- 1Department of Cardiology, Sichuan Provincial People's Hospital, University of Electronic Science and Technology of China, Chengdu, China
- 2Department of Cardiology, West China Hospital, Sichuan University, Chengdu, China
- 3Emergency Department, Sichuan Provincial People's Hospital, University of Electronic Science and Technology of China, Chengdu, China
Atherosclerosis is the pathological basis of many cardiovascular and cerebrovascular diseases. The development of gene chip and high-throughput sequencing technologies revealed that the immune microenvironment of coronary artery disease (CAD) in high-risk populations played an important role in the formation and development of atherosclerotic plaques. Three gene expression datasets related to CAD were assessed using high-throughput profiling. CIBERSORT analysis revealed significant differences in five types of immune cells: activated dendritic cells (DCs), T follicular helper cells (Tfhs), resting CD4+ T cells, regulatory T cells (Tregs), and γδ T cells. Immune transcriptome analysis indicated higher levels of inflammatory markers (cytolytic activity, antigen presentation, chemokines, and cytokines) in the cases than in the controls. The level of activated DCs and the lipid clearance signaling score were negatively correlated. We observed a positive correlation between the fraction of Tfhs and lipid biosynthesis. Resting CD4+ T cells and the activity of pathways related to ossification in bone remodeling and glutathione synthesis showed a negative correlation. Gamma delta T cells negatively correlated with IL-23 signaling activity. GSEA revealed a close association with the inflammatory immune microenvironment. The present study revealed that CAD patients may have an inflammatory immune microenvironment and provides a timely update on anti-inflammatory therapies under current investigation.
Introduction
Coronary artery disease (CAD) leads to myocardial infarction (MI), ischemic cardiomyopathy, and arrhythmia, and it is the main source of cardiovascular morbidity, mortality, and the economic health burden worldwide (1). The past decade of research has provided a deeper understanding of the pathogenesis and treatment of CAD (2). Despite this success, little progress has been made on elucidating the function of the immune microenvironment and its therapeutic Implications (3). Growing evidence suggests that that many aforementioned risk factors, such as smoking habits, obesity, hypertension, insulin resistance, diabetes, stress, and hyperlipidemia are primarily responsible for CAD (4). A significant link exists between the pathophysiology of CAD and inflammatory mediators, immune cells, oxidative stress, lipid infiltration, extracellular matrix, and hormone metabolism (4–8).
High-throughput sequencing technologies examine the role of the immune microenvironment of CAD in high-risk populations in the formation and development of atherosclerotic plaques (APs) (4, 9–12). A growing number of studies indicate that cardiovascular events are determined by multiple biological processes that require different tailored therapeutic approaches, and future therapies for CAD should tackle the residual inflammatory process that statin therapy only partially addresses (13). Inhibitors of specific components of the immune microenvironment in atherosclerosis were developed for considerable treatment (13–15). For example, trials involving anti-cytokine therapy for atherosclerotic CAD targeted interleukin (IL)-1β, which plays numerous roles in atherogenesis, plaque growth, and subsequent rupture (16). Pre-clinical studies also revealed that the inhibition of IL-6 or its receptor achieved atheroprotective effects (17, 18).
Converging lines of evidence support the importance of the immune microenvironment in the initiation, progression, and vulnerability of atherosclerotic plaques. Therefore, we analyzed the immune microenvironment based on three CAD datasets. Several immune infiltration analyses were used to reveal the molecular mechanisms of various factors, such as immune cells, cytokines, chemokines, and abnormally activated pathways, in the occurrence and development of CAD and provide a theoretical basis for anti-atherosclerotic treatment strategies and the prevention of this disease.
Materials and Methods
Data Processing
We collected three datasets related to CAD from the Gene Expression Omnibus database (GEO, https://www.ncbi.nlm.nih.gov/geo/) (19), for analysis using the GEOquery R package (20). The GSE40231 dataset based on the GPL570 platform included samples from 40 CAD patients (40 atherosclerotic aortic walls vs. internal mammary arteries) and was used to examine differences in immune filtration in APs. The GSE20681 dataset, based on GPL4133, included 99 whole blood samples from CAD patients and 99 whole blood samples from healthy controls. The GSE20681 samples were paired based on case/control status, age, and gender. GSE20680 included whole blood samples from 143 CAD patients and 52 healthy individuals and were both based on GPL4133. We used normalizeBetweenArrays of the limma R package to the normalize expression data in GSE40231, GSE20681, and GSE20680 (21). Figure 1A shows the workflow of our study.
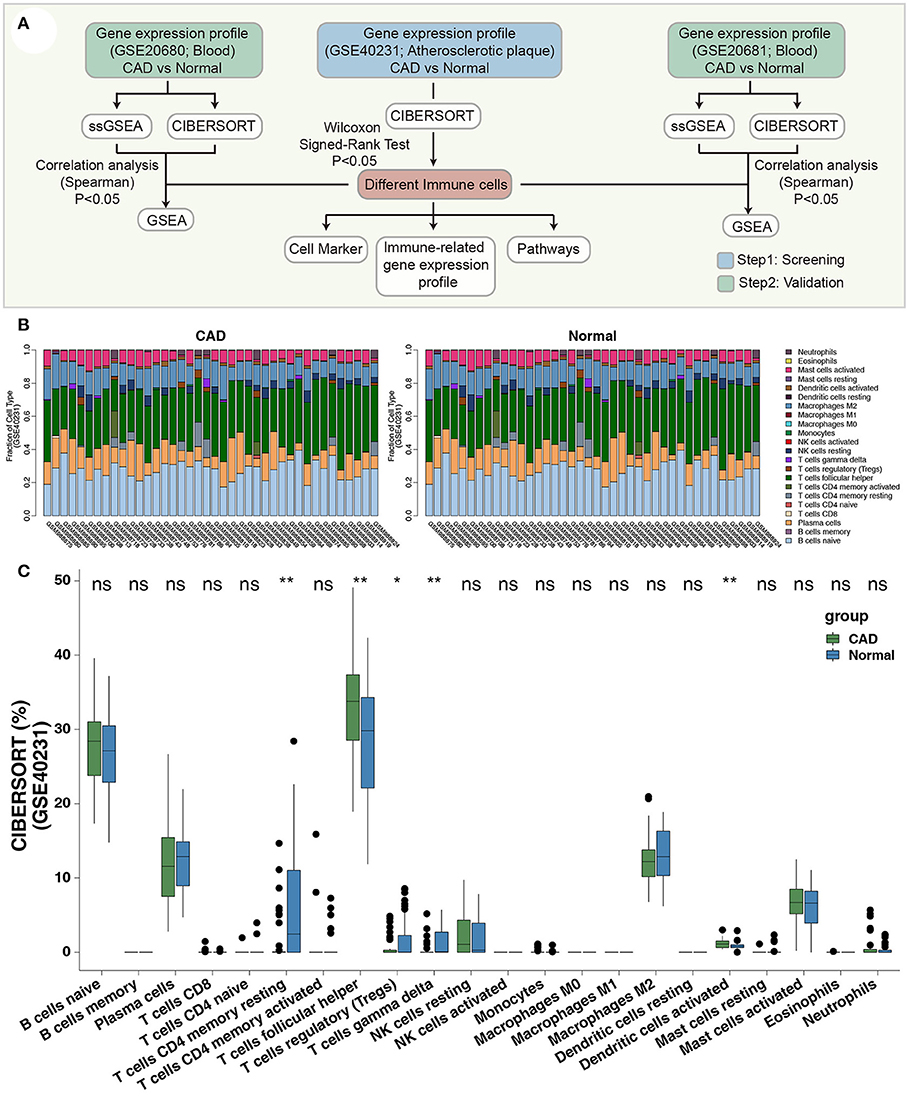
Figure 1. (A) The workflow of the present study. (B) The bar plot indicates the proportions of the 22 immune cells of each sample in GSE40231. (C) The proportions of immune cells of the CAD cases vs. the healthy controls in GSE40231 using CIBERSORT analysis. Wilcoxon signed-rank test (paired): **P < 0.01; *P < 0.05. CAD, coronary artery disease.
