fields of a bileaflet mechanical heart valve under aortic conditions. Ann Biomed Eng. (2010) 38:841–53. doi: 10.1007/s10439-009-9857-0
25. Keshavarz-Motamed Z, Khodaei S, Rikhtegar Nezami F, Amrute JM, Lee SJ, Brown J, et al. Mixed valvular disease following transcatheter aortic valve replacement: quantification and systematic differentiation using clinical measurements and image-based patient-specific in silico modeling. J Am Heart Assoc. (2020) 9:e015063. doi: 10.1161/JAHA.119.015063
26. Raghav V, Clifford C, Midha P, Okafor I, Thurow B, Yoganathan A. Three-dimensional extent of flow stagnation in transcatheter heart valves. J R Soc Interface. (2019) 16:20190063. doi: 10.1098/rsif.2019.0063
27. Luraghi G, Rodriguez Matas JF, Migliavacca F. In silico approaches for transcatheter aortic valve replacement inspection. Expert Rev Cardiovasc Ther. (2021) 19:61–70. doi: 10.1080/14779072.2021.1850265
28. Becsek B, Pietrasanta L, Obrist D. Turbulent systolic flow downstream of a bioprosthetic aortic valve: velocity spectra, wall shear stresses, and turbulent dissipation rates. Front Physiol. (2020) 11:577188. doi: 10.3389/fphys.2020.577188
29. Luraghi G, Wu W, De Gaetano F, Rodriguez Matas JF, Moggridge GD, Serrani M, et al. Evaluation of an aortic valve prosthesis: Fluid-structure interaction or structural simulation? J Biomech. (2017) 58:45–51. doi: 10.1016/j.jbiomech.2017.04.004
30. Sadri V, Bloodworth CH, Madukauwa-David ID, Midha PA, Raghav V, Yoganathan AP. A mechanistic investigation of the EDWARDS INTUITY Elite valve's hemodynamic performance. Gen Thorac Cardiovasc Surg. (2020) 68:9–17. doi: 10.1007/s11748-019-01154-y
31. de Jaegere P, De Santis G, Rodriguez-Olivares R, Bosmans J, Bruining N, Dezutter T, et al. Patient-specific computer modeling to predict aortic regurgitation after transcatheter aortic valve replacement. JACC Cardiovasc Interv. (2016) 9:508–12. doi: 10.1016/j.jcin.2016.01.003
32. Noble C, Choe J, Uthamaraj S, Deherrera M, Lerman A, Young M. In silico performance of a recellularized tissue engineered transcatheter aortic valve. J Biomech Eng. (2019) 141:061004. doi: 10.1115/1.4043209
33. Singh-Gryzbon S, Ncho B, Sadri V, Bhat SS, Kollapaneni SS, Balakumar D, et al. Influence of patient-specific characteristics on transcatheter heart valve neo-sinus flow: an in silico study. Ann Biomed Eng. (2020) 48:2400–11. doi: 10.1007/s10439-020-02532-x
34. Pirola S, Jarral OA, O'Regan DP, Asimakopoulos G, Anderson JR, Pepper JR, et al. Computational study of aortic hemodynamics for patients with an abnormal aortic valve: The importance of secondary flow at the ascending aorta inlet. APL Bioengineering. (2018) 2:026101. doi: 10.1063/1.5011960
35. Ranga A, Bouchot O, Mongrain R, Ugolini P, Cartier R. Computational simulations of the aortic valve validated by imaging data: evaluation of valve-sparing techniques. Interact Cardiovasc Thorac Surg. (2006) 5:373–8. doi: 10.1510/icvts.2005.121483
36. Hammer PE, Chen PC, del Nido PJ, Howe RD. Computational model of aortic valve surgical repair using grafted pericardium. J Biomech. (2012) 45:1199–204. doi: 10.1016/j.jbiomech.2012.01.031
37. Jermihov PN, Jia L, Sacks MS, Gorman RC, Gorman JH, Chandran KB. Effect of geometry on the leaflet stresses in simulated models of congenital bicuspid aortic valves. Cardiovasc Eng Technol. (2011) 2:48–56. doi: 10.1007/s13239-011-0035-9
38. Cao K, Sucosky P. Computational comparison of regional stress and deformation characteristics in tricuspid and bicuspid aortic valve leaflets. Int j Numer Methods Biomed Eng. (2017) 33:2798. doi: 10.1002/cnm.2798
