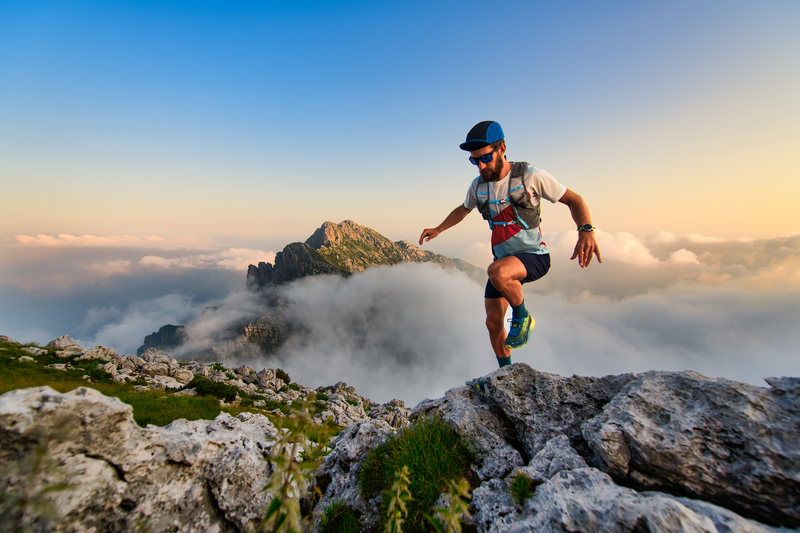
94% of researchers rate our articles as excellent or good
Learn more about the work of our research integrity team to safeguard the quality of each article we publish.
Find out more
ORIGINAL RESEARCH article
Front. Cardiovasc. Med. , 21 May 2021
Sec. Cardiovascular Surgery
Volume 8 - 2021 | https://doi.org/10.3389/fcvm.2021.672627
Background: Postoperative adverse events remain excessively high in surgical patients with coarctation of aorta (CoA). Currently, there is no generally accepted strategy to predict these patients' individual outcomes.
Objective: This study aimed to develop a risk model for the prediction of postoperative risk in pediatric patients with CoA.
Methods: In total, 514 patients with CoA at two centers were enrolled. Using daily clinical practice data, we developed a model to predict 30-day or in-hospital adverse events after the operation. The least absolute shrinkage and selection operator approach was applied to select predictor variables and logistic regression was used to develop the model. Model performance was estimated using the receiver-operating characteristic curve, the Hosmer–Lemeshow test and the calibration plot. Net reclassification improvement (NRI) and integrated discrimination improvement (IDI) compared with existing risk strategies were assessed.
Results: Postoperative adverse events occurred in 195 (37.9%) patients in the overall population. Nine predictive variables were identified, including incision of left thoracotomy, preoperative ventilation, concomitant ventricular septal defect, preoperative cardiac dysfunction, severe pulmonary hypertension, height, weight-for-age z-score, left ventricular ejection fraction and left ventricular posterior wall thickness. A multivariable logistic model [area under the curve = 0.8195 (95% CI: 0.7514–0.8876)] with adequate calibration was developed. Model performance was significantly improved compared with the existing Aristotle Basic Complexity (ABC) score (NRI = 47.3%, IDI = 11.5%) and the Risk Adjustment for Congenital Heart Surgery (RACHS-1) (NRI = 75.0%, IDI = 14.9%) in the validation set.
Conclusion: Using daily clinical variables, we generated and validated an easy-to-apply postoperative risk model for patients with CoA. This model exhibited a remarkable improvement over the ABC score and the RACHS-1 method.
Coarctation of aorta (CoA), which accounts for 6–8% of congenital heart disease (CHD), is a common disease with an incidence of about 1 in 2,500 live births (1–3). With advances in surgical technology, the peri-operative mortality has decreased to <3% (4, 5). However, the incidence of postoperative complications still remains high, at 36–68.8% (5, 6). There is no generally accepted tool to accurately predict the risk of postoperative adverse events individually in patients with CoA. Contemporary risk strategies for CHD mainly include the Aristotle Basic Complexity (ABC) score and the Risk Adjustment for Congenital Heart Surgery (RACHS-1), the Society of Thoracic Surgeons–European Association for Cardio-Thoracic Surgery Congenital Heart Surgery (STAT) mortality score and category, the STAT morbidity score and category. Such risk tools were developed on the basis of expert opinions or procedural complexity at the population-level, and focus primarily on in-hospital mortality or morbidity (6–10). Therefore, there is an unmet clinical need for comprehensive, individual assessment of CoA prognosis (10, 11).
In addition to surgical procedures, abnormal hemodynamics caused by structural malformation can lead to a series of complex pathophysiologic processes, such as hypertension, compensatory left ventricular hypertrophy, fibrosis, and cardiac insufficiency. These factors are known to contribute to the risks on mortality and morbidity (3, 12). Hence, the correlations between the patient specific influence factors and outcomes should be assessed.
