- Department of Cardiovascular Surgery, Zhongshan Hospital, Fudan University, Shanghai, China
Background: Atrial fibrillation (AF) is the most common tachyarrhythmia in the clinic, leading to high morbidity and mortality. Although many studies on AF have been conducted, the molecular mechanism of AF has not been fully elucidated. This study was designed to explore the molecular mechanism of AF using integrative bioinformatics analysis and provide new insights into the pathophysiology of AF.
Methods: The GSE115574 dataset was downloaded, and Cibersort was applied to estimate the relative expression of 22 kinds of immune cells. Differentially expressed genes (DEGs) were identified through the limma package in R language. Weighted gene correlation network analysis (WGCNA) was performed to cluster DEGs into different modules and explore relationships between modules and immune cell types. Functional enrichment analysis was performed on DEGs in the significant module, and hub genes were identified based on the protein-protein interaction (PPI) network. Hub genes were then verified using quantitative real-time polymerase chain reaction (qRT-PCR).
Results: A total of 2,350 DEGs were identified and clustered into eleven modules using WGCNA. The magenta module with 246 genes was identified as the key module associated with M1 macrophages with the highest correlation coefficient. Three hub genes (CTSS, CSF2RB, and NCF2) were identified. The results verified using three other datasets and qRT-PCR demonstrated that the expression levels of these three genes in patients with AF were significantly higher than those in patients with SR, which were consistent with the bioinformatic analysis.
Conclusion: Three novel genes identified using comprehensive bioinformatics analysis may play crucial roles in the pathophysiological mechanism in AF, which provide potential therapeutic targets and new insights into the treatment and early detection of AF.
Introduction
Atrial fibrillation, the most common sustained arrhythmias in the clinic (1), affects ~1 to 2% of the population (2), which increases the morbidity, mortality, and medical burden worldwide (3). The risk of stroke in patients with AF is approximately five-fold higher than that in healthy people (4). AF is a complex disease (5) associated with common risk factors, including advancing age, male sex, hypertension, obesity, and diabetes (6). However, the pathophysiological mechanism leading to AF is still unclear. Increasing evidence demonstrates that immune cells play a significant role in the pathogenesis of AF (7). Several immune-mediated serum inflammatory markers such as CRP and IL-6 have been confirmed to be elevated in patients with AF (8, 9). Nevertheless, the association between immune cells and the biological molecular mechanism of AF still needs further research to clarify the pathogenesis of AF and find potential therapeutic targets.
Weighted gene correlation network analysis (WGCNA) is a system biology method used to cluster genes into modules according to expression patterns among different samples (10). Based on the interconnectivity of genes, WGCNA can explore the relationships between gene modules and the clinical phenotypes to identify candidate biomarkers or therapeutic targets. It has been applied to numerous kinds of diseases (11–14).
In this study, we aimed to explore the association between immune cells and AF using comprehensive bioinformatics analysis. Cibersort was used to evaluate immune cell composition, and WGCNA was used to identify the hub gene module. We then analyzed the functional enrichment of the genes in the hub module. Based on the protein-protein interaction (PPI) network, hub genes were identified for further analysis and validation. We hope that this research can provide potential targets and new research ideas for the treatment of AF.
Methods and Materials
Data Acquisition and Processing
The GSE115574 dataset, including fourteen gene expression profiles from left atrial tissues of AF patients and fifteen gene expression profiles from left atrial tissues of sinus rhythm (SR) patients, were downloaded in the Gene Expression Omnibus (GEO) database. The R language was used to process the original expression profile of the GSE115574 dataset. Cibersort, a bioinformatics algorithm that could estimate 22 immune cell types, was applied to evaluate immune cell composition based on the gene expression matrix (15). The Linear Models for Microarray data (limma) package in the R language (16) was utilized to identify differentially expressed genes (DEGs) with a p < 0.05 between patients with AF and SR. The GSE31821, GSE41177, and GSE79768 datasets were used for validation. The batch effect was removed using the SVA package in the R language.
WGCNA
The WGCNA (10) was applied to construct the mRNA co-expression network based on the DEGs. Briefly, an appropriate soft-thresholding power β was determined to realize the scale-free topology. Then DEGs were clustered into modules, which were labeled with different colors using the average linkage hierarchical clustering method. The minimum number of genes in each module was twenty, and the threshold for module merging was 0.25. Pearson's correlation method was utilized to calculate the correlation between each module and the relative expression of immune cells identified by the Cibersort. The module with the highest correlation coefficient was selected for further analyses.
Functional Enrichment Analyses
The Database for Annotation, Visualization and Integrated Discovery (DAVID, v6.8) (17) was used to perform the Gene Ontology (GO) (18, 19) and Kyoto Encyclopedia of Genes and Genomes (KEGG) pathway enrichment analyses, which revealed the biological processes (BPs), cellular components (CCs), molecular functions (MFs), and pathways related to genes in the module identified above. GO terms and KEGG maps with a p < 0.05 were considered significant enrichment.
Construction of PPI Network and Hub Genes Identification
The DEGs in the selected module were imported into the Search Tool for the Retrieval of Interacting Genes (STRING, v11.0) (20) to generate the PPI network identifying the interactions between the genes with the threshold of interaction score >0.4. Nodes represent proteins, and edges represent protein-protein associations in the PPI network. The results downloaded from the STRING database was then visualized utilizing Cytoscape software (v3.8.1). CytoHubba (21), a plug-in of the Cytoscape software, was used to identified hub genes. The intersection of five algorithms in CytoHubba was generated for hub genes identification to ensure the accuracy and robustness of the results.
Sample Collection
Adult patients with persistent AF undergoing cardiac surgery in Zhongshan Hospital were included in this study. Persistent AF is defined as AF which is continuously sustained beyond 7 days, including episodes terminated by cardioversion (drugs or electrical cardioversion) after more than seven days (3). Excluded from the research were patients with coronary artery heart disease, hypertension, diabetes, or obstructive sleep apnea syndrome, whose ejection fractions were <30%, and those who had contraindications to surgery. The left atrium tissues and blood samples of twenty patients with persistent AF and ten healthy donors with SR were collected during cardiac surgery. The samples were then immediately preserved in liquid nitrogen for the later experiment. This study was in full compliance with the Declaration of Helsinki and approved by the Medical Ethics Committee of Zhongshan Hospital, Fudan University (Approval No. B2019-198R). All patients participating in this study have signed written informed consent before surgery.
