- 1Department of Cardiology, Zhongshan Hospital, Shanghai Institute of Cardiovascular Diseases, Fudan University, Shanghai, China
- 2Department of Cardiovascular Surgery, Zhongshan Hospital, Fudan University, Shanghai, China
- 3Department of Cardiovascular Surgery, Shanghai East Hospital, Tongji University School of Medicine, Shanghai, China
Background: Coronary artery disease (CAD) is the leading cause of cardiovascular death. The competitive endogenous RNAs (ceRNAs) hypothesis is a new theory that explains the relationship between lncRNAs and miRNAs. The mechanism of ceRNAs in the pathological process of CAD has not been fully elucidated. The objective of this study was to explore the ceRNA mechanism in CAD using the integrative bioinformatics analysis and provide new research ideas for the occurrence and development of CAD.
Methods: The GSE113079 dataset was downloaded, and differentially expressed lncRNAs (DElncRNAs) and genes (DEGs) were identified using the limma package in the R language. Weighted gene correlation network analysis (WGCNA) was performed on DElncRNAs and DEGs to explore lncRNAs and genes associated with CAD. Functional enrichment analysis was performed on hub genes in the significant module identified via WGCNA. Four online databases, including TargetScan, miRDB, miRTarBase, and Starbase, combined with an online tool, miRWalk, were used to construct ceRNA regulatory networks.
Results: DEGs were clustered into ten co-expression modules with different colors using WGCNA. The brown module was identified as the key module with the highest correlation coefficient. 188 hub genes were identified in the brown module for functional enrichment analysis. DElncRNAs were clustered into sixteen modules, including seven modules related to CAD with the correlation coefficient more than 0.5. Three ceRNA networks were identified, including OIP5-AS1-miR-204-5p/miR-211-5p-SMOC1, OIP5-AS1-miR-92b-3p-DKK3, and OIP5-AS1-miR-25-3p-TMEM184B.
Conclusion: Three ceRNA regulatory networks identified in this study may play crucial roles in the occurrence and development of CAD, which provide novel insights into the ceRNA mechanism in CAD.
Introduction
Cardiovascular disease (CVD) is one of the leading causes of death in the world. Coronary artery disease (CAD) is the main cause of cardiovascular death (1), which increases the morbidity, mortality, and economic burden on societies worldwide (2). The occurrence of CAD is associated with the interplay of genetic and environmental factors (3). Diabetes, hypertension, obesity, and smoking are significant risk factors for CAD (4, 5). Vascular stenosis caused by CAD is the main cause of coronary atherosclerosis and ischemia. The sclerotic plaques are at risk of rupture, leading to myocardial infarction and eventually death (4, 5). Although considerable efforts have been made, it remains a daunting task to prevent and cure CAD. Therefore, further research is urgently needed to understand its pathophysiological process.
Non-coding RNA (ncRNA), including lncRNA, circRNA, and miRNA, is a kind of RNA that does not encode proteins, which plays an essential role in the occurrence and development of CAD. LncRNAs are transcripts with a length of >200 nucleotides participating in a variety of critical biological processes (6). It was reported that lncRNAs play a significant role in the core stages of CAD, including lipid metabolism, inflammation, vascular cell proliferation, apoptosis, adhesion and migration, and angiogenesis (7). The competitive endogenous RNAs (ceRNAs) hypothesis is a theory that explains the relationship between lncRNAs and miRNAs. In this hypothesis, lncRNAs rich in miRNA binding sites can bind miRNAs and act as a miRNA sponge, leading to changes in expression levels of miRNA-target genes (8, 9). Although a growing body of evidence demonstrated that ncRNAs were associated with the development of CAD, research on the role of ceRNAs in the pathological process of CAD is still insufficient. Therefore, further efforts are warranted to elucidate the ceRNA mechanism in CAD.
In this study, we aimed to explore the complex interaction between lncRNAs, miRNAs, and mRNAs to investigate the potential mechanism of ceRNAs in CAD. We performed weighted gene correlation network analysis (WGCNA) on the GSE113079 to screen out lncRNAs and mRNAs associated with CAD. Then several online databases and tools were used to construct the ceRNA regulatory networks. We hope this study can provide potential targets and new research ideas for understanding the ceRNA mechanism in CAD.
Methods and Materials
Data Processing
The GSE113079 dataset was downloaded from the Gene Expression Omnibus (GEO) database. The dataset was based on the GPL20115 platform (Agilent-067406 Human CBC lncRNA + mRNA microarray V4.0), containing 141 samples of peripheral blood mononuclear cells (PBMCs) in 93 patients with CAD and 48 healthy controls. The R language was applied to process the dataset. The Linear Models for Microarray data (limma), a package in the R language, was used to identify differentially expressed genes (DEGs) and differentially expressed lncRNAs (DElncRNAs) with the cut-off point of adj. p < 0.05.
WGCNA
DEGs identified by the limma package were imported for WGCNA to construct the gene co-expression network. First, the correlation network was constructed with an appropriate soft-thresholding power β realizing the scale-free topology criterion of R2 > 0.85. Second, the average linkage hierarchical clustering method was applied to cluster DEGs into different modules with different colors. The threshold for module merging was set as 0.25, and the minimum number of genes in each module was thirty. Hub genes correlation threshold was 0.9. Third, the correlation between each module and CAD was calculated using Pearson's correlation method. The module with a p < 0.05 and the highest correlation coefficient was screened out for further analysis. Similarly, DElncRNAs were also imported for WGCNA using the same parameters and procedures.
Functional Enrichment
The Database for Annotation, Visualization and Integrated Discovery (DAVID, v6.8) was used to perform the Gene Ontology (GO) enrichment analysis, which revealed the biological processes (BPs), cellular components (CCs), and molecular functions (MFs) related to hub genes in the module identified above. GO terms with a p < 0.05 were considered significant enrichment. Metascape, a powerful online tool for gene function annotation, was also applied for functional enrichment.
Construction of the ceRNA Network
The online tool miRWalk applies a machine-learning algorithm to predict miRNA-target interactions, including those that have been validated experimentally. Three online databases, including TargetScan, miRDB, and miRTarBase, combined with miRWalk, were used for the prediction of target miRNAs to ensure the robustness of the interactions between miRNAs and hub genes identified above. The Starbase is a public database that can search for potential miRNA-lncRNA interactions through high-throughput data. It was used to predict the relationships between target miRNAs and DElncRNAs to identify potential interactions. LncRNA-miRNA-mRNA regulatory network was visualized utilizing the Cytoscape software (v3.8.1).
