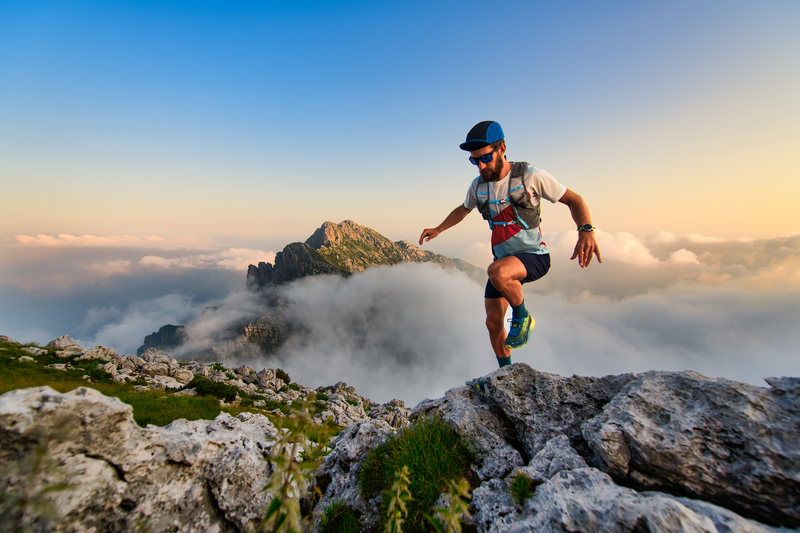
94% of researchers rate our articles as excellent or good
Learn more about the work of our research integrity team to safeguard the quality of each article we publish.
Find out more
ORIGINAL RESEARCH article
Front. Cardiovasc. Med. , 20 May 2021
Sec. Atherosclerosis and Vascular Medicine
Volume 8 - 2021 | https://doi.org/10.3389/fcvm.2021.616081
Background: Acute coronary syndrome (ACS) is the main cause of death and morbidity worldwide. The present study aims to investigate the altered metabolites in plasma from patients with ACS and sought to identify metabolic biomarkers for ACS.
Methods: The plasma metabolomics profiles of 284 ACS patients and 130 controls were carried out based on an untargeted liquid chromatography coupled with tandem mass spectrometry (LC-MS) approach. Multivariate statistical methods, pathway enrichment analysis, and univariate receiver operating characteristic (ROC) curve analysis were performed.
Results: A total of 328 and 194 features were determined in positive and negative electrospray ionization mode in the LC-MS analysis, respectively. Twenty-eight metabolites were found to be differentially expressed, in ACS patients relative to controls (p < 0.05). Pathway analysis revealed that these metabolites are mainly involved in synthesis and degradation of ketone bodies, phenylalanine metabolism, and arginine and proline metabolism. Furthermore, a diagnostic model was constructed based on the metabolites identified and the areas under the curve (AUC) for 5-oxo-D-proline, creatinine, phosphatidylethanolamine lyso 16:0, and LPC (20:4) range from 0.764 to 0.844. The higher AUC value of 0.905 was obtained for the combined detection of phosphatidylethanolamine lyso 16:0 and LPC (20:4).
Conclusions: Differential metabolic profiles may be useful for the effective diagnosis of ACS and may provide additional insight into the molecular mechanisms underlying ACS.
Despite a variety of available therapeutic options, coronary artery disease (CAD) remains a leading cause of mortality worldwide (1, 2). Atherosclerosis has a major pathogenic role for the progression of atherosclerotic coronary artery lesions. Subsequent atherosclerotic plaque rupture or disruption of the overlying endothelial surface is generally associated with obstructive CAD and thus predominantly leads to a wide range of acute coronary syndromes (ACS) (3–5). Rapid and early detection of ACS is an urgent clinical need, as delays increase the risk of morbidity and mortality. Circulating biomarkers within the blood stream are measurable and quantifiable biological parameters that possess a big diagnostic potential serve as indices for physiological and pathophysiological assessments of ACS (6–8). The study of novel biomarkers for the screening and diagnosis of ACS has been the focus of extensive research, but it is still not fully understood.
Recent advances in genomics, proteomics, and other omics technologies led to the innovative development of alternative biomarkers that can facilitate earlier diagnosis of diseases (9, 10). Metabolomics has emerged as a powerful tool for small-molecule identification and quantification through using various metabolomics detection methods (11, 12). Indeed, the small molecules are directly involved in cell composition and metabolism, which might be related to disease-specific biochemical changes and may provide key information for the state of the organism (13, 14). Analytical methods have improved tremendously, enabling the identification of metabolite alterations at the molecular level in biological samples and thereby characterizing aberrant physiological status (15, 16). In the past decade, human metabolomics has been extensively exercised for understanding the fine-tuning of comprehensive metabolic profiling that is associated with different kinds of diseases, such as cancer, Alzheimer, diabetes, and renal disease (17–22).
