- 1Cardiovascular Division, Department of Medicine, Beth Israel Deaconess Medical Center and Harvard Medical School, Boston, MA, United States
- 2Division of Cardiology, Hypertrophic Cardiomyopathy Center, Tufts Medical Center, Boston, MA, United States
- 3Department of Radiology, Beth Israel Deaconess Medical Center and Harvard Medical School, Boston, MA, United States
Background: Development of advanced heart failure (HF) symptoms is the most common adverse pathway in hypertrophic cardiomyopathy (HCM) patients. Currently, there is a limited ability to identify HCM patients at risk of HF.
Objectives: In this study, we present a machine learning (ML)-based model to identify individual HCM patients who are at high risk of developing advanced HF symptoms.
Methods: From a consecutive cohort of HCM patients evaluated at the Tufts HCM Institute from 2001 to 2018, we extracted a set of 64 potential risk factors measured at baseline. Only patients with New York Heart Association (NYHA) functional class I/II and LV ejection fraction (LVEF) by echocardiography >35% were included. The study cohort (n = 1,427 patients) was split into three disjoint subsets: development (50%), model selection (10%), and independent validation (40%). The least absolute shrinkage and selection operator was used to select the most influential clinical variables. An ensemble of ML classifiers, including logistic regression, was used to identify patients with high risk of developing a HF outcome. Study outcomes were defined as progression to NYHA class III/IV, drop in LVEF below 35%, septal reduction procedure, and/or heart transplantation.
Results: During a mean follow-up of 4.7 ± 3.7 years, advanced HF occurred in 283 (20% out of 1,427) patients. The model features included patients' sex, NYHA class (I or II), HCM type (i.e., obstructive or not), LV wall thickness, LVEF, presence of HF symptoms (e.g., dyspnea, presyncope), comorbidities (atrial fibrillation, hypertension, mitral regurgitation, and systolic anterior motion), and type of cardiac medications. The developed risk stratification model showed strong differentiation power to identify patients at advanced HF risk in the testing dataset (c-statistics = 0.81; 95% confidence interval [CI]: 0.76, 0.86). The model allowed correct identification of high-risk patients with accuracy 74% (CI: 0.70, 0.78), sensitivity 80% (CI: 0.77, 0.83), and specificity 72% (CI: 0.68, 0.76). The model performance was comparable among different sex and age groups.
Conclusions: A 5-year risk prediction of progressive HF in HCM patients can be accurately estimated using ML analysis of patients' clinical and imaging parameters. A set of 17 clinical and imaging variables were identified as the most important predictors of progressive HF in HCM.
Summary
Heart failure (HF) progression is the most common adverse disease consequence in hypertrophic cardiomyopathy. However, identification of at-risk patients is currently limited and predominantly relies on identifying dynamic left ventricular outflow tract obstruction, which has limited specificity and does not allow for tailored treatment planning. A few recent studies investigated the prognostic value of individual HF risk factors (e.g., left ventricular function or longitudinal strain), each with limited sensitivity and specificity. To our knowledge, no study has reported a risk stratification model for progressive HF in HCM. In this study, we present a prediction model to identify individual HCM patients who are at high risk of developing advanced HF symptoms. Our model allows personalization of individual patients' clinical course and enables the potential development of future studies investigating earlier treatment in high-risk patients to determine if this can improve patient outcomes.
Introduction
Hypertrophic cardiomyopathy (HCM) is the most common genetic heart disease with sudden cardiac death as the most visible and devastating consequence (1–4). Much attention has been placed on the identification of HCM patients at risk for sudden death, allowing for a mature sudden death risk stratification strategy that identifies the vast majority of at-risk individuals (3, 5). However, the most common adverse consequence of HCM is the development of advanced heart failure (HF) symptoms, occurring in 35–50% of patients and leading to substantial function disability and reduced quality of life (6–8).
The mechanism of exertional disability in HCM is predominantly secondary to dynamic left ventricular (LV) outflow tract (LVOT) obstruction occurring either at rest or with provocation, with these patients at higher risk for progressive symptoms (9–11), while nonobstructive patients are at substantially lower risk for symptom progression. However, risk stratification of patients based on the LVOT obstruction falls short of specificity needed for accurate disease management and treatment planning. For example, there is limited ability to stratify patients with LVOT obstruction who are at high risk for development of HF, as compared to those who survive to advance ages with no or mild symptoms. In contrast, nonobstructive HCM patients are considered at lower risk for development of advanced HF. However, medical therapy for patients with symptomatic nonobstructive HCM is limited and patients who develop advanced HF symptoms may ultimately require cardiac transplant as the only definitive treatment option (5, 9).
Few recent studies investigated the potential prognostic value of individual imaging and clinical parameters such as LV structural and functional parameters, cardiopulmonary exercise testing parameters, serum biomarkers, and global longitudinal strain (12–14). However, there is still a limited ability to predict HF progression in HCM and there is a need for a HF risk prediction model that allows more comprehensive evaluation of the patients' clinical parameters. Machine learning (ML) algorithms provide a powerful tool for learning complex relationships between the risk predictors and outcomes from a representative sample of the patients. ML-based models have been used to predict cardiovascular events with improved accuracy and generalizability compared to traditional risk predictors (15–19). Several studies showed that further improvement can be achieved by combining a number of ML models in an ensemble utilizing their versatile characteristics (15, 20, 21). In this study, we present an ML-based HF risk prediction model in HCM patients. To avoid arbitrarily selecting a specific ML model, we followed a systematic approach to build an ensemble of models that can learn the association between HF risk and clinical and imaging risk markers. We report the performance metrics of each individual model in the ensemble to illustrate the designing steps rather than providing a rigorous comparison of the different models.