Immune Profiling Based on the CIBERSORT Algorithm
The CIBERSORT algorithm uses the LM22 gene sets to estimate the proportion of 22 infiltrating immune cells in samples based on the expression data (22). We used this algorithm based on the default parameters to calculate the relative proportion of 22 infiltrating immune cells in our samples.
We also used this LM22 gene set to compare differences in the detailed gene expression of the significantly altered immune cells. The genes related to cytolytic activity (CYT), antigen presentation, chemokines, and cytokines in CAD identified in previous studies were used to evaluate differences in immune function between the CAD and control groups (23, 24).
Evaluation of the Abnormal Signaling Signature Based on Single-Sample Gene Set Enrichment Analysis (ssGSEA) and Gene Set Enrichment Analysis (GSEA)
The abnormal signaling score was calculated from expression data (GSE40231, GSE20681, and GSE20680) using the ssGSEA algorithm and the GSVA R package (25–27). Gene sets of pathways were collected from the MsigDB database (28). The limma R package was used to calculate the difference in gene expression (logFC) between the CAD and control groups in this study (21). GSEA was performed using the “clusterProfiler” R package and the Molecular Signatures Database (MSigDB) to annotate the dataset, and Gene Ontology (GO), Kyoto Encyclopedia of Genes and Genomes (KEGG) and Reactome terms were considered significant at P < 0.05 (29).
Statistical Analysis
The Wilcoxon signed-rank test (paired) was used to compare differences in the relative proportion of infiltrating immune cells and differences in the immune-related gene expression profile (GEP) between the CAD and control groups. P < 0.05 was considered statistically significant, and all statistical tests were two-sided. R software (version 3.6) was used for statistical analyses. The R package ComplexHeatmap was used to visualize the heatmap (30). The R package ggplot2 was used to create the violin plot (31). The corrplot R package was used to visualize the correlation heatmap (32).
Results
Immune Cells in CAD Cases
To identify a robust immune infiltrating pattern, we normalized three gene expression datasets (GSE40231, GSE20681, and GSE20680) based on the normalizeBetweenArrays method of the limma R package (Supplementary Figure 1). The CIBERSORT algorithm was used to calculate the proportion of 22 immune cells based on the expression data of the discovery dataset (GSE40231; Figure 1B). Notably, the fractions of activated DCs and Tfhs were significantly higher in the CAD than in the control groups (Figure 1C). In contrast, the CAD group had fewer Tregs, γδ T cells, and resting CD4+ T cells (Figure 1C) than the control group. These results suggest that CAD exhibits inflammatory microenvironment patterns.
Different GEPs Related to Immune Cells, CYT, Antigen Presentation, Chemokines, and Cytokines
We focused on the comparison of five differential immune cell markers between the CAD and control groups. Two cell types in CAD (activated DCs and Tfhs) exhibited increased expression levels of immune cell-related genes (Figures 2A,B; all Wilcoxon signed-rank Ps < 0.05). Tregs, γδ T cells, and resting CD4+ T cells in the control group had low expression levels of immune cell-related genes (Figures 2C–E; all Wilcoxon signed-rank Ps < 0.05). We performed the Wilcoxon signed-rank test on the expression levels of genes related to immune function between the CAD and control groups and found that the GEPs related to CYT (CD8A), antigen presentation (HLA-A, HLA-DQA1, HLA-DQA2, and HLA-DQB1), chemokines (CCL5, CX3CL1, and CXCL10), and cytokines (IFNA1 and IFNA2) were significantly stronger in the CAD group than in the control group (all Wilcoxon signed-rank Ps < 0.05).
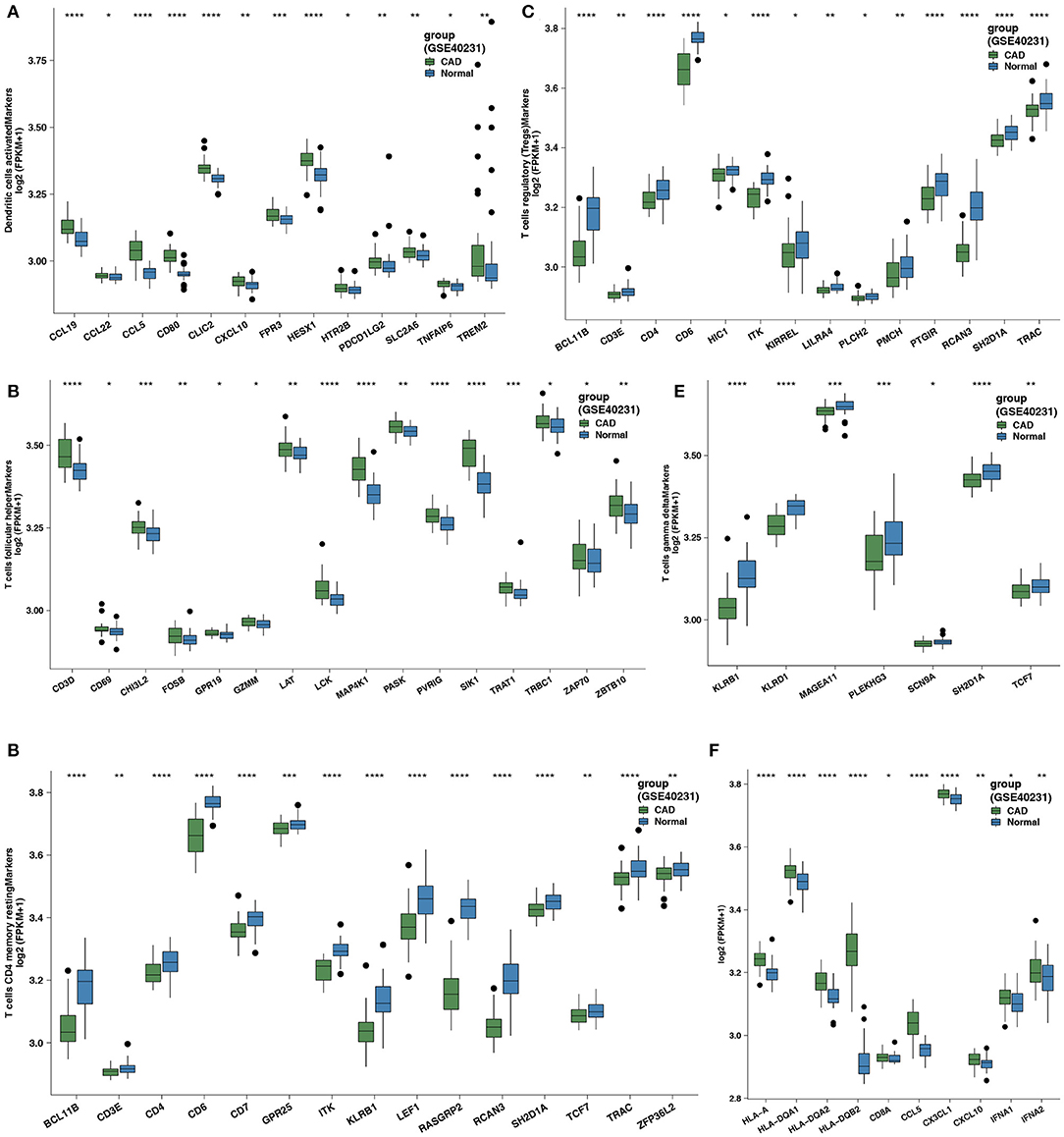
