- 1Division of Cardiology, Department of Medicine, Columbia University Irving Medical Center, New York, NY, United States
- 2Department of Cardiovascular Medicine, Tokushima University, Tokushima, Japan
- 3Department of Medical Image Informatics, Graduate School of Biomedical Sciences, Tokushima University, Tokushima, Japan
- 4Department of Emergency Medicine, Massachusetts General Hospital, Boston, MA, United States
- 5Irving Institute for Clinical and Translational Research, Columbia University Irving Medical Center, New York, NY, United States
- 6Cardiology Division, Department of Medicine, Massachusetts General Hospital, Boston, MA, United States
Genetic testing provides valuable insights into family screening strategies, diagnosis, and prognosis in patients with hypertrophic cardiomyopathy (HCM). On the other hand, genetic testing carries socio-economical and psychological burdens. It is therefore important to identify patients with HCM who are more likely to have positive genotype. However, conventional prediction models based on clinical and echocardiographic parameters offer only modest accuracy and are subject to intra- and inter-observer variability. We therefore hypothesized that deep convolutional neural network (DCNN, a type of deep learning) analysis of echocardiographic images improves the predictive accuracy of positive genotype in patients with HCM. In each case, we obtained parasternal short- and long-axis as well as apical 2-, 3-, 4-, and 5-chamber views. We employed DCNN algorithm to predict positive genotype based on the input echocardiographic images. We performed 5-fold cross-validations. We used 2 reference models—the Mayo HCM Genotype Predictor score (Mayo score) and the Toronto HCM Genotype score (Toronto score). We compared the area under the receiver-operating-characteristic curve (AUC) between a combined model using the reference model plus DCNN-derived probability and the reference model. We calculated the p-value by performing 1,000 bootstrapping. We calculated sensitivity, specificity, positive predictive value (PPV), and negative predictive value (NPV). In addition, we examined the net reclassification improvement. We included 99 adults with HCM who underwent genetic testing. Overall, 45 patients (45%) had positive genotype. The new model combining Mayo score and DCNN-derived probability significantly outperformed Mayo score (AUC 0.86 [95% CI 0.79–0.93] vs. 0.72 [0.61–0.82]; p < 0.001). Similarly, the new model combining Toronto score and DCNN-derived probability exhibited a higher AUC compared to Toronto score alone (AUC 0.84 [0.76–0.92] vs. 0.75 [0.65–0.85]; p = 0.03). An improvement in the sensitivity, specificity, PPV, and NPV was also achieved, along with significant net reclassification improvement. In conclusion, compared to the conventional models, our new model combining the conventional and DCNN-derived models demonstrated superior accuracy to predict positive genotype in patients with HCM.
Introduction
Hypertrophic cardiomyopathy (HCM) is the most common genetic cardiac disease, affecting ~1 in 200–500 people (1). HCM is caused by mutations in the genes coding for proteins constructing the contractile apparatus of the myocardium (2). Investigators have documented dozens of genes and >1,000 gene mutations associated with HCM pathogenesis (2). Genetic testing has now become a powerful tool for family screening, diagnosis, and prognostication in HCM (3, 4). For example, genetic testing can determine whether each of the first-degree relatives is at risk of developing HCM (3, 5). Genetic testing can also help clinicians establish the diagnosis of HCM in patients with atypical clinical features (5). Furthermore, positive genotype carries a significant prognostic impact (6). On the other hand, genetic testing is time- and resource-intensive, and can introduce substantial financial (7, 8), social (e.g., insurability) (9), and psychological burdens (10). Thus, it is important to precisely determine the pre-test probability in each patient with HCM prior to performing genetic testing.
Several prediction tools have been developed to predict positive genotype in HCM—e.g., the Mayo HCM Genotype Predictor score (“the Mayo score” in this manuscript), the Toronto HCM Genotype score (“the Toronto score”) (11, 12). These scoring systems are based on a limited number of clinical parameters including echocardiographic features [e.g., left ventricular (LV) wall thickness, interventricular septal morphology] (11, 12). However, these measurements can be subjective and are prone to intra- and inter-observer variability. Further, these scoring systems only offer limited predictive accuracy (11–15).
Deep learning is a rapidly evolving approach in a variety of medical settings including cardiovascular imaging (16–20). This technology has the potential to overcome the aforementioned human limitations (21). In the HCM population, a previous study demonstrated that deep learning-derived classification model using echocardiographic images can distinguish HCM from other cardiovascular diseases (22). Nonetheless, no previous studies examined the ability of deep learning to predict positive genotype in HCM. We therefore designed the present study to investigate, in patients with HCM, whether deep convolutional neural network (DCNN, a type of deep learning) analysis of echocardiographic images improves the ability to predict positive genotype compared to the conventional models based only on clinical parameters.