Accordingly, we aimed to develop a risk model for the prediction of postoperative risk in pediatric patients with CoA, using daily clinical variables.
Figure 1 shows the flow chart of the study process we have followed to build our predictive model, carry out the data collection, and conduct the model development and validation.
Figure 1. Overview of the phases we have followed to construct the predictive model. The Lasso method used clinical data input and selected predictor variables. In the end, a postoperative risk modeled for 30-day or in-hospital adverse events after operation was developed that including multiple variables contained prognostic information. The performance of the newly developed model was compared with that of the existing risk strategies. The speech bubbles illustrate the type of input used in the Lasso method. The input examples shown are from parts of the candidate variables.
The data used to develop and validate the model came from the multi-center registration study of Prognosis and Integrative Assessment of Aortic Coarctation Patients in China (PICC) (ClinicalTrials.gov ID: NCT 04011956), and data on children undergoing CoA correction were retrospectively collected in the two centers: Center 1 (Beijing Anzhen Hospital, Beijing, China) from January 2002 to July 2020 and Center 2 (Bayi Children's Hospital affiliated to the Seventh Medical Center of PLA General Hospital, Beijing, China) from January 2012 to July 2020.
Patients were diagnosed by echocardiography and computed tomography, and they were scheduled for surgery according to the widely accepted indications for treatment (13–16). Given the informed consents of parents/legal guardians, we included patients who underwent CoA surgery in the two centers. Among all patients with CoA who underwent surgery at age≤17 years, we excluded those with complicated co-morbidities that could independently affect cardiovascular function, such as hypoplastic left heart syndrome, interruption of the aortic arch, Shone syndrome, and moderate or severe mitral stenosis, as well as patients with a history of known vasculopathy, genetic syndromes, diabetes mellitus, hyperlipoidemia or other cardiovascular risk factors. Patients with incomplete data on the variables of interest were also excluded.
The aim of our study was to predict postoperative adverse events in patients with CoA undergoing surgery correction. Postoperative adverse events were defined as death and complication. Death was defined as death for any reason during hospitalization or after discharge within 30 days after operation (11, 17), and complications were defined as previously reported (18) and exampled in the Society of Thoracic Surgeons Congenital Heart Surgery Database. The main complications considered included low cardiac output, renal dysfunction or renal failure, cardiopulmonary resuscitation, ECMO assistance, pulmonary hypertensive crisis, postoperative infection, sepsis, chylothorax, pleural effusion requiring drainage, pericardial effusion, recurrent laryngeal nerve injury, diaphragm paralysis, unplanned thoracotomy during hospitalization or within 30 days after surgery, unplanned readmission, spinal cord injury, arrhythmia, and wound infection or poor healing (detailed categories and definition were listed in Supplementary Method 1).
All clinical data were identified from electronic health records, including diagnosis, clinical status/symptoms, and operation performed. Data quality control was performed before the data analysis.
Data reflecting key pathophysiology obtained in routine clinical practice were included. Candidate variables included patient demographic and clinical characteristics, as well as imaging, surgical and laboratory variables. A total of 64 daily variables with <20% missing data that reflected the patient-specific characteristics or pathophysiological factors and were related to the prognosis were selected a priori based on the published data and clinical experiences (candidate variables and definitions are listed in Supplementary Method 2) (5, 6, 19–23).
Dataset from the Center 1 and the Center 2 were randomly separated into discovery set and validation set with a ratio of 7:3. There were 360 patients (70%) in the discovery set and 154 patients (30%) in the validation set. We used multiple imputation to handle the missing values. The number and proportion of missing data in discovery dataset were described in Supplementary Table 1. The majority of the variables had no missing information. We used 10 datasets for the multiple imputation. The model was trained in each imputed dataset. Common variables across the datasets were selected in the final model.
The least absolute shrinkage and selection operator (Lasso) regularization was used for variable selection. Stringent thresholds were used for retaining variables, which helped in the selection of a parsimonious, predictive subset of variables to train the logistic regression model, and the lambda parameter was calculated through three-fold cross-validation (the maximum value of λ is shown in Supplementary Figure 1). Then the screened variables with statistical significance (p < 0.05) in univariate logistic regression were further included as predictors to develop the multivariable model for assessing risk of outcomes with Stata software.
Receiver operating characteristic curves were used to estimate model discrimination by calculating the area under the curve (AUC). Calibration measured the agreement between the observed and predictive probabilities, and it was assessed by the Hosmer–Lemeshow test and calibration plot in this study. We defined five groups based on the quantiles of the predicted probabilities. In addition, net reclassification improvement (NRI) and integrated discrimination improvement (IDI) were used to demonstrate the improvement in the performance when comparing the developed model with the existing risk strategies. Moreover, we analyzed the predictor variables' effects using their odds ratio (OR) values and coefficients in the model. In addition, a nomogram was presented to predict the individual incidence of adverse events for each patient.