To date, interest has emerged on the role of differential plasma metabolite occurred in the development of various types of CAD (23–25). Metabolomics is a promising tool of cardiovascular biomarker discovery. Several recent studies have investigated the metabolic profiles in CAD and a fraction of significant altered metabolites have been identified such as amino acids, fatty acid, and several lipid classes, which have been associated with atherosclerosis development (26–29). Despite the progress made, the relevant findings and conclusions of the metabolic signature in ACS are very scarce.
In the present study, to gain a deeper insight into atherosclerosis-related ACS, we first used the untargeted liquid chromatography coupled with tandem mass spectrometry (LC-MS) metabolomics profiling approach to uncover the metabolite alterations in plasma samples from ACS patients. We highlight that novel and intriguing metabolites may serve as diagnostic biomarkers for the differential diagnosis of ACS and improve our understanding of the complex cardiac pathogenic mechanism of atherosclerosis.
The consecutive patients were derived from the department of cardiology in our hospital between January 2016 and December 2018 in this study. Of these, 284 ACS patients with angiographically confirmed ≥50% stenosis of the left main coronary trunk or ≥70% stenosis in a major epicardium artery were included. All of the ACS patients were newly diagnosed and comprised of the following three clinical subtypes: non-ST-elevation myocardial infarction (NSTEMI), ST-elevation myocardial infarction (STEMI), and unstable angina (UA). The diagnoses of NSTEMI and STEMI were made by the electrocardiographic (ECG) changes as well as cardiac enzyme levels. The diagnosis of UA patients was made by the presence of angina pectoris together with evidence of ischemic ECG changes and no elevation in troponin. On the other hand, a total of 130 outpatients for health examination during the same period were selected as the healthy control group. ACS patients with severe liver and/or kidney diseases, malignant tumor, autoimmune disorders, acute or chronic inflammatory disease, and heart failure were ruled out in this study. The study flowchart is illustrated in Figure 1. The demographic and clinical data were collected retrospectively by a trained study coordinator based on a standardized protocol. The presented study was approved by the Medical Ethics Committee of the Meizhou People's Hospital and complied with the principles of Declaration of Helsinki. All study participants included gave written informed consent.
Whole-venous blood samples were drawn in plastic EDTA-coated tubes from all subjects on admission and centrifuged at 3,000 rpm, at 4°C for 10 min. The supernatant plasma was aliquoted and stored at −80°C to prevent sample degradation. Hemolytic samples were excluded. Prior to metabolomics analysis, frozen samples were taken out at −80°C and acclimated at room temperature. An aliquot of 100 μl plasma sample was transferred into 1.5-mL polypropylene tubes and precipitated with 200 μl of precooled methanol. Thereafter, samples were vortexed for 60 s and then centrifuged at 12,000 rpm, at 4°C for 10 min. Subsequently, the supernatant was carefully filtered using a 0.22-μm membrane and then pipetted directly into a 2-ml LC-MS autosampler vial. To ensure instrument stability and data quality for metabolic profiling, a quality control (QC) sample was generated by pooling 20 μL of all the plasma samples.
To obtain a comprehensive metabolic profile, untargeted metabolomics analysis for metabolic profiling was conducted using the Acquity UPLC system (Waters Corporation; Milford, MA, USA) connected to a Thermo LTQ-Orbitrap XL mass spectrometer (Thermo Fisher Scientific, Inc.; Waltham, MA, USA) equipped with an electrospray ionization (ESI) source. Chromatographic separation was performed in an Acquity UPLC system equipped with an Acquity UPLC BEH-C18 column (100 × 2.1 mm, 1.7 μm, Waters). Five microliters of each sample was injected after equilibration at a constant injector temperature of 40°C. The mobile phase was composed of A (0.1% formic acid, v/v) and B (acetonitrile) at a flow rate of 0.25 mL/min. A gradient of solvent B (v/v) from 2 to 98% was run over 15.0 min. The column was then re-equilibrated for 3 min for starting conditions. The ESI-MSn experiment was executed as follows: The voltages of capillary and tube were 35 and 50 V, −15 and −50 V in positive and negative modes, respectively. The sheath gas flow rate was 45 arbitrary units (AU), auxiliary gas flow rate 15 AU, capillary temperature 325°C, and spray voltages of 4.8 and −4.5 kV. The Orbitrap analyzer was used in Fourier scanned transform mass spectrometry (FTMS) full MS scan mode. The MS data was collected which range from 50 to 1,000 m/z with a high mass resolution of 60,000. Collision-induced dissociation product ion mass spectra were conducted in data-dependent acquisition (DDA) MS/MS mode with a normalized collision energy set as 30 eV. Further, dynamic exclusion was set to a repeat count of 2 with a 15-s exclusion duration.