Methods
Study Design and Population
We prospectively enrolled patients who were seen at the Center for Advanced Cardiac Care at Columbia University Medical Center (New York, NY, USA) and ≥18 years of age with a clinical diagnosis of HCM between 1988 and 2018. We diagnosed HCM if there was echocardiographic evidence of LV hypertrophy—i.e., max LV wall thickness ≥15 mm—out of proportion to systemic loading conditions and a non-dilated LV (3, 23, 24). We excluded patients based on the following criteria; (1) Patients who have never had genetic testing; (2) Patients with HCM phenocopies such as Fabry disease and cardiac amyloidosis confirmed with appropriate testing (3); and (3) Patients who underwent septal reduction therapy—i.e., septal myectomy, alcohol septal ablation—or heart transplant before enrollment. We collected baseline characteristics of the study sample including medical and family history, medication use, and echocardiographic parameters at the time of genetic testing. The institutional review boards of Columbia University Irving Medical Center and Tokushima University Hospital approved this study.
Outcome Measure
The primary outcome was positive genotype. By convention, variants categorized as “definitely pathogenic” or “likely pathogenic” were regarded positive in the present analysis (6, 11, 12). Variants classified as “variant of uncertain significance,” “likely benign,” or “benign” were considered negative (6, 11, 12). This definition of positive genotype was used in the present study because only these mutations are clinically actionable (i.e., allow treating physicians and the proband's family members to proceed with cascade genetic screening) and carry diagnostic and prognostic impact (3–5). All the patients were offered genetic testing for HCM using one of the commercially available testing kits (e.g., GeneDx, Invitae). Genetic testing kit was chosen based on available insurance reimbursement and patient preference. A sensitivity analysis was also performed after excluding patients with variant of uncertain significance.
The Reference Models
We used 2 reference models: (1) the Mayo score and (2) the Toronto score. To calculate the Mayo score, we assigned 1 point for the presence of the following variables: age at diagnosis ≤45 years, maximal LV wall thickness ≥20 mm on transthoracic echocardiography, reverse curve septal morphology on transthoracic echocardiography, positive family history of HCM, and positive family history of sudden cardiac death (11). We subtracted 1 point from the score if hypertension was present (11). We made the diagnosis of hypertension based on past medical history, blood pressure measurements, and antihypertensive medication use. We did not count antihypertensives solely used for non-antihypertensive purposes—e.g., β-blockers and/or non-dihydropyridine calcium channel blockers for symptomatic relief of obstructive HCM and/or rate control of atrial fibrillation. For the calculation of the Toronto score, we used the following weighted variables: age at diagnosis, sex, hypertension, family history of HCM, septal morphological subtype (i.e., reverse or neutral), and the ratio of maximal LV wall thickness to posterior wall thickness (12).
Acquisition of Echocardiographic Images
Standard echocardiographic examinations were performed using a commercially available ultrasound system (iE33, Philips Healthcare, Amsterdam, The Netherlands) as a part of routine clinical care according to the guideline recommendations (25). The 2-dimensional echocardiographic images of all subjects were obtained from the parasternal short- (SAX) and long-axis (LAX) views as well as the apical 2- (AP2), 3- (AP3), 4- (AP4), and 5-chamber (AP5) views. We selected cases with good or adequate imaging quality on the basis of the visualization of the LV walls and endocardial borders. Echocardiographic images were stored digitally as a DICOM file and analyzed offline.
Import of the Echocardiographic Images
Echocardiographic images from the SAX, LAX, AP2, AP3, AP4, and AP5 views were analyzed. All DICOM images were rigidly registered and rescaled into a reference image to adjust the size of the echocardiographic images. The images were cut and down-sampled to 18.07 × 18.07 cm with 120 × 120 monochrome pixels. Simultaneously, metadata presented in the periphery of the images were removed. To adjust for differences in frame rate and heart rate between patients, 10 equally-spaced images per 1 cardiac cycle were chosen with the use of a semi-automatic heartbeat analysis algorithm. The starting frame was defined by the R wave on the electrocardiogram as a recording of echocardiographic images are triggered by the R wave. The methodological details are provided in Supplementary Methods and have been published previously (16).
Deep Learning Algorithm
Figure 1 visualizes the processing steps of DCNN. Positive genotype was predicted by a DCNN algorithm using the 6 views (SAX, LAX, AP2, AP3, AP4, and AP5). All data were randomly divided into 5 groups and 4 of the groups were used as the training set to develop the model, and the rest was used as the test set to examine the model performance (i.e., 5-fold cross-validation; Supplementary Figure 1). To avoid an unexpected extraction of undesired features for the evaluation, training data were augmented in each dataset. The output was the probability of positive genotype. Model training was performed on a graphics processing unit (GeForce GTX 1080 Ti, NVIDIA, Santa Clara, California, USA). The Adam optimizer was used for training (Supplementary Figure 2) (26). The details are provided in Supplementary Methods. Deep learning was performed with the Python 3.6 programming language with Keras 2.1.5. Additionally, to visually display which part of the heart the DCNN-based models were focused on, gradient-weighted class activation mapping (grad-CAM) analysis was performed (27).