Quantitative Real-Time Polymerase Chain Reaction (qRT-PCR)
Total left atrium tissue RNA was extracted with the RNeasy™ Mini Kit (QIAGEN, Frankfurt, Germany) following the manufacturer's instruction. The PrimeScript™ RT reagent Kit (Takara, Otsu, Japan) was used to conduct reverse transcription. QRT-PCR was performed with the TB Green® Premix Ex Taq™ II (Takara, Otsu, Japan) on QuantStudio™ 5 System (Thermo Fisher Science, Waltham, MA, USA). The expression data was normalized by GAPDH, and the 2−ΔΔCT method was applied to analyze the results. All sequences for RNA primers (Sangon Biotech, Shanghai, China) are shown in Table 1.
Results
Identification of DEGs
A total of 2,350 genes, including 1,115 upregulated and 1,235 downregulated, which were differentially expressed between AF samples and SR samples, were identified in the GSE115574 dataset with the limma package.
Relative Immune Cells Expression
The Cibersort with 22 types of immune cell subtypes was applied to estimate the putative relative expression of immune cells. The results are shown in Figure 1. Naïve B cells, CD4 naïve T cells, CD4 memory activated T cells, gamma delta T cells, resting NK cells, M0 macrophages, activated mast cells, eosinophils, and neutrophils were eliminated because most of the samples were not inferred to express in these immune cells. The correlation of remaining immune cells was calculated using the Pearson correlation coefficient, as shown in Figure 2. M1 macrophages were significantly negatively correlated with activated NK cells (r = −0.42, p = 0.0247). CD4 memory resting T cells were significantly negatively correlated with CD8 T cells (r = −0.43, p = 0.0191). Resting NK cells were significantly negatively correlated with activated NK cells (r = −0.78, p < 0.001).
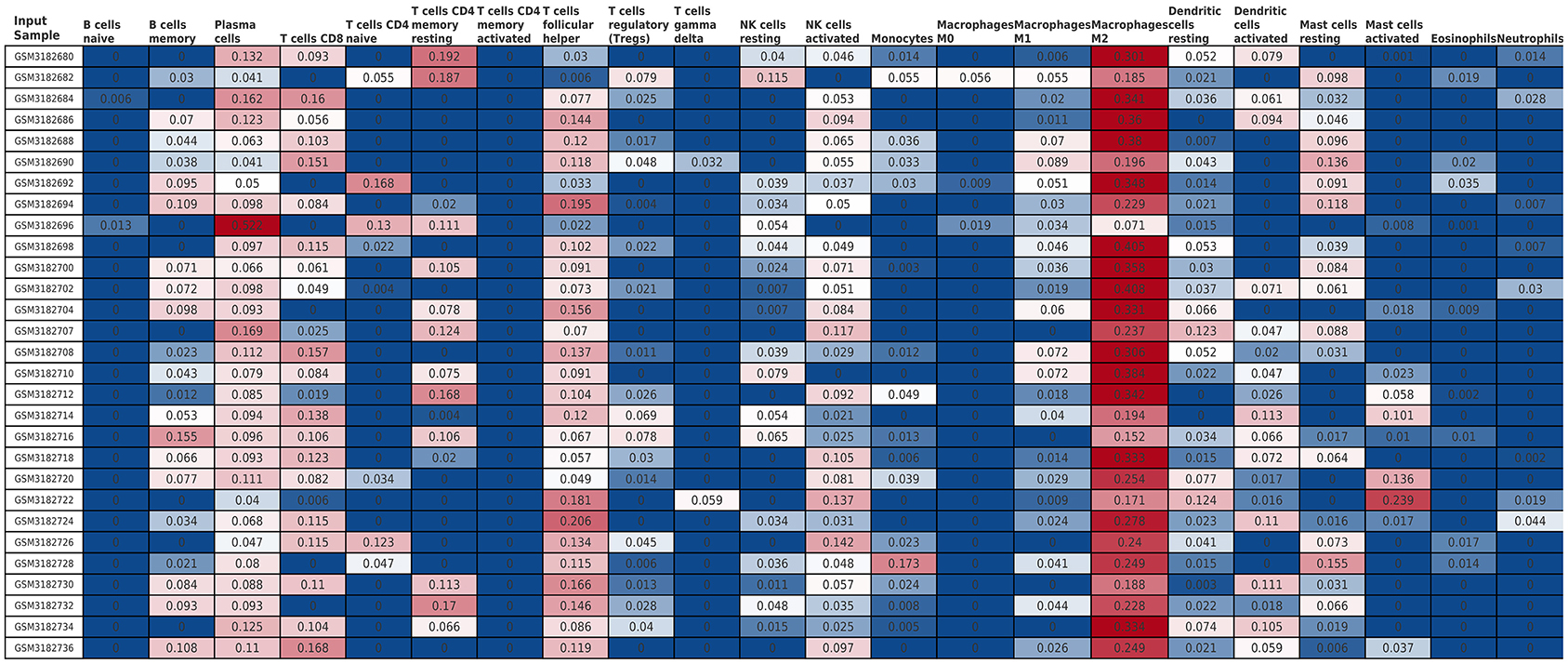
Figure 1. The relative expression of 22 immune cell subtypes in each sample estimated using Cibersort. The relative expression was higher from blue to red.
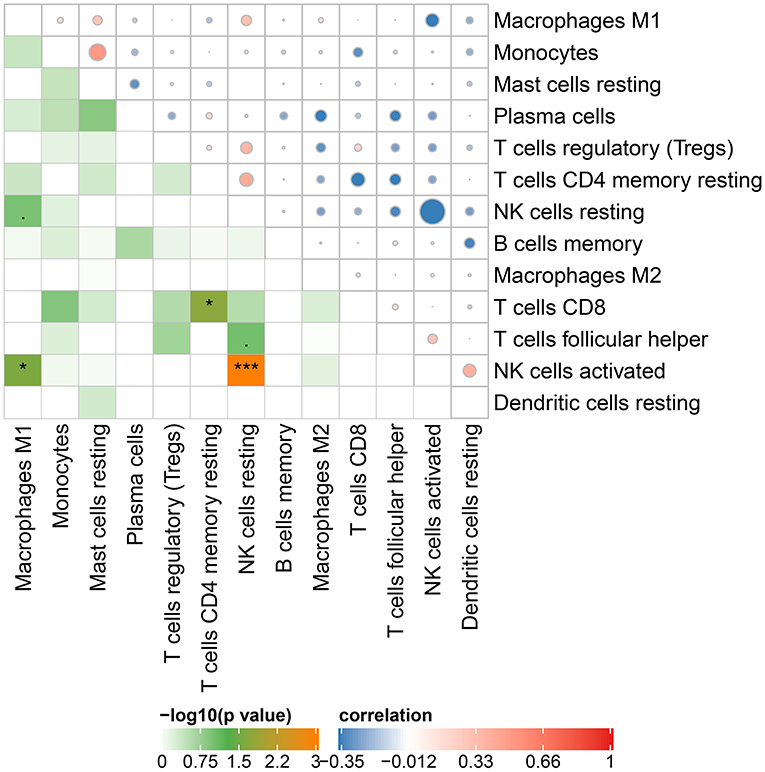
Figure 2. Correlation matrix of 13 immune cell subtype compositions. Blue dots represent negative correlation, and red dots represent positive correlation. The size of the dot is positively correlated with the correlation coefficient. *p < 0.05 and ***p < 0.001.