The raw data were processed using the XCMS software, which incorporates non-linear retention time alignment, peak discrimination, filtering, alignment, matching, and identification (30). XCMS parameters were default settings except for the following: bw = 5, ppm = 15, peak width = c (10, 120), mzwid = 0.015, mzdiff = 0.01, method = “centWave.” The mass spectrometry data were converted to the mzXML format using ProteoWizard software (version 3.0). Subsequently, the resultant data matrices were fed to SIMCA-P software (Version 15.0, Umetrics AB, Umeå, Sweden) and R package RoPLS for multivariate principal component analysis (PCA), partial least-square discriminant analysis (PLS-DA), and orthogonal partial least-square discriminant analysis (OPLS-DA). Total explained variance (R2) and predictability (Q2) were performed to determine the validity of the models. Metabolites were identified by mass spectrum matching against reference spectra of the Human Metabolome Database (http://www.hmdb.ca), Metlin (http://metlin.scripps.edu), massbank (http://www.massbank.jp/), LipidMaps (http://www.lipidmaps.org), MONA (http://mona.fiehnlab.ucdavis.edu/) with a mass accuracy of 20 ppm, and database built by BioNovoGene Co., Ltd. A two-sided Student's t-test was applied to find the level of the significance between the metabolites. Variable importance in the projection (VIP) scores more than 1.0 and false discovery rate (FDR) value < 0.05 was used for the identification of potential metabolic biomarkers. Hierarchical cluster analysis (HCA) was performed using Cluster (version 3.0). Furthermore, the disturbed metabolites and their respective metabolic pathways were identified by using Kyoto Encyclopedia of Genes and Genomes (KEGG) pathway database (http://www.genome.jp/kegg/) and MetaboAnalyst (http://www.metaboanalyst.ca/).
All statistical analyses were performed with Statistical Package for the Social Sciences 19.0 software (SPSS, Inc., USA). Continuous variables were expressed as mean ± standard deviation. Categorical data were expressed as count and percentages. The comparison between the ACS patients and controls using Fisher's exact test or Student's t-tests as appropriate. Univariate receiver operating characteristic (ROC) curve analysis was plotted as a measure for assessing the clinical performance of metabolites, and the area under the curve (AUC) was assessed. P-value of <0.05 was considered statistically significant.
The present study population comprised of 284 patients with newly diagnosed ACS (47 NSTEMI patients, 114 STEM patients, and 123 UA patients) and 130 health controls. The baseline characteristics and laboratory data of all the study population are shown in Table 1. There were no statistically significant differences in clinical parameters, such as systolic blood pressure (SBP), diastolic blood pressure (DBP), smoking, drinking, and hypercholesterolemia between the groups for ACS patients and controls (p > 0.05). Compared with the control group, ACS patients had significantly older age, higher proportion of males, higher prevalence of hypertension, and diabetes mellitus (p < 0.05). In addition, the ACS patients had higher low-density lipoprotein (LDL) levels and lower high-density lipoprotein (HDL) levels (p < 0.05). Other laboratory values such as triglyceride (TG), total cholesterol (TC), hemoglobin, and platelet count were not significantly different between groups (p > 0.05).
Representative spectra of the identified metabolites obtained from untargeted LC-MS metabolomics profiling analysis are shown in Supplementary Figure 1. All data were normalized to the summed total ion intensity per chromatogram, and the resultant data matrices were introduced to SIMICA-P 15.0 software. Briefly, after the standard procedure in signal de-noising and dataset normalization, a total of 582 molecular features (328 negative-mode features and 194 positive-mode features) were identified for subsequent analyses. In this study, PCA was initially used to determine the reliability of the metabolic profiling method. The red dots indicated that the QC samples were center clustered, suggesting that the mass accuracy of the MS data fit the requirement (Figure 2A). PCA, PLS-DA, and OPLS-DA were carried out to determine whether it was possible to distinguish controls and ACS patients on the basis of the metabolomics data. PCA indicated that there was no discernible separation between the controls and ACS patients (R2X = 52.0%, Q2 = 24.5%) (Figure 2B). The statistical evaluation by the PLS-DA model showed a good separation of the two groups, implying distinct metabolic variations associated with ACS (R2Y = 69.6%, Q2 = 49.8%) (Figure 2C). Similarly, OPLS-DA score plots also display a distinct separation between these two groups (R2Y = 69.6%, Q2 = 44.3%) (Figure 2D). These results demonstrated the reliable differentiation in metabolic changes between ACS patients and controls.
Figure 2. Results of multivariate statistical analysis for plasma metabolomics profiling. (A) PCA for the QC. (B) PCA for the sample. (C) PLS-DA for the sample. (D) OPLS-DA for the sample. Each dot represents a plasma sample.
The metabolite features were set as VIP scores > 1.0 and FDR < 0.05. Thereby, a total of 28 significant metabolites were selected that can distinguish ACS patients from control subjects, as shown in Table 2 and Supplementary Table 1. The heat map and hierarchical cluster analysis illustrate the different distribution patterns of a total of 28 potential biomarkers between the two groups, as presented in Figure 3. Next, these differential metabolites were entered as input data to perform the pathway enrichment analysis. The detailed results of the pathway analysis are shown in Figure 4 and Table 3, respectively. The potential pathways were characterized as synthesis and degradation of ketone bodies; phenylalanine metabolism; arginine and proline metabolism; valine, leucine, and isoleucine degradation; and others. These results provided biological relevance between ACS patients and control subjects.
Figure 3. The hierarchical clustering heat map of the 28 metabolites. The rows represent the 28 metabolites, and the columns represent samples in the control and ACS patients. VIP scores > 1.0 and FDR < 0.05 were considered as significant differences.