Materials and Methods
Study Population and Outcome
The database of the HCM Institute at Tufts Medical Center (Boston, MA) containing data from 2,732 consecutive patients with HCM from June 2001 to Dec 2018 was interrogated. Data records for 880 patients (32%) with advanced HF symptoms at baseline (defined by New York Heart Association (NYHA) functional class III or IV) (n = 863), heart transplantation (n = 1), or septal reduction procedure (n = 11) or with LV ejection fraction by echocardiography <35% (n = 5) were excluded. Data on the most recent status of HF were obtained up to December 30, 2019, in 1,427 (77% of 1,852) patients by hospital visit or telephone contact with patients, family members, and referring physicians. Study outcomes were defined as progression in HF symptoms from NYHA functional classes I/II to classes III/IV, drop in LV ejection fraction to <35%, having underwent septal reduction procedure, or having had (or added to the waiting list of) heart transplantation during follow-up. The mean ± SD follow-up duration from initial clinical evaluation at the Tufts Medical Center to the earliest of progression to class III/IV date or most recent contact was 4.7 ± 3.7 years. The average time to advanced HF symptoms in our cohort was 2.7 ± 2.6 years. The clinical diagnosis of HCM was based on two-dimensional transthoracic echocardiographic identification of otherwise unexplained hypertrophied non-dilated LV (wall thickness ≥13 mm) (3, 22). Patients had been referred for targeted subspecialty evaluations, including diagnosis, risk stratification, and treatment. Patients with phenocopies of HCM (e.g., Fabry disease, LAMP2 cardiomyopathy, PRKAG2, or amyloidosis) were excluded. This study was approved by the institutional review board at Tufts Medical Center, allowing a retrospective review of medical records and granting a waiver of informed consent in accordance with 45 CFR 46.116(d).
Potential Risk Predictors
The model was built using potential clinical, demographic, and imaging risk markers (n = 64; Supplementary Table 1) measured at the time of initial patient evaluation including (1) baseline demographics (e.g., age and sex); (2) HF risk factors (e.g., symptoms of fatigue, dyspnea, and syncope); (3) imaging data (e.g., echocardiography LV ejection fraction, LA size, and maximum wall thickness); (4) cardiac medications (e.g., beta blocker and calcium channel blocker); and (5) comorbidities (e.g., hypertension, atrial fibrillation, stroke, and implantable cardiac device). A risk factor representing obstructive (or non-obstructive) HCM was defined by a LV outflow tract (LVOT) gradient ≥30 mmHg at rest or with provocation (i.e., exercise or Valsalva maneuver). Nonobstructive HCM was identified by a LVOT gradient <30 mmHg both at rest and with provocation. Categorical variables were replaced by an integer ranging from 0 to the maximum number of categories (as indicated in Supplementary Table 1). Variables with >5% missing data were not included. Missing measurements of the included variables were imputed using the k-nearest neighbor method, with k set to 1 to preserve the original variability in data distribution (23).
For the purpose of developing the HF risk model, the patients were split into three subsets (Figure 1): (1) development subset (713 patients (50%); (2) model-selection subset (142 patients (10%); and (3) independent-validation subset (572 patients (40%). Stratified random sampling was used to split the data such that the ratio of positive to negative HF outcomes was the same in all subsets.
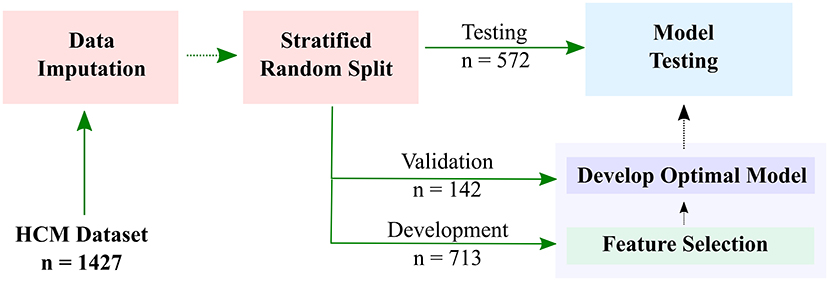
Figure 1. Workflow of developing a machine learning-based model for predicting risk of heart failure (HF) in hypertrophic cardiomyopathy (HCM) patients. Datasets are imputed and split into development (50%), model-selection (10%), and independent-validation (40%) subsets. The development subset is used to select the most important features, and the validation subset is used for model optimization. The final HF stratification model is then used to predict HF risk in the testing subset.
Risk Predictor Selection
The set of most important clinical variables was selected using the least absolute shrinkage and selection operator (LASSO) (24). To determine the optimal number of features, LASSO feature selection was repeated to select the best k features (with k ranging from 1 to 40). For each value of k, a logistic regression model was developed and evaluated using a 10-fold cross-validation scheme. In this scheme, the development dataset is split into 10 disjoint subsets, where nine subsets were used for training the model and one subset is used for model evaluation. The process was repeated 10 times to try all possible 10 different selections of training-evaluation subsets. The average model performance [measured by the area under the curve (AUC) of the receiving operating characteristics (ROC), or c-statistics] over the 10 repetitions was used to determine the optimal number, k, and specify the most important clinical variables.