Quantitative Real-Time PCR (qRT-PCR)
Ten patients with CAD and ten without CAD confirmed by coronary angiography were included in this study. This study was in full compliance with the Declaration of Helsinki and approved by the Medical Ethics Committee of Shanghai Tenth People's Hospital, Tongji University. Written informed consent was obtained from all subjects participating in this study. Blood samples were collected and total RNA was extracted following the manufacturer's instruction (QIAGEN, Frankfurt, Germany). Briefly, mix one volume of whole blood with five volumes of buffer in an eppendorf tube. After incubating for 15 min on ice, centrifuge at 3,000 rpm for 10 min at 4°C and discard supernatant. Transfer lysate to spin column to centrifuge at 14,000 rpm and pipet 50 μl of RNase-free water. The Complementary DNA (cDNA) was synthesized by reverse transcription at 42°C for 60 min and then at 95°C for 5 min with the PrimeScriptTM RT reagent Kit (Takara, Otsu, Japan). TB Green® Premix Ex TaqTM II (Takara, Otsu, Japan) was applied to perform qRT-PCR at the temperature of 95°C for 30 s, followed by 40 cycles with the temperature of 95°C for 5 s and 60°C for 34 s on QuantStudioTM 5 System (Thermo Fisher Scientific, Waltham, MA, USA). The expression of RNA levels was normalized by GAPDH and U6, and the 2−ΔΔCT method was applied to calculate the relative expression levels. All sequences for RNA primers (Sangon Biotech, Shanghai, China) are shown in Table 1.
Results
Identification of DEGs and DElncRNAs
A total of 20,128 DElncRNAs, including 6,103 upregulated and 14,025 downregulated, which were differentially expressed between CAD samples and healthy controls, were identified in the GSE113079 dataset with the limma package. And 11,487 DEGs were identified, including 5,993 upregulated and 5,494 downregulated.
WGCNA
DEGs with |logFC| > 0.5 were selected for WGCNA, and a scale-free co-expression network was established. The soft-thresholding power β was nine with scale-free R2 > 0.85 (Supplementary Figure 1). Then DEGs were clustered into ten co-expression modules through the average linkage hierarchical clustering method to ensure that the number of genes in each module is more than thirty. Different modules were represented by different colors, including black, blue, brown, greenyellow, pink, purple, red, tan, yellow, and gray (Figure 1). Genes in the gray module were uncorrelated and excluded from the subsequent analysis. We then calculated the correlation between module memberships and the gene significance for CAD (Supplementary Figure 2). Modules that meet the following two conditions were selected: (1) the correlation coefficient between the module and CAD was >0.5; (2) the correlation coefficient between module memberships and the gene significance for CAD was more than 0.7. According to the above criteria, 193 hub genes in the brown and pink modules were identified for further analysis. These genes were listed in Supplementary Table 1.
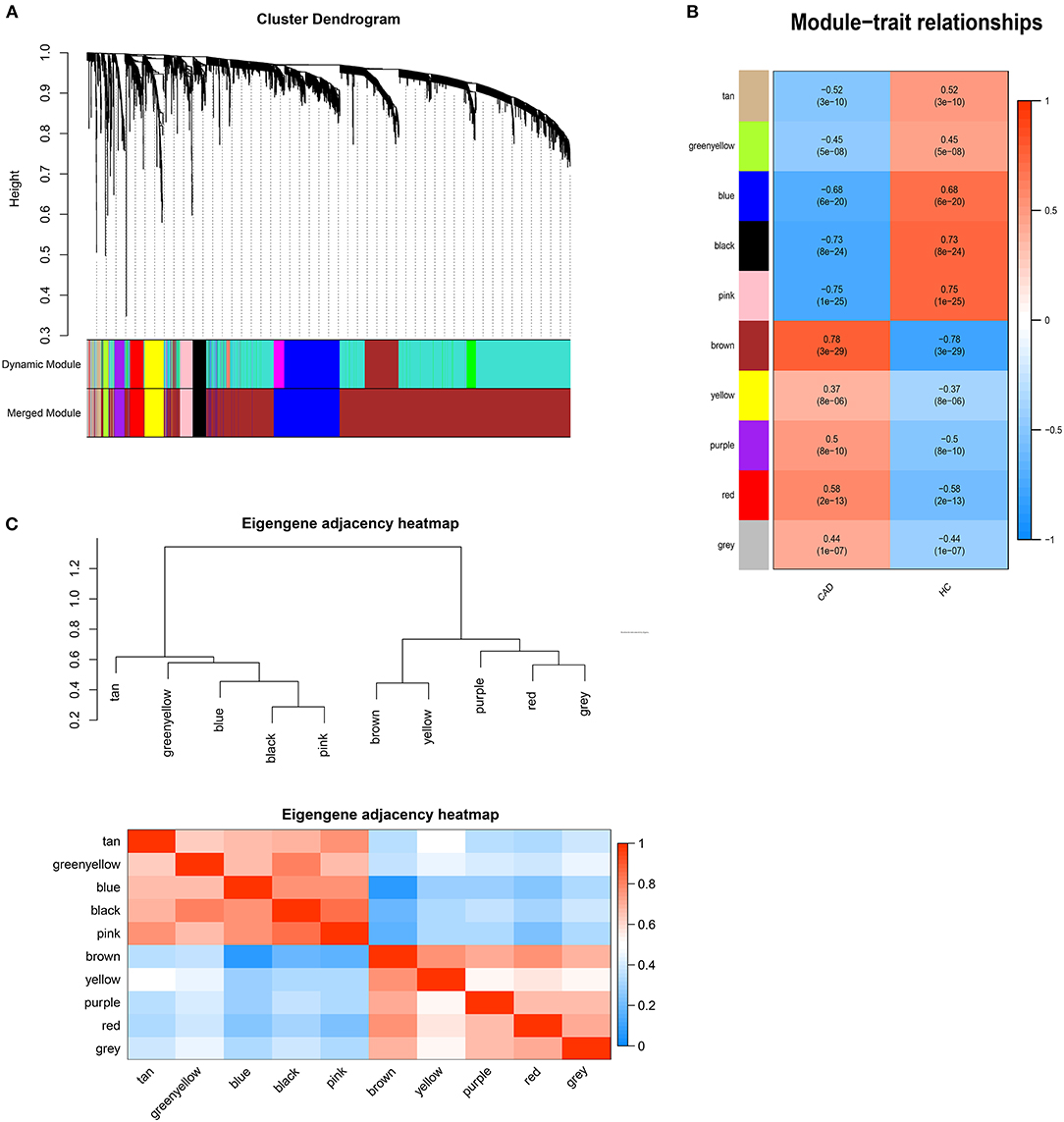
Figure 1. Weighted genes correlation network analysis. (A) The dendrogram of differentially expressed genes. (B) The heatmap of module-trait correlations. Blue represents negative correlation, and red represents positive correlation. (C) The clustering heatmap between modules. Red means closer similarity, and blue means farther similarity.