Figure 4. Metabolomics view from pathway analysis performed using MetaboAnalyst. The node color is based on its p-value, and the node radius is based on their pathway impact values.
On the basis of the above studies, box plots and classical univariate ROC curve analyses were applied to further investigate the clinical diagnostic ability of the metabolite candidates. As would be expected, the box plot represents the relative changes in the four potential metabolites 5-oxo-D-proline, 3-hydroxy-3-methylglutaric acid, phosphatidylethanolamine lyso 16:0, and LPC (20:4) which differ significantly among subclinical types of ACS patients and controls (Figure 5). Furthermore, ROC curves also showed an apparent discrimination of these four metabolites between groups, as shown in Figure 6 and Table 4. Among them, the ROC curves of phosphatidylethanolamine lyso 16:0 and LPC (20:4) showed a relatively higher diagnostic performance from controls with AUC values of 0.844 (95% CI: 0.800–0.888; p < 0.001) and 0.821 (95% CI: 0.774–0.868; p < 0.001), respectively, while the ROC curves of 5-oxo-D-proline and creatinine exhibited a moderate diagnostic performance with AUC values of 0.772 (95% CI: 0.720–0.825; p < 0.001) and 0.764 (95% CI: 0.711–0.817; p < 0.001), respectively. In addition, the combination of phosphatidylethanolamine lyso 16:0 and LPC (20:4) significantly improved the AUC value of 0.905 (95% CI: 0.873–0.937; p < 0.001) than in single metabolite detection mode.
Figure 5. Box plots of metabolites to discriminate ACS patients from controls (ACS patients comprise of UA, NSTEMI, and STEMI patients). (A) 5-Oxo-D-proline. (B) Creatinine. (C) Phosphatidylethanolamine lyso 16:0. (D) LPC (20:4).
Figure 6. Receiver operating characteristic (ROC) curve model of metabolites to discriminate ACS patients from controls.
Metabolomics could be of great advantage in the study of metabolite profiling in human disease, where metabolism is changeable in the biochemical activities of an organism (7, 13, 26). Plasma metabolomics analysis can provide a more comprehensive understanding of the metabolite alterations in ACS (10, 27). Herein, an untargeted LC-MS-based metabolomics profiling platform was conducted to analyze the changes of plasma metabolite levels in 284 ACS patients and 130 controls. The presented results demonstrate the metabolic level in ACS patients differing from those of controls. Particularly, 28 significantly changed metabolites were determined and subsequent metabolic pathway analysis showed that these altered metabolites were mainly involved in several biochemical pathways, including synthesis and degradation of ketone bodies, phenylalanine metabolism, and arginine and proline metabolism. Furthermore, the univariate ROC curve revealed that the existence of four metabolites can correctly distinguish ACS patients from the control group at a certain extent, respectively. Within the limits of our untargeted metabolomics approach, we postulate that a subset of differentially abundant metabolites can serve as potential ACS biomarkers and may provide corroborating evidence of the involvement of plasma metabolite in the pathogenesis of ACS.
The atherosclerotic processes are often present for years before developing into the onset of overt disease; there has been great interest in determining whether metabolomic profiling can identify individuals for the development of subclinical atherosclerosis. By design, in the present research, plasma metabolite profiling of ACS patients revealed differences with those of healthy controls. These results were in concordance with other recently published data describing abnormal metabolites in CAD. For example, of these metabolites, changes in serum creatinine are known to contribute to the incidence of CAD independent of traditional risk factors (26). The ketone body acetoacetate is an energy-providing substrate of respiration of the heart, but it varies with the pathophysiological state (31, 32). In spite that the underlying mechanisms for these changes remain unclear, it could be speculated that the pathological process of ACS is related with slower metabolism and less energy expenditure requirements. Choline has various metabolic roles, and experimental evidence clearly indicates that the choline moiety in dietary phosphatidylcholine (PC) could produce proatherogenic species after metabolism by intestinal microbiota (13, 33, 34). Consistently, other changes are in lysophosphatidylcholine (LPC) and lysophosphatidylethanolamine (LPE), which are lipid classes known to contribute to the risk of CVD (35, 36). Several prior studies also have demonstrated that LPC is one of the major by-products of phospholipid oxidation, and it may be an implication for atherosclerotic plaque characteristics (36–38). Collectively, this consistency reinforces the potential causal relationship between significantly altered concentrations of metabolites and ACS, and the relatively subtle perturbations in metabolic might serve as potential diagnostic biomarkers in patients with ACS.