Model Selection
The development subset was used to train and optimize six different state-of-the-art ML classifiers: logistic regression (LG), random forests (RF), support-vector machines (SVM), gradient boosted decision trees (GBC), adaptive boosted decision trees (ADB), and neural networks (NN). Ten-fold cross-validation was used to determine the optimal model parameters. Each resulting model was then evaluated using the model-selection subset (142 patients) to determine the best model. An ensemble of the three best-performing models was used as the final HF risk stratification model. The outputs of models comprising the ensemble were merged using logistic regression. The final ensemble output was a normalized probability value (i.e., from 0 to 1) representing the patient's risk to develop HF outcome.
Model Testing and Performance Evaluation
The final optimal models were used to predict the HF risk for the patients in the independent validation dataset. The models output a value representing the probability that a patient develops advanced HF symptoms within a 5-year follow up interval. We used AUC (or c-statistics) to estimate the discriminatory power of the model to identify patients at risk of progressive HF. An arbitrary operating point represented by a probability of 50% was used to identify patients at high risk of HF and used to compute the F1 score, sensitivity, specificity, and accuracy of each model. The contribution of each input variable to the model output for each patient (i.e., probability of developing progressive HF) was assessed by the Shapley values (25). Shapley values approximate the impact of removing the variable on the model prediction while taking into account the interactions among all variables. Model development was done using Python-V3.7 (Python Software Foundation, Fredericksburg, VA) and Scikit-learn Ver-0.23.2 (scikit-learn.org) on a PC with Quadro K620 graphics processing unit (Nvidia, Santa Clara, CA). For Shapley value computations, we used the SHapley Additive exPlanations (SHAP) analysis library (26). The final model is available at https://doi.org/10.7910/dvn/ffnlpe for external validation by other researchers.
Statistical Data Analyses
Data are displayed as mean ± SD for continuous variables and as proportions for categorical variables. The Student (two-sample) t-test was used to assess statistical significance for continuous variables and z-test for comparing population proportions. AUC, sensitivity, specificity, and average F1 score were used to evaluate the model performance. Parametric estimation for the variance was used to compute the 95% confidence interval (CI), and a p ≤ 0.05 was considered significant (reported as two-sided). Statistical calculations were performed with the Matlab statistical toolbox (version R2018b, Mathworks, Natick, MA).
Results
The mean age of the patients included in this study (n = 1,427; 69% men) was 52 ± 17 years with a mean follow-up time of 4.7 ± 3.6 years (median 3.7 years). The baseline characteristics of the patient cohort are shown in Table 1. Twenty-three features (of 64) showed a non-zero importance score using LASSO feature selection analysis (Figure 2). The optimal number of important features that maximized HF risk stratification performance (c-statistics) in the development subset was 17 features (Table 1). Four classifiers yielded the highest three AUC scores: LG (0.79), GBC (0.79), NN (0.78), and SVM (0.78) (Table 2). An ensemble of LG-GBC-SVM was used as the final prediction model. The final model showed strong power to differentiate low- from high-risk patients in the testing subset (572 patients) with AUC = 0.81 [95% CI: 0.76–0.86] (Figure 3). The model showed accuracy of 74% [95% CI: 0.70–0.78], sensitivity of 80% [95% CI: 0.77–0.83], and specificity of 72% [95% CI: 0.68–0.76] (Table 3). The model performance metrics for the different age and sex subgroups was comparable and showed overlapped 95% CI, as indicated in Table 3. SHAP analysis showed that obstructive HCM and NYHA functional class II were associated with higher risk compared to non-obstructive HCM and NYHA functional class I (Figure 4). Also, presence of HF symptoms (dyspnea, fatigue, syncope, and presyncope) or abnormal heart function or structure (e.g., reduced LV ejection fraction, increased wall thickness, septal anterior motion, and mitral regurgitation) increased the risk of developing progressive HF. Also, three cardiac medications (Coumadin, beta blockers, and calcium channel blockers) showed an association with increased HF risk while the angiotensin-converting enzyme inhibitor (ACEi) or angiotensin-receptor blocker (ARB) was associated with low HF risk. Additionally, risk of progressive HF was higher in males and patients with history of atrial fibrillation and/or without hypertension (Figure 4).