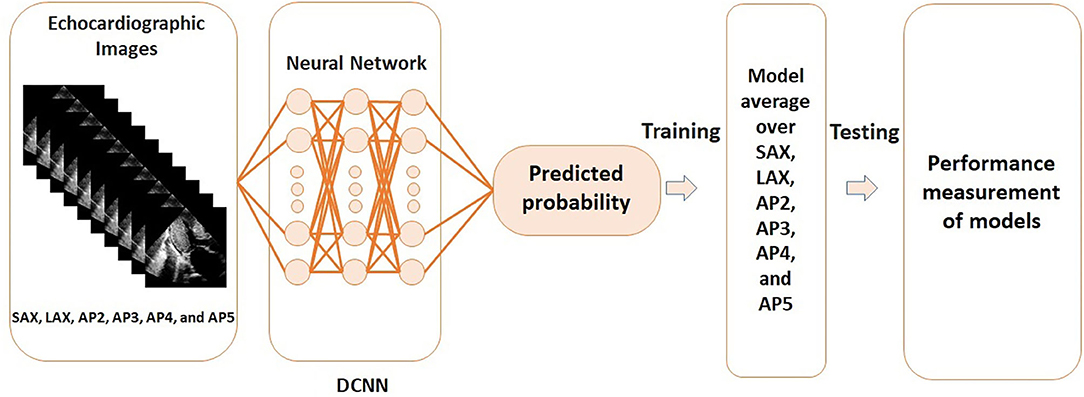
Figure 1. Steps of the deep convoluted neural network analysis using echocardiographic images. DCNN, deep convoluted neural network; AP2, apical 2-chamber view; AP3, apical 3-chamber view; AP4, apical 4-chamber view; AP5, apical 5-chamber view; LAX, parasternal long-axis view; SAX, parasternal short-axis view.
Statistical Analysis
For comparisons of the baseline characteristics between patients with positive and negative genotype, Fisher's exact test, Student's t-test, or chi-squared test was used, as appropriate. The following steps were taken to compare the area under the receiver operating characteristics curve (AUC) of one of the reference models (i.e., the Mayo score or the Toronto score) and that of a new model combining the reference model with the DCNN-derived model. First, logistic regression model was constructed to estimate the coefficient values and the constant to combine the reference model and the DCNN-derived probability. Second, the AUC of the reference model and that of the combined model were compared using non-parametric receiver operating characteristic estimation with 1,000 bootstrapping. The Stata command rocreg with auc option was used to perform this step. Additionally, the net reclassification improvement was examined using the Stata command incrisk. The sensitivity, specificity, positive predictive value (PPV), and negative predictive value (NPV) were also calculated. Statistical significance was declared if the 2-sided p-value was <0.05. These analyses were performed using Stata Statistical Software: Release 12 (StataCorp LP, College Station, TX).
Results
Initially, 105 patients with HCM who underwent genetic testing and had at least 1 echocardiographic study were screened. In this cohort, six patients were excluded based on the exclusion criteria. The most common reasons for exclusion were prior septal reduction therapy and prior heart transplant. As a result, 99 patients were included in the analysis. A total of 45 (45%) patients had positive genotype. This proportion is similar to what has been reported in the literature (6). Baseline patient characteristics are shown in Table 1. Patients with positive genotype were younger and more likely to have family history of HCM as well as reverse septal contour, and had lower systolic blood pressure.
The DCNN-predicted probability showed the AUC of 0.76 (95% CI 0.66–0.86). The AUC of the Mayo score was 0.72 (95% CI 0.61–0.82). Table 2 summarizes the net reclassification improvement, sensitivity, specificity, PPV, and NPV using the Mayo score as the reference model. The new model combining the Mayo score with the DCNN-predicted probability significantly improved the predictive accuracy compared to the Mayo score (AUC = 0.86; 95% CI 0.79–0.93; p < 0.001; Figure 2). There was also a significant net reclassification improvement (Table 2), indicating that a larger number of patients were reclassified in the right direction compared to the number of patients who were reclassified in the wrong direction. The coefficients and constant to construct the combined model are shown in Supplementary Results. The sensitivity analysis after excluding patients with variant of uncertain significance showed similar findings; the AUC of the Mayo score was 0.73, whereas that of the combined model was 0.87 (p = 0.0002).

Table 2. Predictive performance of Mayo score and a new model combining Mayo score and deep convolutional neural network-based probability to predict positive genotype in patients with hypertrophic cardiomyopathy.