Additionally, observations of changes in aberrant metabolites in the setting of ACS in fact reflected coordinate changes in several important metabolic pathways. For instance, among these metabolic pathways, synthesis and degradation of the ketone body pathway were identified as vital pathways involved in the pathogenesis of atherosclerosis, which have been well-characterized and widely studied (39, 40). Phenylalanine is an essential aromatic amino acid and the precursor for tyrosine and dopamine-related neurotransmitters. A small case–control study using LC-MS suggested that an amino acid score including phenylalanine is associated with cardiovascular risk (17). Subsequent metabolite profiling in a large prospective cohort has also affirmed this conclusion (14). Valine has been reported to be associated with metabolic risk factors, insulin resistance, incident type 2 diabetes, and future cardiovascular events (41, 42). Recently, it has been reported that arginine is crucial for nitric oxide (NO) synthesis and the bioavailability of NO is particularly involved in endothelium dysfunction, eventually leading to an increased risk of cardiovascular mortality (43). A previous metabolomics study has revealed that branched-chain amino acid concentrations were independently associated with death or myocardial infarction during follow-up (44). Altogether, these results illustrate the potential importance metabolic pathways in ACS, as function and metabolism are inextricably linked. Further studies are required to understand the mechanistic significance of these altered metabolites in cardiovascular disease.
In addition, we examined the discriminative capability of the metabolites identified in our study using ROC analysis. Indeed, the current clinical symptoms from the differential diagnosis of ACS have been a bottleneck to solve because of the interindividual variability (7, 45). Of interest, in the present study, groups were apparently differentiated depending on four candidate metabolites (5-oxo-D-proline, creatinine, phosphatidylethanolamine lyso 16:0, and LPC (20:4), with an AUC range from 0.764 to 0.821. The combined analysis of phosphatidylethanolamine lyso 16:0 and LPC (20:4) was interesting and potentially applicable for improved identification of ACS patients. Although our study shows that single metabolites could be used as biomarkers to differentiate between controls and ACS patients, and a combination of these metabolites may improve predictive performance (46), it remains to be determined in clinical trials.
Prior substantial studies have identified many potential metabolites with CAD in a wide range of population; however, results are not always unequivocal. It is important to recognize that the clinical manifestations vary greatly among ACS patients, ranging from unstable angina without myocardial necrosis to ST segment elevation myocardial infarction. Metabolites obtained from patients with ACS may be very promiscuous, and no single analysis or method is adequate to reliably identify all of these metabolites in human samples. For example, a prior study analyzed peripheral plasma from non-ST segment elevation ACS patients, suggesting that the panel of biomarkers consisting of 5-OH-tryptophan, 2-OH-butyric acid, and 3-OH-butyric have a great potential for early diagnosis of ACS (47). Another recent study comprehensively analyzed the serum metabolomic change in ACS patients, proposing that the combined model of serum betaine and ejection fraction might serve as a potential diagnostic biomarker for the vulnerability of plaque stability (48). In this study, we also successfully characterized the significantly altered metabolites using blood samples in the setting of ACS. However, our findings are not entirely consistent with previous studies. The results of these studies indicated that serum metabolite profiling of ACS patients revealed a myriad of differentially occurring metabolites. The heterogeneity of the results might relate to differences in age, sex, ethnicity, geographical region, nutritional intake, medications, and lifestyle factors, as well as differences in analytical methods, which are known to influence metabolite levels (49). These characteristics are particularly important when analyzing the complex mixtures of metabolites.
Several limitations of our study should be noted. Firstly, it must be mentioned that, due to the one single cohort and the choice for an untargeted metabolomics approach, the results and conclusions drawn to these metabolites should be interpreted with caution. Therefore, our findings should be validated in a larger population, as well as in other regions and ethnic groups. Secondly, the cross-sectional design rather than longitudinal design of the study may limit the clinical value of the measured metabolites. Hence, additional prospective validation is required to judge the clinical usefulness of these presented metabolites in pretreatment and post-treatment samples in ACS patients.
In conclusion, this study provided relatively wider metabolite coverage in patients with ACS by using an untargeted LC/MS metabolomics profiling approach. The apparent differences may reflect the onset and progression of atherosclerosis in ACS and could lead to novel diagnostic biomarker development and clinical application. However, these results are explorative and further investigation with external validation is warranted to underpin these observations and specify their role in ACS-specific metabolic alteration.
The datasets used and analyzed during the current study are available from the corresponding author on reasonable request.
The studies involving human participants were reviewed and approved by Medical Ethics Committee of the Meizhou People's Hospital. The patients/participants provided their written informed consent to participate in this study.
WZ, ZZ, and JH contributed to the study concept and design, conducted the literature search, and wrote the manuscript. WZ, QD, and XD contributed to the data analysis and made the tables and figures. QD and XD contributed to the collection of patients' samples and medical information. ZZ and JH critically revised the manuscript. All authors contributed to the article and approved the submitted version.
This work was supported by the Medical Scientific Research Foundation of Guangdong Province (No. A2020418); Science and Technology Program of Meizhou (No. 2018B024); Research and Cultivation Project of Meizhou People's Hospital (Nos. PYC2019013 and PYA2019001).
The authors declare that the research was conducted in the absence of any commercial or financial relationships that could be construed as a potential conflict of interest.
We sincerely thank Suzhou BioNovoGene Metabolomics Platform for the assistance in LC-MS analysis.
The Supplementary Material for this article can be found online at: https://www.frontiersin.org/articles/10.3389/fcvm.2021.616081/full#supplementary-material
Supplementary Figure 1. Representative LC-MS chromatograms of plasma derived from controls and ACS patients.
