- 1Laboratory of Experimental Cardiology, University Medical Center Utrecht, University of Utrecht, Utrecht, Netherlands
- 2Central Diagnostics Laboratory, University Medical Center Utrecht, University of Utrecht, Utrecht, Netherlands
- 3Quorics B.V., Rotterdam, Netherlands
- 4TNO-Metabolic Health Research, Gaubius Laboratory, Leiden, Netherlands
- 5Translational Metabolic Laboratory, Radboudumc, Nijmegen, Netherlands
- 6TNO- Microbiology & Systems Biology, Zeist, Netherlands
- 7Department of Vascular Surgery, University Medical Center Utrecht, University of Utrecht, Utrecht, Netherlands
- 8Department of Cardiology, Division Heart & Lungs, University Medical Center Utrecht, Utrecht University, Utrecht, Netherlands
- 9Faculty of Population Health Sciences, Institute of Cardiovascular Science, University College London, London, United Kingdom
- 10Health Data Research UK and Institute of Health Informatics, University College London, London, United Kingdom
- 11SkylineDx B.V., Rotterdam, Netherlands
Background and Aims: Oncostatin M (OSM) signaling is implicated in atherosclerosis, however the mechanism remains unclear. We investigated the impact of common genetic variants in OSM and its receptors, OSMR and LIFR, on overall plaque vulnerability, plaque phenotype, intraplaque OSMR and LIFR expression, coronary artery calcification burden and cardiovascular disease susceptibility.
Methods and Results: We queried Genotype-Tissue Expression data and found that rs13168867 (C allele) was associated with decreased OSMR expression and that rs10491509 (A allele) was associated with increased LIFR expression in arterial tissues. No variant was significantly associated with OSM expression.
We associated these two variants with plaque characteristics from 1,443 genotyped carotid endarterectomy patients in the Athero-Express Biobank Study. After correction for multiple testing, rs13168867 was significantly associated with an increased overall plaque vulnerability (β = 0.118 ± s.e. = 0.040, p = 3.00 × 10−3, C allele). Looking at individual plaque characteristics, rs13168867 showed strongest associations with intraplaque fat (β = 0.248 ± s.e. = 0.088, p = 4.66 × 10−3, C allele) and collagen content (β = −0.259 ± s.e. = 0.095, p = 6.22 × 10−3, C allele), but these associations were not significant after correction for multiple testing. rs13168867 was not associated with intraplaque OSMR expression. Neither was intraplaque OSMR expression associated with plaque vulnerability and no known OSMR eQTLs were associated with coronary artery calcification burden, or cardiovascular disease susceptibility. No associations were found for rs10491509 in the LIFR locus.
Conclusions: Our study suggests that rs1316887 in the OSMR locus is associated with increased plaque vulnerability, but not with coronary calcification or cardiovascular disease risk. It remains unclear through which precise biological mechanisms OSM signaling exerts its effects on plaque morphology. However, the OSM-OSMR/LIFR pathway is unlikely to be causally involved in lifetime cardiovascular disease susceptibility.
Introduction
The prevalence of cardiovascular disease (CVD) is high, poses a significant global burden and is expected to rise (1). Arterial inflammation, leading to asymmetric focal arterial thickening and atherosclerotic plaque formation and progression, is the primary mechanism underlying CVD (2). Inflammatory cytokines contribute to arterial inflammation and subsequent atherosclerotic plaque formation (3). One cytokine, for which there is mounting evidence suggesting a role in atherosclerosis development is OSM (4, 5). It has been shown that OSM is present in both murine and human atherosclerotic plaques (6). Moreover, murine studies showed that OSM receptor (OSMR)−/−ApoE−/− mice have reduced plaque size and improved plaque stability compared to their OSMR-expressing littermates (7), indicating that OSM drives atherosclerosis development. These observations are in line with our previous work, in which we showed that simultaneous signaling of OSM through OSMR and leukemia inhibitory factor receptor (LIFR), induces activation in human endothelial cells, suggestive of a role in atherosclerosis development (8). In contrast, chronic OSM administration to APOE*3Leiden.CETP mice reduces the atherosclerotic lesion size and severity, and high circulating OSM levels correlate with increased post-incident coronary heart disease survival probability in humans (9).
Although all these studies implicate that OSM is involved in atherosclerosis, little is known about the effects of OSM on plaque composition in humans. Grouped in the interleukin 6 subfamily of cytokines, OSM is released by activated immune cells (10–12), and exerts pleiotropic effects on cell proliferation, inflammation, hematopoiesis, tissue remodeling, and development (13). Its signals are transduced through binding to either OSMR or LIFR, which form a heterodimer with glycoprotein 130 (8, 14), that in turn activates multiple pathways (14). It is suggested that the ratio of the two receptor types expressed on the cell membrane is a potential regulatory mechanism for the multiple, and sometimes opposing, effects that are exerted by OSM (15).
Thus, given its pleiotropic function, it is difficult to predict how OSM contributes to atherosclerotic plaque formation. Cell and murine studies have shown that OSM promotes angiogenesis (4), endothelial activation (8), vessel permeability (16), and osteoblastic differentiation (17). Therefore, increased OSM levels hypothetically results in a higher intraplaque microvessel density, intraplaque hemorrhages and plaque calcification, thereby contributing to plaque destabilization (18, 19). In other cell and murine studies, OSM promotes fibroblast proliferation (20), collagen formation (20), smooth muscle cell proliferation (6), and M2 macrophage polarization (21). These processes hypothetically lead to enhanced fibrosis, and attenuated inflammation, thereby contributing to plaque stabilization (22).
Large-scale studies have shown that cis-acting genetic variants associated to gene expression [expression quantitative trait loci (eQTLs) (23)] are key to disease susceptibility (24). This means that gene expression in a given tissue differs between individuals carrying different genotypes which ultimately results in differential disease susceptibility. Thus, on the premise that alleles are randomly distributed at conception and are invariant throughout a lifetime, meaning that genetics is not influenced by disease or risk factors, eQTLs can be used as proxies of gene expression to examine the effect on plaque morphology (25). We hypothesized that if circulating OSM, or arterial OSMR or LIFR expression has an effect on plaque morphology, these phenotypic differences will be observed among genotype groups of the eQTL. We aimed to investigate the double-edged sword of OSM signaling on the composition of human atherosclerotic plaques using known eQTLs of circulating OSM, and arterial OSMR and LIFR.