39. Chandra S, Rajamannan NM, Sucosky P. Computational assessment of bicuspid aortic valve wall-shear stress: implications for calcific aortic valve disease. Biomech Model Mechanobiol. (2012) 11:1085–96. doi: 10.1007/s10237-012-0375-x
40. Ge L, Sotiropoulos F. Direction and magnitude of blood flow shear stresses on the leaflets of aortic valves: is there a link with valve calcification? J Biomech Eng. (2010) 132:014505. doi: 10.1115/1.4000162
41. Cao K, Bukač M, Sucosky P. Three-dimensional macro-scale assessment of regional and temporal wall shear stress characteristics on aortic valve leaflets. Comput Methods Biomech Biomed Engin. (2016) 19:603–13. doi: 10.1080/10255842.2015.1052419
42. Youssefi P, Gomez A, He T, Anderson L, Bunce N, Sharma R, et al. Patient-specific computational fluid dynamics-assessment of aortic hemodynamics in a spectrum of aortic valve pathologies. J Thorac Cardiovasc Surg. (2017) 153:8–20.e3. doi: 10.1016/j.jtcvs.2016.09.040
43. Lee C-H, Amini R, Gorman RC, Gorman JH, Sacks MS. An inverse modeling approach for stress estimation in mitral valve anterior leaflet valvuloplasty for in-vivo valvular biomaterial assessment. J Biomech. (2014) 47:2055–63. doi: 10.1016/j.jbiomech.2013.10.058
44. Krishnamurthy G, Ennis DB, Itoh A, Bothe W, Swanson JC, Karlsson M, et al. Material properties of the ovine mitral valve anterior leaflet in vivo from inverse finite element analysis. Am J Physiol Heart Circ Physiol. (2008) 295:H1141–9. doi: 10.1152/ajpheart.00284.2008
45. Baillargeon B, Rebelo N, Fox DD, Taylor RL, Kuhl E. The living heart project: a robust and integrative simulator for human heart function. Eur J Mech A Solids. (2014) 48:38–47. doi: 10.1016/j.euromechsol.2014.04.001
46. Maher G, Parker D, Wilson N, Marsden A. Neural network vessel lumen regression for automated lumen cross-section segmentation in cardiovascular image-based modeling. Cardiovasc Eng Technol. (2020) 11:621–35. doi: 10.1007/s13239-020-00497-5
47. Maher G, Wilson N, Marsden A. Accelerating cardiovascular model building with convolutional neural networks. Med Biol Eng Comput. (2019) 57:2319–35. doi: 10.1007/s11517-019-02029-3
48. Seaman C, Akingba AG, Sucosky P. Steady flow hemodynamic and energy loss measurements in normal and simulated calcified tricuspid and bicuspid aortic valves. J Biomech Eng. (2014) 136:26575. doi: 10.1115/1.4026575
49. McNally A, Madan A, Sucosky P. Morphotype-dependent flow characteristics in bicuspid aortic valve ascending aortas: a benchtop particle image velocimetry study. Front Physiol. (2017) 8:44. doi: 10.3389/fphys.2017.00044
50. Saikrishnan N, Yap C-H, Milligan NC, Vasilyev NV, Yoganathan AP. In vitro characterization of bicuspid aortic valve hemodynamics using particle image velocimetry. Ann Biomed Eng. (2012) 40:1760–75. doi: 10.1007/s10439-012-0527-2
51. Hatoum H, Dasi LP. Spatiotemporal complexity of the aortic sinus vortex as a function of leaflet calcification. Ann Biomed Eng. (2019) 47:1116–28. doi: 10.1007/s10439-019-02224-1
52. Moore B, Dasi LP. Spatio-temporal complexity of the aortic sinus vortex. Exp Fluids. (2014) 55:1770. doi: 10.1007/s00348-014-1770-0
53. de Hart J, de Weger A, van Tuijl S, Stijnen JMA, van den Broek CN, Rutten MCM, et al. An ex vivo platform to simulate cardiac physiology: a new dimension for therapy development and assessment. Int J Artif Organs. (2011) 34:495–505. doi: 10.5301/IJAO.2011.8456
54. Yap CH, Kim H-S, Balachandran K, Weiler M, Haj-Ali R, Yoganathan AP. Dynamic deformation characteristics of porcine aortic valve leaflet under normal and hypertensive conditions. Am J Physiol Heart Circ Physiol. (2010) 298:H395–405. doi: 10.1152/ajpheart.00040.2009
55. Padala M, Sacks MS, Liou SW, Balachandran K, He Z, Yoganathan AP. Mechanics of the mitral valve strut chordae insertion region. J Biomech Eng. (2010) 132:081004. doi: 10.1115/1.4001682