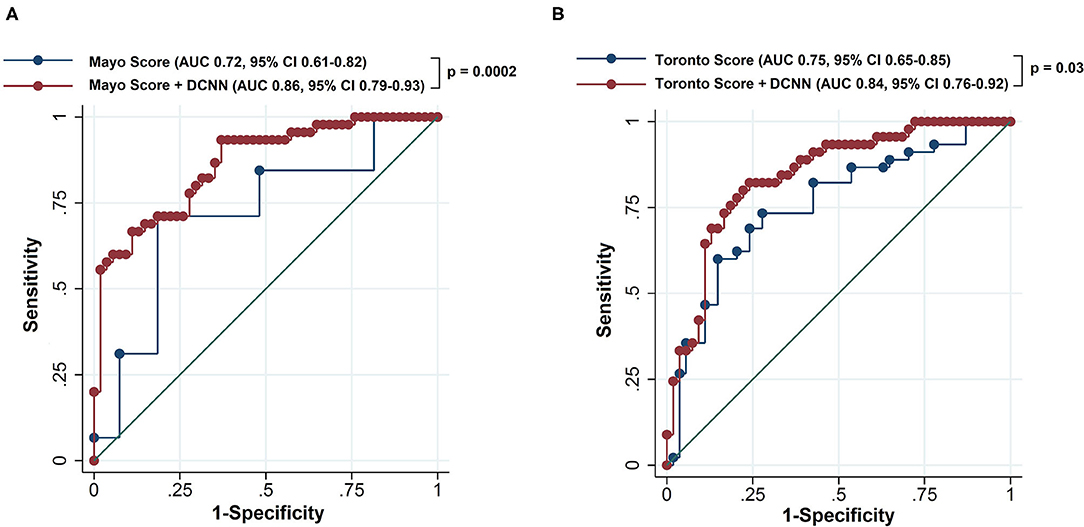
Figure 2. Receiver operating characteristics curve of the reference scoring system and new model combining the reference scoring system and deep convolutional neural network-based probability to predict positive genotype in patients with hypertrophic cardiomyopathy. The reference scoring system was the Mayo score in (A) and the Toronto score in (B). The dots represent different threshold levels. DCNN, deep convoluted neural network; AUC, area under the receiver operating characteristic curve; CI, confidence interval.
When the Toronto score was used as the reference model, the AUC was 0.75 (95% CI 0.65–0.85; Table 3). The new model combining the Toronto score with the DCNN-predicted probability exhibited significant improvement in the AUC compared to the Toronto score alone (AUC 0.84, 95% CI 0.76–0.92, p = 0.03; Figure 2). A significant net reclassification improvement was also achieved along with improvement in the sensitivity, specificity, PPV, and NPV (Table 3). After excluding patients with variant of uncertain significance, the AUC of the Toronto score was 0.74 and that of the combined model was 0.85 (p = 0.01).

Table 3. Predictive performance of Toronto score and a new model combining Toronto score and deep convolutional neural network-based probability to predict positive genotype in patients with hypertrophic cardiomyopathy.
To improve the interpretability of DCNN models, representative visualizations generated by grad-CAM are shown in Figure 3. This visualization method revealed that the DCNN-based models applied a large weight on the LV walls (e.g., the interventricular septum and posterior wall) and the left atrium.
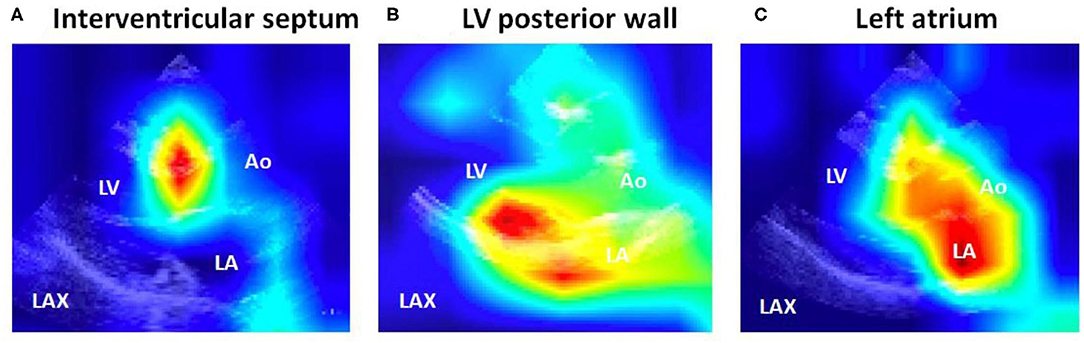
Figure 3. Spatial display of important features detected by the deep convoluted neural network analysis models. Note that the important features were localized to (A) the interventricular septum, (B) the LV posterior wall, and (C) the left atrium. Ao, aorta; LA, left atrium; LAX: parasternal long-axis view; LV, left ventricular/ventricle.
Discussion
Summary of Findings
In this study that examined the incremental value of deep learning-based models to predict positive genotype, the predictive ability of our novel models combining the conventional model and the deep learning-based probability significantly outperformed that of the conventional models. The present study serves as the first investigation demonstrating the additional value of deep learning-based analysis of echocardiographic images in predicting positive genotype in patients with HCM.