Materials and Methods
Sample Collection
The Athero-Express Biobank Study (https://www.atheroexpress.nl) is an ongoing prospective study, containing biomaterial of patients elected for endarterectomy at two Dutch tertiary referral centers. Details of the study design were described before (26). Briefly, blood subfractions are obtained before and arterial plaque material during endarterectomy. Each plaque is dissected into segments of 0.5 cm. The culprit lesion is reserved for histological assessment (see below), while surrounding segments are immediately snap frozen in liquid nitrogen and stored at −80°C for later use, e.g., in order to perform RNA-seq (see below). Only carotid endarterectomy (CEA) patients were included in the present study. All research was conducted according to the principles of the Declaration of Helsinki and its later amendments, all patients provided informed consent and the study was approved by the medical ethics committees.
Athero-Express Genotyping, Quality Control, and Imputation
Details of genotyping were previously described (26). Briefly, DNA was extracted from EDTA blood or (when no blood was available) plaque samples of 1,858 consecutive patients from the Athero-Express Biobank Study and genotyped in 2 batches. For the Athero-Express Genomics Study 1 (AEGS1), 836 patients, included between 2002 and 2007, were genotyped using the Affymetrix Genome-Wide Human SNP Array 5.0 (SNP5) chip (Affymetrix Inc., Santa Clara, CA, USA). For the Athero-Express Genomics Study 2 (AEGS2), 1,022 patients, included between 2002 and 2013 and not overlapping AEGS1, were genotyped using the Affymetrix Axiom® GW CEU 1 Array (AxM).
Both studies were carried out according to OECD standards. After genotype calling, we adhered to community standard quality control and assurance (QA/QA) procedures of the genotype data from AEGS1 and AEGS2. Samples with low average genotype calling and sex discrepancies (compared to the clinical data available) were excluded. The data was further filtered on (1) individual (sample) call rate >97%, (2) SNP call rate >97%, (3) minor allele frequencies (MAF) >3%, (4) average heterozygosity rate ± 3.0 s.d., (5) relatedness (pi-hat >0.20), (6) Hardy–Weinberg Equilibrium (HWE p < 1.0 × 10−6), and (7) population stratification (based on HapMap 2, release 22, b36) by excluding samples deviating more than 6 standard deviations from the average in five iterations during principal component analysis (PCA) and by visual inspection as previously described (26). After QA/QA, 657 samples and 403,789 SNPs in AEGS1, and 869 samples and 535,983 SNPs in AEGS2 remained. To correct for genetic ancestry and population stratification we performed PCA in each cleaned dataset to obtain principal components for downstream analyses as described before (26).
We used SHAPEIT2 (27) for phasing and finally the data was imputed with 1000G phase 3 (28) and GoNL 5 (29) as a reference on genome build 37. Note that we only selected the CEA patients in these datasets, leaving 1,443 samples for our further analyses.
Variant Selection
We queried data from the Genotype-Tissue Expression (GTEx) Portal (https://gtexportal.org) (23) for cis-acting variants [defined as variants within 1Mb of a given gene (30)] that alter OSM expression in whole blood, and OSMR or LIFR expression in non-diseased arterial tissue. We selected common variants with a MAF > 3%, which yielded two variants in total: rs13168867 for OSMR in tibial arterial tissue and rs10491509 for LIFR in aortic arterial tissue. We found no eQTL for circulating OSM expression, i.e., in whole blood. We harmonized the effect alleles and effect sizes from these eQTLs to match the allele orientation in the Athero-Express Biobank Study data.
Plaque Phenotyping
The (immuno)histochemical analysis of plaques have been described previously (26, 31, 32). Briefly, per plaque, the culprit lesion was identified directly after dissection, fixed in 4% formaldehyde, embedded in paraffin and cut in 5 μm sections on a microtome for (immuno)histochemical analysis by pathology experts. Calcification (hematoxylin & eosin, H&E) and collagen content (picrosirius red) were semi-quantitatively scored and defined as no/minor or moderate/heavy. Atheroma size (H&E and picrosirius red) was defined as <10% or ≥10% fat content. Macrophages (CD68) and smooth muscle cells (ACTA2) were quantitatively scored and classified as percentage of plaque area. Intraplaque hemorrhage (H&E) was defined as absent or present, and vessel density was classified as the number of intraplaque vessels (CD34) per 3–4 hotspots.
Plaque Vulnerability
Assessment of overall plaque vulnerability was performed as previously described by Verhoeven et al. (25). Briefly, macrophages and smooth muscle cells were semi-quantitatively defined as no/minor or moderate/heavy. Each plaque characteristic that defines a stable plaque (i.e., no/minor macrophages, moderate/heavy collagen, moderate/heavy smooth muscle cells and <10% fat) was given a score of 0, while each plaque characteristic that defines a vulnerable plaque (i.e., moderate/heavy macrophages, no/minor collagen, no/minor smooth muscle cells and ≥10% fat) was given a score of 1. The score of each plaque characteristic was summed resulting in a final plaque score ranging from 0 (most stable plaque) to 4 (most vulnerable plaque). Intraobserver and interobserver variability were examined previously and showed good concordance (κ = 0.6–0.9) (33).
Plaque Expression
Detailed information on the RNA sequencing (RNAseq) experiment is described in the Supplemental Material. In short, to assess the global expression profile, plaque segments were thawed, cut up, and homogenized using ceramic beads and tissue homogenizer (Precellys, Bertin instruments, Montigny-le-Bretonneux, France), in the presence of TriPure (Sigma Aldrich), and RNA was isolated according to TriPure manufacturer's protocol.
Library Preparation
was performed, adapting the CEL-Seq2 protocol for library preparation (34, 35), as described before (36). The primer used for initial reverse-transcription reaction was designed as follows: an anchored polyT, a unique 6bp barcode, a unique molecular identifier (UMI) of 6bp, the 5' Illumina adapter and a T7 promoter, as described (36). Complementary DNA (cDNA) was then used in the in vitro transcription (IVT) reaction (AM1334; Thermo-Fisher). Amplified RNA (aRNA) was fragmented, and cleaned, and RNA yield and quality in the suspension were checked by Bioanalyzer (Agilent).
cDNA library construction was initiated according to the manufacturer's protocol, adding randomhexRT primer as random primer. PCR amplification was done with Phusion High-Fidelity PCR Master Mix with HF buffer (NEB, MA, USA) and a unique indexed RNA PCR primer (Illumina) per reaction. Library cDNA yield and quality were checked by Qubit fluorometric quantification (Thermo-Fisher) and Bioanalyzer (Agilent), respectively. Libraries were sequenced on the Illumina Nextseq500 platform; paired end, 2 × 75 bp.