56. Weiler M, Yap CH, Balachandran K, Padala M, Yoganathan AP. Regional analysis of dynamic deformation characteristics of native aortic valve leaflets. J Biomech. (2011) 44:1459–65. doi: 10.1016/j.jbiomech.2011.03.017
57. Yap CH, Saikrishnan N, Tamilselvan G, Yoganathan AP. Experimental technique of measuring dynamic fluid shear stress on the aortic surface of the aortic valve leaflet. J Biomech Eng. (2011) 133:061007. doi: 10.1115/1.4004232
58. Rabbah J-P, Saikrishnan N, Yoganathan AP. A novel left heart simulator for the multi-modality characterization of native mitral valve geometry and fluid mechanics. Ann Biomed Eng. (2013) 41:305–15. doi: 10.1007/s10439-012-0651-z
59. Yap CH, Saikrishnan N, Tamilselvan G, Vasilyev N, Yoganathan AP. The congenital bicuspid aortic valve can experience high-frequency unsteady shear stresses on its leaflet surface. Am J Physiol Heart Circ Physiol. (2012) 303:H721–31. doi: 10.1152/ajpheart.00829.2011
60. Saikrishnan N, Mirabella L, Yoganathan AP. Bicuspid aortic valves are associated with increased wall and turbulence shear stress levels compared to trileaflet aortic valves. Biomech Model Mechanobiol. (2015) 14:577–88. doi: 10.1007/s10237-014-0623-3
61. Spinner EM, Buice D, Yap CH, Yoganathan AP. The effects of a three-dimensional, saddle-shaped annulus on anterior and posterior leaflet stretch and regurgitation of the tricuspid valve. Ann Biomed Eng. (2012) 40:996–1005. doi: 10.1007/s10439-011-0471-6
62. Dolensky JR, Casa LDC, Siefert AW, Yoganathan AP. In vitro assessment of available coaptation area as a novel metric for the quantification of tricuspid valve coaptation. J Biomech. (2013) 46:832–6. doi: 10.1016/j.jbiomech.2012.11.054
63. Spinner EM, Shannon P, Buice D, Jimenez JH, Veledar E, Del Nido PJ, et al. In vitro characterization of the mechanisms responsible for functional tricuspid regurgitation. Circulation. (2011) 124:920–9. doi: 10.1161/CIRCULATIONAHA.110.003897
64. Midha PA, Raghav V, Condado JF, Okafor IU, Lerakis S, Thourani VH, et al. Valve type, size, and deployment location affect hemodynamics in an in vitro valve-in-valve model. JACC Cardiovasc Interv. (2016) 9:1618–28. doi: 10.1016/j.jcin.2016.05.030
65. Vismara R, Gelpi G, Prabhu S, Romitelli P, Troxler LG, Mangini A, et al. Transcatheter edge-to-edge treatment of functional tricuspid regurgitation in an ex vivo pulsatile heart model. J Am Coll Cardiol. (2016) 68:1024–33. doi: 10.1016/j.jacc.2016.06.022
66. Pierce EL, Kohli K, Ncho B, Sadri V, Bloodworth CH, Mangan FE, et al. Novel in vitro test systems and insights for transcatheter mitral valve design, part II: radial expansion forces. Ann Biomed Eng. (2019) 47:392–402. doi: 10.1007/s10439-018-02139-3
67. Pierce EL, Sadri V, Ncho B, Kohli K, Shah S, Yoganathan AP. Novel in vitro test systems and insights for transcatheter mitral valve design, part I: paravalvular leakage. Ann Biomed Eng. (2019) 47:381–91. doi: 10.1007/s10439-018-02154-4
68. Gunning PS, Saikrishnan N, McNamara LM, Yoganathan AP. An in vitro evaluation of the impact of eccentric deployment on transcatheter aortic valve hemodynamics. Ann Biomed Eng. (2014) 42:1195–206. doi: 10.1007/s10439-014-1008-6
69. Ncho B, Sadri V, Ortner J, Kollapaneni S, Yoganathan A. In-vitro assessment of the effects of transcatheter aortic valve leaflet design on neo-sinus geometry and flow. Ann Biomed Eng. (2020) 49:1046–57. doi: 10.1007/s10439-020-02664-0
70. Kumar G, Raghav V, Lerakis S, Yoganathan AP. High transcatheter valve replacement may reduce washout in the aortic sinuses: an in-vitro study. J Heart Valve Dis. (2015) 24:22–29.