Figure 2. (A) Comparison of the expression of cell markers of activated DCs between the CAD cases vs. the healthy controls in GSE40231. (B) Comparison of the expression of cell markers of Tfhs between the CAD cases vs. the healthy controls in GSE40231. (C) Comparison of the expression of cell markers of Tregs between the CAD cases vs. the healthy controls in GSE40231. (D) Comparison of the expression of cell markers of resting CD4+ T cells between the CAD cases vs. the healthy controls in GSE40231. (E) Comparison of the expression of cell markers of γδ T cells between the CAD cases vs. the healthy controls in GSE40231. (F) Comparison of the expression of CYT-related, antigen-presentation, and proinflammatory-related genes (chemokine and cytokine) between the CAD cases vs. the healthy controls in GSE40231. DCs, dendritic cells; CAD, coronary artery disease; CYT, cytochrome; Tfhs, T follicular helper cells; Tregs, regulatory T cells; γδ T cells, gamma delta T cells. Wilcoxon signed-rank test: ****P < 0.0001; ***P < 0.001; **P < 0.01; *P < 0.05.
Identification of Abnormal Pathways Related to Five Immune Cell Types in CAD
To uncover the different signaling signatures between the CAD and control groups, we performed ssGSEA to calculate the scores of the pathways of each sample using the GSVA R package. Spearman's correlation analysis was performed to determine the relationship between the score of the ssGSEA and the fraction of each of the five immune cells. The proportion of activated DCs and the activity of lipid clearance–related signatures, including LDL and plasma lipoprotein clearance pathways (Figure 3A), showed a significant negative correlation (Spearman's R = −0.23; P = 0.04, and R = −0.23; P = 0.04, respectively; Figure 3A). Patients with a high proportion of activated DCs showed lower activity of the negative regulation of muscle hypertrophy (Figure 3A). To further explore whether activated DCs negatively correlated with pathways related to lipid clearance/muscle hypertrophy, we performed the above analyses in two datasets (GSE26081 and GSE26080). Notably, the content of activated DCs also negatively correlated with the signature of lipid clearance and muscle hypertrophy (Supplementary Figure 2A). The increase in Tfhs significantly correlated with the upregulated lipid biosynthetic process (Spearman's R = 0.3; P = 0.0073; Figure 3B). In contrast, this increase negatively correlated with the negative regulation of cellular extravasation (Spearman's R = −0.26; P = 0.022; Figure 3B). The score for negative regulation of glucocorticoid metabolic process negatively correlated with an increased proportion of Tfhs (Spearman's R = −0.24, P = 0.31; Figure 3B). These results showed a similar trend in these datasets (GSE26081 and GSE26080; Supplementary Figure 2B). Supplementary Figures 2C,D shows the correlation between the fractions of the two immune cell types and the activity of abnormal pathways. Overall, these results further indicated that CAD was associated with increased activation of DCs and Tfhs, the downregulated activity of lipid clearance and glucocorticoid metabolism, and the upregulated activity of cellular extravasation (Figure 3C). Notably, the proportion of Tregs and the score of these pathways, including metabolism of angiotensinogen to angiotensins, lipopolysaccharide, and smooth muscle cell migration, exhibited negative Spearman's correlations (R = −0.27, P = 0.017; R = −0.23, P = 0.036; and R = −0.25, P = 0.024, respectively; Figure 4A). We observed that the increased resting CD4+ T cells negatively correlated with the activity of some pathways related to ossification in bone remodeling and glutathione synthesis (R = −0.32; P = 0.0033; R = −0.34; P = 0.036; R = −0.25; P = 0.024; Figure 4B). There was a negative correlation between the fraction of γδ T cells and inflammation-related pathways, such as IL-23 signaling (R = −0.25; P = 0.025; Figure 4C) and NOTCH2 signaling (R = −0.25; P = 0.027; Figure 4C). Spearman correlation analysis showed similar results between these immune cell types (Tregs, resting CD4+ T cells and γδ T cells) and abnormal signaling signatures (Figure 4D and Supplementary Figures 4A–C). These results demonstrated that differentially abundant immune cells that are associated with abnormal signaling signatures may play an important role in the pathogenesis of CAD.