Upon sequencing, retrieved fastq files were de-barcoded, split into forward and reverse reads. Subsequently, these were mapped making use of Burrows-Wheel aligner [BWA (37)] version 0.7.17-r1188 and a cDNA reference (assembly hg19, Ensembl release 84). Read counts and UMI counts were derived from SAM files using custom perl code, and then gathered into count matrices. Genes were annotated with Ensembl ID's, and basic quality control was performed, encompassing filtering out samples with low gene numbers (<10,000 genes), and read numbers (<18,000 reads). These steps resulted in 641 samples with up to 60,674 genes (Ensembl ID's), and median of 178,626 reads per sample.
Data Analysis
Plaque vulnerability scores, and genotypes for rs10491509 and rs13168867, were added to metadata, upon which this was combined with counts and annotation in a SummarizedExperiment object (38). Counts were normalized and transformed making use of the variance stabilization transformation function (vst()) in DESeq2 (39). This results in transformed data on a log2-scale, normalized for library size, for visualization and ordination purposes. Differential expression analysis between plaque vulnerability scores or genotypes, used as “condition variables” was performed using the DESeq2-function DESeq() on the raw counts. In short, three steps are performed: 1. estimation of size factors, controlling for sequencing depth; 2. estimation of dispersion values, that capture variation around expected values. These expected values take into account sequencing depth and differences caused by variables in the design formula argument, i.e., “design = ~ condition” where condition is a variable that specifies which group samples belong to; and 3. fitting a generalized linear model using the above-mentioned size factors and dispersion values, estimating log fold changes. This results in a results table, showing estimated log2 fold changes and p-values comparing between two levels of the condition variable. Complete details for statistical procedures used by the DESeq function are described elsewhere (39).
Genetic Analyses
Quantitatively scored characteristics (macrophages, smooth muscle cells, and the vessel density) were Box-Cox transformed (40) to obtain a normal distribution. For genetic analyses we used GWASToolKit (https://swvanderlaan.github.io/GWASToolKit/) which is a wrap-around collection of scripts for SNPTEST (41). Continuous and categorical variables were tested using linear and logistic regression models, respectively. Models for genetic analyses were corrected for age, sex, genotyping chip, and genetic ancestry using principal components 1 through 4. Thus, the models were of the form
Multiple Testing and Power
Correction for multiple testing resulted in a corrected p-value of p = 0.05/[(7 plaque phenotypes + plaque vulnerability) × 2 common variants] = 3.13 × 10−3. The power of the study was estimated at ±75% based on a sample size of 1,443, a minor allele frequency (MAF) of 0.409 and a relative risk of 1.28 (http://csg.sph.umich.edu/abecasis/cats/gas_power_calculator/).
Data and Scripts
Data is available upon request. Scripts are posted at GitHub https://github.com/swvanderlaan/2019_vankeulen_d_osmr.
Results
Common Variants Altering OSM, OSMR, and LIFR Expression
We included and genotyped 1,443 carotid endarterectomy patients in this study. We combined these groups (Table 1) for overall plaque vulnerability and phenotype analyses, as we previously showed that the baseline characteristics between the two genotyping groups (AEGS1 and AEGS2) are comparable (26).
OSM is secreted by, among others, neutrophils (12), monocytes (11), macrophages (11) and T-cells (10), and acts through binding to OSMR and LIFR (14, 42, 43) in the arterial wall (7, 44). Thus, we queried data from the Genotype-Tissue Expression project (GTEx) (23) for SNPs that alter OSM expression in whole blood and LIFR and OSMR expression in arterial tissue. There were no significant eQTLs for OSM, but there were two eQTLs associated with altered OSMR (rs13168867) or LIFR (rs10491509) expression in arterial tissue. The C allele of rs13168867 is associated with decreased OSMR expression in the tibial artery (Figure 1A), and the A allele of rs10491509 is associated with increased LIFR expression in the aortic artery (Figure 1B). Cross-tissue meta-analysis showed that these variants have m-values >0.9 in both tibial and aortic artery tissue, indicating a high posterior probability that they are single cis-eQTLs in both tissues (Supplementary Figures 1, 2).
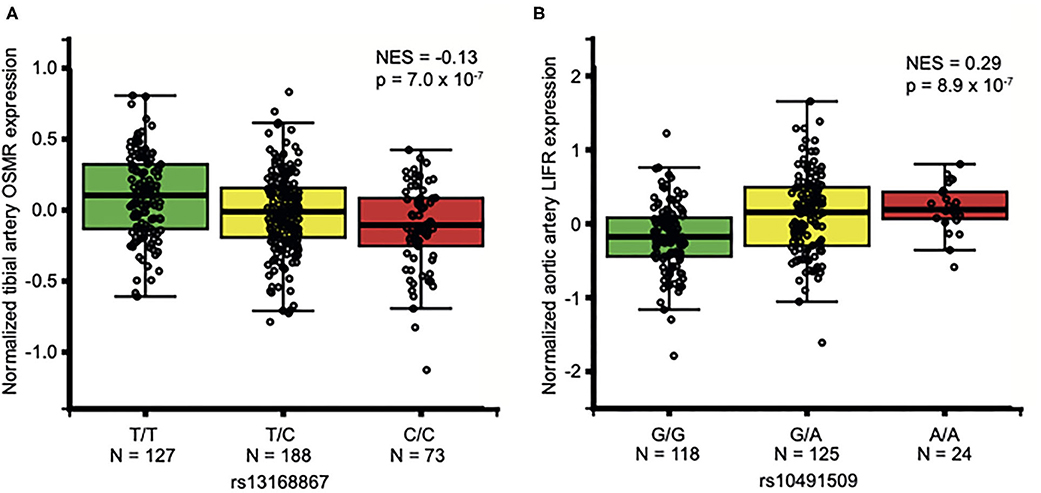
Figure 1. Association of OSMR and LIFR variants in non-diseased arterial tissues. Per variant, the normalized expression of OSMR (A) and LIFR (B) is given in non-diseased arterial tissue. Data from GTEx Portal (www.gtexportal.org). NES: Normalized effect size. In aortic arterial tissue, rs13168867 had a NES of −0.123 and in tibial arterial tissue, rs10491509 had a NES of 0.0881 (Supplementary Figures 1, 2).