DElncRNAs identified by limma package with a p < 0.001 were selected for WGCNA. The soft-thresholding power β was ten to ensure scale-free R2 > 0.85 (Supplementary Figure 3). The DElncRNAs were clustered into sixteen modules with different colors, including black, darkgray, darkmagenta, darkorange, darkred, darkturquoise, lightgreen, lightyellow, midnightblue, orange, paleturquoise, red, royalblue, salmon, sienna3, and steelblue, excluding the gray module (Figure 2). The correlation between module memberships and the gene significance for CAD was also calculated (Supplementary Figure 4). Same as the module selection criteria above, the salmon and lightgreen modules were selected to construct the ceRNA networks.
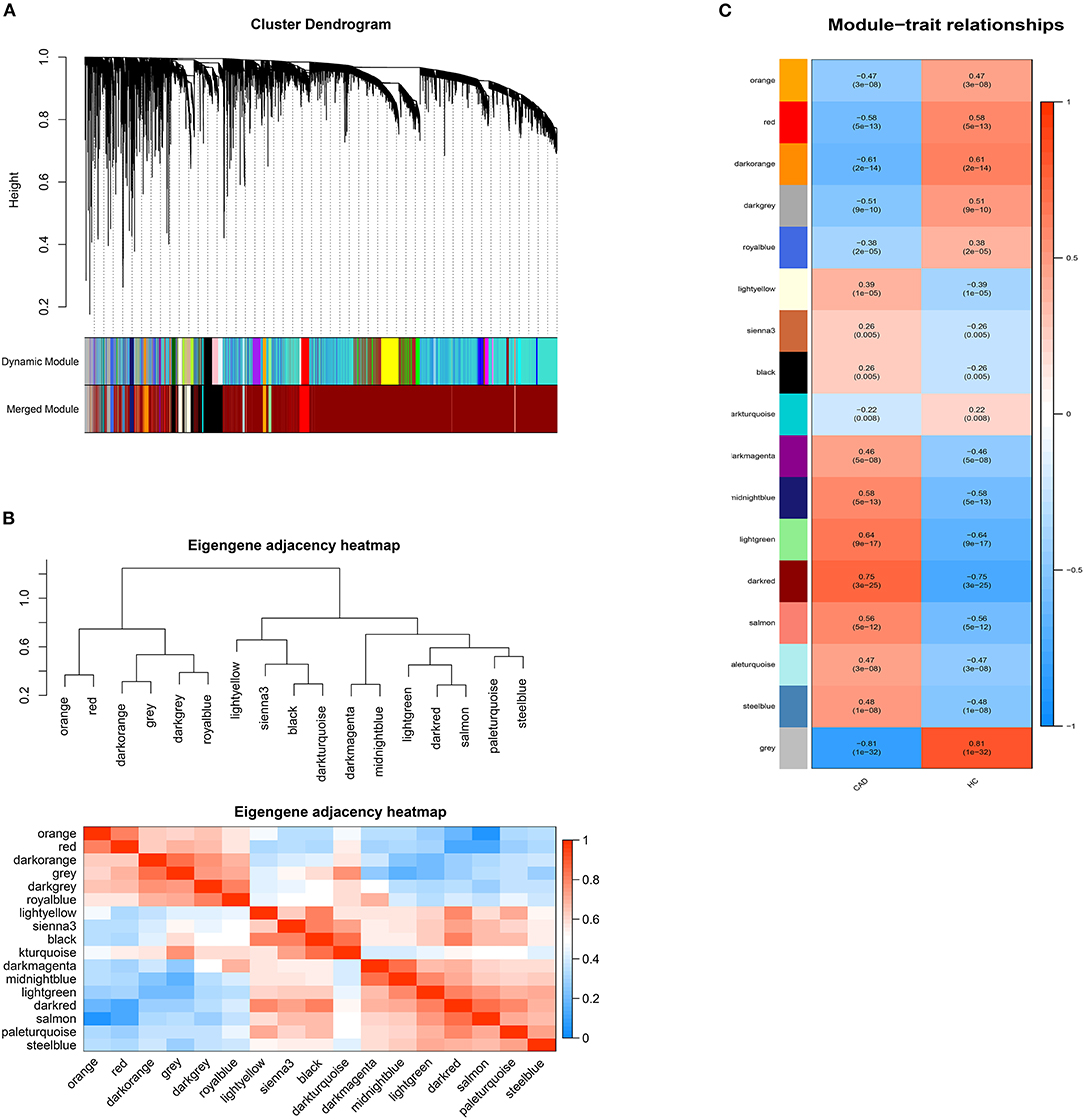
Figure 2. Weighted genes correlation network analysis. (A) The dendrogram of differentially expressed lncRNAs. (B) The heatmap of module-trait correlations. Blue represents negative correlation, and red represents positive correlation. (C) The clustering heatmap between modules. Red means closer similarity, and blue means farther similarity.
Functional Enrichment
193 hub genes in the brown and pink module were subjected to perform functional enrichment analysis utilizing the DAVID and Metascape online tool to investigate the biological effects. The significant enriched GOBPs included regulation of ion transmembrane transport, O-glycan processing, telencephalon cell migration, positive regulation of glucose import, and renal water homeostasis. In addition, golgi lumen, plasma membrane, and extracellular region were significantly enriched in GOCCs. For MF, the most significant entries were G-protein coupled receptor binding, channel activity, and passive transmembrane transporter activity (Figure 3). The results of Metascape demonstrated that hub genes were mainly enriched in the matrisome-associated pathway, cell-cell recognition, regulation of cellular component size, regulation of transmembrane transport, and cell-cell adhesion via plasma-membrane adhesion molecules (Figure 4).