Supplementary Table 1. Summary of the molecular features of differential metabolites between ACS patients and controls.
1. Roth GA, Forouzanfar MH, Moran AE, Barber R, Nguyen G, Feigin VL, et al. Demographic and epidemiologic drivers of global cardiovascular mortality. N Engl J Med. (2015) 372:1333–41. doi: 10.1056/NEJMoa1406656
2. Roth GA, Nguyen G, Forouzanfar MH, Mokdad AH, Naghavi M, Murray CJ. Estimates of global and regional premature cardiovascular mortality in 2025. Circulation. (2015) 132:1270–82. doi: 10.1161/CIRCULATIONAHA.115.016021
3. Hansson GK. Inflammation, atherosclerosis, and coronary artery disease. N Engl J Med. (2005) 352:1685–95. doi: 10.1056/NEJMra043430
4. Hoefer IE, Steffens S, Ala-Korpela M, Back M, Badimon L, Bochaton-Piallat ML, et al. Novel methodologies for biomarker discovery in atherosclerosis. Eur Heart J. (2015) 36:2635–42. doi: 10.1093/eurheartj/ehv236
5. O'Connor RE, Brady W, Brooks SC, Diercks D, Egan J, Ghaemmaghami C, et al. Part 10: acute coronary syndromes: 2010 American Heart Association Guidelines for Cardiopulmonary Resuscitation and Emergency Cardiovascular Care. Circulation. (2010) 122:S787–817. doi: 10.1161/CIRCULATIONAHA.110.971028
6. Gerszten RE, Wang TJ. The search for new cardiovascular biomarkers. Nature. (2008) 451:949–52. doi: 10.1038/nature06802
7. Shah SH, Kraus WE, Newgard CB. Metabolomic profiling for the identification of novel biomarkers and mechanisms related to common cardiovascular diseases: form and function. Circulation. (2012) 126:1110–20. doi: 10.1161/CIRCULATIONAHA.111.060368
8. Gilstrap LG, Wang TJ. Biomarkers and cardiovascular risk assessment for primary prevention: an update. Clin Chem. (2012) 58:72–82. doi: 10.1373/clinchem.2011.165712
9. Becker S, Kortz L, Helmschrodt C, Thiery J, Ceglarek U. LC-MS-based metabolomics in the clinical laboratory. J Chromatogr B Anal Technol Biom. (2012) 883–4:68–75. doi: 10.1016/j.jchromb.2011.10.018
10. Didangelos A, Stegemann C, Mayr M. The -omics era: proteomics and lipidomics in vascular research. Atherosclerosis. (2012) 221:12–7. doi: 10.1016/j.atherosclerosis.2011.09.043
11. Ellis DI, Dunn WB, Griffin JL, Allwood JW, Goodacre R. Metabolic fingerprinting as a diagnostic tool. Pharmacogenomics. (2007) 8:1243–66. doi: 10.2217/14622416.8.9.1243
12. Zhang A, Sun H, Wang P, Han Y, Wang X. Modern analytical techniques in metabolomics analysis. Analyst. (2012) 137:293–300. doi: 10.1039/C1AN15605E
13. Tang WH, Wang Z, Levison BS, Koeth RA, Britt EB, Fu X, et al. Intestinal microbial metabolism of phosphatidylcholine and cardiovascular risk. N Engl J Med. (2013) 368:1575–84. doi: 10.1056/NEJMoa1109400
14. Würtz P, Havulinna AS, Soininen P, Tynkkynen T, Prieto-Merino D, Tillin T, et al. Metabolite profiling and cardiovascular event risk: a prospective study of 3 population-based cohorts. Circulation. (2015) 131:774–85. doi: 10.1161/CIRCULATIONAHA.114.013116
15. Beckonert O, Keun HC, Ebbels TM, Bundy J, Holmes E, Lindon JC, et al. Metabolic profiling, metabolomic and metabonomic procedures for NMR spectroscopy of urine, plasma, serum and tissue extracts. Nat Protoc. (2007) 2:2692–703. doi: 10.1038/nprot.2007.376
16. Nicholson JK, Wilson ID. Opinion: understanding ‘global’ systems biology: metabonomics and the continuum of metabolism. Nat Rev Drug Discov. (2003) 2:668–76. doi: 10.1038/nrd1157
17. Magnusson M, Lewis GD, Ericson U, Orho-Melander M, Hedblad B, Engström G, et al. A diabetes-predictive amino acid score and future cardiovascular disease. Eur Heart J. (2013) 34:1982–9. doi: 10.1093/eurheartj/ehs424
18. Kalim S, Clish CB, Wenger J, Elmariah S, Yeh RW, Deferio JJ, et al. A plasma long-chain acylcarnitine predicts cardiovascular mortality in incident dialysis patients. J Am Heart Assoc. (2013) 2:e000542. doi: 10.1161/JAHA.113.000542
19. Wang TJ, Larson MG, Vasan RS, Cheng S, Rhee EP, McCabe E, et al. Metabolite profiles and the risk of developing diabetes. Nat Med. (2011) 17:448–53. doi: 10.1038/nm.2307