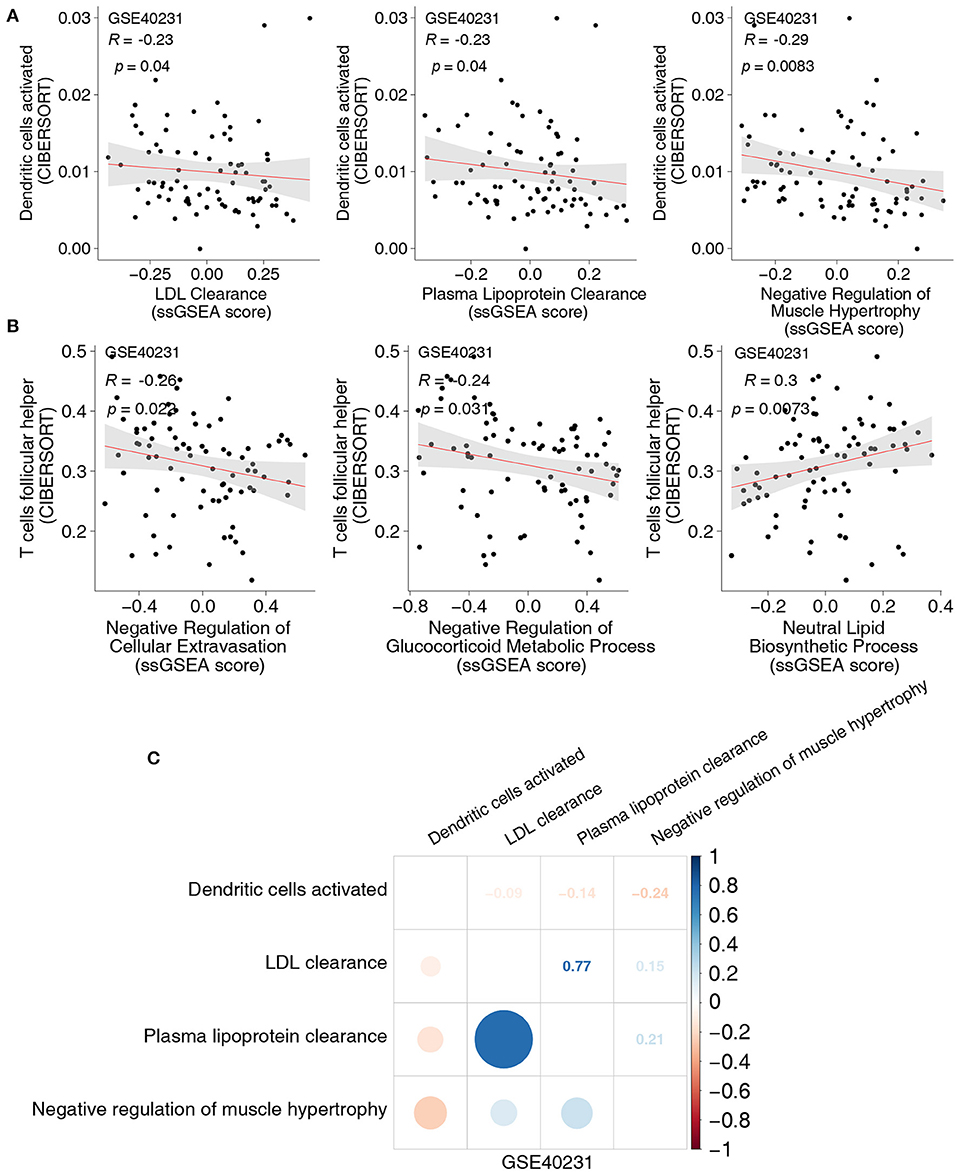
Figure 3. (A) The correlation between the proportions of activated DCs and the score of ssGSEA in GSE40231. (B) The correlation between the proportions of Tfhs and the score of ssGSEA in GSE40231. (C) The correlation heatmap of the proportions of activated DCs and the score of ssGSEA in each three-gene expression dataset (GSE40231). DCs, dendritic cells; Tfhs, T follicular helper cells; ssGSEA, enrichment analysis.
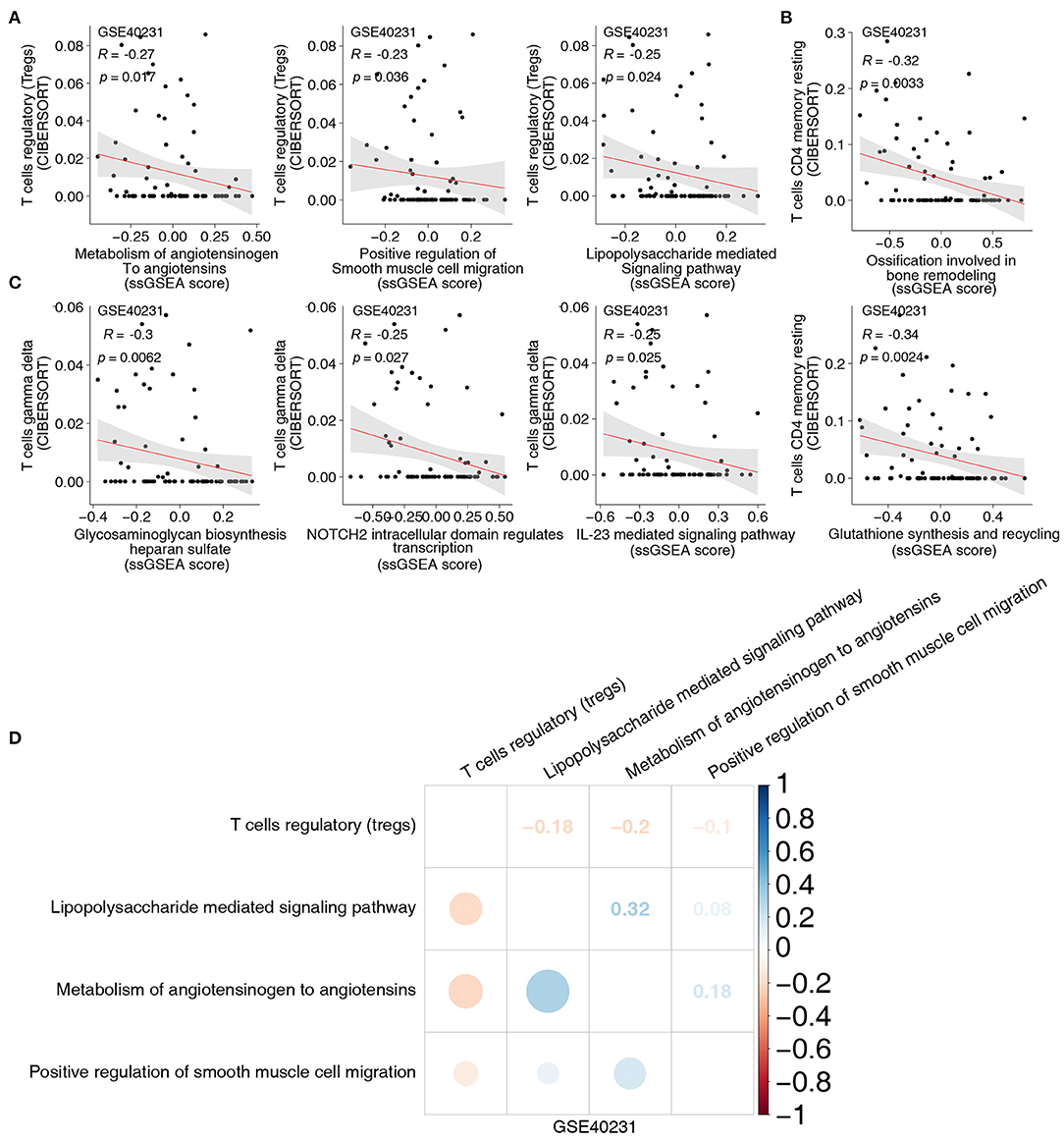
Figure 4. (A) The correlation between the proportions of Tregs and the score of ssGSEA. (B) The correlation between the proportions of resting CD4+ T cells and the score of ssGSEA. (C) The correlation between the proportions of γδ T cells and the score of ssGSEA. (D) The correlation heatmap of the proportions of Tregs and the score of ssGSEA in each three-gene expression dataset (GSE40231). Tregs, regulatory T cells; ssGSEA, enrichment analysis; γδ T cells, gamma delta T cells.