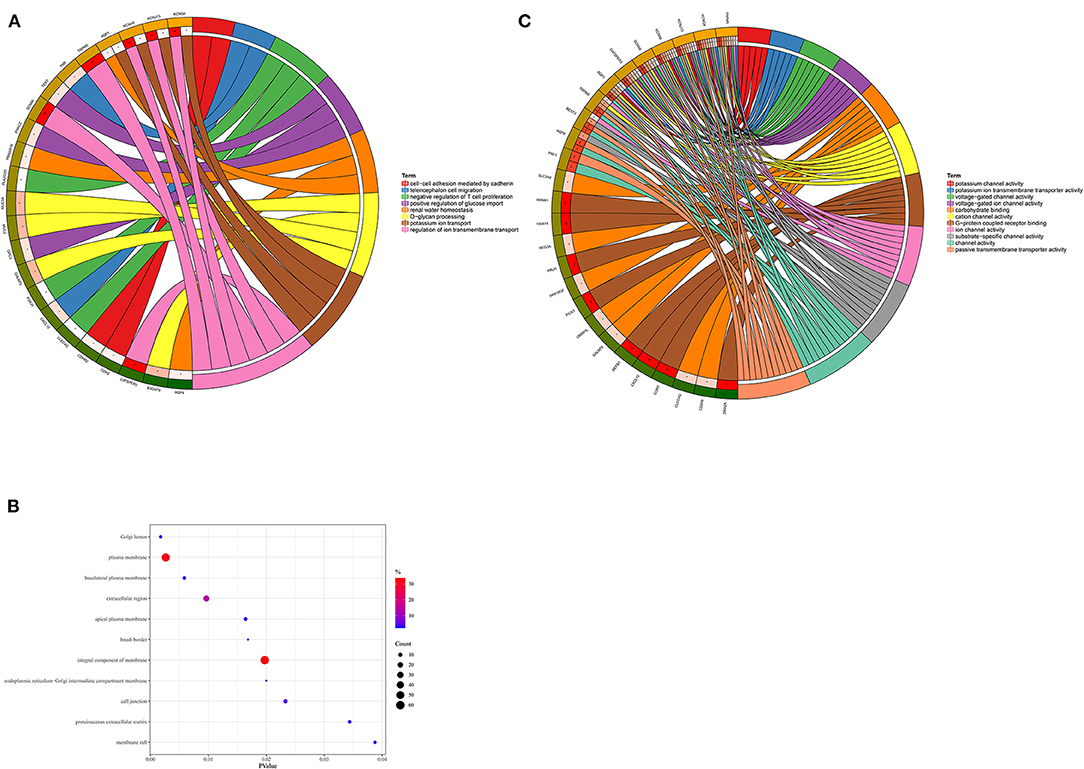
Figure 3. Gene Ontology enrichment analysis. (A) Biological process. (B) Cellular component. (C) Molecular function.
The ceRNA Regulatory Network
The intersection of the online tool miRWalk and three databases, TargetScan, miRDB, and miRTarBase was established to predict the target miRNAs of hub genes in the brown module. A total of seven miRNAs were screened out, including hsa-miR-195-3p, hsa-miR-188-5p, hsa-miR-204-5p, hsa-miR-211-5p, hsa-miR-526b-3p, hsa-miR-92b-3p, and hsa-miR-25-3p. Among them, hsa-miR-195-3p and hsa-miR-188-5p regulate UBE2I; hsa-miR-204-5p, hsa-miR-211-5p, and hsa-miR-526b-3p regulate SMOC1; hsa-miR-92b-3p regulates DKK3 and has-miR-25-3p regulates TMEM184B; has-miR-15b-5p and has-miR-503-5p regulate C1orf21. The starbase database was used to predict interaction relationships between miRNAs and lncRNAs, and the intersection of the predicted lncRNAs and DElncRNAs in modules identified above was established to search for lncRNAs which may play a potential role in the pathophysiological process of CAD. The lncRNA OIP5-AS1 was identified to regulate hsa-miR-204-5p, hsa-miR-211-5p, hsa-miR-92b-3p, and hsa-miR-25-3p. The ceRNA regulatory network was then visualized using the Cytoscape software (v3.8.1) (Table 2; Figure 5).
Validation Using qRT-PCR
The lncRNAs, miRNAs, and mRNAs identified above were verified utilizing qRT-PCR. The results demonstrated that the expression levels of OIP5-AS1, DKK3, SMOC1, and TMEM184B were significantly higher in patients with CAD, while miR-204-5p, miR-211-5p, miR-92b-3p, and miR-25-3p levels were significantly lower, which were consistent with our bioinformatic analysis (Figure 6).
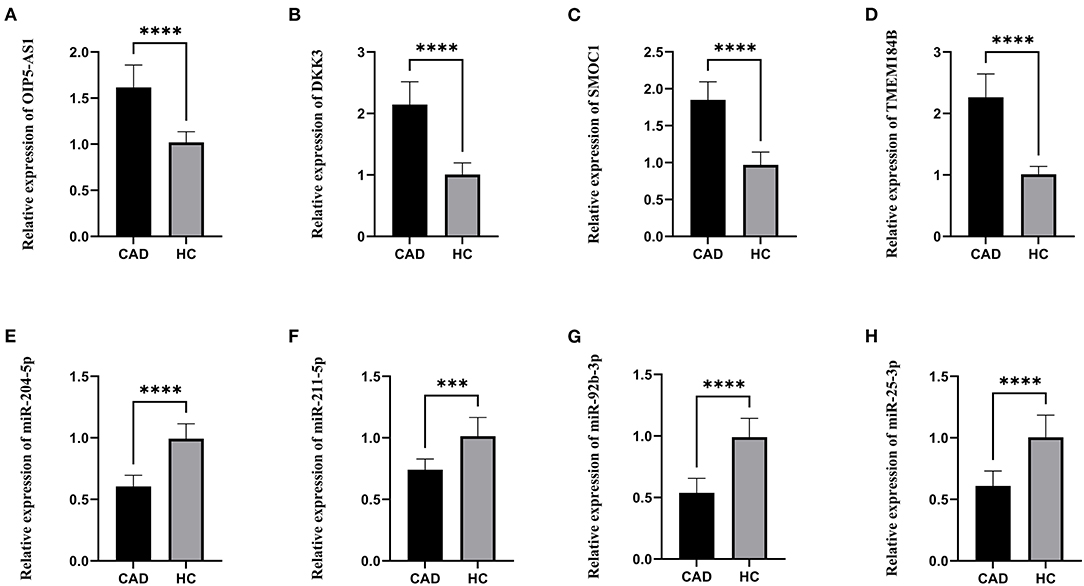
Figure 6. The relative expression levels. (A) The relative expression level of OIP5-AS1. (B) The relative expression level of DKK3. (C) The relative expression level of SMOC1. (D) The relative expression level of TMEM184B. (E) The relative expression level of miR-204-5p. (F) The relative expression level of miR-211-5p. (G) The relative expression level of miR-92b-3p. (H) The relative expression level of miR-25-3p. CAD, Coronary artery disease; HC, Healthy control. ***p < 0.001, ****p < 0.0001.