71. Trusty PM, Bhat SS, Sadri V, Salim MT, Funnell E, Kamioka N, et al. The role of flow stasis in transcatheter aortic valve leaflet thrombosis. J Thorac Cardiovasc Surg. (2020). doi: 10.1016/j.jtcvs.2020.10.139. [Epub ahead of print].
72. Amini Khoiy K, Asgarian KT, Loth F, Amini R. Dilation of tricuspid valve annulus immediately after rupture of chordae tendineae in ex-vivo porcine hearts. PLoS ONE. (2018) 13:e0206744. doi: 10.1371/journal.pone.0206744
73. Padala M, Gyoneva L, Yoganathan AP. Effect of anterior strut chordal transection on the force distribution on the marginal chordae of the mitral valve. J Thorac Cardiovasc Surg. (2012) 144:624–33.e2. doi: 10.1016/j.jtcvs.2011.10.032
74. Okafor I, Raghav V, Condado JF, Midha PA, Kumar G, Yoganathan AP. Aortic regurgitation generates a kinematic obstruction which hinders left ventricular filling. Ann Biomed Eng. (2017) 45:1305–14. doi: 10.1007/s10439-017-1790-z
75. Sadri V, Madukauwa-David ID, Yoganathan AP. In vitro evaluation of a new aortic valved conduit. J Thorac Cardiovasc Surg. (2021) 161:581–90.e6. doi: 10.1016/j.jtcvs.2019.09.181
76. Midha PA, Raghav V, Okafor I, Yoganathan AP. The effect of valve-in-valve implantation height on sinus flow. Ann Biomed Eng. (2017) 45:405–12. doi: 10.1007/s10439-016-1642-2
77. Okafor I, Raghav V, Midha P, Kumar G, Yoganathan A. The hemodynamic effects of acute aortic regurgitation into a stiffened left ventricle resulting from chronic aortic stenosis. Am J Physiol Heart Circ Physiol. (2016) 310:H1801–7. doi: 10.1152/ajpheart.00161.2016
78. Rabbah J-P, Chism B, Siefert A, Saikrishnan N, Veledar E, Thourani VH, et al. Effects of targeted papillary muscle relocation on mitral leaflet tenting and coaptation. Ann Thorac Surg. (2013) 95:621–8. doi: 10.1016/j.athoracsur.2012.09.007
79. Okafor IU, Santhanakrishnan A, Raghav VS, Yoganathan AP. Role of mitral annulus diastolic geometry on intraventricular filling dynamics. J Biomech Eng. (2015) 137:121007. doi: 10.1115/1.4031838
80. Padala M, Gyoneva LI, Thourani VH, Yoganathan AP. Impact of mitral valve geometry on hemodynamic efficacy of surgical repair in secondary mitral regurgitation. J Heart Valve Dis. (2014) 23:79–87.