Genetic Association With Plaque Vulnerability
To determine the effect of OSM signaling on the overall plaque vulnerability, we correlated the rs13168867 and rs10491509 genotypes to the overall plaque vulnerability, which was given a score ranging from 0 (least vulnerable plaque) to 4 (most vulnerable plaque). The effect allele of variant rs13168867 in the OSMR locus was significantly correlated with an increased overall plaque vulnerability (β = 0.118 ± s.e. = 0.040 (C allele), p = 3.00 × 10−3, Figure 2). No association was observed with rs10491509 and overall plaque vulnerability.
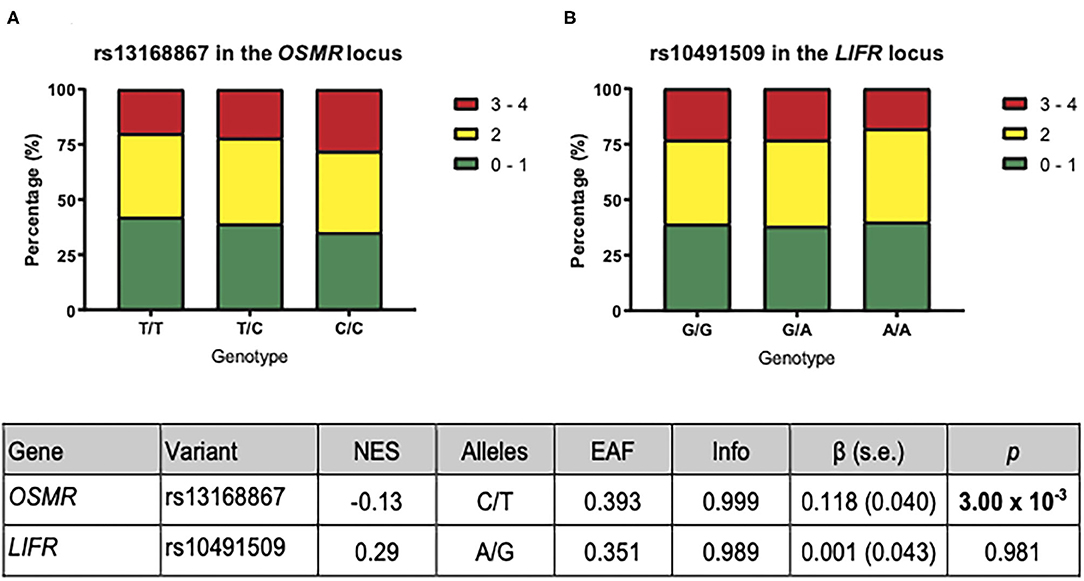
Figure 2. Association of OSMR and LIFR variants with overall plaque vulnerability. The plaques were given a vulnerability score ranging from 0 (least vulnerable) to 4 (most vulnerable). The bars represent the proportion of each plaque score per genotype for rs13168867 in the OSMR locus (A) and rs10491509 in the LIFR locus (B). The table shows the results from GTEx Portal (23) where Gene is the gene of interest; Variant is the eQTL as found in GTEx Portal, and NES refers to the normalized effect size on expression from GTEx Portal. The Alleles refer to the effect allele and the other allele in both GTEx Portal and the Athero-Express Biobank. The remaining columns in the table are referring to the analysis of these eQTLs with respect to plaque vulnerability in the Athero-Express Biobank Study. Here EAF represents effect allele frequency; Info refers to the estimated imputation score. The effect size (β) and standard error (s.e.) are relative to the effect allele; p indicates the p-value of association with plaque vulnerability of the given eQTL; Bold p: p-value of association surpasses significance threshold (p < 3.13 × 10−3).
Genetic Association With Plaque Phenotypes
To determine the effect of OSM signaling on the individual plaque characteristics comprising the overall plaque vulnerability, we assessed the association between rs13168867 and rs10491509 and seven plaque phenotypes in the Athero-Express Biobank Study. Although not significant after correction for multiple testing, the strongest associations were observed between the effect allele of variant rs13168867 in the OSMR locus and intraplaque fat (β = 0.248 ± s.e. = 0.088 (C allele), p = 4.66 × 10−3), and collagen content (β = −0.259 ± s.e. = 0.095 (C allele), p = 6.22 × 10−3, Table 2). No associations were observed between rs10491509 and any of the plaque phenotypes.
Known eQTLs of OSMR and LIFR Expression in Non-diseased Arterial Tissue Are Not Associated With Expression in Atherosclerotic Plaques
Atherosclerotic disease progression changes the artery-specific transcriptional dynamics, and may therefore abolish the effects of known OSMR and LIFR eQTLs in non-atherosclerotic arterial tissues. Thus, we tested whether these eQTLs were associated with OSMR and LIFR expression in carotid atherosclerotic plaques. Neither variant showed associations with expression of OSM, OSMR, and LIFR (Supplementary Table 1).
Intraplaque OSMR Expression Is Not Associated With Plaque Vulnerability
As rs13168867 was associated with an increased overall plaque vulnerability, we next investigated if intraplaque OSMR expression levels associated with overall plaque vulnerability. Differential expression analyses, comparing the reference score (0) with each increasing vulnerability score (1, 2, 3, or 4) showed no associations between OSMR plaque expression levels and plaque vulnerability (Supplementary Table 2). Neither did intraplaque OSM or LIFR expression associate with plaque severity.
Known eQTLs of OSMR and LIFR Do Not Associate With Cardiovascular Diseases
The Athero-Express comprises patients with advanced stage atherosclerotic plaques. Therefore, we assessed the effect of known OSMR and LIFR eQTLs on coronary calcification (CAC) as intermediate phenotype of atherosclerotic burden, and primary cardiovascular outcomes as clinical manifestation. We queried summary statistics from GWAS on CAC (n = 2,674) (45), coronary artery disease (CAD, n = 336,755) (45, 46), and ischemic stroke subtypes (sample sizes 242,573–522,258) (45–47). Neither eQTL associated with increased CAC burden, or cardiovascular disease susceptibility (Supplementary Table 3).
Discussion
We investigated whether common variants associated to gene expression, i.e., eQTLs, near OSM, OSMR and LIFR affect overall plaque vulnerability and phenotype. We showed that one cis-acting eQTL (rs13168867, C/T), of which the C allele associates with reduced OSMR expression in non-diseased arterial tissue, significantly associates with increased plaque vulnerability after correction for multiple testing. This suggests that a decrease in OSMR expression and therefore possibly a decrease in OSM signaling, increases the chance of developing a vulnerable plaque.