Discussion
In this study, we downloaded the GSE113079 dataset from the GEO database for bioinformatics analysis. The limma package in the R language was applied to identify the DEGs and DELncRNAs between patients with CAD and healthy controls. Then WGCNA was performed to cluster DEGs and DELncRNAs into different modules and calculate the relationships between modules and CAD. The brown module of DEGs was identified as the key module with the highest correlation coefficient. 188 hub genes in the brown module were selected for functional enrichment analysis. The significant enriched GOBPs included regulation of ion transmembrane transport, O-glycan processing, telencephalon cell migration, positive regulation of glucose import, and renal water homeostasis. The results of Metascape showed that these hub genes were mainly enriched in the matrisome-associated pathway, regulation of cellular component size, and cell-cell recognition. Then three online databases, including TargetScan, miRDB, and miRTarBase, and the online tool miRWalk were used to predict the potential target miRNAs. Nine miRNAs which may regulate hub genes were identified, including hsa-miR-195-3p, hsa-miR-188-5p, hsa-miR-204-5p, hsa-miR-211-5p, hsa-miR-526b-3p, hsa-miR-92b-3p, hsa-miR-25-3p, has-miR-15b-5p and has-miR-503-5p. The Starbase database was used to predict the relationships between seven miRNAs and DElncRNAs in modules selected above to identify potential interactions. The lncRNA OIP5-AS1 was screened out to regulate hsa-miR-204-5p, hsa-miR-211-5p, hsa-miR-92b-3p, and hsa-miR-25-3p. In all, we identified three novel ceRNA networks, including OIP5-AS1 - miR-204-5p/miR-211-5p - SMOC1, OIP5-AS1 - miR-92b-3p – DKK3, and OIP5-AS1 - miR-25-3p - TMEM184B, which have not been studied in CAD before. In the previous study conducted by He et al. (10), they also utilized the GSE113079 dataset to identify the DEGs and DElncRNAs in CAD. However, we applied WGCNA, which can explore the relationships between gene modules and the clinical phenotypes and make results more reliable, to further analyze DEGs and DElncRNAs in the present study and identified the different regulatory nodes. Moreover, He et al. only verified five miRNAs in ceRNA networks, whereas we verified all lncRNAs, miRNAs, and mRNAs in the ceRNA networks using our clinical samples to make our results more credible and worthy of further study in clinical application.
OIP5-AS1 is a long non-coding RNA located on human chromosome 15q15.1, which is involved in regulating cell proliferation (11). Some studies have shown that OIP5-AS1 is related to the pathophysiological process of atherosclerosis. A previous study demonstrated that OIP5-AS1 contributed to the progression of atherosclerosis by targeting miR-26a-5p, and OIP5-AS1 knockdown could promote cell proliferation and reduce apoptosis and inflammatory response (12). A recent integrated analysis identified six key lncRNAs, including OIP5-AS1, whose expression pattern was highly correlated with the disease stage of atherogenesis (13). Another study showed that OIP5-AS1 promoted oxidative low-density lipoprotein induced endothelial cell injury, which may be involved in the pathological process of atherogenesis (14). Moreover, OIP5-AS1 could activate the SIRT1/AMPK/PGC1α pathway by sponging miR-29a to attenuate myocardial ischemia/reperfusion injury (15). OIP5-AS1 is also considered as a carcinoma-related lncRNA in many types of cancer. It was proved to be overexpressed in lung cancer (16, 17), breast cancer (18), osteosarcoma (19, 20), and hepatoblastoma (21), which were related to later cancer stages and metastasis. However, in multiple myeloma and radioresistant colorectal cancer, OIP5-AS1 was downregulated and played an important role in anti-tumor effects (22). In addition, current evidence suggested that OIP5-AS1 was related to osteoarthritis (23), rheumatoid arthritis (24), primary open angle glaucoma (25), and diabetes (26, 27).
The microRNA miR-204-5p and miR-211-5p were predicted to regulate SMOC1. Down-regulation of miR-204-5p was proved to attenuate endothelial cell dysfunction, which was associated with atherogenesis (28, 29). Another study demonstrated that the expression level of miR-204-5p was significantly lower in atherosclerotic plaque tissues and blood samples than in healthy controls. Further studies indicated that miR-204-5p played a crucial role in the growth and migration of human vascular smooth muscle cells by targeting MMP-9 (30). Moreover, mir-204-5p was also involved in the pathophysiological process of aortic valve calcification, which shared many common characteristics of atherogenesis (31, 32). The microRNA miR-211-5p could inhibit cortical neuron differentiation and survival and strengthen oxidative stress in Alzheimer's disease (33, 34). In osteoarthritis, miR-211-5p contributed to chondrocyte differentiation by suppressing Fibulin-4 expression (35, 36). Several previous studies showed that mir-211-5p was also associated with many types of cancer, including renal cancer, hepatocellular carcinoma, breast cancer, and malignant melanoma (37–43). SMOC1 is a protein-coding gene that may play an important role in ocular development (44–47). Recent studies demonstrated that SMOC1 was also associated with Alzheimer's disease (48–50). However, the role of miR-211-5p and SMOC1 in cardiovascular diseases has rarely been studied.
MiR-92b-3p-DKK3 and miR-25-3p-TMEM184B were also identified in our study. Compared with the peripheral venous circulation, the level of expression was lower for miR-92b-3p in the coronary sinus of patients with heart failure (51). The expression level of miR-92b-3p was lower under the hypoxic condition, and it can inhibit proliferation and cell cycle progression in pulmonary arterial hypertension (52). Previous research indicated that miR-92b-3p played a crucial role in vascular smooth muscle cell proliferation by hypoxia (53). Another study showed that it could inhibit cardiomyocyte hypertrophy by targeting HAND2 (54). DKK3 is a member of the Dickkopf family, which is decreased in a variety of cancers serving as a tumor suppressor gene (55). In ApoE-deficient mice, the expression of DKK3 was involved in the pathogenesis of atherosclerosis via the Wnt/β-catenin pathway (56). In a prospective population-based study, the expression of plasma DKK3 was inversely related to the 5-year progression of carotid atherosclerosis (57). Serum DKK3 level was also inversely associated with coronary stenosis in a Chinese cohort (58). Another study demonstrated that DKK3 might have a therapeutic effect in reducing intraplaque hemorrhage related to atherosclerotic plaque phenotype (59). These pieces of evidence indicated that DKK3 might play an important role in CAD. The miR-25-3p inhibited coronary vascular endothelial cell inflammation through the NF-kappaB pathway in ApoE−/− mice (60). It was reported that miR-25-3p could promote endothelial cell angiogenesis in aging mice (61). TMEM184B is a protein-coding gene that may activate the MAPK signaling pathway. The role of TMEM184B in cardiovascular diseases has never been reported, which is worth of further research.
Previous WGCNA studies in CAD all focused on the expression of mRNA. To the best of our knowledge, it is the first time that WGCNA was used to analyze the expression of lncRNA and mRNA between patients with CAD and healthy controls and to construct the ceRNA regulatory networks. Nevertheless, there are some limitations to our study. First, the data we acquired was from the public database, lacking clinical trait data. Second, although we identified three novel ceRNA networks in this study, further mechanistic studies should be conducted for a better understanding of the pathological process in CAD.
Conclusion
In this study, we identified three novel ceRNA regulatory networks, including OIP5-AS1-miR-204-5p/miR-211-5p-SMOC1, OIP5-AS1-miR-92b-3p-DKK3, and OIP5-AS1-miR-25-3p-TMEM184B, using integrated bioinformatics analysis, which were worthy of further study. Our research might provide a novel insight into ceRNA mechanisms in CAD progression.