Identification of Differential GEPs and Key Pathways in CAD
We extracted the GEPs of hub genes in key pathways (related to Figures 3, 4) and compared these GEPs between the CAD and control groups in GSE40231. Genes related to lipid biosynthesis were significantly upregulated in the CAD group compared to the control group, and genes associated with lipid clearance signaling were significantly downregulated in the CAD group compared to the control group (Figure 5A). GSEA in three independent datasets indicated that immune cell-related signatures, such as natural killer cells (NKs), B cells, myeloid leukocytes, neutrophils, Th2, mononuclear cells, and CD8+ T cells were significantly enriched in the CAD group compared to the control group in all datasets (Figure 5B). Similarly, cytokine-related pathways, such as IL-1, IL-4, IL-6, IL-8, IL-13, IL-18, and IFN-γ signaling were significantly enriched in the CAD samples compared to the control group (Figure 5C). Endothelial cell morphogenesis, regulation of glomerular filtration, ROS metabolic process, insulin resistance, positive regulation of vascular smooth muscle cell proliferation, ERK/MAPK targets, and regulation of angiotensin levels in blood were significantly upregulated in the CAD group (Figure 5D).
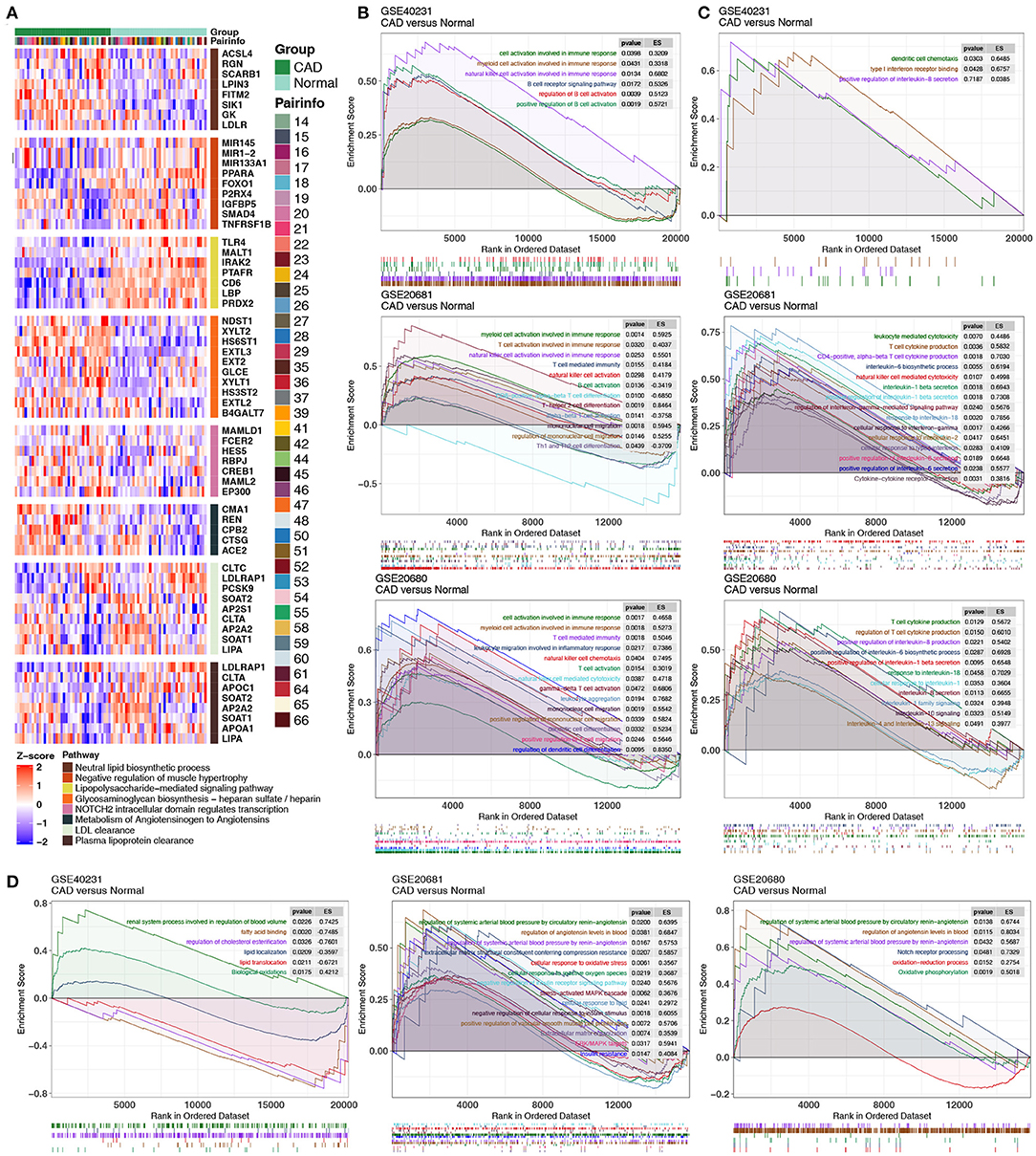
Figure 5. (A) Heatmap of core genes in significantly enriched pathways between the CAD cases vs. the healthy controls in GSE40231. (B–D) GSEA of hallmark gene sets in the CAD cases and the healthy controls downloaded from MSigDB (GSE40231, GSE20681, and GSE20680). CAD cases were associated with activated immune cell- (B), cytokine- (C), and inflammation-related pathways (D). All transcripts were ranked as the log2 (fold-change) between the CAD cases and the healthy controls. Each run was performed with 1,000 permutations. CAD, coronary artery disease; MsigDB, Molecular signatures database.
Discussion
We analyzed gene expression data of CAD patients and healthy controls from three independent datasets—a discovery dataset (GSE40231) and two validation datasets (GSE20681 and GSE60280)—to examine the immune microenvironment of coronary artery disease (CAD). CIBERSORT analysis revealed significant differences in five immune cells: activated DCs, Tfhs, resting CD4+ T cells, Tregs, and γδ T cells. Cell markers of these immune cells and immune-related functional genes were used for downstream analyses. Immune transcriptome analysis indicated that higher expression levels of inflammatory markers (CYT, antigen presentation, chemokines, and cytokines) were detected in the CAD group than in the controls. The ssGSEA algorithm based on the GSVA R package was used to calculate the activity of pathways that may be associated with the pathogenesis of CAD. The proportion of activated DCs and the lipid clearance signaling score showed significant negative correlations. We also observed a positive correlation between the fraction of Tfhs and the lipid biosynthetic signature. Spearman's correlation analysis revealed that the increased proportion of Tregs correlated with the downregulated activity of some pathways, such as metabolism of angiotensinogen to angiotensins, lipopolysaccharide, and smooth muscle cell migration. Notably, resting CD4+ T cells and the activity of some pathways related to ossification in bone remodeling and glutathione synthesis showed a negative correlation. The γδ T cells negatively correlated with IL-23 signaling activity. GSEA revealed a close association between the inflammatory immune microenvironment, including activated immune cells, chemokines and cytokines, and CAD. Therefore, we identified the immune microenvironment associated with inflammatory signaling based on the expression profiles of CAD cases. We summarized the possible mechanisms underlying the immune microenvironment in CAD (Figure 6).