Performance was assessed in the validation set and overall study population, and sensitivity analyses were performed across subgroups by gender, age and RACHS-1 category in the datasets. Specifically, the datasets were divided into the following four age subgroups: ≤ 1month, 1–6 months, 6–12 months, and > 12 months. With regard to the RACHS-1 category, the patients were divided into three subgroups: category 1, category 2 or 3, and category 4. The prediction of adverse events was assessed to compare the models' performance in different subgroups.
Regarding the comparisons of the developed model and existing risk strategies, seven existing risk strategies, including the RACHS-1 method, the ABC score and category, the STAT mortality score and category, the STAT morbidity score and category, were applied as predictors of postoperative adverse events in the overall population (Supplementary Method 3).
For continuous variables, normally distributed variables were expressed as means ± standard deviations, and non-normally distributed variables were expressed as medians (interquartile ranges). Categorical variables were expressed as the frequencies or percentages. Continuous variables of two groups were compared by the bilateral independent t-test or the Wilcoxon test, and categorical variables were compared by chi-square test or Fisher's exact test and analyzed by Mann–Whitney U-test. Analyses were performed using Stata14.1 (StataCorp, College Station, Texas, USA), and p < 0.05 was considered statistically significant.
There were a total of 514 patients with CoA correction enrolled in PICC study as of July 2020 in Center 1 (n = 360) and Center 2 (n = 154) (Supplementary Figure 2). The overall incidence of adverse events was 37.9% (n = 195), and there was a statistically significantly difference in this incidence between the two centers (30% for Center 1 and 56.5% for Center 2, p < 0.05). The Categories and incidence rates of adverse events in Center 1 and Center 2 were listed in Supplementary Table 2, showing that there were more than 30 categories of adverse events, with slightly different distributions in the two centers.
Demographic and clinical characteristics, imaging and surgical parameters, and laboratory values were compared between patients with and without adverse events (Table 1). Patients with adverse events were more likely to be younger, to be shorter in height, to weigh less, to have a lower left ventricular ejection fraction (LVEF), to have a history of preoperative ventilation, and to have a preoperative cardiac dysfunction. In addition, adverse events were associated with concomitant ventricular septal defect (VSD) and incision other than left thoracotomy, as well as a higher RACHS-1 category and a higher ABC score.
Table 1. Baseline clinical characteristics of CoA patients with and without adverse events (N = 514)*.
There was no significant difference between the discovery set and the validation set in most of the demographic factors and clinical characteristics such as age, gender, weight, height, preoperative ventilation, preoperative cardiac dysfunction, surgical procedure type, incision, left ventricular remodeling, or in the RACHS-1 or ABC score (p > 0.05). This finding indicated that most of the baseline clinical features of the patients were similar between the discovery set and validation set (Supplementary Table 1).
Using Lasso followed by univariate logistic regression, nine variables of surgical factors, patient-specific and pathophysiological factors were identified, such as incision of left thoracotomy, preoperative ventilation, concomitant VSD, preoperative cardiac dysfunction, severe pulmonary hypertension (PH), height, weight-for-age z-score, LVEF and left ventricular posterior wall thickness (LVPW). And the final Lasso combined with logistic regression model (the Lasso model) was developed by these nine predictor variables.
The missing values for the predictor variables in the discovery set, the validation set and the overall population were demonstrated in Supplemental Table 3, the beta coefficients and ORs of the variables in the Lasso model were shown in Table 2. The adjusted OR values of the four variables—preoperative cardiac dysfunction, preoperative ventilation, severe PH, and concomitant VSD—were > 1 (2.908, 1.939, 1.684, and 1.087, respectively). The top five variables in terms of the high absolute value of their coefficients were LVPW, left thoracotomy incision, preoperative cardiac dysfunction, preoperative ventilation and severe PH. A nomogram was constructed according to the regression coefficients in the model (Figure 2) for easily and better clinical use, such as in information sharing and decision-making for both clinicians and patients.
The final Lasso model achieved an AUC of 0.8195 (95%CI: 0.7514–0.8876). Calibration of the model, assessed using the Hosmer–Lemeshow chi-square test in the validation set, indicated adequate calibration (goodness-of-fit p = 0.257). The corresponding predicted and observed adverse event rates according to the model were detailed in Figure 3A, which showed slightly higher predicted than observed rates.
Figure 3. Performance assessments of the Lasso model. (A) Calibration plot of the Lasso model in the validation set. The dashed diagonal line represents perfect calibration. Observed adverse events are shown with the corresponding 95% CIs. The x-axis is the predicted probability estimated by the model and the y-axis is the actual probability. (B) Calibration plot of the Lasso model in the overall population. There was a good agreement between the predicted and observed probabilities in the overall population. The dashed diagonal line represents perfect calibration. Observed adverse events are shown with the corresponding 95% CIs. (C) Receiver operating characteristic (ROC) curves comparing the Lasso model with the ABC score and the RACHS-1 in validation set. This comparison showed that the Lasso model outperformed the ABC score and the RACHS-1 in prediction of postoperative adverse events in the validation set (p < 0.05). (D) Receiver operating characteristic (ROC) curves comparing the Lasso model with the ABC score and the RACHS-1 in the overall population. The Lasso model outperformed the ABC score and the RACHS-1 in prediction of postoperative adverse events in the overall population (p < 0.001). Lasso, least absolute shrinkage and selection operator; ABC, Aristotle Basic Complexity; RACHS-1, Risk Adjustment for Congenital Heart Surgery.