Data Availability Statement
The dataset presented in this study can be found at https://www.ncbi.nlm.nih.gov/geo/query/acc.cgi?acc=GSE113079.
Ethics Statement
The studies involving human participants were reviewed and approved by Medical Ethics Committee of Shanghai Tenth People's Hospital, Tongji University. The patients/participants provided their written informed consent to participate in this study.
Author Contributions
All authors listed have made a substantial, direct and intellectual contribution to the work, reviewed the final manuscript, and approved it for publication.
Funding
This study was supported by the General Program of the National Natural Science Foundation of China (No. 81770408).
Conflict of Interest
The authors declare that the research was conducted in the absence of any commercial or financial relationships that could be construed as a potential conflict of interest.
The reviewer JY declared a shared affiliation, with no collaboration, with the authors to the handling editor at the time of review.
Publisher's Note
All claims expressed in this article are solely those of the authors and do not necessarily represent those of their affiliated organizations, or those of the publisher, the editors and the reviewers. Any product that may be evaluated in this article, or claim that may be made by its manufacturer, is not guaranteed or endorsed by the publisher.
Acknowledgments
We thanked Professor Ban Liu, Dr. Qi Wu, and Dr. Zhengyang Hu for their valuable advice.
Supplementary Material
The Supplementary Material for this article can be found online at: https://www.frontiersin.org/articles/10.3389/fcvm.2021.647953/full#supplementary-material
References
1. Roth GA, Johnson C, Abajobir A, Abd-Allah F, Abera SF, Abyu G, et al. Global, regional, and national burden of cardiovascular diseases for 10 causes, 1990 to 2015. J Am Coll Cardiol. (2017) 70:1–25. doi: 10.1016/j.jacc.2017.04.052
2. Ohira T, Iso H. Cardiovascular disease epidemiology in Asia: an overview. Circ J. (2013) 77:1646–52. doi: 10.1253/circj.cj-13-0702
3. Musunuru K, Kathiresan S. Genetics of common, complex coronary artery disease. Cell. (2019) 177:132–45. doi: 10.1016/j.cell.2019.02.015
4. Mahmood SS, Levy D, Vasan RS, Wang TJ. The Framingham Heart Study and the epidemiology of cardiovascular disease: a historical perspective. Lancet. (2014) 383:999–1008. doi: 10.1016/S0140-6736(13)61752-3
5. McCullough PA. Coronary artery disease. Clin J Am Soc Nephrol. (2007) 2:611–6. doi: 10.2215/CJN.03871106
6. Zhong W, Deng Q, Deng X, Zhong Z, Hou J. Long non-coding RNA expression profiles in peripheral blood mononuclear cells of patients with coronary artery disease. J Thorac Dis. (2020) 12:6813–25. doi: 10.21037/jtd-20-3105
7. Meng Q, Pu L, Luo X, Wang B, Li F, Liu B. Regulatory roles of related long non-coding RNAs in the process of atherosclerosis. Front Physiol. (2020) 11:564604. doi: 10.3389/fphys.2020.564604
8. Salmena L, Poliseno L, Tay Y, Kats L, Pandolfi PPA. ceRNA hypothesis: the Rosetta Stone of a hidden RNA language? Cell. (2011) 146:353–8. doi: 10.1016/j.cell.2011.07.014
9. Qi X, Zhang DH, Wu N, Xiao JH, Wang X, Ma W. ceRNA in cancer: possible functions and clinical implications. J Med Genet. (2015) 52:710–8. doi: 10.1136/jmedgenet-2015-103334
10. He J, Li X, Zhang Y, Zhang Q, Li L. Comprehensive analysis of ceRNA regulation network involved in the development of coronary artery disease. Biomed Res Int. (2021) 2021:6658115. doi: 10.1155/2021/6658115
11. Naemura M, Kuroki M, Tsunoda T, Arikawa N, Sawata Y, Shirasawa S, et al. The Long noncoding RNA OIP5-AS1 is involved in the regulation of cell proliferation. Anticancer Res. (2018) 38:77–81. doi: 10.21873/anticanres.12194
12. Ren M, Wang T, Han Z, Fu P, Liu Z, Ouyang C. Long noncoding RNA OIP5-AS1 contributes to the progression of atherosclerosis by targeting miR-26a-5p through the AKT/NF-kappaB pathway. J Cardiovasc Pharmacol. (2020) 76:635–44. doi: 10.1097/FJC.0000000000000889
13. Qian C, Xia M, Yang X, Chen P, Ye Q. Long noncoding RNAs in the progression of atherosclerosis: an integrated analysis based on competing endogenous RNA theory. DNA Cell Biol. (2020) 40:283–92. doi: 10.1089/dna.2020.6106
14. Zheng Z, Zhang G, Liang X, Li T. LncRNA OIP5-AS1 facilitates ox-LDL-induced endothelial cell injury through the miR-98-5p/HMGB1 axis. Mol Cell Biochem. (2020) 476:443–55. doi: 10.1007/s11010-020-03921-5
15. Niu X, Pu S, Ling C, Xu J, Wang J, Sun S, et al. lncRNA Oip5-as1 attenuates myocardial ischaemia/reperfusion injury by sponging miR-29a to activate the SIRT1/AMPK/PGC1alpha pathway. Cell Prolif. (2020) 53:e12818. doi: 10.1111/cpr.12818
16. Wang M, Sun X, Yang Y, Jiao W. Long non-coding RNA OIP5-AS1 promotes proliferation of lung cancer cells and leads to poor prognosis by targeting miR-378a-3p. Thorac Cancer. (2018) 9:939–49. doi: 10.1111/1759-7714.12767
17. Deng J, Deng H, Liu C, Liang Y, Wang S. Long non-coding RNA OIP5-AS1 functions as an oncogene in lung adenocarcinoma through targeting miR-448/Bcl-2. Biomed Pharmacother. (2018) 98:102–10. doi: 10.1016/j.biopha.2017.12.031
18. Zeng H, Wang J, Chen T, Zhang K, Chen J, Wang L, et al. Downregulation of long non-coding RNA Opa interacting protein 5-antisense RNA 1 inhibits breast cancer progression by targeting sex-determining region Y-box 2 by microRNA-129-5p upregulation. Cancer Sci. (2019) 110:289–302. doi: 10.1111/cas.13879