20. Kimberly WT, Wang Y, Pham L, Furie KL, Gerszten RE. Metabolite profiling identifies a branched chain amino acid signature in acute cardioembolic stroke. Stroke. (2013) 44:1389–95. doi: 10.1161/STROKEAHA.111.000397
21. Lanning NJ, Castle JP, Singh SJ, Leon AN, Tovar EA, Sanghera A, et al. Metabolic profiling of triple-negative breast cancer cells reveals metabolic vulnerabilities. Cancer Metab. (2017) 5:6. doi: 10.1186/s40170-017-0168-x
22. de Leeuw FA, Peeters CFW, Kester MI, Harms AC, Struys EA, Hankemeier T, et al. Blood-based metabolic signatures in Alzheimer's disease. Alzheimers Dement. (2017) 8:196–207. doi: 10.1016/j.dadm.2017.07.006
23. Rhee EP, Gerszten RE. Metabolomics and cardiovascular biomarker discovery. Clin Chem. (2012) 58:139–47. doi: 10.1373/clinchem.2011.169573
24. Ruiz-Canela M, Toledo E, Clish CB, Hruby A, Liang L, Salas-Salvado J, et al. Plasma branched-Chain amino acids and incident cardiovascular disease in the PREDIMED trial. Clin Chem. (2016) 62:582–92. doi: 10.1373/clinchem.2015.251710
25. Ganna A, Salihovic S, Sundstrom J, Broeckling CD, Hedman AK, Magnusson PK, et al. Large-scale metabolomic profiling identifies novel biomarkers for incident coronary heart disease. PLoS Genet. (2014) 10:e1004801. doi: 10.1371/journal.pgen.1004801
26. Vaarhorst AA, Verhoeven A, Weller CM, Bohringer S, Goraler S, Meissner A, et al. A metabolomic profile is associated with the risk of incident coronary heart disease. Am Heart J. (2014) 168:45–52.e7. doi: 10.1016/j.ahj.2014.01.019
27. Sabatine MS, Liu E, Morrow DA, Heller E, McCarroll R, Wiegand R, et al. Metabolomic identification of novel biomarkers of myocardial ischemia. Circulation. (2005) 112:3868–75. doi: 10.1161/CIRCULATIONAHA.105.569137
28. Brindle JT, Antti H, Holmes E, Tranter G, Nicholson JK, Bethell HW, et al. Rapid and noninvasive diagnosis of the presence and severity of coronary heart disease using 1H-NMR-based metabonomics. Nat Med. (2002) 8:1439–44. doi: 10.1038/nm1202-802
29. Shah SH, Sun JL, Stevens RD, Bain JR, Muehlbauer MJ, Pieper KS, et al. Baseline metabolomic profiles predict cardiovascular events in patients at risk for coronary artery disease. Am Heart J. (2012) 163:844–50.e1. doi: 10.1016/j.ahj.2012.02.005
30. Smith CA, Want EJ, O'Maille G, Abagyan R, Siuzdak G. XCMS: processing mass spectrometry data for metabolite profiling using nonlinear peak alignment, matching, and identification. Anal Chem. (2006) 78:779–87. doi: 10.1021/ac051437y
31. Stanley WC, Recchia FA, Lopaschuk GD. Myocardial substrate metabolism in the normal and failing heart. Physiol Rev. (2005) 85:1093–129. doi: 10.1152/physrev.00006.2004
32. Carley AN, Taegtmeyer H, Lewandowski ED. Matrix revisited: mechanisms linking energy substrate metabolism to the function of the heart. Circ Res. (2014) 114:717–29. doi: 10.1161/CIRCRESAHA.114.301863
33. Danne O, Lueders C, Storm C, Frei U, Mockel M. Whole blood choline and plasma choline in acute coronary syndromes: prognostic and pathophysiological implications. Clin Chim Acta. (2007) 383:103–9. doi: 10.1016/j.cca.2007.05.001
34. Bidulescu A, Chambless LE, Siega-Riz AM, Zeisel SH, Heiss G. Usual choline and betaine dietary intake and incident coronary heart disease: the atherosclerosis risk in communities (ARIC) study. BMC Cardiovasc Disord. (2007) 7:20. doi: 10.1186/1471-2261-7-20
35. Stegemann C, Pechlaner R, Willeit P, Langley SR, Mangino M, Mayr U, et al. Lipidomics profiling and risk of cardiovascular disease in the prospective population-based Bruneck study. Circulation. (2014) 129:1821–31. doi: 10.1161/CIRCULATIONAHA.113.002500
36. Goncalves I, Edsfeldt A, Ko NY, Grufman H, Berg K, Bjorkbacka H, et al. Evidence supporting a key role of Lp-PLA2-generated lysophosphatidylcholine in human atherosclerotic plaque inflammation. Arterioscler Thromb Vasc Biol. (2012) 32:1505–12. doi: 10.1161/ATVBAHA.112.249854
37. Cunningham TJ, Yao L, Lucena A. Product inhibition of secreted phospholipase A2 may explain lysophosphatidylcholines' unexpected therapeutic properties. J Inflamm. (2008) 5:17. doi: 10.1186/1476-9255-5-17
38. Lin H, Zhang J, Gao P. Silent myocardial ischemia is associated with altered plasma phospholipids. J Clin Lab Anal. (2009) 23:45–50. doi: 10.1002/jcla.20288