Construction of the Weighted Coexpression Networks
Based on the DEGs identified above, a total of 2,350 genes were subjected to WGCNA. We then established a scale-free (scale-free R2 > 0.85) coexpression network with the soft-thresholding power β = 8. Using the average linkage hierarchical clustering method, DEGs were clustered into eleven modules with different colors, including black, blue, brown, cyan, greenyellow, gray, lightcyan, magenta, midnightblue, tan, and turquoise (Figure 3).
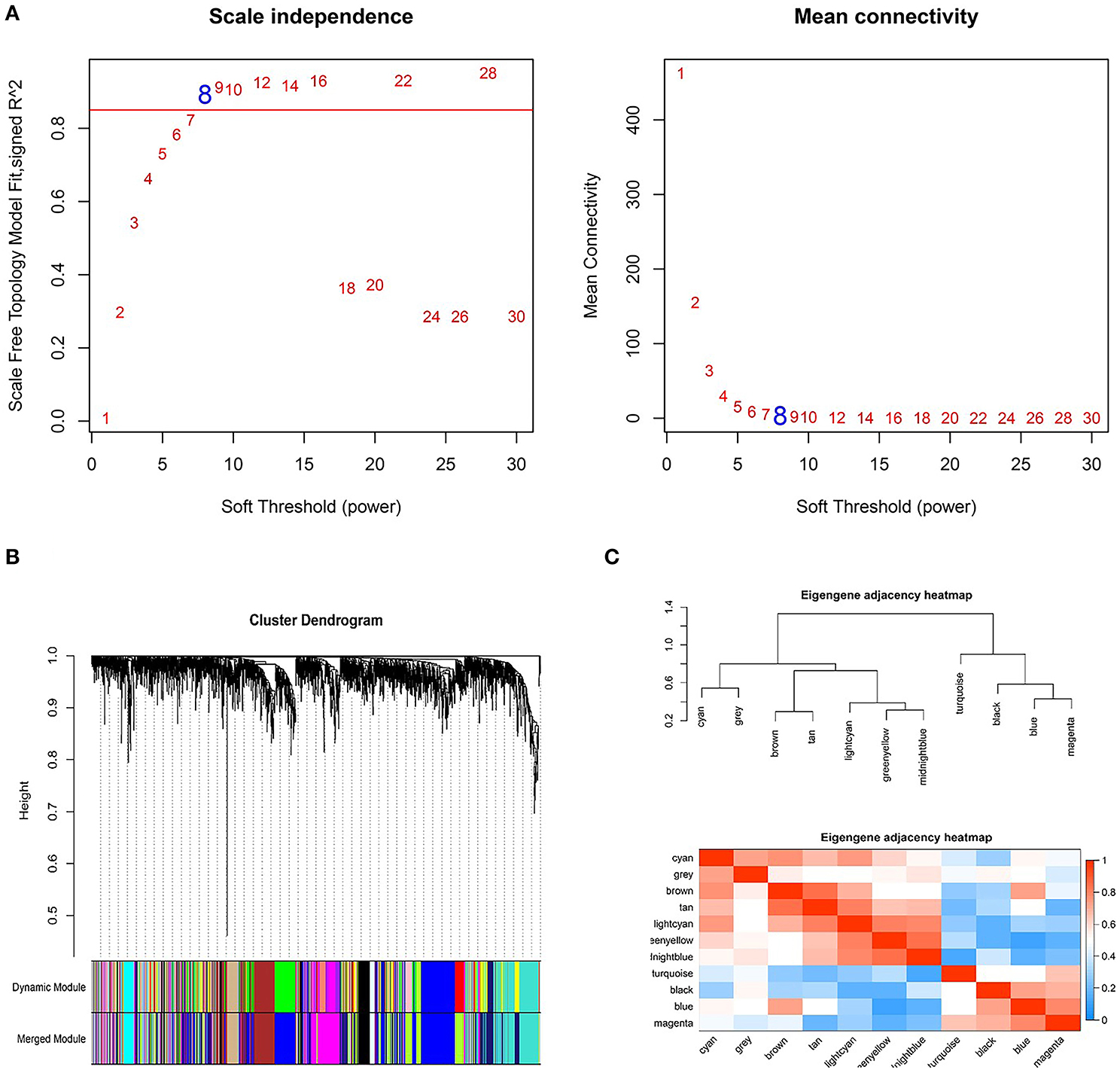
Figure 3. Weighted genes correlation network analysis to cluster genes into different modules. (A) The selection of the soft-thresholding power β. (B) Dendrogram of all differentially expressed genes. (C) The clustering heat map between modules. Red means closer similarity, and blue means farther similarity.
Correlation Between Modules and Immune Cell Types
Correlation analysis was performed between each module and immune cell types selected above, including memory B cells, plasma cells, CD8 T cells, CD4 memory T cells, follicular helper T cells, regulatory T cells (Tregs), resting NK cells, activated NK cells, monocytes, M1 macrophages, M2 macrophages, resting dendritic cells, resting mast cells. The results demonstrated that the magenta module (r = 0.67, p < 0.001) and the blue module (r = 0.58, p = 0.008) were significantly positively correlated with M1 macrophages, while the greenyellow module (r = −0.62, p = 0.004) was significantly negatively correlated with M1 macrophages, as shown in Figure 4. The magenta module with 246 genes was identified as the key module associated with M1 macrophages with the highest correlation coefficient.