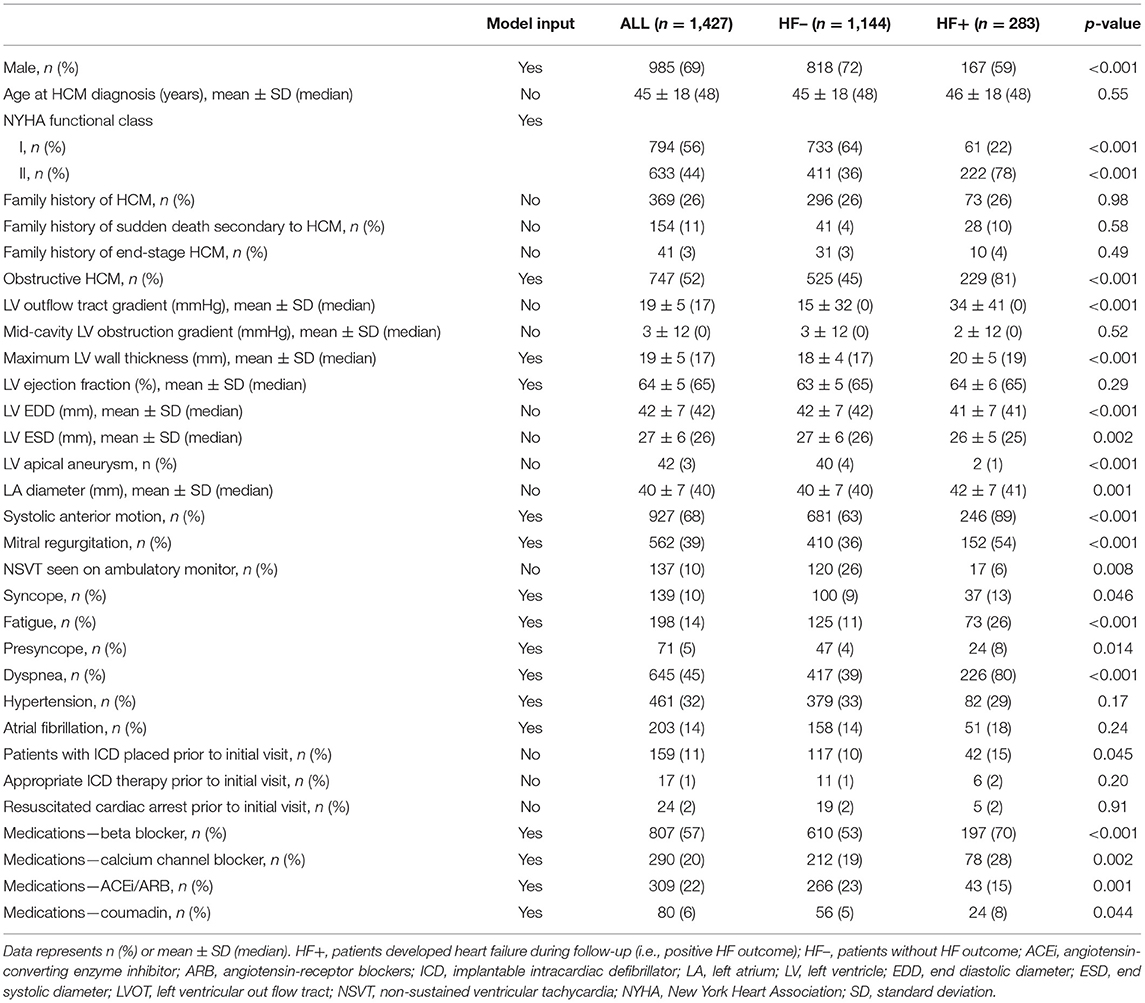
Table 1. Baseline clinical characteristics for the hypertrophic cardiomyopathy (HCM) patients at initial clinical assessment.
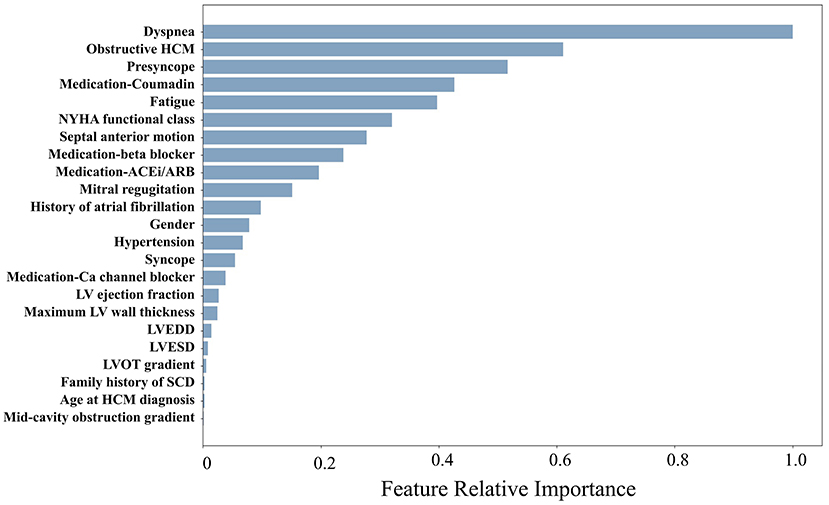
Figure 2. Relative importance scores for the risk factors included in the study (only factors with nonzero scores are displayed).
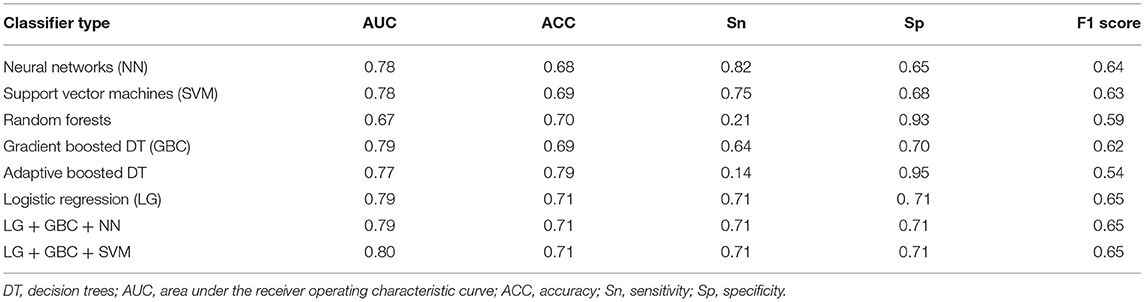
Table 2. Performance evaluation of the different machine learning models using the model-selection dataset (143 patients; 28 positive heart failure outcomes).