The model calibrated well in the overall population using the Hosmer–Lemeshow test (goodness-of-fit p = 0.307). Good calibration was also shown by the closeness of the line fitting the data to the diagonal line in the calibration plot (Figure 3B). This finding indicated an agreement between the predicted probabilities and the actual observations.
In addition, to test the model's performance in a broader set of patients, the postoperative adverse events were assessed in subgroups divided by gender, age, and RACHS-1 category in the discovery set, validation set and the overall population, respectively (shown in Supplementary Figure 3). The AUCs in the subgroups were consistent with each other in all datasets (p > 0.05), which suggested the ability of the model to predict outcomes across different gender, age and RACHS-1 category subgroups. Moreover, given the potential discrepancy between predicted probabilities and outcomes in the validation set, a stratified analysis with measures of calibration as observed/expected ratios was also conducted in the overall study population. The results showed that the observed/expected ratios in the gender- and RACHS-1 category-specific subgroups were close to one, and that the ratios deviated from one in the age-specific subgroups, especially for children older than 6 months (Supplementary Table 4).
There were no statistical difference in the AUCs among the seven current clinically used risk strategies (0.6851–0.7050, p = 0.11) (Supplementary Table 5). Hence, two commonly used methods (the ABC score and RACHS-1) were adopted for further comparisons with the newly developed Lasso model. The AUC for the Lasso model (0.8195) was greater than that for either the ABC score (0.7422) or the RACHS-1 method (0.7084) in the validation set (Figure 3C) (p < 0.05 for each comparison with the Lasso model). In addition, model performances in terms of reclassification and discrimination was significantly improved compared with the ABC score (NRI = 47.3%, IDI = 11.5%) and the RACHS-1(NRI = 75.0%, IDI = 14.9%) (Table 3).
The Lasso model was superior to both of the ABC score and the RACHS-1 category when these models were applied to the overall population (AUC = 0.8131, 0.7046, and 0.6895, respectively; p < 0.001) (Figure 3D). Moreover, remarkable improvements in reclassification and discrimination was achieved in the overall population, comparing the Lasso model with the ABC score (NRI = 70.8%, IDI = 13.0%) and with the RACHS-1(NRI = 74.4%, IDI=15.3%) (Table 3).
To the best of our knowledge, this is the first study based on a large multi-center pediatric CoA cohort in China. Predictor variables from routine clinical data were identified to generate a risk prediction model.
The obstructive structural malformation of CoA can lead to a series of abnormal pathophysiology changes, such as hemodynamic abnormalities, compensatory left ventricular hypertrophy, left ventricular remodeling, subendocardial myocardial hypoperfusion, ischemia, fibrosis, and cardiac dysfunction. With the correction of the anatomical malformation and hemodynamics, left ventricular remodeling occurs, and ventricular morphology and function are improved (24, 25). Variations and variances in these processes lead to different postoperative outcomes for individual patients.
In this study, we combined the death and complications as the outcome for several reasons. First, although death and complications are adverse events of differing severity, there are many commonalities in mechanisms and pathologies, which are mainly related to three categories of factors: individual factors, operation-related factors and pathological factors. In this study, the multivariable model also reflects the impact of different factors on the outcome events. Second, as the postoperative adverse events in our composite outcomes are heterogeneous, with scattered specific outcomes and different detailed classifications due to the large sample size, it is not feasible to accurately evaluate the predictive effectiveness of the model for each outcome in an individual-level analysis.
To better reflect prognoses at the individual level compared with the currently used methods, patient-specific and pathophysiologic variables were screened using the Lasso method. Given the combined advantages of ridge regression and subset selection (26), the Lasso method has better filtering capability compared with stepwise selection (27) and can better identify the key factors influencing postoperative prognosis to generate interpretable models. In addition, logistic regression models are commonly used in the field of medical research. Therefore, we chose these methods for selecting the variables and constructing the risk model.