Impact of Positive Genotype on Family Screening, Diagnosis, and Prognostication
Genetic testing is useful in determining family screening strategies in HCM. Without genetic testing, first-degree relatives have to undergo phenotypic screening with electrocardiogram and echocardiography every 5 years, and more frequently if the age is <18 years (3, 5). This burden can be relieved if the proband has positive genotype and the family member does not carry the identified gene mutation (3, 5). Furthermore, genetic testing has both diagnostic and prognostic values. In patients with suspected HCM, positive genotype confirms the diagnosis of HCM (2). With regard to prognostication, patients with positive genotype had a 2-fold higher risk of adverse outcomes (e.g., heart failure, atrial fibrillation) compared to those with negative phenotype in a prospective cohort study of patients with HCM (6). Thus, positive genotype can have a substantial impact on the clinical management of patients with HCM and their family members.
On the other hand, genetic testing can carry substantial financial and social burdens. For example, genetic testing costs a few thousand dollars in the US, and the proportion of the patient's out-of-pocket payment depends on the insurance type and plan. With regard to the social burden of genetic testing, while the Genetic Information Non-discrimination Act prohibits discrimination of insurability based on genetic testing results, the law is silent regarding life, disability, and long-term care insurance (9, 28, 29). As such, genetic testing can result in non-negligible burdens, and accurate identification of patients with HCM who have high pre-test probability carries clinical, socio-economical, and psychological importance.
Nevertheless, the currently available conventional models—i.e., the Mayo score, the Toronto score—offer only modest ability to predict positive genotype. The AUCs of these scoring systems have been reported to be ~0.75 (11–15), which is in agreement with those in the present study (0.72 with the Mayo score and 0.75 with the Toronto score). In this context, findings in the current analysis add to the body of knowledge by demonstrating that the deep learning-based analysis of echocardiographic images provides incremental value to the conventional models in predicting positive genotype in patients with HCM.
Advantages of Deep Learning-Based Approach Over the Conventional Prediction Methods
The Mayo and Toronto scoring systems include a limited number of parameters determined by echocardiography—e.g., maximal LV wall thickness, septal morphological subtypes. However, these parameters have been known to have large intra- and inter-observer variability (11, 30). It is a time- and cost-intensive process to train physicians until they can accurately measure the wall thickness and classify the septal morphology (31). Even after going through such specialized trainings, the interpretation of echocardiographic images still remains interpreter-dependent and subjective, and can be affected by fatigue (31). Moreover, these parameters used in the conventional models do not account for dynamic (i.e., non-static) image information.
By contrast, deep learning has a potential to overcome such variability in human assessment of echocardiographic measurements (32). Deep learning is also able to extract information that is not readily apparent to humans (33). Thus, deep learning-based models can offer a new avenue to generate an accurate, consistent, rapid, and automated interpretation of echocardiographic images while reducing the risk of human errors. Its application has shown a high potential to revolutionize the process of diagnosis and prognostication, with promising results in the fields of dermatology (34), radiology (35), and cardiology (16, 36). In the HCM population, a prior study reported that a deep learning-derived classification model using echocardiographic images can differentiate HCM from cardiac amyloidosis and pulmonary arterial hypertension (22). Furthermore, our DCNN approach utilizes not only spatial but also temporal information by incorporating the additional dimension of time.
Despite the potential usefulness, no prior studies have applied deep learning-based methods to predict positive genotype in HCM. The present analysis represents the first study to exhibit the incremental value of deep learning-based analysis of echocardiographic images in addition to the conventional clinical parameters to predict positive genotype in the HCM population. The ability of our deep learning-based approach to analyze echocardiographic images obtained in routine clinical care—as opposed to “research-quality” images gained for investigational purposes—further underscores the feasibility and generalizability of this novel method.
Spatial Visualization of Important Features to Identify Genotype-Positive Patients
Deep learning technology is frequently referred to as a black box—i.e., it does not provide information as to which features are mainly used for the development of discrimination models. Our deep learning method is not an exception. To address this issue, in the present study, we have performed the grad-CAM analysis and provided visualization of the important features that the deep learning models focused on, which greatly enhances the interpretability (27). This analysis demonstrated an interesting finding—in addition to the LV, features spatially located in the left atrium were frequently used to distinguish between patients with positive and negative genotype in HCM. This observation is consistent with our prior knowledge; the left atrial diameter has been known to predict sudden cardiac death (37) and cardiovascular death in the HCM population (38). The inferences from our study suggest that echocardiographic parameters related to the left atrium—e.g., left atrial diameter, volume, and ejection fraction—have a potential to predict positive genotype in the HCM population.
Potential Limitations
Findings in the present study should be interpreted with several limitations in mind. First, the present study is subject to selection bias. The study sample was limited to patients with HCM who underwent genetic testing. Second, positive genotype was defined by the currently available classification of mutations; however, the classification of each mutation can change in the future. Third, validation with external samples was not performed. This study should prompt model validation with a new cohort. Last, the study samples were relatively homogeneous in terms of race and sex. Further, there is a possibility that the spectrum of mutations observed in the study samples may not exactly represent those in the general HCM population. Therefore, generalizability of the results to other HCM populations (e.g., those who are not followed at HCM referral centers) needs to be established.