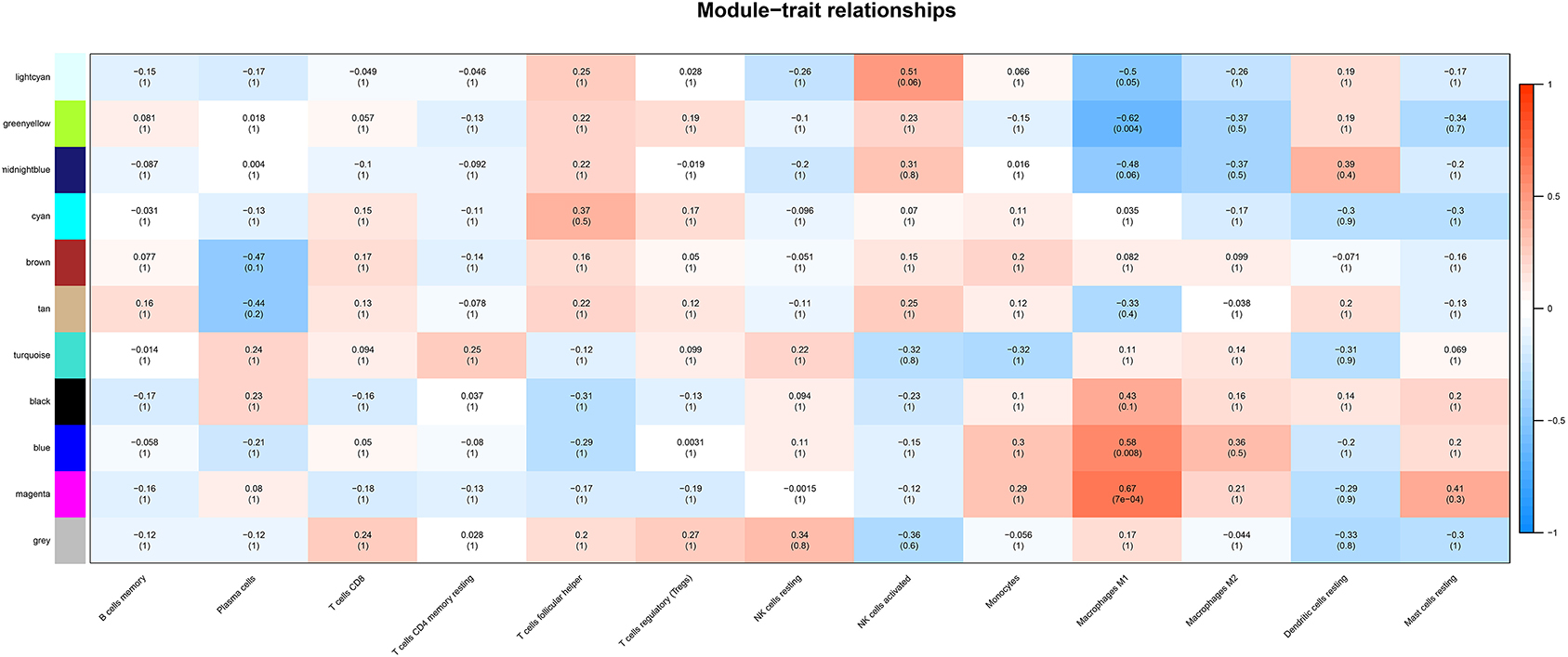
Figure 4. The heatmap showing module-trait correlations. Blue represent negative correlation, and red represent positive correlation. The magenta module had the strongest correlation with M1 macrophages.
Functional Enrichment Analyses
Genes in the magenta module were selected to perform GO and KEGG functional enrichment analyses utilizing the DAVID online tool to investigate the biological effects, as shown in Figure 5. The significant enriched BPs included immune response, ureteric bud development, aorta development, extracellular matrix organization, cyclic nucleotide biosynthetic process, and calcium ion transmembrane transport. In addition, extracellular matrix, extracellular space, extracellular region, basement membrane, and actin filament were significant enriched in CC. For MF, the most significant entries were heparin binding, extracellular matrix structural constituent, calcium channel activity, protein complex binding, and calcium ion binding. Furthermore, the KEGG pathway analysis suggested that DEGs were mainly enriched in staphylococcus aureus infection, MAPK signaling pathway, mTOR signaling pathway, calcium signaling pathway, and complement and coagulation cascades.
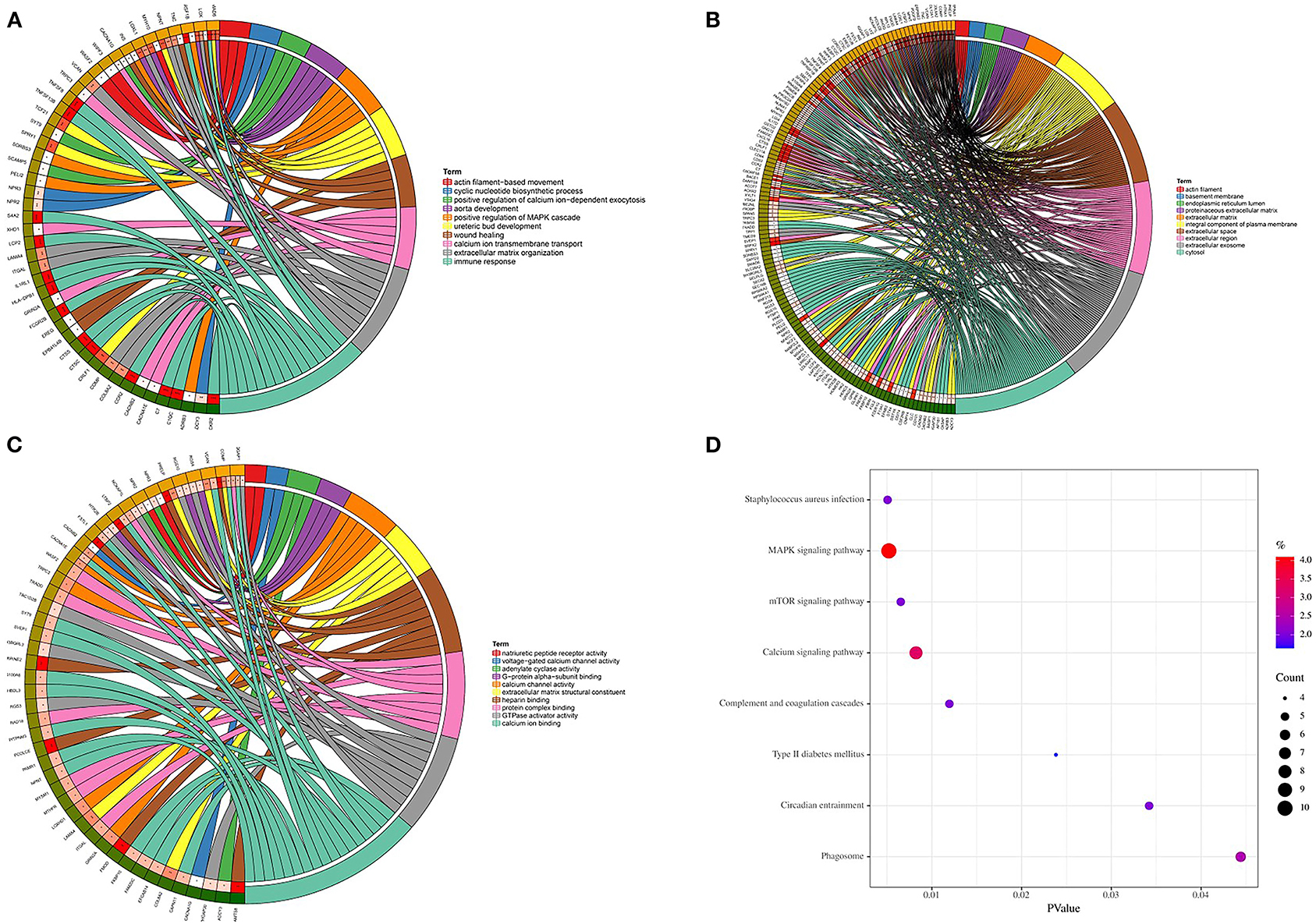
Figure 5. GO and KEGG enrichment analyses. (A) Biological process. (B) Cellular component. (C) Molecular function. (D) KEGG pathways.