19. Kun-Peng Z, Chun-Lin Z, Xiao-Long M, Lei Z. Fibronectin-1 modulated by the long noncoding RNA OIP5-AS1/miR-200b-3p axis contributes to doxorubicin resistance of osteosarcoma cells. J Cell Physiol. (2019) 234:6927–39. doi: 10.1002/jcp.27435
20. Song L, Zhou Z, Gan Y, Li P, Xu Y, Zhang Z, et al. Long noncoding RNA OIP5-AS1 causes cisplatin resistance in osteosarcoma through inducing the LPAATbeta/PI3K/AKT/mTOR signaling pathway by sponging the miR-340-5p. J Cell Biochem. (2019) 120:9656–66. doi: 10.1002/jcb.28244
21. Zhang Z, Liu F, Yang F, Liu Y. Kockdown of OIP5-AS1 expression inhibits proliferation, metastasis and EMT progress in hepatoblastoma cells through up-regulating miR-186a-5p and down-regulating ZEB1. Biomed Pharmacother. (2018) 101:14–23. doi: 10.1016/j.biopha.2018.02.026
22. Li Y, Han X, Feng H, Han J. Long noncoding RNA OIP5-AS1 in cancer. Clin Chim Acta. (2019) 499:75–80. doi: 10.1016/j.cca.2019.08.031
23. Zhi L, Zhao J, Zhao H, Qing Z, Liu H, Ma J. Downregulation of LncRNA OIP5-AS1 induced by IL-1beta aggravates osteoarthritis via regulating miR-29b-3p/PGRN. Cartilage. (2020):940077951. doi: 10.1177/1947603519900801
24. Qing P, Liu Y. Inhibitory role of long non-coding RNA OIP5-AS1 in rheumatoid arthritis progression through the microRNA-448-paraoxonase 1-toll-like receptor 3-nuclear factor kappaB axis. Exp Physiol. (2020) 105:1708–19. doi: 10.1113/EP088608
25. Zhou M, Lu B, Tan W, Fu M. Identification of lncRNA-miRNA-mRNA regulatory network associated with primary open angle glaucoma. BMC Ophthalmol. (2020) 20:104. doi: 10.1186/s12886-020-01365-5
26. Fu JX, Sun GQ, Wang HL, Jiang HX. LncRNA OIP5-AS1 induces epithelial-to-mesenchymal transition and renal fibrosis in diabetic nephropathy via binding to miR-30c-5p. J Biol Regul Homeost Agents. (2020) 34:961–8. doi: 10.23812/20-199-A-68
27. Xie W, Wu D, Ren Y, Jiang Y, Zhang H, Yang S, et al. OIP5-AS1 attenuates microangiopathy in diabetic mouse by regulating miR-200b/ACE2. World Neurosurg. (2020) 139:e52–60. doi: 10.1016/j.wneu.2020.03.063
28. Wang Z, Zhang M, Wang Z, Guo Z, Wang Z, Chen Q. Cyanidin-3-O-glucoside attenuates endothelial cell dysfunction by modulating miR-204-5p/SIRT1-mediated inflammation and apoptosis. Biofactors. (2020) 46:803–12. doi: 10.1002/biof.1660
29. Lu G, Tian P, Zhu Y, Zuo X, Li X. LncRNA XIST knockdown ameliorates oxidative low-density lipoprotein-induced endothelial cells injury by targeting miR-204-5p/TLR4. J Biosci. (2020) 45:52. doi: 10.1007/s12038-020-0022-0
30. Wang N, Yuan Y, Sun S, Liu G. microRNA-204-5p participates in atherosclerosis via targeting MMP-9. Open Med (Wars). (2020) 15:231–9. doi: 10.1515/med-2020-0034
31. Wang Y, Han D, Zhou T, Zhang J, Liu C, Cao F, et al. Melatonin ameliorates aortic valve calcification via the regulation of circular RNA CircRIC3/miR-204-5p/DPP4 signaling in valvular interstitial cells. J Pineal Res. (2020) 69:e12666. doi: 10.1111/jpi.12666
32. Yu C, Li L, Xie F, Guo S, Liu F, Dong N, et al. LncRNA TUG1 sponges miR-204-5p to promote osteoblast differentiation through upregulating Runx2 in aortic valve calcification. Cardiovasc Res. (2018) 114:168–79. doi: 10.1093/cvr/cvx180
33. Zhu R, Qi X, Liu C, Wang D, Li L, Liu X, et al. The silent information regulator 1 pathway attenuates ROS-induced oxidative stress in Alzheimer's disease. J Integr Neurosci. (2020) 19:321–32. doi: 10.31083/j.jin.2020.02.1151
34. Fan C, Wu Q, Ye X, Luo H, Yan D, Xiong Y, et al. Role of miR-211 in neuronal differentiation and viability: implications to pathogenesis of Alzheimer's disease. Front Aging Neurosci. (2016) 8:166. doi: 10.3389/fnagi.2016.00166
35. Prasadam I, Batra J, Perry S, Gu W, Crawford R, Xiao Y. Systematic identification, characterization and target gene analysis of microRNAs involved in osteoarthritis subchondral bone pathogenesis. Calcif Tissue Int. (2016) 99:43–55. doi: 10.1007/s00223-016-0125-7
36. Liu H, Luo J. miR-211-5p contributes to chondrocyte differentiation by suppressing Fibulin-4 expression to play a role in osteoarthritis. J Biochem. (2019) 166:495–502. doi: 10.1093/jb/mvz065
37. Wang K, Jin W, Jin P, Fei X, Wang X, Chen X. miR-211-5p suppresses metastatic behavior by targeting SNAI1 in renal cancer. Mol Cancer Res. (2017) 15:448–56. doi: 10.1158/1541-7786.MCR-16-0288
38. Jiang G, Wen L, Deng W, Jian Z, Zheng H. Regulatory role of miR-211-5p in hepatocellular carcinoma metastasis by targeting ZEB2. Biomed Pharmacother. (2017) 90:806–12. doi: 10.1016/j.biopha.2017.03.081
39. Chen LL, Zhang ZJ, Yi ZB, Li JJ. MicroRNA-211-5p suppresses tumour cell proliferation, invasion, migration and metastasis in triple-negative breast cancer by directly targeting SETBP1. Br J Cancer. (2017) 117:78–88. doi: 10.1038/bjc.2017.150
40. Lunavat TR, Cheng L, Einarsdottir BO, Olofsson BR, Veppil MS, Sharples RA, et al. BRAF(V600) inhibition alters the microRNA cargo in the vesicular secretome of malignant melanoma cells. Proc Natl Acad Sci U S A. (2017) 114:E5930–9. doi: 10.1073/pnas.1705206114
41. Diaz-Martinez M, Benito-Jardon L, Alonso L, Koetz-Ploch L, Hernando E, Teixido J. miR-204-5p and miR-211-5p contribute to BRAF inhibitor resistance in melanoma. Cancer Res. (2018) 78:1017–30. doi: 10.1158/0008-5472.CAN-17-1318
42. Qin X, Zhang J, Lin Y, Sun XM, Zhang JN, Cheng ZQ. Identification of MiR-211-5p as a tumor suppressor by targeting ACSL4 in hepatocellular carcinoma. J Transl Med. (2020) 18:326. doi: 10.1186/s12967-020-02494-7