Conclusions
Compared to the conventional models based on clinical and echocardiographic parameters, our new models integrating the conventional and deep learning-based analysis of echocardiographic images demonstrated a superior ability to predict positive genotype in patients with HCM. For patients and treating physicians, the novel deep learning-based method introduced in the present study can be used as an assistive technology to inform the decision-making process of performing genetic testing; deep learning coupled to human expertise can provide more accurate pre-test probability. For researchers, the current analysis would prompt further investigation into developing a better deep learning model to predict positive genotype in patients with HCM.
Data Availability Statement
The original contributions presented in the study are included in the article/Supplementary Materials. Further inquiries can be directed to the corresponding author.
Ethics Statement
The studies involving human participants were reviewed and approved by the institutional review boards of Columbia University Irving Medical Center and Tokushima University Hospital. The patients/participants provided their written informed consent to participate in this study.
Author Contributions
SM wrote the manuscript. KK and YS conceived the idea for the manuscript. KK and AH performed the deep learning analyses of echocardiographic images. YS was guarantor and performed the statistical analyses. SM and YS contributed to data acquisition. MS, KH, YR, MR, MF, MM, and YS contributed to interpreting the data and revising the work critically for intellectual content. All authors made the decision to submit.
Funding
YS was supported by research grants from the National Institute of Health (Bethesda, MD; R01 HL157216), American Heart Association (Dallas, TX) National Clinical and Population Research Awards and Career Development Award, The Feldstein Medical Foundation (Clifton, NJ) Medical Research Grant, Korea Institute of Oriental Medicine (Daejeon, Republic of Korea), and Columbia University Irving Medical Center Irving Institute for Clinical & Translational Research (New York, NY) Precision Medicine Pilot Award. MM was supported by the National Institute of Health (K24 AG036778). MR was supported by the National Institute of Health (UL1 TR001873 and K24 HL107643). The funding organizations did not have any role in the study design, collection, analysis, or interpretation of data, in writing of the manuscript, or in the decision to submit the article for publication. The researchers were independent from the funding organizations.
Conflict of Interest
The authors declare that the research was conducted in the absence of any commercial or financial relationships that could be construed as a potential conflict of interest.
Publisher's Note
All claims expressed in this article are solely those of the authors and do not necessarily represent those of their affiliated organizations, or those of the publisher, the editors and the reviewers. Any product that may be evaluated in this article, or claim that may be made by its manufacturer, is not guaranteed or endorsed by the publisher.
Supplementary Material
The Supplementary Material for this article can be found online at: https://www.frontiersin.org/articles/10.3389/fcvm.2021.669860/full#supplementary-material
References
1. Maron BJ. Clinical course and management of hypertrophic cardiomyopathy. N Engl J Med. (2018) 379:655–68. doi: 10.1056/NEJMra1710575
2. Seidman CE, Seidman JG. Identifying sarcomere gene mutations in hypertrophic cardiomyopathy: a personal history. Circ Res. (2011) 108:743–50. doi: 10.1161/CIRCRESAHA.110.223834
3. Ommen SR, Mital S, Burke MA, Day SM, Deswal A, Elliott P, et al. 2020 AHA/ACC Guideline for the diagnosis and treatment of patients with hypertrophic cardiomyopathy. Circulation. (2020) 142:e558–631. doi: 10.1161/CIR.0000000000000937
4. Elliott PM, Anastasakis A, Borger MA, Borggrefe M, Cecchi F, Charron P, et al. 2014 ESC Guidelines on diagnosis and management of hypertrophic cardiomyopathy. Eur Heart J. (2014) 35:2733–79. doi: 10.1093/eurheartj/ehu284
5. Veselka J, Anavekar NS, Charron P. Hypertrophic obstructive cardiomyopathy. Lancet. (2017) 389:1253–67. doi: 10.1016/S0140-6736(16)31321-6
6. Ho CY, Day SM, Ashley EA, Michels M, Pereira AC, Jacoby D, et al. Genotype and lifetime burden of disease in hypertrophic cardiomyopathy: insights from the Sarcomeric Human Cardiomyopathy Registry (SHaRe). Circulation. (2018) 138:1387–98.