The developed nine-variable Lasso model was well-validated and showed significant improvement over existing risk strategies for postoperative prediction in patients with CoA. Moreover, the identified predictor variables reflecting patient-specific and pathophysiological factors were interpretable. First, the incision variable was added to reflect the surgical effects on the postoperative prognosis. Repair of CoA through left thoracotomy has been shown to have low risks of perioperative morbidity and mortality (28). The protective role is partly because of its associated isolated CoA and non-CPB conditions, which have been shown to be associated with a reduction in some adverse consequences (29). Second, preoperative ventilation has been identified as a patient-specific risk factor for patients of different ages (19) that, when combined with severe PH, may reflect the status of pulmonary circulation, perfusion, vascular resistance and function, when combined with the variable of severe PH. Therefore, the risk of various pulmonary complications (such as pulmonary infection, pneumothorax, atelectasis, respiratory insufficiency resulting in repeated intubations, and tracheotomy) will increase with preoperative ventilation. Furthermore, severe PH is thought to be associated with restrictive left ventricular physiology, accompanied by left ventricular endocardial fibrosis, and is an independent risk factor for death and readmission of heart failure (30). Third, the presence of VSD is associated with increased left ventricular volume load and pulmonary perfusion resulting from abnormal hemodynamics (31). Furthermore, median sternotomy is indicated in patients who have one-stage correction of concomitant VSD, and median sternotomy increases the risk of postoperative complications such as bleeding, chylothorax, and atelectasis (32). Fourth, as both the left ventricular systolic and diastolic function have been found to be impaired to different degrees among patients with CoA (33), and preoperative LVEF reflects the reserved function of the left ventricle, cardiac dysfunction indicated the state of decompensation. Even if structural malformations and hemodynamic abnormalities are corrected by the operation, reverse remodeling of the left ventricle would be incomplete, resulting in postoperative adverse events such as low cardiac output and pericardial effusion (34). Fifth, variables of patient-specific factors such as height, weight-for-age z-score reflect preoperative growth, nutritional status, and perfusion of systemic circulation, so a deficiency in height and weight indicates a high risk of death and other postoperative adverse events (35, 36). Finally, the variable of LVPW reflects the status of pre-operation left ventricular remodeling, but this variable was discovered also to be related to other factors such as diagnosis group (LVPW was higher in the group without VSD), age (to a certain extent reflecting the course of disease for congenital malformation), and severe PH (LVPW was greater in the non-severe PH group than in the severe PH group) in this study. In view of the above, predictor variables included in the Lasso model reflect the combined effects of the systemic pathological states and should be interpreted in an integrated manner.
Regarding the variables' weights and contributions to the model, it could be inferred from the coefficients that the variables with relatively high contribution to the model were LVPW, incision of left thoracotomy, preoperative cardiac dysfunction, preoperative ventilation and severe PH, which reflected the inherent pathophysiology including ventricular remodeling, surgery, cardiac load and function, and had good concordance with clinical findings.
In addition, we noticed that an overestimation occurred among patients with a middle level of risk in the validation dataset. We further compared the baseline characteristics of patients in middle range of the risk group between discovery and validation datasets to explore the possible reasons for this overestimation. We found that the proportion of female, as well as the levels of ALT, hemoglobin and relative wall thickness were higher in the validation dataset than in the discovery dataset (Supplementary Table 6), suggesting the existence of a difference in the populations of the discovery and validation datasets. Further validation is still needed to assess the model performance, especially among Western populations.
The main strength of our study was that, compared with the existing risk strategies, the newly developed model was able to provide better prediction of outcomes in patients with CoA by incorporating pathophysiological factors. For better understanding and more objective comparison, two postoperative evaluation methods that are commonly used in clinical practice (the ABC score and the RACHS-1 method) were applied as comparisons. Although the ABC score and the RACHS-1 method are mainly designed to evaluate the risk of death, they also reflect the state of being at high risk for postoperative complications. Our newly developed model is aimed at the composite outcome of death and complications, potentially contributing to the reduction in risks of both complications and death.
The most important issue for a clinical risk model is how it can help the clinicians and benefit the patients. Regarding our newly developed model, first, using the clinical variables collected preoperatively, an individual patient's risk of adverse events can be assessed by the model or the associated nomograph. Patients can also be informed regarding their risk factors and the incidence rates of adverse events in detail before the operation to facilitate thorough communication and appropriate decision-making together with the clinicians, which will contribute to avoiding unpredictable medical disputes later in the process. Second, our newly developed model could also be served as a tool in the identification of high-risk patients, allowing clinicians to optimize monitoring and the selection of treatment strategies, and reducing adverse events.
Nevertheless, further efforts toward precision medicine are warranted, such as either defining outcomes as certain subgroup of complications, or detecting circulatory biomarkers as an additional screening tool in risk stratification. This was an exploratory study and we hope to expand the sample size and to perform prospective research to make the findings more generalizable and applicable for clinical use in the future.
This study had several limitations. First, the data used in this study derived from two centers located in the same city; therefore the established model may not be generalizable to other centers. However, the patient population was representative, and the total sample size was relatively large. Second, the data were collected retrospectively across a relatively long time-span to attain a large sample size, it is possible that the weight of some of the risk factors considered into the model may have changed over time, and limited daily variables were available in the database. A better prediction model might be established by adding more variables and applying a different modeling approach. Third, an overestimation occurred among patients with a middle level of risk in the validation sample, which indicated the existence of a difference in the populations in the discovery and validation datasets, and further validation is still needed to assess model performance. Fourth, given that there was no existing evaluation method for the postoperative composite outcome of CoA, we chose relatively classical methods that are widely used in clinical application but that were not initially developed for the purpose of comparison; because of this, the comparison is not completely “fair”.