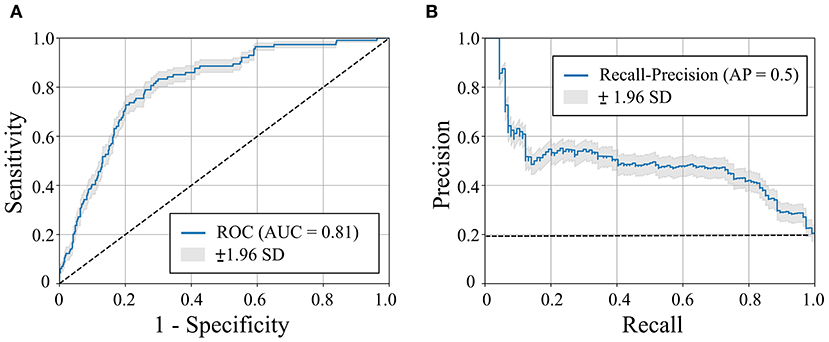
Figure 3. Receiver operating characteristic (ROC) curve (A) and recall-precision curve (B) for the machine learning-based heart failure (HF) risk stratification in hypertrophic cardiomyopathy patients (n = 572). Dashed line represents pure-chance stratification AUC = 0.5 in (A) or precision = ratio of HF outcomes in the dataset (=20%) (B). AUC = area under the curve. AP, average precision; SD, standard deviation.
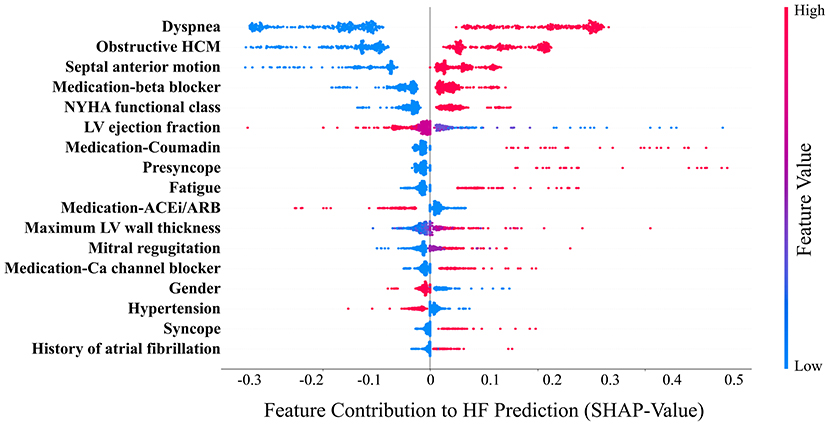
Figure 4. Relative contribution (SHAP-values) of the model variables (n = 17) to heart failure (HF) prediction. Each point in the graph indicates the contribution of the corresponding clinical variable to the HF prediction of one patient. Ca, calcium; ACEi, angiotensin-converting enzyme inhibitor; ARB, angiotensin-receptor blockers. HCM, hypertrophic cardiomyopathy; LV, left ventricle; NYHA, New York Heart Association.
Discussion
We present an ML-based study to develop and test a prediction model for progressive HF in HCM. There has previously been limited ability to predict HF risk in HCM as a number of disease features appear to impact symptom progression limiting accuracy of traditional prediction models. In our study, an ensemble of machine learning classifiers, including logistic regression, is used to accurately predict the risk of progressive HF over an average of a 5-year follow-up period. The most significant variables in our models included clinical and imaging variables that have previously been individually linked to progressive HF in HCM, but with limited accuracy. Thereby, the ability to predict progressive HF symptoms appears to be related to an interaction of these variables. We initially included all 64 measured risk factors to determine if specific symptoms (e.g., dyspnea, fatigue, or chest pain) were predictive of the development of advanced HF over time. This allowed the final model to include risk factors that are not completely independent. For example, both dyspnea and NYHA class were significant factors in the model. While dyspnea is included as part of NYHA class evaluation, notably a number of other factors ultimately play into the determination of NYHA class (e.g., degree of effort leading to dyspnea and degree of fatigue with exertion). In our cohort, 68 patients with dyspnea were in NYHA class I while 56 patients without dyspnea were in NYHA class II. All machine learning techniques studied in this work, except random forests, showed comparable accuracy (77–79%) for predicting the endpoints. An ensemble of the three best models showed a slightly higher accuracy (80%). Although the study endpoints included LV ejection fraction depression and cardiac transplantation, the small number of events during our follow-up period (n = 3 and 1, respectively) does not allow separate prediction of these events. Prediction of these events separate from progression of the NYHA class requires longer follow-up periods and a larger patient cohort to account for the low incidence rate of these events.
Our results demonstrate that the model performance is comparable in male and female patients. Also, there was no statistical significance in performance among the different age groups. However, the model average discriminating power, measured by AUC, was relatively high (≥0.81) in patients within the 20–60-year-old groups compared to the other two groups. This may be explained by the generally high representation of patients in this age range in our dataset (62%). We also note that the limited number of positive events in the youngest age group does not allow reliable prediction of HF, which was indicated by the wide 95% CI.