7. Andersen PS, Havndrup O, Hougs L, Sorensen KM, Jensen M, Larsen LA, et al. Diagnostic yield, interpretation, and clinical utility of mutation screening of sarcomere encoding genes in Danish hypertrophic cardiomyopathy patients and relatives. Hum Mutat. (2009) 30:363–70. doi: 10.1002/humu.20862
8. Hoss S, Habib M, Silver J, Care M, Chan RH, Hanneman K, et al. Genetic testing for diagnosis of hypertrophic cardiomyopathy mimics: yield and clinical significance. Circ Genom Precis Med. (2020) 13:e002748. doi: 10.1161/CIRCGEN.119.002748
9. Mohammed S, Lim Z, Dean PH, Potts JE, Tang JN, Etheridge SP, et al. Genetic insurance discrimination in sudden arrhythmia death syndromes: Empirical evidence from a cross-sectional survey in North America. Circ Cardiovasc Genet. (2017) 10:e001442. doi: 10.1161/CIRCGENETICS.116.001442
10. Wynn J, Holland DT, Duong J, Ahimaz P, Chung WK. Examining the psychosocial impact of genetic testing for cardiomyopathies. J Genet Couns. (2018) 27:927–34. doi: 10.1007/s10897-017-0186-4
11. Bos JM, Will ML, Gersh BJ, Kruisselbrink TM, Ommen SR, Ackerman MJ. Characterization of a phenotype-based genetic test prediction score for unrelated patients with hypertrophic cardiomyopathy. Mayo Clin Proc. (2014) 89:727–37. doi: 10.1016/j.mayocp.2014.01.025
12. Gruner C, Ivanov J, Care M, Williams L, Moravsky G, Yang H, et al. Toronto hypertrophic cardiomyopathy genotype score for prediction of a positive genotype in hypertrophic cardiomyopathy. Circ Cardiovasc Genet. (2013) 6:19–26. doi: 10.1161/CIRCGENETICS.112.963363
13. Bonaventura J, Norambuena P, Tomasov P, Jindrova D, Sediva H, Macek M, et al. The utility of the Mayo Score for predicting the yield of genetic testing in patients with hypertrophic cardiomyopathy. Arch Med Sci. (2019) 15:641–9. doi: 10.5114/aoms.2018.78767
14. Murphy SL, Anderson JH, Kapplinger JD, Kruisselbrink TM, Gersh BJ, Ommen SR, et al. Evaluation of the Mayo Clinic Phenotype-Based Genotype Predictor Score in patients with clinically diagnosed hypertrophic cardiomyopathy. J Cardiovasc Transl Res. (2016) 9:153–61. doi: 10.1007/s12265-016-9681-5
15. Newman R, Jefferies JL, Chin C, He H, Shikany A, Miller EM, et al. Hypertrophic cardiomyopathy genotype prediction models in a pediatric population. Pediatr Cardiol. (2018) 39:709–17. doi: 10.1007/s00246-018-1810-2
16. Kusunose K, Haga A, Inoue M, Fukuda D, Yamada H, Sata M. Clinically feasible and accurate view classification of echocardiographic images using deep learning. Biomolecules. (2020) 10. doi: 10.3390/biom10050665
17. Nagendran M, Chen Y, Lovejoy CA, Gordon AC, Komorowski M, Harvey H, et al. Artificial intelligence versus clinicians: systematic review of design, reporting standards, and claims of deep learning studies. BMJ. (2020) 368:m689. doi: 10.1136/bmj.m689
18. Xu B, Kocyigit D, Grimm R, Griffin BP, Cheng F. Applications of artificial intelligence in multimodality cardiovascular imaging: a state-of-the-art review. Prog Cardiovasc Dis. (2020) 63:367–76. doi: 10.1016/j.pcad.2020.03.003
19. Kusunose K, Haga A, Abe T, Sata M. Utilization of artificial intelligence in echocardiography. Circ J. (2019) 83:1623–29. doi: 10.1253/circj.CJ-19-0420
20. Kusunose K, Abe T, Haga A, Fukuda D, Yamada H, Harada M, et al. A deep learning approach for assessment of regional wall motion abnormality from echocardiographic images. J Am Coll Cardiol Img. (2020) 13:374–81. doi: 10.1016/j.jcmg.2019.02.024
21. Davis A, Billick K, Horton K, Jankowski M, Knoll P, Marshall JE, et al. Artificial intelligence and echocardiography: a primer for cardiac sonographers. J Am Soc Echocardiogr. J Am Soc Echocardiogr. (2020) 33:1061–6. doi: 10.1016/j.echo.2020.04.025
22. Zhang J, Gajjala S, Agrawal P, Tison GH, Hallock LA, Beussink-Nelson L, et al. Fully automated echocardiogram interpretation in clinical practice. Circulation. (2018) 138:1623–35. doi: 10.1161/CIRCULATIONAHA.118.034338
23. Shimada YJ, Hasegawa K, Kochav SM, Mohajer P, Jung J, Maurer MS, et al. Application of proteomics profiling for biomarker discovery in hypertrophic cardiomyopathy. J Cardiovasc Transl Res. (2019) 12:569–79. doi: 10.1007/s12265-019-09896-z
24. Shimada YJ, Hoeger CW, Latif F, Takayama H, Ginns J, Maurer MS. Myocardial contraction fraction predicts cardiovascular events in patients with hypertrophic cardiomyopathy and normal ejection fraction. J Card Fail. (2019) 25:450–6. doi: 10.1016/j.cardfail.2019.03.016