DCs are the most powerful known antigen presenting cells and are a key link between innate immunity and acquired immunity. DCs play an important role in the occurrence and development of atherosclerosis (33). The release of proinflammatory cytokines, such as CCL5, CX3CL1, and CXCL10 promotes the migration of DCs (34–36). Mature and activated DCs accumulate locally with the progression of Aps (37). Liu et al. reported that the decreased expression of CX3CR1 and CX3CL1 and the downregulation of DCs narrowed plaques in CX3CR1−/−ApoE−/− mouse models compared to healthy controls (38). The migration of DCs may also be impaired in hyperlipidemia (39), but the ability to stimulate these is not weakened (40), which may lead to a local accumulation of DCs and ultimately increase the inflammatory response, DC maturation, T cell activation, cytokine secretion (IL-1, IL-6, IL-8, INF-γ, and TNF), and TNF-α participation in the formation of atherosclerosis (41). Several recent trials focused on pro-inflammatory mediators in patients with CAD. The CANTOS (Canakinumab Anti-Inflammatory Thrombosis Outcome Study) trial was the most noteworthy of the studies and targeted IL-1β (42). The CANTOS trial provided favorable evidence that inhibition of the IL-1β/IL-6 signaling cascade led to a significant reduction in cardiovascular risk, independent of a lipid-lowering effect, but with an increased risk of serious infection (42). Experimental data in hyperlipidemic mice also revealed that inhibition of IL-6 or its receptor achieved atheroprotective effects (17). DCs may play an important role in the occurrence and development of atherosclerosis via lipid accumulation. Paulson et al. investigated the formation of early APs in Ldlr−/− mice using immunofluorescence (43). After a high cholesterol diet, lipid accumulation in the blood vessels of CD11c+ DCs was induced. These DCs exhibited foam cell morphology and may participate in the early formation of plaques. The antigen presentation function of DCs plays an important role in the occurrence and development of atherosclerosis (44). MHC-I and MHC-II are known antigen-presenting molecules of DCs (44). DCs present antigens to T cells via MHC-I and MHC-II, which leads to the activation and proliferation of T cells (45). A previous study found that atherosclerosis was significantly decreased in Ldlr−/−CD74−/− mice, and the activated T cells were also decreased. This finding may be related to the decrease in the presenting function of the DCs (46). DCs mainly take up and present specific atherosclerotic antigens in atherosclerosis. Arterial DCs activate DCs via ox-LDL uptake and increase the presentation of lipid and polypeptide antigens to T cells, thereby participating in the development of atherosclerosis (44). DCs regulate the process of atherosclerosis by controlling the activation of T cells (47). Koltsova et al. observed that DCs in ApoE−/−CD11c–YFP+ mice interacted with CD4+ T cells and resulted in T cell activation, proliferation, and the secretion of TNF-α and IFN-γ, which accelerated the process of atherosclerosis (47). Foam cells are one of the hallmarks of atherosclerotic plaques and develop when smooth muscle cells within the arterial wall take up ox-LDL via scavenger receptors (48). Consistent with previous findings (47), the results of the present study also suggest that activated DCs are associated with downregulated lipid clearance, increased antigen presentation, activation of cytotoxic responses, recruitment, and migration. Therefore, a critical protection mechanism toward avoiding immune cell infiltration into the vessel wall stems from wall-embedded DCs (49).
Tregs are a negative immune regulator that play an important anti-atherosclerotic role via inhibition of the autoimmune response and the maintenance of homeostasis of the body's immune response (50–52). Wigren et al. reported that individuals with low levels of Tregs were at increased risk of a first coronary event (53).
Hermansson et al. showed that an increase in Tregs was associated with a significant decrease in AP size (~70%), and a decrease in CD4+ T cell infiltration and systemic inflammation was observed (54). A previous study investigating the distribution of Tregs in human atherosclerotic lesions found that low numbers of Tregs were present during all developmental stages (55). Our data were consistent with the observation of the deficiency of Tregs in the experimental atherosclerosis model (56–58) and suggest decreased CD4+CD25+ Treg participation in plaque destabilization.
Tfhs cells promote B cell proliferation, differentiation, class conversion, and antibody production and the occurrence and development of atherosclerosis. Taghaviemoghadam et al. found decreased Tfhs and plasma cells in the spleen and aortic arch of STAT4−/−Ldlr−/− mice compared to Ldlr−/− mice, but CD8+ Tregs were increased, and the size of the APs decreased (59). A recent study showed that the expression of Foxp3 in Tregs and the immunosuppressive function were lost during the process of atherosclerosis, which resulted in the transformation of some Tregs into Tfhs. Tfhs promote atherosclerosis, and Tfh depletion alleviates atherosclerosis, and ApoAI prevents Tregs from transforming into Tfhs, which affects the development of atherosclerosis (60). Tfhs in the peripheral blood of CAD patients are significantly higher than healthy populations and have a stronger proinflammatory function. An in vitro co-culture revealed a significant increase in the expression of INF-γ, IL-17, and IL-21 in coronary heart disease patients, and an increase in the expression level of B cell inflammatory genes (61). Our results suggest that CAD patients have a decreased proportion of γδ T cells, which negatively correlated with IL-23 signaling. A previous study showed that the expression level of IL-23 in the peripheral blood of CAD patients was significantly higher than that in healthy people. The high expression level of IL-23 was associated with a higher mortality of CAD patients (5). These results provide potential targets of inflammation to improve outcomes in atherosclerotic cardiovascular disease.
Although the findings in the present study systematically summarized the immune microenvironment of CAD cases based on their expression profiles using multiple bioinformatics methods, the results should be validated in prospective studies with larger populations. The present study also had some limitations. First, cell experiments and animal experiments are lacking. Second, this study was based solely on gene expression arrays (bulk transcriptome). All datasets in this study lacked clinical data, which may have potential confounders. This report lacks other omics analyses to further validate our results, and the traditional bulk array may obscure the heterogeneity of various immune cells in CAD. Third, there were limited tissue data for CAD, and the present study used two blood dataset and one tissue dataset of CAD to further validate the immune microenvironment. Fourth, the related clinical characteristics are lacking, a subgroup analysis will be conducted in the future using prospective cohorts.
Conclusions
The present study revealed that CAD patients may have an inflammatory immune microenvironment. The study also provides a timely update on inflammatory components in the immune microenvironment of patients with CAD.
Data Availability Statement
The original contributions presented in the study are included in the article/Supplementary Material, further inquiries can be directed to the corresponding author/s.
Author Contributions
Conceptualization, formal analysis, supervision, and visualization: HKH and RSD. Software: HKH, RSD, and PC. Writing–original draft: HKH, RSD, PC, JCZ, YC, and GL. Writing–review & editing: HKH, RSD, YC, and GL. All authors contributed to the article and approved the submitted version.
Conflict of Interest
The authors declare that the research was conducted in the absence of any commercial or financial relationships that could be construed as a potential conflict of interest.
Acknowledgments
We thank Wenjie Tian gave writing assistance and technical editing.
Supplementary Material
The Supplementary Material for this article can be found online at: https://www.frontiersin.org/articles/10.3389/fcvm.2020.602345/full#supplementary-material
Supplementary Figure 1. The boxplot of gene expression profiles in each of the three datasets before and after normalization.