To gain further insight into the role of genetically decreased OSMR expression on developing a vulnerable plaque, we examined the effect of rs13168867 on individual plaque characteristics in more detail. The strongest associations were found for rs13168867 with increased intraplaque fat and decreased collagen content, suggesting that reduced OSM signaling results in a larger lipid core and less fibrosis - in line with a more vulnerable plaque phenotype. We previously showed that OSM enhances intercellular adhesion molecule (ICAM)-1 expression on human endothelial cells (8). Reduced OSMR expression, which hypothetically results in reduced OSM signaling, may therefore result in reduced ICAM-1 expression. ICAM-1 depletion leads to M1 macrophage polarization (48), which is the pro-inflammatory macrophage subtype that promotes an unstable plaque phenotype (49). Reduced OSM signaling could also explain the decreased collagen content as OSM enhances in vitro fibroblast proliferation and collagen formation (20). Moreover, it was previously shown that OSM enhances liver fibrosis in mice (50) and that OSM is upregulated in patients with pulmonary fibrosis (51). A reduction in OSM signaling caused by decreased OSMR expression may therefore result in decreased collagen content. Further studies are needed to investigate these hypotheses.
A possible explanation for the lack of associations for the variant (rs10491509) in the LIFR locus could be that an increase in LIFR expression would not affect OSM signaling as, hypothetically, there might already be a LIFR surplus and therefore, an increase in LIFR expression will not affect OSM signaling.
Although rs13168867 did associate with plaque vulnerability, no associations were found between rs13168867 and intraplaque OSMR expression, intraplaque OSMR expression and plaque vulnerability, nor did rs13168867 associate with cardiovascular disease outcomes. Possibly, OSM signaling mainly affects atherogenesis and atherosclerosis development in the initial phases of the disease. Arterial OSMR expression is reduced in human atherosclerotic plaques when compared to normal arteries9 and may therefore have bigger effects in the initial phase, when OSMR expression is still high. Another possible explanation is that OSM signaling may be overruled by for example, other cytokines in later stages of the disease. Lastly, although coronary thrombosis, and therefore cardiovascular disease, is most often caused by plaque rupture, which is most likely to happen in vulnerable plaques, thrombosis can also be triggered by other processes, including plaque erosion and atrial fibrillation (52, 53). Xie et al. showed that OSM is associated with thrombosis in patients with atrial fibrillation and suggested that OSM exerts thrombogenic effects by increasing tissue factor expression and decreasing the expression of tissue factor pathway inhibitors (53). So, OSM could potentially increase the risk of cardiovascular disease through its thrombogenic effects and at the same time decrease the risk of cardiovascular disease by its atheroprotective effects. Potentially, the seemingly atheroprotective effect of OSM that we described in our current study may be neutralized by the thrombogenic or potential other cardiovascular disease driving effects of OSM.
A limitation of association studies like ours, is that it is challenging to uncover the biological meaning of the discovered associations. It is likely that a reduction in OSMR expression on the arterial wall reduces OSM signaling, but this is difficult to verify. Firstly, OSM signaling is not only dependent on OSMR, but also on the blood OSM levels. If there is no or little OSM present in the blood, there might have been a surplus of OSMR and in this case, there will be no change in OSM signaling. Another possibility is that there is not only a decrease of OSMR expression on the arterial wall, but also a decrease in circulating OSMR levels, which can also bind to OSM and acts as a neutralizer (54), also resulting in no net difference in OSM signaling. Moreover, this study cannot make a distinction between the timing and the duration of OSM signaling, which may differentially affect atherosclerosis development as previous studies have shown that OSM, like IL-6, can act differently in the acute phase than in the chronic phase (8, 9, 55, 56). Finally, we focused on only three genes (OSM, OSMR and LIFR), while atherosclerosis is a multifactorial disease. Although studies like ours can be very insightful to better understand the disease, single variants seldomly show big correlations with disease outcome.
Compared to genome-wide association studies that include thousands of individuals, the Athero-Express Biobank Study is relatively small (n=1,443), and, given its design, finite in size. However, it is well suited to examine the effect of common disease-associated genetic variation on plaque morphology and characteristics. Indeed, we estimated the power at ±75% given a MAF=0.40 (approximately the frequency of rs13168867) and relative risk = 1.28 (http://csg.sph.umich.edu/abecasis/gas_power_calculator/).
Recent developments in single-cell expression analyses might extend on the present study by investigating which cell types, that are present in the plaque, most abundantly express OSM, OSMR and LIFR. Furthermore, it would be interesting to investigate if the OSMR/LIFR expression ratio correlates with plaque vulnerability and if this ratio might be a predictor of plaque vulnerability.
Conclusion
Based on this work we conclude that the variant rs13168867 in the OSMR locus is associated with increased plaque vulnerability, but not with coronary calcification or cardiovascular disease susceptibility. Given the multiple testing burden for individual plaque characteristics, it remains unclear through which precise biological mechanisms OSM signaling exerts its effects on plaque morphology, although our data point toward lipid metabolism and extracellular matrix remodeling. However, the OSM-OSMR/LIFR pathway is unlikely to be causally involved in lifetime cardiovascular disease susceptibility as none of the investigated eQTLs associated with cardiovascular diseases.
Data Availability Statement
Data can be accessed at https://dataverse.nl/ using accession number 0RB5IZ.
Ethics Statement
The studies involving human participants were reviewed and approved by Medisch Ethische Toetsingscommissie (METC) Utrecht. The patients/participants provided their written informed consent to participate in this study.
Author Contributions
DvK: conceptualization, formal analysis, and writing - original draft. IvK: data curation. AB: conceptualization, formal analysis, and writing – review & editing. HP and AvG: writing – review & editing. GdB and DT: conceptualization. DT and GP: conceptualization and writing – review & editing. SvL: conceptualization, formal analysis, writing – original draft and review & editing. All authors contributed to the article and approved the submitted version.