25. Mitchell C, Rahko PS, Blauwet LA, Canaday B, Finstuen JA, Foster MC, et al. Guidelines for performing a comprehensive transthoracic echocardiographic examination in adults: recommendations from the American Society of Echocardiography. J Am Soc Echocardiogr. (2019) 32:1–64. doi: 10.1016/j.echo.2018.06.004
26. Kusunose K, Haga A, Yamaguchi N, Abe T, Fukuda D, Yamada H, et al. Deep learning for assessment of left ventricular ejection fraction from echocardiographic images. J Am Soc Echocardiogr. (2020) 33:632–5el. doi: 10.1016/j.echo.2020.01.009
27. Selvaraju RR, Cogswell M, Das A, Vedantam R, Parikh D, Batra D. Grad-CAM: visual explanations from deep networks via gradient-based localization. IEEE I Conf Comp Vis. (2017) 618–26. doi: 10.1109/ICCV.2017.74
28. Christiaans I, Kok TM, van Langen IM, Birnie E, Bonsel GJ, Wilde AA, et al. Obtaining insurance after DNA diagnostics: a survey among hypertrophic cardiomyopathy mutation carriers. Eur J Hum Genet. (2010) 18:251–3. doi: 10.1038/ejhg.2009.145
29. Prince AER. Political economy, stakeholder voices, and saliency: lessons from international policies regulating insurer use of genetic information. J Law Biosci. (2018) 5:461–94. doi: 10.1093/jlb/lsz001
30. Urbano-Moral JA, Gonzalez-Gonzalez AM, Maldonado G, Gutierrez-Garcia-Moreno L, Vivancos-Delgado R, De Mora-Martin M, et al. Contrast-enhanced echocardiographic measurement of left ventricular wall thickness in hypertrophic cardiomyopathy: comparison with standard echocardiography and cardiac magnetic resonance. J Am Soc Echocardiogr. (2020) 33:1106–15. doi: 10.1016/j.echo.2020.04.009
31. Krittanawong C, Johnson KW, Rosenson RS, Wang Z, Aydar M, Baber U, et al. Deep learning for cardiovascular medicine: a practical primer. Eur Heart J. (2019) 40:2058–73. doi: 10.1093/eurheartj/ehz056
32. Ouyang D, He B, Ghorbani A, Yuan N, Ebinger J, Langlotz CP, et al. Video-based AI for beat-to-beat assessment of cardiac function. Nature. (2020) 580:252–6. doi: 10.1038/s41586-020-2145-8
33. Kusunose K. Radiomics in echocardiography: deep learning and echocardiographic analysis. Curr Cardiol Rep. (2020) 22:89. doi: 10.1007/s11886-020-01348-4
34. Haenssle HA, Fink C, Schneiderbauer R, Toberer F, Buhl T, Blum A, et al. Man against machine: diagnostic performance of a deep learning convolutional neural network for dermoscopic melanoma recognition in comparison to 58 dermatologists. Ann Oncol. (2018) 29:1836–42. doi: 10.1093/annonc/mdy166
35. Ueda D, Shimazaki A, Miki Y. Technical and clinical overview of deep learning in radiology. Jpn J Radiol. (2019) 37:15–33. doi: 10.1007/s11604-018-0795-3
36. Litjens G, Ciompi F, Wolterink JM, de Vos BD, Leiner T, Teuwen J, et al. State-of-the-art deep learning in cardiovascular image analysis. J Am Coll Cardiol Img. (2019) 12:1549–65. doi: 10.1016/j.jcmg.2019.06.009
37. O'Mahony C, Jichi F, Pavlou M, Monserrat L, Anastasakis A, Rapezzi C, et al. A novel clinical risk prediction model for sudden cardiac death in hypertrophic cardiomyopathy (HCM risk-SCD). Eur Heart J. (2014) 35:2010–20. doi: 10.1093/eurheartj/eht439
Keywords: hypertrophic cardiomyopathy, echocardiography, deep learning, genotype, prediction
Citation: Morita SX, Kusunose K, Haga A, Sata M, Hasegawa K, Raita Y, Reilly MP, Fifer MA, Maurer MS and Shimada YJ (2021) Deep Learning Analysis of Echocardiographic Images to Predict Positive Genotype in Patients With Hypertrophic Cardiomyopathy. Front. Cardiovasc. Med. 8:669860. doi: 10.3389/fcvm.2021.669860
Received: 19 February 2021; Accepted: 09 August 2021;
Published: 27 August 2021.
Edited by:
Zahra K. Motamed, McMaster University, CanadaReviewed by:
Emmanuel Androulakis, Royal Brompton and Harefield NHS Foundation Trust, United KingdomMax Olender, Massachusetts Institute of Technology, United States
Copyright © 2021 Morita, Kusunose, Haga, Sata, Hasegawa, Raita, Reilly, Fifer, Maurer and Shimada. This is an open-access article distributed under the terms of the Creative Commons Attribution License (CC BY). The use, distribution or reproduction in other forums is permitted, provided the original author(s) and the copyright owner(s) are credited and that the original publication in this journal is cited, in accordance with accepted academic practice. No use, distribution or reproduction is permitted which does not comply with these terms.
*Correspondence: Yuichi J. Shimada, ys3053@cumc.columbia.edu
†These authors have contributed equally to this work and share first authorship