81. Easley TF, Bloodworth CH, Bhal V, Yoganathan AP. Effects of annular contraction on anterior leaflet strain using an in vitro simulator with a dynamically contracting mitral annulus. J Biomech. (2018) 66:51–6. doi: 10.1016/j.jbiomech.2017.10.045
82. Paulsen MJ, Imbrie-Moore AM, Wang H, Bae JH, Hironaka CE, Farry JM, et al. Mitral chordae tendineae force profile characterization using a posterior ventricular anchoring neochordal repair model for mitral regurgitation in a three-dimensional-printed ex vivo left heart simulator. Eur J Cardiothorac Surg. (2020) 57:535–44. doi: 10.1093/ejcts/ezz258
83. Imbrie-Moore AM, Paulsen MJ, Thakore AD, Wang H, Hironaka CE, Lucian HJ, et al. Ex vivo biomechanical study of apical versus papillary neochord anchoring for mitral regurgitation. Ann Thorac Surg. (2019) 108:90–7. doi: 10.1016/j.athoracsur.2019.01.053
84. Paulsen MJ, Bae JH, Imbrie-Moore A, Wang H, Hironaka C, Farry JM, et al. Development and ex vivo validation of novel force-sensing neochordae for measuring chordae tendineae tension in the mitral valve apparatus using optical fibers with embedded Bragg gratings. J Biomech Eng. (2019) 142:0145011–9. doi: 10.1115/1.4044142
85. Imbrie-Moore AM, Zhu Y, Park MH, Paulsen MJ, Wang H, Woo YJ. Artificial papillary muscle device for off-pump transapical mitral valve repair. J Thorac Cardiovasc Surg. (2020). doi: 10.1016/j.jtcvs.2020.11.105. [Epub ahead of print].
86. Siefert AW, Rabbah J-PM, Pierce EL, Kunzelman KS, Yoganathan AP. Quantitative evaluation of annuloplasty on mitral valve chordae tendineae forces to supplement surgical planning model development. Cardiovasc Eng Technol. (2014) 5:35–43. doi: 10.1007/s13239-014-0175-9
87. Padala M, Sweet M, Hooson S, Thourani VH, Yoganathan AP. Hemodynamic comparison of mitral valve repair: techniques for a flail anterior leaflet. J Heart Valve Dis. (2014) 23:171–6.
88. Rabbah J-PM, Siefert AW, Spinner EM, Saikrishnan N, Yoganathan AP. Peak mechanical loads induced in the in vitro edge-to-edge repair of posterior leaflet flail. Ann Thorac Surg. (2012) 94:1446–53. doi: 10.1016/j.athoracsur.2012.05.024
89. Kawata M, Vasilyev NV, Perrin DP, del Nido PJ. Beating-heart mitral valve suture annuloplasty under real-time three-dimensional echocardiography guidance: an ex vivo study. Interact Cardiovasc Thorac Surg. (2010) 11:6–9. doi: 10.1510/icvts.2010.233320
90. Padala M, Cardinau B, Gyoneva LI, Thourani VH, Yoganathan AP. Comparison of artificial neochordae and native chordal transfer in the repair of a flail posterior mitral leaflet: an experimental study. Ann Thorac Surg. (2013) 95:629–33. doi: 10.1016/j.athoracsur.2012.09.055
91. Paulsen MJ, Imbrie-Moore AM, Baiocchi M, Wang H, Hironaka CE, Lucian HJ, et al. Comprehensive ex vivo comparison of 5 clinically used conduit configurations for valve-sparing aortic root replacement using a 3-dimensional-printed heart simulator. Circulation. (2020) 142:1361–73. doi: 10.1161/CIRCULATIONAHA.120.046612
92. Paulsen MJ, Kasinpila P, Imbrie-Moore AM, Wang H, Hironaka CE, Koyano TK, et al. Modeling conduit choice for valve-sparing aortic root replacement on biomechanics with a 3-dimensional-printed heart simulator. J Thorac Cardiovasc Surg. (2019) 158:392–403. doi: 10.1016/j.jtcvs.2018.10.145
93. Zhu Y, Imbrie-Moore AM, Paulsen MJ, Priromprintr B, Wang H, Lucian HJ, et al. Novel bicuspid aortic valve model with aortic regurgitation for hemodynamic status analysis using an ex vivo simulator. J Thorac Cardiovasc Surg. (2020). doi: 10.1016/j.jtcvs.2020.06.028. [Epub ahead of print].