Funding
SvL was funded through grants from the Netherlands CardioVascular Research Initiative of the Netherlands Heart Foundation [CVON 2011/B019 and CVON 2017-20: Generating the best evidence-based pharmaceutical targets for atherosclerosis (GENIUS I&II)]. This work was supported by ERA-CVD, grant number: 01KL1802. FA was supported by UCL Hospitals NIHR Biomedical Research Center. DvK, HP, and DT were funded through the FP7 EU project CarTarDis (FP7/2007-2013) under grant agreement 602936. AB was funded through the Taxinomisis grant, part of the European Union's Horizon 2020 research and innovation program (No 755320). HP received funding from the TNO research program Preventive Health Technologies. The funding sources were not involved in study design, collection, analysis and interpretation of data, writing of the report and in the decision to submit the article for publication.
Conflict of Interest
DvK is employed by Quorics B.V., and DT is employed by SkylineDx B.V and Quorics B.V. Quorics B.V. and SkylineDx B.V. had no part whatsoever in the conception, design, or execution of this study, nor the preparation and contents of this manuscript.
The remaining authors declare that the research was conducted in the absence of any commercial or financial relationships that could be construed as a potential conflict of interest.
Acknowledgments
We would like to thank Dr. Jessica van Setten and acknowledge her for imputing our datasets using an in-house developed imputation pipeline. Evelyn Velema and Petra Homoet-Van der Kraak are acknowledged for the immunohistochemical stainings. We also acknowledge the support from the Netherlands CardioVascular Research Initiative from the Dutch Heart Foundation, Dutch Federation of University Medical Centers, the Netherlands Organization for Health Research and Development and the Royal Netherlands Academy of Sciences (GENIUS I & II, CVON2011-19) and the TNO research program Preventive Health Technologies.
Supplementary Material
The Supplementary Material for this article can be found online at: https://www.frontiersin.org/articles/10.3389/fcvm.2021.658915/full#supplementary-material
References
1. Moodie DS. The global burden of cardiovascular disease. Congenit Heart Dis. (2016) 11:213. doi: 10.1111/chd.12383
2. Hansson GK. Inflammation, atherosclerosis, and coronary artery disease. N Engl J Med. (2005) 352:1685–95. doi: 10.1056/NEJMra043430
3. Sprague AH, Khalil RA. Inflammatory cytokines in vascular dysfunction and vascular disease. Biochem Pharmacol. (2009) 78:539–52. doi: 10.1016/j.bcp.2009.04.029
4. Vasse M, Pourtau J, Trochon V, Muraine M, Vannier J-P, Lu H, et al. Oncostatin M induces angiogenesis in vitro and in vivo. Arterioscler Thromb Vasc Biol. (1999) 19:1835–42. doi: 10.1161/01.ATV.19.8.1835
5. Nagata T, Kai H, Shibata R, Koga M, Yoshimura A, Imaizumi T. Oncostatin M, an interleukin-6 family cytokine, upregulates matrix metalloproteinase-9 through the mitogen-activated protein kinase kinase-extracellular signal-regulated kinase pathway in cultured smooth muscle cells. Arterioscler Thromb Vasc Biol. (2003) 23:588–93. doi: 10.1161/01.ATV.0000060891.31516.24
6. Albasanz-Puig A, Murray J, Preusch M, Coan D, Namekata M, Patel Y, et al. Oncostatin M is expressed in atherosclerotic lesions: a role for Oncostatin M in the pathogenesis of atherosclerosis. Atherosclerosis. (2011) 216:292–8. doi: 10.1016/j.atherosclerosis.2011.02.003
7. Zhang X, Li J, Qin J-J, Cheng W-L, Zhu X, Gong F-H, et al. Oncostatin M receptor β deficiency attenuates atherogenesis by inhibiting JAK2/STAT3 signaling in macrophages. J Lipid Res. (2017) 58:895–906. doi: 10.1194/jlr.M074112
8. Van Keulen D, Pouwer MG, Pasterkamp G, Van Gool AJ, Sollewijn Gelpke MD, Princen HMG, et al. Inflammatory cytokine oncostatin M induces endothelial activation in macro-and microvascular endothelial cells and in APOE*3Leiden.CETP mice. PLoS ONE. (2018) 13:e0204911. doi: 10.1016/j.atherosclerosissup.2018.04.056
9. van Keulen D, Pouwer MG, Emilsson V, Matic LP, Pieterman EJ, Hedin U, et al. Oncostatin M reduces atherosclerosis development in APOE3Leiden.CETP mice and is associated with increased survival probability in humans. PLoS One. (2019) 14:e0221477. doi: 10.1371/journal.pone.0221477
10. Brown TJ, Lioubin MN, Marquardt H. Purification and characterization of cytostatic lymphokines produced by activated human T lymphocytes. Synergistic antiproliferative activity of transforming growth factor beta 1, interferon-gamma, and oncostatin M for human melanoma cells. J Immunol. (1987) 139:2977–83.
