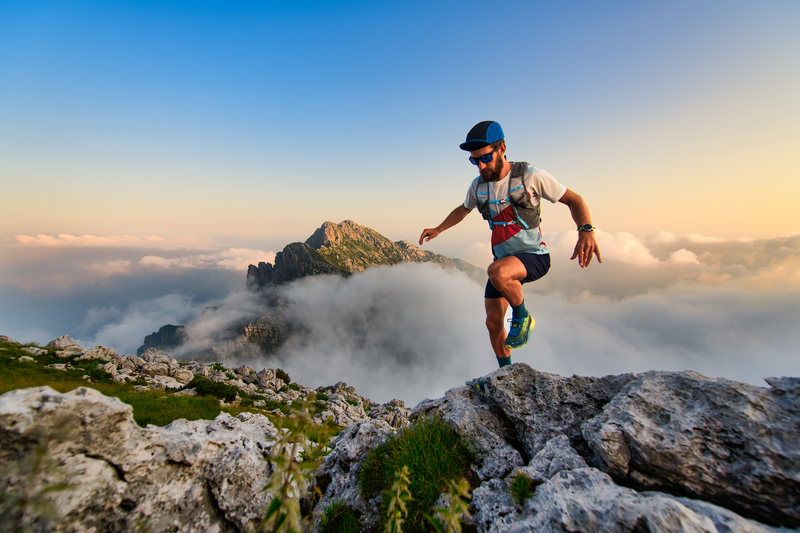
94% of researchers rate our articles as excellent or good
Learn more about the work of our research integrity team to safeguard the quality of each article we publish.
Find out more
BRIEF RESEARCH REPORT article
Front. Cardiovasc. Med. , 05 February 2021
Sec. Cardiac Rhythmology
Volume 8 - 2021 | https://doi.org/10.3389/fcvm.2021.608592
This article is part of the Research Topic Risk Stratification Strategies for Cardiac Rhythm Abnormalities View all 49 articles
A retraction of this article was approved in:
Retraction: Territory-Wide Chinese Cohort of Long QT Syndrome: Random Survival Forest and Cox Analyses
Citation: Tse G, Lee S, Zhou J, Liu T, Wong ICK, Mak C, Mok NS, Jeevaratnam K, Zhang Q, Cheng SH and Wong WT (2021) Territory-Wide Chinese Cohort of Long QT Syndrome: Random Survival Forest and Cox Analyses. Front. Cardiovasc. Med. 8:608592. doi: 10.3389/fcvm.2021.608592
Received: 28 October 2020; Accepted: 11 January 2021;
Published: 05 February 2021; Retracted: 28 November 2024.
Edited by:
Marina Cerrone, New York University, United StatesReviewed by:
Wojciech Zareba, University of Rochester, United StatesDisclaimer: All claims expressed in this article are solely those of the authors and do not necessarily represent those of their affiliated organizations, or those of the publisher, the editors and the reviewers. Any product that may be evaluated in this article or claim that may be made by its manufacturer is not guaranteed or endorsed by the publisher.
Research integrity at Frontiers
Learn more about the work of our research integrity team to safeguard the quality of each article we publish.