Supplementary Figure 2. The correlation between the proportions of activated DCs and the score of ssGSEA in the two validation GEO datasets (GSE20681 and GSE20680). The correlation between the proportions of Tfhs and the score of ssGSEA in each validation GEO dataset (GSE20681 and GSE20680). The correlation heatmap between the proportions of activated DCs and the score of ssGSEA in the two validation GEO datasets (GSE20681 and GSE20680). The correlation heatmap between the proportions of Tfhs and the score of ssGSEA in each validation GEO dataset (GSE20681 and GSE20680). DCs, dendritic cells; ssGSEA, enrichment analysis; GEO, Gene Expression Omnibus database; Tfhs, T follicular helper cells.
Supplementary Figure 3. The correlation between the proportions of Tregs and the score of ssGSEA in the two validation GEO datasets (GSE20681 and GSE20680). The correlation between the proportions of resting CD4+ T cells and the score of ssGSEA in the two validation GEO datasets (GSE20681 and GSE20680). The correlation between the proportions of γδ T cells and the score of ssGSEA in GSE20681. Tregs, regulatory T cells; ssGSEA, enrichment analysis; GEO, Gene Expression Omnibus database; γδ T cells, gamma delta T cells.
Supplementary Figure 4. The correlation heatmap between the proportions of Tregs and the score of ssGSEA in the two validation GEO datasets (GSE20681 and GSE20680). The correlation heatmap between the proportions of resting CD4+ T cells and the score of ssGSEA in the two validation GEO datasets (GSE20681 and GSE20680). The correlation heatmap between the proportions of γδ T cells and the score of ssGSEA in GSE20681. ssGSEA, enrichment analysis; GEO, Gene Expression Omnibus database; γδ T cells, gamma delta T cells.
Abbreviations
CAD, coronary artery disease; DCs, dendritic cells; Tfhs, T follicular helper cells; Tregs, regulatory T cells; AS, Atherosclerosis; APs, atherosclerotic plaques; TNF, tumor necrosis factor; ROS, reactive oxygen species; GEO, Gene Expression Omnibus database; CYT, cytolytic activity; ssGSEA, single-sample gene set enrichment analysis; GSEA, gene set enrichment analysis; GEP, gene expression profile; γδ T cells, gamma delta T cells; NKs, natural killer cells; LDL, low density lipoprotein.
References
1. Global, regional, and national age-sex specific all-cause and cause-specific mortality for 240 causes of death 1990-2013: a systematic analysis for the Global Burden of Disease Study 2013. Lancet. (2015) 385:117–1. doi: 10.1016/S0140-6736(14)61682-2
2. Nikpay M, Soubeyrand S, Tahmasbi R, McPherson R. Multiomics screening identifies molecular biomarkers causally associated with the risk of coronary artery disease. Circul. Genomic Prec Med. (2020) 13:e002876. doi: 10.1161/CIRCGEN.119.002876
3. Peng X-Y, Wang Y, Hu H, Zhang X-J, Li Q. Identification of the molecular subgroups in coronary artery disease by gene expression profiles. J Cell Physiol. (2019) 234:16540–16548. doi: 10.1002/jcp.28324
4. Fioranelli M, Bottaccioli AG, Bottaccioli F, Bianchi M, Rovesti M, Roccia MG. Stress and inflammation in coronary artery disease: a review psychoneuroendocrineimmunology-based. Front Immunol. (2018) 9:2031. doi: 10.3389/fimmu.2018.02031
5. Abbas A, Gregersen I, Holm S, Daissormont I, Bjerkeli V, Krohg-Sørensen K, et al. Interleukin 23 levels are increased in carotid atherosclerosis: possible role for the interleukin 23/interleukin 17 axis. Stroke. (2015) 46:793–9. doi: 10.1161/STROKEAHA.114.006516
6. Goel PK, Ashfaq F, Khanna R, Ramesh V, Pandey CM. The association between small dense low density lipoprotein and coronary artery disease in north indian patients. IJCB. (2017) 32:186–92. doi: 10.1007/s12291-016-0592-7
7. van Raalte DH, Diamant M, Ouwens DM, Ijzerman RG, Linssen MML, Guigas B, et al. Glucocorticoid treatment impairs microvascular function in healthy men in association with its adverse effects on glucose metabolism and blood pressure: a randomised controlled trial. Diabetologia. (2013) 56:2383–91. doi: 10.1007/s00125-013-3016-8
8. Wu G-C, Liu H-R, Leng R-X, Li X-P, Li X-M, Pan H-F, et al. Subclinical atherosclerosis in patients with systemic lupus erythematosus: a systemic review and meta-analysis. Autoimmun Rev. (2016) 15:22–37. doi: 10.1016/j.autrev.2015.10.002
9. Mirzaei H, Ferns GA, Avan A, Mobarhan MG. Cytokines and MicroRNA in coronary artery disease. Adv Clin Chem. (2017) 82:47–70. doi: 10.1016/bs.acc.2017.06.004
10. Yuan S, Lin A, He Q-Q, Burgess S, Larsson SC. Circulating interleukins in relation to coronary artery disease, atrial fibrillation and ischemic stroke and its subtypes: a two-sample Mendelian randomization study. Int J Cardiol. (2020) 313:99–104. doi: 10.1016/j.ijcard.2020.03.053
11. Gisterå A, Hansson GK. The immunology of atherosclerosis. Nat Rev Nephrol. (2017) 13:368–80. doi: 10.1038/nrneph.2017.51
12. Taleb S. Inflammation in atherosclerosis. Arch Cardiovasc Dis. (2016) 109:708–15. doi: 10.1016/j.acvd.2016.04.002
13. Montarello NJ, Nguyen MT, Wong DTL, Nicholls SJ, Psaltis PJ. Inflammation in coronary atherosclerosis and its therapeutic implications. Cardiovasc Drugs Therapy. (2020). doi: 10.1007/s10557-020-07106-6. [Epub ahead of print].