Using daily clinical variables, we generated and validated a postoperative risk model for pediatric patients with CoA. With significantly improved performance over the existing risk strategies to which it was compared, the newly developed model could be served as a tool for preoperative risk stratification and contribute to the reduction of adverse events.
The original contributions presented in the study are included in the article/Supplementary Materials, further inquiries can be directed to the corresponding author/s.
This study was approved by the Ethics Review Board of Beijing Anzhen Hospital and Bayi Children's Hospital affiliated to the Seventh Medical Center of PLA General Hospital, and all informed consents of parents/legal guardians were obtained.
YG conceptualized the study, carried out the analyses, and drafted the initial manuscript. YW and MJ conceptualized the study, supervised the analyses, and reviewed and revised the manuscript. JD conceptualized the study, supervised the analyses, reviewed and revised the manuscript, and was responsible for the administration of PICC registry study. QL conducted data collection and statistical analysis. PG supervised the analyses, and reviewed the manuscript. RL, WJ, and XW collected the data and conducted the literature search. GZ, JS, and XF conceptualized the study and reviewed the manuscript. All authors were involved in the manuscript review, and agree to be accountable for the content of the work.
This work was supported by the National Key R&D Program of China (Grant No. 2017YFC0908400) and the National Natural Science Foundation of China (Grant No. 82070413). JD is the Fellow of the Collaborative Innovation Center for Cardiovascular Disease Translational Medicine, Nanjing Medical University.
The authors declare that the research was conducted in the absence of any commercial or financial relationships that could be construed as a potential conflict of interest.
We thank Mr. Qiang Meng from the Department of Pediatric Cardiology, Bayi Children's Hospital affiliated to the Seventh Medical Center of PLA General Hospital, Mr. Qili Wang from the Beijing Institute of Heart, Lung and Blood Vessel Diseases, and Ms. Yifei Yang from the Department of Pediatric Heart Center, Beijing Anzhen Hospital, Capital Medical University for helping with the collection of clinical data. We also thank Mr. Bingbing Ke from the Beijing Institute of Heart, Lung and Blood Vessel Diseases for helping modifying the language of this article, and Jennifer Barrett, Ph.D., from Liwen Bianji, Edanz Editing China (www.liwenbianji.cn/ac), for editing the English text of a draft of this manuscript.
The Supplementary Material for this article can be found online at: https://www.frontiersin.org/articles/10.3389/fcvm.2021.672627/full#supplementary-material
1. Kenny D, Hijazi ZM. Coarctation of the aorta: from fetal life to adulthood. Cardiol J. (2011) 18:487–95. doi: 10.5603/cj.2011.0003
2. Ganigara M, Doshi A, Naimi I, Mahadevaiah GP, Buddhe S, Chikkabyrappa SM. Preoperative physiology, imaging, and management of coarctation of aorta in children. Semin Cardiothorac Vasc Anesth. (2019) 23:379–86. doi: 10.1177/1089253219873004
3. Baumgartner H, Bonhoeffer P, De Groot NM, de Haan F, Deanfield JE, Galie N, et al. ESC Guidelines for the management of grown-up congenital heart disease (new version 2010) The Task Force on the Management of Grown-up Congenital Heart Disease of the European Society of Cardiology (ESC). Eur Heart J. (2010) 31:2915–57. doi: 10.1093/eurheartj/ehq249
4. Serfontein SJ, Kron IL. Complications of coarctation repair. Semin Thorac Cardiovasc Surg Pediatr Card Surg Annu. (2002) 5:206–11. doi: 10.1053/pcsu.2002.31488
5. Ungerleider RM, Pasquali SK, Welke KF, Wallace AS, Ootaki Y, Quartermain MD, et al. Contemporary patterns of surgery and outcomes for aortic coarctation: an analysis of the Society of Thoracic Surgeons Congenital Heart Surgery Database. J Thorac Cardiovasc Surg. (2013) 145:150–8. doi: 10.1016/j.jtcvs.2012.09.053
6. Zeng X, An J, Lin R, Dong C, Zheng A, Li J, et al. Prediction of complications after paediatric cardiac surgery. Eur J Cardiothorac Surg. (2020) 57:350–8. doi: 10.1093/ejcts/ezz198