Construction of the PPI Network and Hub Genes Identification
DEGs in the magenta module were imported into the STRING online tool to evaluate the interaction between these genes, and a total of 167 nodes and 393 edges were identified from the PPI network, as shown in Figure 6. Five algorithms of the CytoHubba, including MCC, DMNC, MNC, Degree, and EPC, were then applied to process the PPI network to identify the top ten genes, which are shown in Table 2. A Venn diagram (Figure 7) was generated to establish the intersection of genes identified by five algorithms, and CTSS, CSF2RB, and NCF2 were determined as hub genes. These three genes may play considerable roles in the pathophysiology of AF.
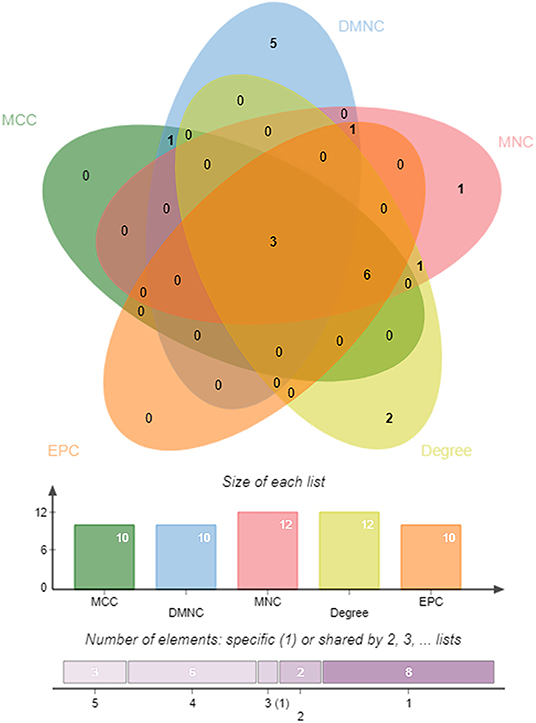
Figure 7. A Venn diagram between five algorithms of CytoHubba. The coincident part represents the three genes (CTSS, CSF2RB, and NCF2) identified by all five algorithms.
Validation of the Hub Genes
The expression levels of three hub genes were detected in LAs and blood samples, respectively, by qRT-PCR. The results showed that the expression levels of CTSS, CSF2RB, and NCF2 in AF were significantly higher than those in SR both in LAs and blood samples, which were consistent with the bioinformatic analysis (Figure 8). Moreover, we combined three datasets, GSE31821, GSE41177, and GSE79768, to verify the expression levels of these three genes between AF and SR. The results also demonstrated that the expression levels of CTSS, CSF2RB, and NCF2 were significantly higher in AF than those in SR (Figure 9).
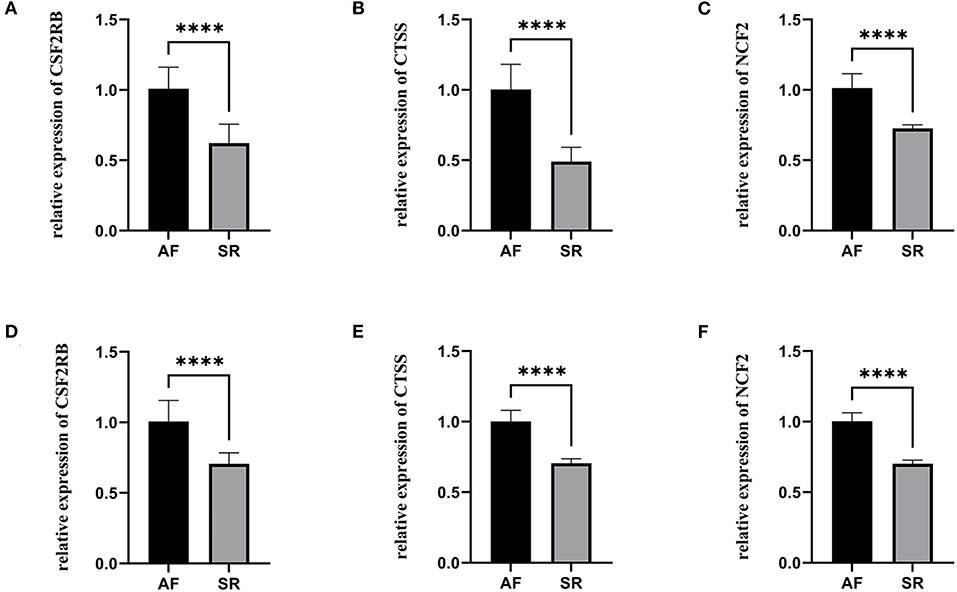
Figure 8. The relative expression of three hub genes between atrial fibrillation (AF) and sinus rhythm (SR). (A–C) The relative expression of CSF2RB, CTSS, and NCF2 in left atriums. (D–F) The relative expression of CSF2RB, CTSS, and NCF2 in blood samples. ****p < 0.0001.
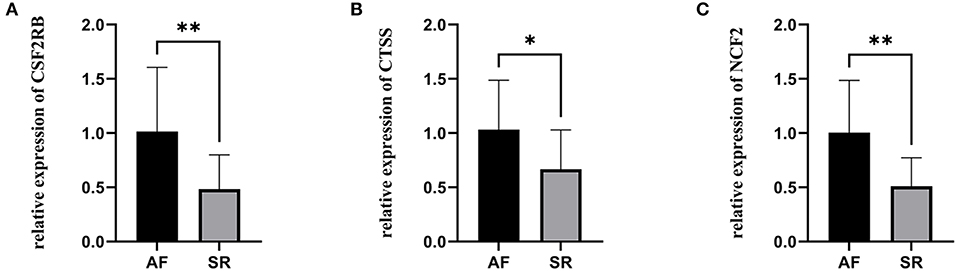
Figure 9. The relative expression of three hub genes verified by three datasets. (A) The relative expression of CSF2RB. (B) The relative expression of CTSS. (C) The relative expression of NCF2. *p < 0.05, **p < 0.01.