94. Zhu Y, Imbrie-Moore AM, Paulsen MJ, Priromprintr B, Park MH, Wang H, et al. A novel aortic regurgitation model from cusp prolapse with hemodynamic validation using an ex vivo left heart simulator. J Cardiovasc Transl Res. (2020) 14:283–9. doi: 10.1007/s12265-020-10038-z
95. Zhu Y, Imbrie-Moore AM, Park MH, Paulsen MJ, Wang H, MacArthur JW, et al. Ex vivo analysis of a porcine bicuspid aortic valve and aneurysm disease model. Ann Thorac Surg. (2021) 111:e113–5. doi: 10.1016/j.athoracsur.2020.05.086
96. Adams J, O'Rourke MJ. In vitro measurement of the coaptation force distribution in normal and functional regurgitant porcine mitral valves. J Biomech Eng. (2015) 137:7. doi: 10.1115/1.4029746
97. Ni X-D, Huang J, Hu Y-P, Xu R, Yang W-Y, Zhou L-M. Assessment of the rotation motion at the papillary muscle short-axis plane with normal subjects by two-dimensional speckle tracking imaging: a basic clinical study. PLoS ONE. (2013) 8:e83071. doi: 10.1371/journal.pone.0083071
98. Imbrie-Moore AM, Park MH, Paulsen MJ, Sellke M, Kulkami R, Wang H, et al. Biomimetic six-axis robots replicate human cardiac papillary muscle motion: pioneering the next generation of biomechanical heart simulator technology. J R Soc Interface. (2020) 17:20200614. doi: 10.1098/rsif.2020.0614
99. Park C, Fan Y, Hager G, Yuk H, Singh M, Rojas A, et al. An organosynthetic dynamic heart model with enhanced biomimicry guided by cardiac diffusion tensor imaging. Sci Robot. (2020) 5:eaay9106. doi: 10.1126/scirobotics.aay9106
100. Llaneras MR, Nance ML, Streicher JT, Lima JA, Savino JS, Bogen DK, et al. Large animal model of ischemic mitral regurgitation. Ann Thorac Surg. (1994) 57:432–39. doi: 10.1016/0003-4975(94)91012-x
101. Verdouw PD, van den Doel MA, de Zeeuw S, Duncker DJ. Animal models in the study of myocardial ischaemia and ischaemic syndromes. Cardiovasc Res. (1998) 39:121–35. doi: 10.1016/s0008-6363(98)00069-8
102. Gorman JH, Jackson BM, Enomoto Y, Gorman RC. The effect of regional ischemia on mitral valve annular saddle shape. Ann Thorac Surg. (2004) 77:544–8. doi: 10.1016/S0003-4975(03)01354-7
103. Ryan LP, Jackson BM, Parish LM, Plappert TJ, St John-Sutton MG, Gorman JH, et al. Regional and global patterns of annular remodeling in ischemic mitral regurgitation. Ann Thorac Surg. (2007) 84:553–9. doi: 10.1016/j.athoracsur.2007.04.016
104. Chawla SK, Frater RWM, Cunningham M, Padala M. Performance and healing of an expanded polytetrafluoroethylene multichordal device at 6 months after repair of mitral leaflet flail in swine. J Thorac Cardiovasc Surg. (2019) 157:932–40.e3. doi: 10.1016/j.jtcvs.2018.07.103
105. Jassar AS, Minakawa M, Shuto T, Robb JD, Koomalsingh KJ, Levack MM, et al. Posterior leaflet augmentation in ischemic mitral regurgitation increases leaflet coaptation and mobility. Ann Thorac Surg. (2012) 94:1438–45. doi: 10.1016/j.athoracsur.2012.05.025
106. Sielicka A, Sarin EL, Shi W, Sulejmani F, Corporan D, Kalra K, et al. Pathological remodeling of mitral valve leaflets from unphysiologic leaflet mechanics after undersized mitral annuloplasty to repair ischemic mitral regurgitation. J Am Heart Assoc. (2018) 7:e009777. doi: 10.1161/JAHA.118.009777
107. Gorman JH, Gorman RC, Jackson BM, Enomoto Y, St John-Sutton MG, Edmunds LH. Annuloplasty ring selection for chronic ischemic mitral regurgitation: lessons from the ovine model. Ann Thorac Surg. (2003) 76:1556–63. doi: 10.1016/s0003-4975(03)00891-9
108. Messas E, Guerrero JL, Handschumacher MD, Conrad C, Chow CM, Sullivan S, et al. Chordal cutting: a new therapeutic approach for ischemic mitral regurgitation. Circulation. (2001) 104:1958–63. doi: 10.1161/hc4201.097135
109. Minakawa M, Robb JD, Morital M, Koomalsinghl KJ, Vergnat M, Gillespie MJ, et al. A model of ischemic mitral regurgitation in pigs with three-dimensional echocardiographic assessment. J Heart Valve Dis. (2014) 23:713–20.