7. Jenkins KJ, Gauvreau K, Newburger JW, Spray TL, Moller JH, Iezzoni LI. Consensus-based method for risk adjustment for surgery for congenital heart disease. J Thorac Cardiovasc Surg. (2002) 123:110–8. doi: 10.1067/mtc.2002.119064
8. Lacour-Gayet F, Clarke D, Jacobs J, Comas J, Daebritz S, Daenen W, et al. The Aristotle score: a complexity-adjusted method to evaluate surgical results. Eur J Cardiothorac Surg. (2004) 25:911–24. doi: 10.1016/j.ejcts.2004.03.027
9. Brien SMO, Clarke DR, Jacobs JP, Jacobs ML, Lacour-Gayet FG, Pizarro C, et al. An empirically based tool for analyzing mortality associated with congenital heart surgery. J Thorac Cardiovasc Surg. (2009) 138:1139–53. doi: 10.1016/j.jtcvs.2009.03.071
10. Pasquali SK, Gaies M, Banerjee M, Zhang W, Donohue J, Russell M, et al. The quest for precision medicine: unmeasured patient factors and mortality after congenital heart surgery. Ann Thorac Surg. (2019) 108:1889–94. doi: 10.1016/j.athoracsur.2019.06.031
11. Jacobs JP. The Society of Thoracic Surgeons Congenital Heart Surgery database public reporting initiative. Semin Thorac Cardiovasc Surg Pediatr Card Surg Annu. (2017) 20:43–8. doi: 10.1053/j.pcsu.2016.09.008
12. Jarrell DK, Lennon ML, Jacot JG. Epigenetics and mechanobiology in heart development and congenital heart disease. Diseases. (2019) 7:52. doi: 10.3390/diseases7030052
13. Doshi AR, Rao PS. Coarctation of aorta-management options and decision making. Pediat Therapeut. (2012) S5:006. doi: 10.4172/2161-0665.S5-006
14. Nguyen L, Cook SC. Coarctation of the aorta. Strategies for improving outcomes. Cardiol Clin. (2015) 33:521–30vii. doi: 10.1016/j.ccl.2015.07.011
15. Dijkema EJ, Leiner T, Grotenhuis HB. Diagnosis, imaging and clinical management of aortic coarctation. Heart. (2017) 103:1148–55. doi: 10.1136/heartjnl-2017-311173
16. Meadows J, Minahan M, McElhinney DB, McEnaney K, Ringel R, COAST Investigators*. Intermediate outcomes in the prospective, multicenter coarctation of the aorta stent trial (COAST). Circulation. (2015) 131:1656–64. doi: 10.1161/CIRCULATIONAHA.114.013937
17. Jacobs JP. Introduction – databases and the assessment of complications associated with the treatment of patients with congenital cardiac disease. Cardiol Young. (2008) 18 (Suppl. 2):1–37. doi: 10.1017/S104795110800334X
18. Part IV – the dictionary of definitions of complications associated with the treatment of patients with congenital cardiac disease. Cardiol Young. (2008) 18 (Suppl. 2):282–530. doi: 10.1017/S1047951108003351
19. Jacobs JP, O'Brien SM, Pasquali SK, Kim S, Gaynor JW, Tchervenkov CI, et al. The importance of patient-specific preoperative factors: an analysis of the Society of Thoracic Surgeons Congenital Heart Surgery database. Ann Thorac Surg. (2014) 98:1653–9. doi: 10.1016/j.athoracsur.2014.07.029
20. Sudarshan CD, Cochrane AD, Jun ZH, Soto R, Brizard CP. Repair of coarctation of the aorta in infants weighing less than 2 kilograms. Ann Thorac Surg. (2006) 82:158–63. doi: 10.1016/j.athoracsur.2006.03.007
21. Brown KL, Pagel C, Ridout D, Wray J, Anderson D, Barron DJ, et al. What are the important morbidities associated with paediatric cardiac surgery? A mixed methods study. BMJ Open. (2019) 9:e028533. doi: 10.1136/bmjopen-2018-028533
22. Baggen VJM, van den Bosch AE, van Kimmenade RR, Eindhoven JA, Witsenburg M, Cuypers JAAE, et al. Red cell distribution width in adults with congenital heart disease: a worldwide available and low-cost predictor of cardiovascular events. Int J Cardiol. (2018) 260:60–5. doi: 10.1016/j.ijcard.2018.02.118
23. Brown KL, Ridout D, Pagel C, Wray J, Anderson D, Barron DJ, et al. Incidence and risk factors for important early morbidities associated with pediatric cardiac surgery in a UK population. J Thorac Cardiovasc Surg. (2019) 158:1185–96. doi: 10.1016/j.jtcvs.2019.03.139
24. Pacileo G, Pisacane C, Russo MG, Crepaz R, Sarubbi B, Tagliamonte E, et al. Left ventricular remodeling and mechanics after successful repair of aortic coarctation. Am J Cardiol. (2001) 87:748–52. doi: 10.1016/s0002-9149(00)01495-8
25. Lam YY, Kaya MG, Li W, Gatzoulis MA, Henein MY. Effect of Chronic afterload increase on left ventricular myocardial function in patients with congenital left-sided obstructive lesions. Am J Cardiol. (2007) 99:1582–7. doi: 10.1016/j.amjcard.2007.01.031