43. De Martino E, Brunetti D, Canzonieri V, Conforti C, Eisendle K, Mazzoleni G, et al. The Association of residential altitude on the molecular profile and survival of melanoma: results of an interreg study. Cancers (Basel). (2020) 12:2796. doi: 10.3390/cancers12102796
44. Okada I, Hamanoue H, Terada K, Tohma T, Megarbane A, Chouery E, et al. SMOC1 is essential for ocular and limb development in humans and mice. Am J Hum Genet. (2011) 88:30–41. doi: 10.1016/j.ajhg.2010.11.012
45. Abouzeid H, Boisset G, Favez T, Youssef M, Marzouk I, Shakankiry N, et al. Mutations in the SPARC-related modular calcium-binding protein 1 gene, SMOC1, cause waardenburg anophthalmia syndrome. Am J Hum Genet. (2011) 88:92–8. doi: 10.1016/j.ajhg.2010.12.002
46. Slavotinek AM. Eye development genes and known syndromes. Mol Genet Metab. (2011) 104:448–56. doi: 10.1016/j.ymgme.2011.09.029
47. Slavotinek A. Genetics of anophthalmia and microphthalmia. Part 2: Syndromes associated with anophthalmia-microphthalmia. Hum Genet. (2019) 138:831–46. doi: 10.1007/s00439-018-1949-1
48. Wang H, Dey KK, Chen PC, Li Y, Niu M, Cho JH, et al. Integrated analysis of ultra-deep proteomes in cortex, cerebrospinal fluid and serum reveals a mitochondrial signature in Alzheimer's disease. Mol Neurodegener. (2020) 15:43. doi: 10.1186/s13024-020-00384-6
49. Sathe G, Albert M, Darrow J, Saito A, Troncoso J, Pandey A, et al. Quantitative proteomic analysis of the frontal cortex in Alzheimer's disease. J Neurochem. (2020) 156:988–1002. doi: 10.1111/jnc.15116
50. Bai B, Wang X, Li Y, Chen PC, Yu K, Dey KK, et al. Deep Multilayer Brain Proteomics Identifies Molecular Networks in Alzheimer's Disease Progression. Neuron. (2020) 105:975–91. doi: 10.1016/j.neuron.2019.12.015
51. Ben-Zvi I, Volinsky N, Grosman-Rimon L, Haviv I, Rozen G, Andria N, et al. Cardiac-peripheral transvenous gradients of microRNA expression in systolic heart failure patients. ESC Heart Fail. (2020) 7:835–43. doi: 10.1002/ehf2.12597
52. Hao X, Ma C, Chen S, Dang J, Cheng X, Zhu D. Reverse the down regulation of miR-92b-3p by hypoxia can suppress the proliferation of pulmonary artery smooth muscle cells by targeting USP28. Biochem Biophys Res Commun. (2018) 503:3064–77. doi: 10.1016/j.bbrc.2018.08.095
53. Lee J, Heo J, Kang H. miR-92b-3p-TSC1 axis is critical for mTOR signaling-mediated vascular smooth muscle cell proliferation induced by hypoxia. Cell Death Differ. (2019) 26:1782–95. doi: 10.1038/s41418-018-0243-z
54. Yu XJ, Huang YQ, Shan ZX, Zhu JN, Hu ZQ, Huang L, et al. MicroRNA-92b-3p suppresses angiotensin II-induced cardiomyocyte hypertrophy via targeting HAND2. Life Sci. (2019) 232:116635. doi: 10.1016/j.lfs.2019.116635
55. Lee EJ, Nguyen Q, Lee M. Dickkopf-3 in human malignant tumours: a clinical viewpoint. Anticancer Res. (2020) 40:5969–79. doi: 10.21873/anticanres.14617
56. Cheng WL, Yang Y, Zhang XJ, Guo J, Gong J, Gong FH, et al. Dickkopf-3 ablation attenuates the development of atherosclerosis in ApoE-deficient mice. J Am Heart Assoc. (2017) 6:e004690. doi: 10.1161/JAHA.116.004690
57. Yu B, Kiechl S, Qi D, Wang X, Song Y, Weger S, et al. A cytokine-like protein dickkopf-related protein 3 is atheroprotective. Circulation. (2017) 136:1022–36. doi: 10.1161/CIRCULATIONAHA.117.027690
58. Wang L, Liu S, Niu J, Zhao Z, Xu M, Lu J, et al. Serum dickkopf-3 level is inversely associated with significant coronary stenosis in an asymptomatic Chinese cohort. Int Heart J. (2020) 61:1107–13. doi: 10.1536/ihj.20-094
59. Karamariti E, Zhai C, Yu B, Qiao L, Wang Z, Potter C, et al. DKK3 (Dickkopf 3) alters atherosclerotic plaque phenotype involving vascular progenitor and fibroblast differentiation into smooth muscle cells. Arterioscler Thromb Vasc Biol. (2018) 38:425–37. doi: 10.1161/ATVBAHA.117.310079
60. Yao Y, Sun W, Sun Q, Jing B, Liu S, Liu X, et al. Platelet-derived exosomal MicroRNA-25-3p inhibits coronary vascular endothelial cell inflammation through Adam10 via the NF-kappaB signaling pathway in ApoE(-/-) mice. Front Immunol. (2019) 10:2205. doi: 10.3389/fimmu.2019.02205
Keywords: coronary artery disease, non-coding RNA, ceRNA, bioinformatics, WGCNA
Citation: Ji Y, Yan T, Zhu S, Wu R, Zhu M, Zhang Y, Guo C and Yao K (2021) The Integrative Analysis of Competitive Endogenous RNA Regulatory Networks in Coronary Artery Disease. Front. Cardiovasc. Med. 8:647953. doi: 10.3389/fcvm.2021.647953
Received: 30 December 2020; Accepted: 25 August 2021;
Published: 22 September 2021.
Edited by:
En-Zhi Jia, Nanjing Medical University, ChinaReviewed by:
Xiao Huang, Second Affiliated Hospital of Nanchang University, ChinaJie Yuan, Fudan University, China
Cláudio Lera Orsatti, University of Western São Paulo, Brazil
Copyright © 2021 Ji, Yan, Zhu, Wu, Zhu, Zhang, Guo and Yao. This is an open-access article distributed under the terms of the Creative Commons Attribution License (CC BY). The use, distribution or reproduction in other forums is permitted, provided the original author(s) and the copyright owner(s) are credited and that the original publication in this journal is cited, in accordance with accepted academic practice. No use, distribution or reproduction is permitted which does not comply with these terms.
*Correspondence: Yangyang Zhang, zhangyangyang_wy@vip.sina.com; Changfa Guo, guo.changfa@zs-hospital.sh.cn; Kang Yao, yao.kang@zs-hospital.sh.cn
†These authors have contributed equally to this work