110. Xu D, McBride E, Kalra K, Wong K, Guyton RA, Sarin EL, et al. Undersizing mitral annuloplasty alters left ventricular mechanics in a swine model of ischemic mitral regurgitation. J Thorac Cardiovasc Surg. (2020). doi: 10.1016/j.jtcvs.2020.09.141. [Epub ahead of print].
111. Matsuzaki K, Morita M, Hamamoto H, Noma M, Robb JD, Gillespie MJ, et al. Elimination of ischemic mitral regurgitation does not alter long-term left ventricular remodeling in the ovine model. Ann Thorac Surg. (2010) 90:788–94. doi: 10.1016/j.athoracsur.2010.04.008
112. Onohara D, Corporan D, Hernandez-Merlo R, Guyton RA, Padala M. Mitral regurgitation worsens cardiac remodeling in ischemic cardiomyopathy in an experimental model. J Thorac Cardiovasc Surg. (2020) 160:e107–25. doi: 10.1016/j.jtcvs.2019.06.109
113. Shi W, McIver BV, Kalra K, Sarin EL, Schmarkey S, Duggan M, et al. A swine model of percutaneous intracoronary ethanol induced acute myocardial infarction and ischemic mitral regurgitation. J Cardiovasc Transl Res. (2017) 10:391–400. doi: 10.1007/s12265-017-9751-3
114. Houser SR, Margulies KB, Murphy AM, Spinale FG, Francis GS, Prabhu SD, et al. Animal models of heart failure: a scientific statement from the American Heart Association. Circ Res. (2012) 111:131–50. doi: 10.1161/RES.0b013e3182582523
115. Yamauchi H, Feins EN, Vasilyev NV, Shimada S, Zurakowski D, Del Nido PJ. Creation of nonischemic functional mitral regurgitation by annular dilatation and nonplanar modification in a chronic in vivo swine model. Circulation. (2013) 128:S263–70. doi: 10.1161/CIRCULATIONAHA.112.000396
116. Couet J, Gaudreau M, Lachance D, Plante E, Roussel E, Drolet M-C, et al. Treatment of combined aortic regurgitation and systemic hypertension: Insights from an animal model study. Am J Hypertens. (2006) 19:843–50. doi: 10.1016/j.amjhyper.2006.01.021
117. Mori Y, Shiota T, Jones M, Wanitkun S, Irvine T, Li X, et al. Three-dimensional reconstruction of the color Doppler-imaged vena contracta for quantifying aortic regurgitation: studies in a chronic animal model. Circulation. (1999) 99:1611–7. doi: 10.1161/01.cir.99.12.1611
118. Lachance D, Plante E, Roussel E, Drolet M-C, Couet J, Arsenault M. Early left ventricular remodeling in acute severe aortic regurgitation: insights from an animal model. J Heart Valve Dis. (2008) 17:300–8.