11. Kastl SP, Speidl WS, Kaun C, Katsaros KM, Rega G, Afonyushkin T, et al. In human macrophages the complement component C5a induces the expression of oncostatin M via AP-1 activation. Arterioscler Thromb Vasc Biol. (2008) 28:498–503. doi: 10.1161/ATVBAHA.107.160580
12. Grenier A, Dehoux M, Boutten A, Arce-Vicioso M, Durand G, Gougerot-Pocidalo M-A, et al. Oncostatin M production and regulation by human polymorphonuclear neutrophils. Blood. (1999) 93:1413–21. doi: 10.1182/blood.V93.4.1413
13. Tanaka M, Miyajima A. Oncostatin M, a multifunctional cytokine. Rev Physiol Biochem Pharmacol. (2003) 149:39–52. doi: 10.1007/s10254-003-0013-1
14. Dey G, Radhakrishnan A, Syed N, Thomas JK, Nadig A, Srikumar K, et al. Signaling network of Oncostatin M pathway. J Cell Commun Signal. (2013) 7:103–8. doi: 10.1007/s12079-012-0186-y
15. Mosley B, De Imus C, Friend D, Boiani N, Thoma B, Park LS, et al. Dual oncostatin M (OSM) receptors. Cloning and characterization of an alternative signaling subunit conferring OSM-specific receptor activation. J Biol Chem. (1996) 271:32635–43. doi: 10.1074/jbc.271.51.32635
16. Takata F, Sumi N, Nishioku T, Harada E, Wakigawa T, Shuto H, et al. Oncostatin M induces functional and structural impairment of blood–brain barriers comprised of rat brain capillary endothelial cells. Neurosci Lett. (2008) 441:163–6. doi: 10.1016/j.neulet.2008.06.030
17. Guihard P, Danger Y, Brounais B, David E, Brion R, Delecrin J, et al. Induction of osteogenesis in mesenchymal stem cells by activated monocytes/macrophages depends on oncostatin M signaling. Stem Cells. (2012) 30:762–72. doi: 10.1002/stem.1040
18. Virmani R, Kolodgie FD, Burke AP, Finn A V, Gold HK, Tulenko TN, et al. Atherosclerotic plaque progression and vulnerability to rupture angiogenesis as a source of intraplaque hemorrhage plaque rupture is the dominant cause of acute coronary thrombosis. Arterioscler Thromb Vasc Biol. (2005) 25:2054–61. doi: 10.1161/01.ATV.0000178991.71605.18
19. Hutcheson JD, Maldonado N, Aikawa E. Small entities with large impact: microcalcifications and atherosclerotic plaque vulnerability. Curr Opin Lipidol. (2014) 25:327–32. doi: 10.1097/MOL.0000000000000105
20. Scaffidi AK, Mutsaers SE, Moodley YP, McAnulty RJ, Laurent GJ, Thompson PJ, et al. Oncostatin M stimulates proliferation, induces collagen production and inhibits apoptosis of human lung fibroblasts. Br J Pharmacol. (2002) 136:793–801. doi: 10.1038/sj.bjp.0704769
21. Shrivastava R, Asif M, Singh V, Dubey P, Ahmad Malik S, Lone M-U-D, et al. M2 polarization of macrophages by Oncostatin M in hypoxic tumor microenvironment is mediated by mTORC2 and promotes tumor growth and metastasis. Cytokine. (2018) 118:130–43. doi: 10.1016/j.cyto.2018.03.032
22. Van Der Wal AC, Becker AE. Atherosclerotic plaque rupture - pathologic basis of plaque stability and instability. Cardiovasc Res. (1999) 41:334–44. doi: 10.1016/S0008-6363(98)00276-4
23. Lonsdale J, Thomas J, Salvatore M, Phillips R, Lo E, Shad S, et al. The genotype-tissue expression (GTEx) project. Nat Genet. (2013) 45:580–5. doi: 10.1038/ng.2653
24. Grundberg E, Small KS, Hedman ÅK, Nica AC, Buil A, Keildson S, et al. Mapping cis-and trans-regulatory effects across multiple tissues in twins. Nat Genet. (2012) 44:1084–9. doi: 10.1038/ng.2394
25. Verhoeven B, Hellings WE, Moll FL, De Vries JP, De Kleijn DPV, De Bruin P, et al. Carotid atherosclerotic plaques in patients with transient ischemic attacks and stroke have unstable characteristics compared with plaques in asymptomatic and amaurosis fugax patients. J Vasc Surg. (2005) 42:1075–81. doi: 10.1016/j.jvs.2005.08.009
26. Van Der Laan SW, Foroughi Asl H, van den Borne P, van Setten J, van der Perk MEM, van de Weg SM, et al. Variants in ALOX5, ALOX5AP and LTA4H are not associated with atherosclerotic plaque phenotypes: The Athero-Express Genomics Study. Atherosclerosis. (2015) 239:528–38. doi: 10.1016/j.atherosclerosis.2015.01.018
27. O'Connell J, Gurdasani D, Delaneau O, Pirastu N, Ulivi S, Cocca M, et al. A general approach for haplotype phasing across the full spectrum of relatedness. PLoS Genet. (2014) 10:1004234. doi: 10.1371/journal.pgen.1004234
28. Auton A, Abecasis GR, Altshuler DM, Durbin RM, Bentley DR, Chakravarti A, et al. A global reference for human genetic variation. Nature. (2015) 526:68–74. doi: 10.1038/nature15393
29. Francioli LC, Menelaou A, Pulit SL, Van Dijk F, Palamara PF, Elbers CC, et al. Whole-genome sequence variation, population structure and demographic history of the Dutch population. Nat Genet. (2014) 46:818–25. doi: 10.1038/ng.3021
30. Aguet F, Barbeira AN, Bonazzola R, Brown A, Castel SE, Jo B, et al. The GTEx Consortium atlas of genetic regulatory effects across human tissues. Science (80-). (2020) 369:1318–30. doi: 10.1126/science.aaz1776
31. Verhoeven BAN, Velema E, Schoneveld AH, De Vries JPPM, De Bruin P, Seldenrijk CA, et al. Athero-express: differential atherosclerotic plaque expression of mRNA and protein in relation to cardiovascular events and patient characteristics. Rationale and design. Eur J Epidemiol. (2004) 19:1127–33. doi: 10.1007/s10564-004-2304-6
32. Van Lammeren GW, Den Ruijter HM, Vrijenhoek JEP, Van Der Laan SW, Velema E, De Vries JPPM, et al. Time-dependent changes in atherosclerotic plaque composition in patients undergoing carotid surgery. Circulation. (2014) 129:2269–76. doi: 10.1161/CIRCULATIONAHA.113.007603
33. Hellings WE, Pasterkamp G, Vollebregt A, Seldenrijk CA, De Vries JPPM, Velema E, et al. Intraobserver and interobserver variability and spatial differences in histologic examination of carotid endarterectomy specimens. J Vasc Surg. (2007) 46:1147–54. doi: 10.1016/j.jvs.2007.08.018
34. Hashimshony T, Wagner F, Sher N, Yanai I. CEL-Seq: single-cell RNA-Seq by multiplexed linear amplification. Cell Rep. (2012) 2:666–73. doi: 10.1016/j.celrep.2012.08.003
35. Hashimshony T, Senderovich N, Avital G, Klochendler A, de Leeuw Y, Anavy L, et al. CEL-Seq2: sensitive highly-multiplexed single-cell RNA-Seq. Genome Biol. (2016) 17:77. doi: 10.1186/s13059-016-0938-8
36. Ferraz MAMM, Rho HS, Hemerich D, Henning HHW, van Tol HTA, Hölker M, et al. An oviduct-on-a-chip provides an enhanced in vitro environment for zygote genome reprogramming. Nat Commun. (2018) 9:1–14. doi: 10.1038/s41467-018-07119-8
37. Li H, Durbin R. Fast and accurate short read alignment with burrows–wheeler transform. Bioinformatics. (2009) 25:1754–60. doi: 10.1093/bioinformatics/btp324
38. Morgan M, Obenchain V, Hester J, Pagès H. Summarized Experiment: Summarized Experiment container. R package version 1.20.0 (2020). Available online at: https://bioconductor.org/packages/SummarizedExperiment
39. Love MI, Huber W, Anders S. Moderated estimation of fold change and dispersion for RNA-seq data with DESeq2. Genome Biol. (2014) 15:550. doi: 10.1186/s13059-014-0550-8
40. Box GEP, Cox DR. An analysis of transformations. J. R. Stat. Soc. (1964) 26:211–43. doi: 10.1111/j.2517-6161.1964.tb00553.x
41. Marchini J, Howie B, Myers S, McVean G, Donnelly P. A new multipoint method for genome-wide association studies by imputation of genotypes. Nat Genet. (2007) 39:906–13. doi: 10.1038/ng2088
42. Thoma B, Bird TA, Friend DJ, Gearing DP, Dower SK. Oncostatin M and leukemia inhibitory factor trigger overlapping and different signals through partially shared receptor complexes. J Biol Chem. (1994) 269:6215–22. doi: 10.1016/S0021-9258(17)37590-7
43. Hermanns H, Radtke S, Haan c, Schmitz-Van de Leur H, Tavernier J, Heinrich P, et al. Contributions of leukemia inhibitory factor receptor and oncostatin M receptor to signal transduction in heterodimeric complexes with glycoprotein 130 - PubMed. J Immunol. (1999) 163:6651–8.