26. Tibshirani, R. Regression Shrinkage and Selection Via the Lasso. J R Stat Soc Series B (Methodological). (1996) 58:267–88. doi: 10.1111/j.2517-6161.1996.tb02080.x
27. Li QQ. Logistic and SVM credit score models based on lasso variable selection. J Appl Math Phys. (2019) 7:1131–48. doi: 10.4236/jamp.2019.75076
28. Mery CM, Guzmán-Pruneda FA, Trost JG Jr, McLaughlin E, Smith BM, Parekh DR, et al. Contemporary results of aortic coarctation repair through left thoracotomy. Ann Thorac Surg. (2015) 100:1039–46. doi: 10.1016/j.athoracsur.2015.04.129
29. Gropler MRF, Marino BS, Carr MR, Russell WW, Gu H, Eltayeb OM, et al. Long-term outcomes of coarctation repair through left thoracotomy. Ann Thorac Surg. (2019) 107:157–64. doi: 10.1016/j.athoracsur.2018.07.027
30. Lee MG, Kowalski R, Galati JC, Cheung MM, Jones B, Koleff J, et al. Twenty-four-hour ambulatory blood pressure monitoring detects a high prevalence of hypertension late after coarctation repair in patients with hypoplastic arches. J Thorac Cardiovasc Surg. (2012) 144:1110–6. doi: 10.1016/j.jtcvs.2012.08.013
31. Brouwer RM, Cromme-Dijkhuis AH, Erasmus ME, Contant C, Bogers AJ, Elzenga NJ, et al. Decision making for the surgical management of aortic coarctation associated with ventricular septal defect. J Thorac Cardiovasc Surg. (1996) 111:168–75. doi: 10.1016/S0022-5223(96)70413-0
32. Sun LZ, Luo XJ, Liu YM. Single-stage treatment of aortic coarctation and aortic valve disease. Asian Cardiovasc Thorac Ann. (2003) 11:208–12. doi: 10.1177/021849230301100306
33. Faganello G, Fisicaro M, Russo G, Iorio A, Mazzone C, Grande E, et al. Insights from cardiac mechanics after three decades from successfully repaired aortic coarctation. Congenit Heart Dis. (2016) 11:254–61. doi: 10.1111/chd.12310
34. Jashari H, Rydberg A, Ibrahimi P, Bajraktari G, Henein MY. Left ventricular response to pressure afterload in children: aortic stenosis and coarctation a systematic review of the current evidence. Int J Cardiol. (2015) 178:2 03–9. doi: 10.1016/j.ijcard.2014.10.089
35. Hager A, Schreiber C, Nutzl S, Hess J. Mortality restenosis rate of surgical coarctation repair in infancy: a study of 191 patients. Cardiology. (2009) 112:36–41. doi: 10.1159/000137697
36. Ergün S, Genç SB, Yildiz O, Öztürk E, Kafali HC, Ayyildiz P, et al. Risk factors for major adverse events after surgical closure of ventricular septal defect in patients less than 1 year of age: a single-center retrospective. Braz J Cardiovasc Surg. (2019) 34:335–43. doi: 10.21470/1678-9741-2018-0299
Keywords: prediction, prognosis, risk stratification, coarctation of aorta, congenital heart disease
Citation: Gu Y, Li Q, Lin R, Jiang W, Wang X, Zhou G, Su J, Fan X, Gao P, Jin M, Wang Y and Du J (2021) Prognostic Model to Predict Postoperative Adverse Events in Pediatric Patients With Aortic Coarctation. Front. Cardiovasc. Med. 8:672627. doi: 10.3389/fcvm.2021.672627
Received: 15 March 2021; Accepted: 21 April 2021;
Published: 21 May 2021.
Edited by:
Massimo Bonacchi, University of Florence, ItalyReviewed by:
Rufaida Mazahir, Sir Ganga Ram Hospital, IndiaCopyright © 2021 Gu, Li, Lin, Jiang, Wang, Zhou, Su, Fan, Gao, Jin, Wang and Du. This is an open-access article distributed under the terms of the Creative Commons Attribution License (CC BY). The use, distribution or reproduction in other forums is permitted, provided the original author(s) and the copyright owner(s) are credited and that the original publication in this journal is cited, in accordance with accepted academic practice. No use, distribution or reproduction is permitted which does not comply with these terms.
*Correspondence: Jie Du, amllZHVAY2NtdS5lZHUuY24=; Yuan Wang, d2FuZ3l1YW45ODA1MTBAMTYzLmNvbQ==; Mei Jin, amlubWVpYXoyMDE2QDEyNi5jb20=
Disclaimer: All claims expressed in this article are solely those of the authors and do not necessarily represent those of their affiliated organizations, or those of the publisher, the editors and the reviewers. Any product that may be evaluated in this article or claim that may be made by its manufacturer is not guaranteed or endorsed by the publisher.
Research integrity at Frontiers
Learn more about the work of our research integrity team to safeguard the quality of each article we publish.