Progressive and advanced HF development is the most common adverse pathway in HCM. With the availability of mature strategy for identification of patients at risk for sudden death and utilization of ICDs for sudden death prevention, HF has become the most common cause of HCM death. While most HCM patients will have a benign clinical course without HF progression, there has been an inability to identify at-risk patients, leading to uncertainty from treating clinicians as to which patients are in need for more aggressive therapy and closer clinical follow-up. Similarly, there has been uncertainty for patients regarding their disease-related natural history and individual risk. The present model allows for clarification of an individual risk and allows for a more personalized treatment approach regarding both need for closer clinical follow-up and more aggressive treatment. For example, the model can identify individual patients who may develop advanced HF with relatively high sensitivity (80%) and specificity (72%). This can open the opportunity for adopting more aggressive treatment to improve clinical outcomes in higher-risk individuals and closer follow-up. Meanwhile, it can offer a substantial reassurance that low-risk patients are unlikely to need interventional procedures over a 5-year period. However, we note that the presented model is developed based on a 5-year follow-up period and may not be accurate to predict HF beyond 5 years. The lack of established HF stratification models in HCM does not allow benchmarking of our model. However, we note that the stratification power and accuracy of our model are comparable to those reported for established sudden cardiac death risk stratification models (27–29).
While the impact of medical therapy to change the natural history of HCM remains controversial without data to routinely support implementation (9, 30), a more targeted approach to initiation of medical therapy specifically in patients identified at higher risk is deserving of a further study. This is particularly relevant given the ongoing research into novel therapeutic interventions in HCM, including myosin modulators which may prove more powerful treatments to alter HCM phenotype and prevent disease progression (31).
Our study has a number of limitations. First, our HF prediction model is designed to accommodate a typical clinical protocol implemented by a single medical center and is not tested using data acquired using different protocols. Also, given the longitudinal nature of our cohort with patients seen and evaluated over a 15-year period, more novel potential risk markers, such as serum biomarkers or mechanical deformation parameters such as global longitudinal strain (12), are not available but may offer additional dimensions to the model. Additionally, not every patient in this study was followed for the full 5-year term and patients who did not develop HF symptoms during the follow-up period were treated as not having the outcome of interest, which could bias the model.
In conclusion, our machine learning model allowed for accurate identification of HCM patients at risk for HF progression within a 5-year follow-up period. The model is based on 17 significant risk factors including imaging parameters (e.g., LVOT obstruction, septal anterior motion, and LV ejection fraction), cardiac medications (e.g., beta-blockers and coumadin), and physical symptoms of heart failure (e.g., dyspnea and fatigue). This may allow personalization of individual patients' clinical course into clinical practice and closer clinical follow-up in high-risk individuals. In addition, the developed models allow the opportunity for future research on implementation of earlier disease-specific treatment in high-risk patients to determine if this can prevent symptom progression and improve outcomes.
Data Availability Statement
The data analyzed in this study was subjected to the following licenses/restrictions: Participant data used in this study are not publicly available at present. The source code of the machine learning algorithm implementation and the final (trained) model was available at https://doi.org/10.7910/dvn/ffnlpe.
Ethics Statement
The studies involving human participants were reviewed and approved by the institutional review board at Tufts Medical Center, allowing retrospective review of medical records and granting a waiver of informed consent in accordance with 45 CFR 46.116(d). Written informed consent from the participants' legal guardian/next of kin was not required to participate in this study in accordance with the national legislation and the institutional requirements.
Author Contributions
MM and RN: guarantor of integrity of entire study. ER and MM: data acquisition. AF: algorithm implementation. AF and ER: statistical analysis. AF, ER, and RN: literature research. ER, WM, MM, and RN: clinical studies. Underlying data was accessed and verified by AF, ER, and RN. All authors conceptualization and formulation of study design and overall goals, data curation and analysis/interpretation, manuscript drafting, editing, or manuscript revision for important intellectual content, approval of final version of submitted manuscript, and agreement to ensure any questions related to the work are appropriately resolved.
Funding
RN was supported by National Institutes of Health 5R01HL127015, 1R01HL154744, 5R01HL129185, and 1R01HL129157-01A1 (Bethesda, MD, USA); American Heart Association (AHA) 15EIA22710040 (Dallas, TX, USA). AF was supported by the AHA Institute for Precision Cardiovascular Medicine 19AIML34850090.
Conflict of Interest
The authors declare that the research was conducted in the absence of any commercial or financial relationships that could be construed as a potential conflict of interest.
Supplementary Material
The Supplementary Material for this article can be found online at: https://www.frontiersin.org/articles/10.3389/fcvm.2021.647857/full#supplementary-material
Abbreviations
ADB, Adaptive boosted decision trees classifier; AUC, Area under curve of the receiving operator characteristics; CI, 95% Confidence interval; CMR, Cardiovascular magnetic resonance imaging; GBC, Gradient boosted decision trees classifier; HCM, Hypertrophic cardiomyopathy; HF, Heart failure; LA, Left atrium; LG, Logistic regression classifier; LV, Left ventricle; LVOT, Left ventricle outflow tract; ML, Machine learning; NYHA, New York Heart Association; NN, Neural networks; RF, Random forests; SVM, Support-vector machine.