44. Rolfe B, Stamatiou S, World C, Brown L, Thomas A, Bingley J. Leukaemia inhibitory factor retards the progression of atherosclerosis. Cardiovasc Res. (2003) 58:222–30. doi: 10.1016/S0008-6363(02)00832-5
45. van Setten J, Isgum I, Smolonska J, Ripke S, de Jong PA, Oudkerk M, et al. Genome-wide association study of coronary and aortic calcification implicates risk loci for coronary artery disease and myocardial infarction. Atherosclerosis. (2013) 228:400–5. doi: 10.1016/j.atherosclerosis.2013.02.039
46. Nelson CP, Goel A, Butterworth AS, Kanoni S, Webb TR, Marouli E, et al. Association analyses based on false discovery rate implicate new loci for coronary artery disease. Nat Genet. (2017) 49:1385–91. doi: 10.1038/ng.3913
47. Malik R, Chauhan G, Traylor M, Sargurupremraj M, Okada Y, Mishra A, et al. Publisher correction: multiancestry genome-wide association study of 520,000 subjects identifies 32 loci associated with stroke and stroke subtypes. Nat Genet. (2019) 51:1192–3. doi: 10.1038/s41588-018-0058-3
48. Gu W, Yao L, Li L, Zhang J, Place AT, Minshall RD, et al. ICAM-1 regulates macrophage polarization by suppressing MCP- 1 expression via miR-124 upregulation. Oncotarget. (2017) 8:111882–901. doi: 10.18632/oncotarget.22948
49. Barrett TJ. Macrophages in atherosclerosis regression. Arterioscler Thromb Vasc Biol. (2020) 40:20–33. doi: 10.1161/ATVBAHA.119.312802
50. Matsuda M, Tsurusaki S, Miyata N, Saijou E, Okochi H, Miyajima A, et al. Oncostatin M causes liver fibrosis by regulating cooperation between hepatic stellate cells and macrophages in mice. Hepatology. (2018) 67:296–312. doi: 10.1002/hep.29421
51. Mozaffarian A, Brewer AW, Trueblood ES, Luzina IG, Todd NW, Atamas SP, et al. Mechanisms of Oncostatin M-induced pulmonary inflammation and fibrosis. J Immunol. (2008) 181:7243–53. doi: 10.4049/jimmunol.181.10.7243
52. Bentzon JF, Otsuka F, Virmani R, Falk E. Mechanisms of plaque formation and rupture. Circ Res. (2014) 114:1852–66. doi: 10.1161/CIRCRESAHA.114.302721
53. Xie J, Zhu S, Dai Q, Lu J, Chen J, Li G, et al. Oncostatin M was associated with thrombosis in patients with atrial fibrillation. Medicine. (2017) 96:e6806. doi: 10.1097/MD.0000000000006806
54. Diveu C, Venereau E, Froger J, Ravon E, Grimaud L, Rousseau F, et al. Molecular and functional characterization of a soluble form of Oncostatin M/interleukin-31 shared receptor. J Biol Chem. (2006) 281:36673–382. doi: 10.1074/jbc.M607005200
55. Low ASL, Symmons DPM, Lunt M, Mercer LK, Gale CP, Watson KD, et al. Relationship between exposure to tumour necrosis factor inhibitor therapy and incidence and severity of myocardial infarction in patients with rheumatoid arthritis. Ann Rheum Dis. (2017) 76:654–60. doi: 10.1136/annrheumdis-2016-209784
Keywords: cardiovascular disease, atherosclerosis, plaque, genetics, OSM, OSMR, LIFR
Citation: van Keulen D, van Koeverden ID, Boltjes A, Princen HMG, van Gool AJ, de Borst GJ, Asselbergs FW, Tempel D, Pasterkamp G and van der Laan SW (2021) Common Variants Associated With OSMR Expression Contribute to Carotid Plaque Vulnerability, but Not to Cardiovascular Disease in Humans. Front. Cardiovasc. Med. 8:658915. doi: 10.3389/fcvm.2021.658915
Received: 26 January 2021; Accepted: 09 March 2021;
Published: 20 April 2021.
Edited by:
Seitaro Nomura, The University of Tokyo, JapanReviewed by:
Christoph Sinning, University Heart and Vascular Center Hamburg (UHZ), GermanyLasse Folkersen, Danish National Genome Center, Denmark
Copyright © 2021 van Keulen, van Koeverden, Boltjes, Princen, van Gool, de Borst, Asselbergs, Tempel, Pasterkamp and van der Laan. This is an open-access article distributed under the terms of the Creative Commons Attribution License (CC BY). The use, distribution or reproduction in other forums is permitted, provided the original author(s) and the copyright owner(s) are credited and that the original publication in this journal is cited, in accordance with accepted academic practice. No use, distribution or reproduction is permitted which does not comply with these terms.
*Correspondence: Gerard Pasterkamp, Zy5wYXN0ZXJrYW1wQHVtY3V0cmVjaHQubmw=; Sander W. van der Laan, cy53LnZhbmRlcmxhYW4tMkB1bWN1dHJlY2h0Lm5s
†These authors have contributed equally to this work