Discussion
AF is the most common tachyarrhythmia in the clinic. The typical clinical manifestations of AF are palpitations, fatigue, chest tightness, and decreased exercise tolerance, which seriously affect the life quality of patients (22). AF increases the risk of ischemic stroke by five times higher than healthy people and leads to high morbidity and mortality (23). Despite the fact that lots of efforts have been made, however, the molecular mechanism of AF development is still not completely understood. Therefore, it is significant urgent to clarify the pathogenesis of AF and find potential therapeutic targets.
In this study, we downloaded the GSE115574 dataset from the GEO database and estimated the composition of the immune cells using Cibersort based on the expression matrix. WGCNA was performed to determine the module with the most robust relationship between genes in the module and immune cell types. A total of eleven modules were identified, and the magenta module was significantly correlated with M1 macrophages. To the best of our knowledge, it is the first time that WGCNA has been used to analyze the relationships between immune cell types and AF. We then performed enrichment analysis on genes in the magenta module. According to GO analysis, genes were mainly enriched in immune response, ureteric bud development, aorta development, and extracellular matrix organization. KEGG pathway analysis demonstrated that genes were mainly enriched in staphylococcus aureus infection, MAPK signaling pathway, and mTOR signaling pathway. Based on the PPI network and CytoHubba, we identified three hub genes, including CTSS, CSF2RB, and NCF2. As we know, the relationships between these three genes and the molecular mechanism of AF has not been studied, which is worth further research. Finally, qRT-PCR was performed to verify the expression of CTSS, CSF2RB, and NCF2. The expression levels of these three genes in patients with AF were significantly higher than those in patients with SR, which were consistent with the bioinformatic analysis. The expression levels of these three genes were also verified in three other datasets, GSE31821, GSE41177, and GSE79768.
Previous studies have shown that macrophages are associated with atrial fibrosis, leading to structural remodeling in the process of AF (2, 7, 24). Cytokines released by macrophages such as tumor necrosis factor-α (TNF-α) and interleukin-1β (IL-1β) can activate fibroblast proliferation, leading to fibrous tissue formation (25). However, few studies have investigated the precise role of these cytokines in the molecular mechanism of AF.
CTSS is a lysosomal cysteine proteinase, playing a crucial role in the degradation of antigenic proteins on major histocompatibility complex (MHC) class II molecules (26). CTSS is associated with many inflammatory and autoimmune diseases. CTSS expressed by intimal macrophages was involved in atherogenesis, and deficiency of CTSS could reduce atherosclerosis in LDL receptor-deficient mice (27). miR4498/CTSS might polarize macrophages into pro-inflammatory phenotype and accelerate chronic atherosclerotic inflammation (28). CTSS participated in the abdominal aortic aneurysm (AAA) formation, and inhibition of CTSS suppressed AAA formation in mice (29). A previous study showed that CTSS-mediated induction of CX3CL1 might contribute to the ocular surface and lacrimal glands inflammation in Sjögren's syndrome with a 4.5-fold increase in CX3CR1-expressing macrophages (30). In chronic obstructive pulmonary disease, reduction in CTSS expression prevents loss of lung function, reduces inflammation, and slows the lung tissue remodeling (31). Moreover, CTSS was identified as novel biomarkers for diseases and physiological processes, including triple-negative breast cancer, sarcoidosis, and particulate-induced lysosomal disruption in macrophages (32–34).
CSF2RB is the common beta chain of the high affinity receptor for interleukin-3, interleukin-5, and colony-stimulating factor. The role of CSF2RB has been studied in many diseases. Runt-related transcription factor 1 directly bound to the promoters of CSF2RB, which regulated apoptosis of neuroblastoma (35). The activating hotspot mutation in CSFR2B was identified in myeloid leukemia in Down syndrome (36). The mutation in CSF2RB can also cause hereditary pulmonary alveolar proteinosis (PAP) (37). In CSF2RB-/- mice, statin therapy reduces cholesterol accumulation in alveolar macrophages and ameliorates PAP (38). Moreover, CSF2RB was found overexpressed on monocytes from Alzheimer's disease patients, which contributed to granulocyte-macrophage colony-stimulating factor-induced monocyte migration (39). However, the role of CSF2RB has never been studied in AF. Further research is required to determine whether CSF2RB can become a novel therapeutic target for AF.
NCF2, encoding neutrophil cytosolic factor 2, mainly results in autoimmune diseases. In a previous case-control study, four single-nucleotide polymorphisms within the NCF2 gene were genotyped, and the rs10911362 variants were associated with a decreased TB risk in the Western Chinese Han population (40). NCF2 deficiency resulted in granulomas, and the NCF2 mutation caused diverse and unusual clinical phenotype of chronic granulomatous disease (41, 42). In multiple sclerosis, NCF2 was identified to be associated with eleven single nucleotide polymorphisms (43). Nevertheless, the relationship between NCF2 and AF has not been elucidated.
There are some limitations to our study. First, the data we used was from public databases, which were limited in the sample size. Further prospective studies on more patients should be carried out to support our results. Second, although we have performed qRT-PCR to verify the expression levels of genes, mechanistic studies need to be conducted.
Conclusion
In this study, we performed WGCNA to analyze the relationships between immune cell types and AF for the first time. Three novel genes (CTSS, CSF2RB, and NCF2) which have never been studied in AF were identified. These three genes are worthy of further study and may become potential therapeutic targets in AF.
Data Availability Statement
The dataset presented in this study can be found at https://www.ncbi.nlm.nih.gov/geo/query/acc.cgi?acc=GSE115574, https://www.ncbi.nlm.nih.gov/geo/query/acc.cgi?acc=GSE31821, https://www.ncbi.nlm.nih.gov/geo/query/acc.cgi?acc=GSE41177, and https://www.ncbi.nlm.nih.gov/geo/query/acc.cgi?acc=GSE79768.
Ethics Statement
The studies involving human participants were reviewed and approved by Medical Ethics Committee of Zhongshan Hospital, Fudan University. The patients/participants provided their written informed consent to participate in this study.
Author Contributions
CG and CW conceived and designed this study. TY, SZ, and MZ contributed to data analysis and prepared the main manuscript. All authors reviewed the final manuscript.
Funding
This study was supported by the General Program of the National Natural Science Foundation of China (No. 81770408).
Conflict of Interest
The authors declare that the research was conducted in the absence of any commercial or financial relationships that could be construed as a potential conflict of interest.
Acknowledgments
We thanked Dr. Zhengyang Hu and Qi Wu for their proofreading and valuable advice.