References
1. Maron BJ. Hypertrophic cardiomyopathy: a systematic review. JAMA. (2002) 287:1308–20. doi: 10.1001/jama.287.10.1308
2. Maron BJ, McKenna WJ, Danielson GK, Kappenberger LJ, Kuhn HJ, Seidman CE, et al. American College of Cardiology/European Society of Cardiology Clinical Expert Consensus Document on Hypertrophic Cardiomyopathy: a report of the American College of Cardiology Foundation Task Force on Clinical Expert Consensus Documents and the European Society of Cardiology Committee for Practice Guidelines. J Am Coll Cardiol. (2003) 42:1687–713. doi: 10.1016/S0195-668X(03)00479-2
3. Elliott PM, Poloniecki J, Dickie S, Sharma S, Monserrat L, Varnava A, et al. Sudden death in hypertrophic cardiomyopathy: identification of high risk patients. J Am Coll Cardiol. (2000) 36:2212–8. doi: 10.1016/S0735-1097(00)01003-2
4. Autore C, Bernabò P, Barillà CS, Bruzzi P, Spirito P. The prognostic importance of left ventricular outflow obstruction in hypertrophic cardiomyopathy varies in relation to the severity of symptoms. J Am Coll Cardiol. (2005) 45:1076–80. doi: 10.1016/j.jacc.2004.12.067
5. Maron BJ, Rowin EJ, Casey SA, Maron MS. How hypertrophic cardiomyopathy became a contemporary treatable genetic disease with low mortality: shaped by 50 years of clinical research and practice. JAMA Cardiol. (2016) 1:98–105. doi: 10.1001/jamacardio.2015.0354
6. Maron MS, Appelbaum E, Harrigan CJ, Buros J, Gibson CM, Hanna C, et al. Clinical profile and significance of delayed enhancement in hypertrophic cardiomyopathy. Circ Heart Fail. (2008) 1:184–91. doi: 10.1161/CIRCHEARTFAILURE.108.768119
7. Lu DY, Pozios I, Haileselassie B, Ventoulis I, Liu H, Sorensen LL, et al. Clinical outcomes in patients with nonobstructive, labile, and obstructive hypertrophic cardiomyopathy. J Am Heart Assoc. (2018) 7:e006657. doi: 10.1161/JAHA.117.006657
8. Maron MS, Rowin EJ, Olivotto I, Casey SA, Arretini A, Tomberli B, et al. Contemporary natural history and management of nonobstructive hypertrophic cardiomyopathy. J Am Coll Cardiol. (2016) 67:1399–409. doi: 10.1016/j.jacc.2016.01.023
9. Maron BJ, Rowin EJ, Udelson JE, Maron MS. Clinical spectrum and management of heart failure in hypertrophic cardiomyopathy. JACC: Heart Failure. (2018) 6:353. doi: 10.1016/j.jchf.2017.09.011
10. Maron MS, Olivotto I, Betocchi S, Casey SA, Lesser JR, Losi MA, et al. Effect of left ventricular outflow tract obstruction on clinical outcome in hypertrophic cardiomyopathy. N Engl J Med. (2003) 348:295–303. doi: 10.1056/NEJMoa021332
11. Maron MS, Olivotto I, Zenovich AG, Link MS, Pandian NG, Kuvin JT, et al. Hypertrophic cardiomyopathy is predominantly a disease of left ventricular outflow tract obstruction. Circulation. (2006) 114:2232–9. doi: 10.1161/CIRCULATIONAHA.106.644682
12. Reant P, Mirabel M, Lloyd G, Peyrou J, Ayala J-ML, Dickie S, et al. Global longitudinal strain is associated with heart failure outcomes in hypertrophic cardiomyopathy. Heart. (2016) 102:741–7. doi: 10.1136/heartjnl-2015-308576
13. Mitter SS, Shah SJ, Thomas JD. A test in context: E/A and E/e′ to assess diastolic dysfunction and LV filling pressure. J Am Coll Cardiol. (2017) 69:1451–64. doi: 10.1016/j.jacc.2016.12.037
14. Coats CJ, Gallagher MJ, Foley M, O'Mahony C, Critoph C, Gimeno J, et al. Relation between serum N-terminal pro-brain natriuretic peptide and prognosis in patients with hypertrophic cardiomyopathy. Eur Heart J. (2013) 34:2529–37. doi: 10.1093/eurheartj/eht070
15. Alaa AM, Bolton T, Di Angelantonio E, Rudd JHF, van der Schaar M. Cardiovascular disease risk prediction using automated machine learning: a prospective study of 423,604 UK Biobank participants. PLoS One. (2019) 14:e0213653. doi: 10.1371/journal.pone.0213653
16. Ambale-Venkatesh B, Yang X, Wu CO, Liu K, Hundley WG, McClelland R, et al. Cardiovascular event prediction by machine learning: the multi-ethnic study of atherosclerosis. Circ Res. (2017) 121:1092–101. doi: 10.1161/CIRCRESAHA.117.311312
17. Kakadiaris IA, Vrigkas M, Yen AA, Kuznetsova T, Budoff M, Naghavi M. Machine learning outperforms ACC / AHA CVD risk calculator in MESA. J Am Heart Assoc. (2018) 7:e009476. doi: 10.1161/JAHA.118.009476
18. Weng SF, Reps J, Kai J, Garibaldi JM, Qureshi N. Can machine-learning improve cardiovascular risk prediction using routine clinical data? PLoS One. (2017) 12:e0174944. doi: 10.1371/journal.pone.0174944