39. Cotter DG, Schugar RC, Crawford PA. Ketone body metabolism and cardiovascular disease. Am J Physiol Heart Circ Physiol. (2013) 304:H1060–76. doi: 10.1152/ajpheart.00646.2012
40. Nasser S, Vialichka V, Biesiekierska M, Balcerczyk A, Pirola L. Effects of ketogenic diet and ketone bodies on the cardiovascular system: concentration matters. World J Diabetes. (2020) 11:584–95. doi: 10.4239/wjd.v11.i12.584
41. Cheng S, Rhee EP, Larson MG, Lewis GD, McCabe EL, Shen D, et al. Metabolite profiling identifies pathways associated with metabolic risk in humans. Circulation. (2012) 125:2222–31. doi: 10.1161/CIRCULATIONAHA.111.067827
42. Würtz P, Soininen P, Kangas AJ, Rönnemaa T, Lehtimäki T, Kähönen M, et al. Branched-chain and aromatic amino acids are predictors of insulin resistance in young adults. Diabetes Care. (2013) 36:648–55. doi: 10.2337/dc12-0895
43. Sourij H, Meinitzer A, Pilz S, Grammer TB, Winkelmann BR, Boehm BO, et al. Arginine bioavailability ratios are associated with cardiovascular mortality in patients referred to coronary angiography. Atherosclerosis. (2011) 218:220–5. doi: 10.1016/j.atherosclerosis.2011.04.041
44. Shah SH, Bain JR, Muehlbauer MJ, Stevens RD, Crosslin DR, Haynes C, et al. Association of a peripheral blood metabolic profile with coronary artery disease and risk of subsequent cardiovascular events. Circ Cardiovasc Genet. (2010) 3:207–14. doi: 10.1161/CIRCGENETICS.109.852814
45. Lewis GD, Asnani A, Gerszten RE. Application of metabolomics to cardiovascular biomarker and pathway discovery. J Am Coll Cardiol. (2008) 52:117–23. doi: 10.1016/j.jacc.2008.03.043
46. Hou W, Meng X, Zhao A, Zhao W, Pan J, Tang J, et al. Development of multimarker diagnostic models from metabolomics analysis for gestational diabetes mellitus (GDM). Mol Cell Proteomics. (2018) 17:431–41. doi: 10.1074/mcp.RA117.000121
47. Laborde CM, Mourino-Alvarez L, Posada-Ayala M, Alvarez-Llamas G, Serranillos-Reus MG, Moreu J, et al. Plasma metabolomics reveals a potential panel of biomarkers for early diagnosis in acute coronary syndrome. Metabolomics. (2014) 10:414–24. doi: 10.1007/s11306-013-0595-9
48. Jiang H, Jiang H, Zhang J, Chen W, Luo C, Li H, et al. The serum metabolic biomarkers in early diagnosis and risk stratification of acute coronary syndrome. Front Physiol. (2020) 11:776. doi: 10.3389/fphys.2020.00776
Keywords: acute coronary syndromes, metabolomics, diagnosis, biomarkers, liquid-chromatography coupled with tandem mass spectrometry
Citation: Zhong W, Deng Q, Deng X, Zhong Z and Hou J (2021) Plasma Metabolomics of Acute Coronary Syndrome Patients Based on Untargeted Liquid Chromatography–Mass Spectrometry. Front. Cardiovasc. Med. 8:616081. doi: 10.3389/fcvm.2021.616081
Received: 11 October 2020; Accepted: 18 March 2021;
Published: 20 May 2021.
Edited by:
Sasha A. Singh, Brigham and Women's Hospital and Harvard Medical School, United StatesCopyright © 2021 Zhong, Deng, Deng, Zhong and Hou. This is an open-access article distributed under the terms of the Creative Commons Attribution License (CC BY). The use, distribution or reproduction in other forums is permitted, provided the original author(s) and the copyright owner(s) are credited and that the original publication in this journal is cited, in accordance with accepted academic practice. No use, distribution or reproduction is permitted which does not comply with these terms.
*Correspondence: Wei Zhong, cmVkc2t5ODlAMTYzLmNvbQ==; Zhixiong Zhong, emhvbmd6aGl4aW9uZ0BtenJteXkuY29t; Jingyuan Hou, bW9ua2V5aGp5QDEyNi5jb20=
Disclaimer: All claims expressed in this article are solely those of the authors and do not necessarily represent those of their affiliated organizations, or those of the publisher, the editors and the reviewers. Any product that may be evaluated in this article or claim that may be made by its manufacturer is not guaranteed or endorsed by the publisher.
Research integrity at Frontiers
Learn more about the work of our research integrity team to safeguard the quality of each article we publish.