References
1. January CT, Wann LS, Calkins H, Chen LY, Cigarroa JE, Cleveland JC, et al. AHA/ACC/HRS Focused Update of the 2014. AHA/ACC/HRS guideline for the management of patients with atrial fibrillation: a report of the American college of cardiology/American heart association task force on clinical practice guidelines and the heart rhythm society in collaboration with the society of thoracic surgeons. Circulation. (2019) 140:104–32. doi: 10.1161/CIR.0000000000000719
2. Andrade J, Khairy P, Dobrev D, Nattel S. The clinical profile and pathophysiology of atrial fibrillation: relationships among clinical features, epidemiology, and mechanisms. Circ Res. (2014) 114:1453–68. doi: 10.1161/CIRCRESAHA.114.303211
3. Hindricks G, Potpara T, Dagres N, Arbelo E, Bax JJ, Blomström-Lundqvist C, et al. (2020). ESC Guidelines for the diagnosis and management of atrial fibrillation developed in collaboration with the European Association of Cardio-Thoracic Surgery (EACTS). Eur Heart J. (2020). doi: 10.1093/eurheartj/ehaa612. [Epub ahead of print].
4. Camm AJ, Kirchhof P, Lip GY, Schotten U, Savelieva I, Ernst S, et al. Guidelines for the management of atrial fibrillation: the task force for the management of atrial fibrillation of the European Society of Cardiology (ESC). Eur Heart J. (2010) 31:2369–429. doi: 10.1093/eurheartj/ehq278
5. Wyse DG, Van Gelder IC, Ellinor PT, Go AS, Kalman JM, Narayan SM, et al. Lone atrial fibrillation: does it exist? J Am Coll Cardiol. (2014) 63:1715–23. doi: 10.1016/j.jacc.2014.01.023
6. Andrade JG, Aguilar M, Atzema C, Bell A, Cairns JA, Cheung CC, et al. The 2020 Canadian cardiovascular society/Canadian heart rhythm society comprehensive guidelines for the management of atrial fibrillation. Can J Cardiol. (2020) 36:1847–948. doi: 10.1016/j.cjca.2020.09.001
7. Liu Y, Shi Q, Ma Y, Liu Q. The role of immune cells in atrial fibrillation. J Mol Cell Cardiol. (2018) 123:198–208. doi: 10.1016/j.yjmcc.2018.09.007
8. Hernandez MA, Moro C. Atrial fibrillation and C-reactive protein: searching for local inflammation. J Am Coll Cardiol. (2007) 49:1649–50. doi: 10.1016/j.jacc.2007.02.009
9. Liu L, Zheng Q, Lee J, Ma Z, Zhu Q, Wang Z. PD-1/PD-L1 expression on CD(4+) T cells and myeloid DCs correlates with the immune pathogenesis of atrial fibrillation. J Cell Mol Med. (2015) 19:1223–33. doi: 10.1111/jcmm.12467
10. Zhang B, Horvath S. A general framework for weighted gene co-expression network analysis. Stat Appl Genet Mol Biol. (2005) 4:e17. doi: 10.2202/1544-6115.1128
11. Huang Q, Deng G, Wei R, Wang Q, Zou D, Wei J. Comprehensive identification of key genes involved in development of diabetes mellitus-related atherogenesis using weighted gene correlation network analysis. Front Cardiovasc Med. (2020) 7:580573. doi: 10.3389/fcvm.2020.580573
12. Cheng Y, Liu C, Liu Y, Su Y, Wang S, Jin L, et al. Immune microenvironment related competitive endogenous RNA network as powerful predictors for melanoma prognosis based on WGCNA analysis. Front Oncol. (2020) 10:577072. doi: 10.3389/fonc.2020.577072
13. Qu Y, Zhang S, Qu Y, Guo H, Wang S, Wang X, et al. Novel gene signature reveals prognostic model in acute myeloid leukemia. Front Genet. (2020) 11:566024. doi: 10.3389/fgene.2020.566024
14. Chen L, Shi L, Ma Y, Zheng C. Hub Genes Identification in a Murine Model of Allergic Rhinitis Based on Bioinformatics Analysis. Front Genet. (2020) 11:970. doi: 10.3389/fgene.2020.00970
15. Newman AM, Liu CL, Green MR, Gentles AJ, Feng W, Xu Y, et al. Robust enumeration of cell subsets from tissue expression profiles. Nat Methods. (2015) 12:453–7. doi: 10.1038/nmeth.3337
16. Ritchie ME, Phipson B, Wu D, Hu Y, Law CW, Shi W, et al. limma powers differential expression analyses for RNA-sequencing and microarray studies. Nucleic Acids Res. (2015) 43:e47. doi: 10.1093/nar/gkv007
17. Dennis GJ, Sherman BT, Hosack DA, Yang J, Gao W, Lane HC, et al. DAVID: Database for Annotation, Visualization, and Integrated Discovery. Genome Biol. (2003) 4:P3. doi: 10.1186/gb-2003-4-5-p3
18. The Gene Ontology Consortium. The Gene Ontology Resource: 20 years and still going strong. Nucleic Acids Res. (2019) 47:D330–8. doi: 10.1093/nar/gky1055
19. Ashburner M, Ball CA, Blake JA, Botstein D, Butler H, Cherry JM, et al. Gene ontology: tool for the unification of biology the gene ontology consortium. Nat Genet. (2000) 25:25–9. doi: 10.1038/75556
20. Szklarczyk D, Gable AL, Lyon D, Junge A, Wyder S, Huerta-Cepas J, et al. STRING v11: protein-protein association networks with increased coverage, supporting functional discovery in genome-wide experimental datasets. Nucleic Acids Res. (2019) 47:D607–13. doi: 10.1093/nar/gky1131
21. Chin CH, Chen SH, Wu HH, Ho CW, Ko MT, Lin CY. cytoHubba: identifying hub objects and sub-networks from complex interactome. BMC Syst Biol. (2014) 8(Suppl. 4):S11. doi: 10.1186/1752-0509-8-S4-S11
22. Piccini JP, Fauchier L. Rhythm control in atrial fibrillation. Lancet. (2016) 388:829–40. doi: 10.1016/S0140-6736(16)31277-6
23. Virani SS, Alonso A, Benjamin EJ, Bittencourt MS, Callaway CW, Carson AP, et al. Heart disease and stroke statistics-2020 update: a report from the American heart association. Circulation. (2020) 141:e139–596. doi: 10.1161/CIR.0000000000000746
24. Burstein B, Nattel S. Atrial fibrosis: mechanisms and clinical relevance in atrial fibrillation. J Am Coll Cardiol. (2008) 51:802–9. doi: 10.1016/j.jacc.2007.09.064