19. Kochav SM, Raita Y, Fifer MA, Takayama H, Ginns J, Maurer MS, et al. Predicting the development of adverse cardiac events in patients with hypertrophic cardiomyopathy using machine learning. Int J Cardiol. (2021) 327:117–24. doi: 10.1016/j.ijcard.2020.11.003
20. Narula S, Shameer K, Salem Omar AM, Dudley JT, Sengupta PP. Machine-learning algorithms to automate morphological and functional assessments in 2D echocardiography. J Am Coll Cardiol. (2016) 68:2287–95. doi: 10.1016/j.jacc.2016.08.062
21. Sánchez-Cabo F, Rossello X, Fuster V, Benito F, Manzano Jose P, Silla Juan C, et al. Machine learning improves cardiovascular risk definition for young, asymptomatic individuals. J Am Coll Cardiol. (2020) 76:1674–85. doi: 10.1016/j.jacc.2020.08.017
22. Gersh BJ, Maron BJ, Bonow RO, Dearani JA, Fifer MA, Link MS, et al. 2011 ACCF/AHA guideline for the diagnosis and treatment of hypertrophic cardiomyopathy: a report of the American College of Cardiology Foundation/American Heart Association Task Force on practice guidelines developed in collaboration with the American Association for Thoracic Surgery, American Society of Echocardiography, American Society of Nuclear Cardiology, Heart Failure Society of America, Heart Rhythm Society, Society for Cardiovascular Angiography and Interventions, and Society of Thoracic Surgeons. J Am Coll Cardiol. (2011) 58:e212–e60. doi: 10.1016/j.jacc.2011.06.011
23. Beretta L, Santaniello A. Nearest neighbor imputation algorithms: a critical evaluation. BMC Med Inf Decis Making. (2016) 16:74. doi: 10.1186/s12911-016-0318-z
24. Tibshirani R. Regression shrinkage and selection via the LASSO. J R Stat Soc Ser B. (1996) 58:267–88. doi: 10.1111/j.2517-6161.1996.tb02080.x
25. Shapley LS. A value for n-person games. Ann Math Stud. (1953) 28:307–17. doi: 10.1515/9781400881970-018
26. Lundberg SM, Lee S-I editors. A unified approach to interpreting model predictions. In: Advances in Neural Information Processing Systems. Red Hook, NY: Curran Associates, Inc. (2017), 4768–77.
27. Vriesendorp Pieter A, Schinkel Arend FL, Liebregts M, Theuns Dominic AMJ, van Cleemput J, ten Cate Folkert J, et al. Validation of the 2014 European Society of Cardiology guidelines risk prediction model for the primary prevention of sudden cardiac death in hypertrophic cardiomyopathy. Circ Arrhythm Electrophysiol. (2015) 8:829–35. doi: 10.1161/CIRCEP.114.002553
28. O'Mahony C, Jichi F, Pavlou M, Monserrat L, Anastasakis A, Rapezzi C, et al. A novel clinical risk prediction model for sudden cardiac death in hypertrophic cardiomyopathy (HCM Risk-SCD). Eur Heart J. (2014) 35:2010–20. doi: 10.1093/eurheartj/eht439
29. Maron MS, Rowin EJ, Wessler BS, Mooney PJ, Fatima A, Patel P, et al. Enhanced American College of Cardiology/American Heart Association strategy for prevention of sudden cardiac death in high-risk patients with hypertrophic cardiomyopathy. JAMA Cardiol. (2019) 4:644–57. doi: 10.1001/jamacardio.2019.1391
30. Rowin EJ, Maron BJ, Kiernan MS, Casey SA, Feldman DS, Hryniewicz KM, et al. Advanced heart failure with preserved systolic function in nonobstructive hypertrophic cardiomyopathy. Circ Heart Fail. (2014) 7:967–75. doi: 10.1161/CIRCHEARTFAILURE.114.001435
Keywords: heart failure, hypertrophic cardiomyopathy, machine learning, risk factors, risk stratification
Citation: Fahmy AS, Rowin EJ, Manning WJ, Maron MS and Nezafat R (2021) Machine Learning for Predicting Heart Failure Progression in Hypertrophic Cardiomyopathy. Front. Cardiovasc. Med. 8:647857. doi: 10.3389/fcvm.2021.647857
Received: 30 December 2020; Accepted: 29 March 2021;
Published: 13 May 2021.
Edited by:
Gaetano Ruocco, Regina Montis Regalis Hospital, ItalyReviewed by:
Mara Gavazzoni, University Hospital Zürich, SwitzerlandKristen M. Tecson, Baylor Scott and White Research Institute (BSWRI), United States
Copyright © 2021 Fahmy, Rowin, Manning, Maron and Nezafat. This is an open-access article distributed under the terms of the Creative Commons Attribution License (CC BY). The use, distribution or reproduction in other forums is permitted, provided the original author(s) and the copyright owner(s) are credited and that the original publication in this journal is cited, in accordance with accepted academic practice. No use, distribution or reproduction is permitted which does not comply with these terms.
*Correspondence: Reza Nezafat, rnezafat@bidmc.harvard.edu