119. Reimold SC, Aranki SF, Caguioa ES, Solomon SD, Birjiniuk V, Cohn LH, et al. An external aortic root device for decreasing aortic regurgitation: in vitro and in vivo animal studies. J Card Surg. (1994) 9:304–13. doi: 10.1111/j.1540-8191.1994.tb00849.x
120. Malinowski M, Proudfoot AG, Langholz D, Eberhart L, Brown M, Schubert H, et al. Large animal model of functional tricuspid regurgitation in pacing induced end-stage heart failure. Interact Cardiovasc Thorac Surg. (2017) 24:905–10. doi: 10.1093/icvts/ivx012
121. Duchenne J, Claus P, Pagourelias ED, Mada RO, Van Puyvelde J, Vunckx K, et al. Sheep can be used as animal model of regional myocardial remodeling and controllable work. Cardiol J. (2019) 26:375–84. doi: 10.5603/CJ.a2018.0007
122. Siefert AW, Jimenez JH, West DS, Koomalsingh KJ, Gorman RC, Gorman JH, et al. In-vivo transducer to measure dynamic mitral annular forces. J Biomech. (2012) 45:1514–6. doi: 10.1016/j.jbiomech.2012.03.009
123. Siefert AW, Jimenez JH, Koomalsingh KJ, Aguel F, West DS, Shuto T, et al. Contractile mitral annular forces are reduced with ischemic mitral regurgitation. J Thorac Cardiovasc Surg. (2013) 146:422–8. doi: 10.1016/j.jtcvs.2012.10.006
124. Siefert AW, Touchton SA, McGarvey JR, Takebayashi S, Rabbah JPM, Jimenez JH, et al. In-vivo mitral annuloplasty ring transducer: implications for implantation and annular downsizing. J Biomech. (2013) 46:2550–3. doi: 10.1016/j.jbiomech.2013.07.013
125. Pierce EL, Bloodworth CH, Imai A, Okamoto K, Saito Y, Gorman RC, et al. Mitral annuloplasty ring flexibility preferentially reduces posterior suture forces. J Biomech. (2018) 75:58–66. doi: 10.1016/j.jbiomech.2018.04.043
126. Siefert AW, Jimenez JH, Koomalsingh KJ, West DS, Aguel F, Shuto T, et al. Dynamic assessment of mitral annular force profile in an ovine model. Ann Thorac Surg. (2012) 94:59–65. doi: 10.1016/j.athoracsur.2012.02.074
127. Ncho BE, Pierce EL, Bloodworth CH, Imai A, Okamoto K, Saito Y, et al. Optimized mitral annuloplasty ring design reduces loading in the posterior annulus. J Thorac Cardiovasc Surg. (2020) 159:1766–74.e2. doi: 10.1016/j.jtcvs.2019.05.048
128. Pierce EL, Bloodworth CH, Siefert AW, Easley TF, Takayama T, Kawamura T, et al. Mitral annuloplasty ring suture forces: Impact of surgeon, ring, and use conditions. J Thorac Cardiovasc Surg. (2018) 155:131–9.e3. doi: 10.1016/j.jtcvs.2017.06.036
129. Siefert AW, Pierce EL, Lee M, Jensen MØ, Aoki C, Takebayashi S, et al. Suture forces in undersized mitral annuloplasty: novel device and measurements. Ann Thorac Surg. (2014) 98:305–9. doi: 10.1016/j.athoracsur.2014.02.036
Keywords: heart valve biomechanics, modeling modalities, heart simulation, clinical translation, surgical therapies
Citation: Park MH, Zhu Y, Imbrie-Moore AM, Wang H, Marin-Cuartas M, Paulsen MJ and Woo YJ (2021) Heart Valve Biomechanics: The Frontiers of Modeling Modalities and the Expansive Capabilities of Ex Vivo Heart Simulation. Front. Cardiovasc. Med. 8:673689. doi: 10.3389/fcvm.2021.673689
Received: 28 February 2021; Accepted: 17 May 2021;
Published: 08 July 2021.
Edited by:
Hanjoong Jo, Emory University, United StatesReviewed by:
Philippe Sucosky, Kennesaw State University, United StatesKartik Balachandran, University of Arkansas, United States
Copyright © 2021 Park, Zhu, Imbrie-Moore, Wang, Marin-Cuartas, Paulsen and Woo. This is an open-access article distributed under the terms of the Creative Commons Attribution License (CC BY). The use, distribution or reproduction in other forums is permitted, provided the original author(s) and the copyright owner(s) are credited and that the original publication in this journal is cited, in accordance with accepted academic practice. No use, distribution or reproduction is permitted which does not comply with these terms.
*Correspondence: Y. Joseph Woo, am9zd29vJiN4MDAwNDA7c3RhbmZvcmQuZWR1