25. Wynn TA, Ramalingam TR. Mechanisms of fibrosis: therapeutic translation for fibrotic disease. Nat Med. (2012) 18:1028–40. doi: 10.1038/nm.2807
26. Campden RI, Zhang Y. The role of lysosomal cysteine cathepsins in NLRP3 inflammasome activation. Arch Biochem Biophys. (2019) 670:32–42. doi: 10.1016/j.abb.2019.02.015
27. Sukhova GK, Zhang Y, Pan JH, Wada Y, Yamamoto T, Naito M, et al. Deficiency of cathepsin S reduces atherosclerosis in LDL receptor-deficient mice. J Clin Invest. (2003) 111:897–906. doi: 10.1172/JCI200314915
28. Li X, He X, Wang J, Wang D, Cong P, Zhu A, et al. The Regulation of Exosome-Derived miRNA on Heterogeneity of Macrophages in Atherosclerotic Plaques. Front Immunol. (2020) 11:2175. doi: 10.3389/fimmu.2020.02175
29. Lai CH, Chang JY, Wang KC, Lee FT, Wu HL, Cheng TL. Pharmacological inhibition of cathepsin S suppresses abdominal aortic aneurysm in Mice. Eur J Vasc Endovasc Surg. (2020) 59:990–9. doi: 10.1016/j.ejvs.2020.01.008
30. Fu R, Guo H, Janga S, Choi M, Klinngam W, Edman MC, et al. Cathepsin S activation contributes to elevated CX3CL1 (fractalkine) levels in tears of a Sjogren's syndrome murine model. Sci Rep. (2020) 10:1455. doi: 10.1038/s41598-020-58337-4
31. Doherty DF, Nath S, Poon J, Foronjy RF, Ohlmeyer M, Dabo AJ, et al. Protein phosphatase 2A reduces cigarette smoke-induced cathepsin S and loss of lung function. Am J Respir Crit Care Med. (2019) 200:51–62. doi: 10.1164/rccm.201808-1518OC
32. Hughes CS, Colhoun LM, Bains BK, Kilgour JD, Burden RE, Burrows JF, et al. Extracellular cathepsin S and intracellular caspase 1 activation are surrogate biomarkers of particulate-induced lysosomal disruption in macrophages. Part Fibre Toxicol. (2016) 13:19. doi: 10.1186/s12989-016-0129-5
33. Tanaka H, Yamaguchi E, Asai N, Yokoi T, Nishimura M, Nakao H, et al. Cathepsin S, a new serum biomarker of sarcoidosis discovered by transcriptome analysis of alveolar macrophages. Sarcoidosis Vasc Diffuse Lung Dis. (2019) 36:141–7. doi: 10.36141/svdld.v36i2.7620
34. Wilkinson R, Burden RE, McDowell SH, McArt DG, McQuaid S, Bingham V, et al. A novel role for cathepsin S as a potential biomarker in triple negative breast cancer. J Oncol. (2019) 2019:3980273. doi: 10.1155/2019/3980273
35. Hong M, He J, Li D, Chu Y, Pu J, Tong Q, et al. Runt-related transcription factor 1 promotes apoptosis and inhibits neuroblastoma progression in vitro and in vivo. J Exp Clin Cancer Res. (2020) 39:52. doi: 10.1186/s13046-020-01558-2
36. Labuhn M, Perkins K, Matzk S, Varghese L, Garnett C, Papaemmanuil E, et al. Mechanisms of progression of myeloid preleukemia to transformed myeloid leukemia in children with down syndrome. Cancer Cell. (2019) 36:123–38. doi: 10.1016/j.ccell.2019.06.007
37. Arumugam P, Suzuki T, Shima K, McCarthy C, Sallese A, Wessendarp M, et al. Long-term safety and efficacy of gene-pulmonary macrophage transplantation therapy of PAP in Csf2ra(-/-) Mice. Mol Ther. (2019) 27:1597–611. doi: 10.1016/j.ymthe.2019.06.010
38. McCarthy C, Lee E, Bridges JP, Sallese A, Suzuki T, Woods JC, et al. Statin as a novel pharmacotherapy of pulmonary alveolar proteinosis. Nat Commun. (2018) 9:3127. doi: 10.1038/s41467-018-05491-z
39. Shang S, Yang YM, Zhang H, Tian L, Jiang JS, Dong YB, et al. Intracerebral GM-CSF contributes to transendothelial monocyte migration in APP/PS1 Alzheimer's disease mice. J Cereb Blood Flow Metab. (2016) 36:1978–91. doi: 10.1177/0271678X16660983
40. Jiao L, Song J, Ding L, Liu T, Wu T, Zhang J, et al. A novel genetic variation in NCF2, the core component of NADPH oxidase, contributes to the susceptibility of tuberculosis in western Chinese Han population. DNA Cell Biol. (2020) 39:57–62. doi: 10.1089/dna.2019.5082
41. Roth IL, Salamon P, Freund T, Gadot YB, Baron S, Hershkovitz T, et al. Novel NCF2 mutation causing chronic granulomatous disease. J Clin Immunol. (2020) 40:977–86. doi: 10.1007/s10875-020-00820-8
42. van der Weyden L, Speak AO, Swiatkowska A, Clare S, Schejtman A, Santilli G, et al. Pulmonary metastatic colonisation and granulomas in NOX2-deficient mice. J Pathol. (2018) 246:300–10. doi: 10.1002/path.5140
Keywords: atrial fibrillation, WGCNA, immune cells, bioinformatics, hub genes
Citation: Yan T, Zhu S, Zhu M, Wang C and Guo C (2021) Integrative Identification of Hub Genes Associated With Immune Cells in Atrial Fibrillation Using Weighted Gene Correlation Network Analysis. Front. Cardiovasc. Med. 7:631775. doi: 10.3389/fcvm.2020.631775
Received: 20 November 2020; Accepted: 30 December 2020;
Published: 21 January 2021.
Edited by:
Abdelaziz Beqqali, University of Edinburgh, United KingdomReviewed by:
Mingxian Chen, Central South University, ChinaElisa Cairrão, Universidade da Beira Interior, Portugal
Copyright © 2021 Yan, Zhu, Zhu, Wang and Guo. This is an open-access article distributed under the terms of the Creative Commons Attribution License (CC BY). The use, distribution or reproduction in other forums is permitted, provided the original author(s) and the copyright owner(s) are credited and that the original publication in this journal is cited, in accordance with accepted academic practice. No use, distribution or reproduction is permitted which does not comply with these terms.
*Correspondence: Chunsheng Wang, d2FuZ2NodW5zaGVuZ0BmdWRhbi5lZHUuY24=; Changfa Guo, Z3VvLmNoYW5nZmFAenMtaG9zcGl0YWwuc2guY24=
†These authors have contributed equally to this work