14. Nguyen MT, Fernando S, Schwarz N, Tan JT, Bursill CA, Psaltis PJ. Inflammation as a therapeutic target in atherosclerosis. J Clin Med. (2019) 8:1109. doi: 10.3390/jcm8081109
15. Ridker PM, Libby P, MacFadyen JG, Thuren T, Ballantyne C, Fonseca F, et al. Modulation of the interleukin-6 signalling pathway and incidence rates of atherosclerotic events and all-cause mortality: analyses from the Canakinumab Anti-Inflammatory Thrombosis Outcomes Study (CANTOS). Europ Heart J. (2018) 39:3499–507. doi: 10.1093/eurheartj/ehy310
16. Libby P. Interleukin-1 beta as a target for atherosclerosis therapy: biological basis of CANTOS and beyond. J Am College Cardiol. (2017) 70:2278–89. doi: 10.1016/j.jacc.2017.09.028
17. Akita K, Isoda K, Sato-Okabayashi Y, Kadoguchi T, Kitamura K, Ohtomo F, et al. An interleukin-6 receptor antibody suppresses atherosclerosis in atherogenic mice. Front Cardiovasc Med. (2017) 4:84. doi: 10.3389/fcvm.2017.00084
18. Kleveland O, Kunszt G, Bratlie M, Ueland T, Broch K, Holte E, et al. Effect of a single dose of the interleukin-6 receptor antagonist tocilizumab on inflammation and troponin T release in patients with non-ST-elevation myocardial infarction: a double-blind, randomized, placebo-controlled phase 2 trial. Europ Heart J. (2016) 37:2406–13. doi: 10.1093/eurheartj/ehw171
19. Barrett T, Wilhite SE, Ledoux P, Evangelista C, Kim IF, Tomashevsky M, et al. NCBI GEO: archive for functional genomics data sets–update. Nucleic Acids Res. (2013) 41:D991–5. doi: 10.1093/nar/gks1193
20. Davis S, Meltzer PS. GEOquery: a bridge between the gene expression omnibus (GEO) and bioconductor. Bioinformatics (Oxford, England). (2007) 23:1846–847. doi: 10.1093/bioinformatics/btm254
21. Ritchie ME, Phipson B, Wu D, Hu Y, Law CW, Shi W, et al. limma powers differential expression analyses for RNA-sequencing and microarray studies. Nucleic Acids Res. (2015) 43:e47–e. doi: 10.1093/nar/gkv007
22. Newman AM, Liu CL, Green MR, Gentles AJ, Feng W, Xu Y, et al. Robust enumeration of cell subsets from tissue expression profiles. Nat Methods. (2015) 12:453–7. doi: 10.1038/nmeth.3337
23. Rooney MS, Shukla SA, Wu CJ, Getz G, Hacohen N. Molecular and genetic properties of tumors associated with local immune cytolytic activity. Cell. (2015) 160:48–61. doi: 10.1016/j.cell.2014.12.033
24. Thorsson V, Gibbs DL, Brown SD, Wolf D, Bortone DS, Ou Yang T-H, et al. The Immune Landscape of Cancer. Immunity. (2018) 48:812–30.e14. doi: 10.1016/j.immuni.2018.03.023
25. Senbabaoglu Y, Gejman RS, Winer AG, Liu M, Van Allen EM, de Velasco G, et al. Tumor immune microenvironment characterization in clear cell renal cell carcinoma identifies prognostic and immunotherapeutically relevant messenger RNA signatures. Genome Biol. (2016) 17:231. doi: 10.1186/s13059-016-1092-z
26. Subramanian A, Tamayo P, Mootha VK, Mukherjee S, Ebert BL, Gillette MA, et al. Gene set enrichment analysis: A knowledge-based approach for interpreting genome-wide expression profiles. Proc Natl Acad Sci USA. (2005) 102:15545–15550. doi: 10.1073/pnas.0506580102
27. Hänzelmann S, Castelo R, Guinney J. GSVA: gene set variation analysis for microarray and RNA-Seq data. BMC Bioinform. (2013) 14:7. doi: 10.1186/1471-2105-14-7
28. Liberzon A, Subramanian A, Pinchback R, Thorvaldsdottir H, Tamayo P, Mesirov JP. Molecular signatures database (MSigDB) 3.0. Bioinformatics. (2011) 27:1739–40. doi: 10.1093/bioinformatics/btr260
29. Yu G, Wang L-G, Han Y, He Q-Y. clusterProfiler: an r package for comparing biological themes among gene clusters. OMICS. (2012) 16:284–7. doi: 10.1089/omi.2011.0118
30. Gu Z, Eils R, Schlesner M. Complex heatmaps reveal patterns and correlations in multidimensional genomic data. Bioinformatics. (2016) 32:2847–9. doi: 10.1093/bioinformatics/btw313
31. Wickham H. ggplot2. Cham: Springer International Publishing. (2016) doi: 10.1007/978-3-319-24277-4
33. Hansson GK, Hermansson A. The immune system in atherosclerosis. Nat Immunol. (2011) 12:204–12. doi: 10.1038/ni.2001
34. Johnson LA, Jackson DG. The chemokine CX3CL1 promotes trafficking of dendritic cells through inflamed lymphatics. J Cell Sci. (2013) 126:5259–70. doi: 10.1242/jcs.135343
35. Bergamaschi C, Pandit H, Nagy BA, Stellas D, Jensen SM, Bear J, et al. Heterodimeric IL-15 delays tumor growth and promotes intratumoral CTL and dendritic cell accumulation by a cytokine network involving XCL1, IFN-γ, CXCL9 and CXCL10. J Immunother Cancer. (2020) 8. doi: 10.1136/jitc-2020-000599
36. Chabot V, Reverdiau P, Iochmann S, Rico A, Sénécal D, Goupille C, et al. CCL5-enhanced human immature dendritic cell migration through the basement membrane in vitro depends on matrix metalloproteinase-9. J Leukocyte Biol. (2006) 79:767–78. doi: 10.1189/jlb.0804464
37. Yilmaz A, Lochno M, Traeg F, Cicha I, Reiss C, Stumpf C, et al. Emergence of dendritic cells in rupture-prone regions of vulnerable carotid plaques. Atherosclerosis. (2004) 176:101–10. doi: 10.1016/j.atherosclerosis.2004.04.027
38. Liu P, Yu Y-RA, Spencer JA, Johnson AE, Vallanat CT, Fong AM, et al. CX3CR1 deficiency impairs dendritic cell accumulation in arterial intima and reduces atherosclerotic burden. Arterioscler Thromb Vasc Biol. (2008) 28:243–50. doi: 10.1161/ATVBAHA.107.158675
39. Angeli V, Llodrá J, Rong JX, Satoh K, Ishii S, Shimizu T, et al. Dyslipidemia associated with atherosclerotic disease systemically alters dendritic cell mobilization. Immunity. (2004) 21:561–74. doi: 10.1016/j.immuni.2004.09.003
40. Packard RRS, Maganto-García E, Gotsman I, Tabas I, Libby P, Lichtman AH. CD11c(+) dendritic cells maintain antigen processing, presentation capabilities, and CD4(+) T-cell priming efficacy under hypercholesterolemic conditions associated with atherosclerosis. Circul Res. (2008) 103:965–73. doi: 10.1161/CIRCRESAHA.108.185793
41. McKellar GE, McCarey DW, Sattar N, McInnes IB. Role for TNF in atherosclerosis? Lessons from autoimmune disease. Nat Rev Cardiol. (2009) 6:410–7. doi: 10.1038/nrcardio.2009.57
42. Ridker PM, Everett BM, Thuren T, MacFadyen JG, Chang WH, Ballantyne C, et al. Antiinflammatory therapy with canakinumab for atherosclerotic disease. N Engl J Med. (2017) 377:1119–31. doi: 10.1056/NEJMoa1707914
43. Paulson KE, Zhu S-N, Chen M, Nurmohamed S, Jongstra-Bilen J, Cybulsky MI. Resident intimal dendritic cells accumulate lipid and contribute to the initiation of atherosclerosis. Circul Res. (2010) 106:383–90. doi: 10.1161/CIRCRESAHA.109.210781
44. Koltsova EK, Ley K. How dendritic cells shape atherosclerosis. Trends Immunol. (2011) 32:540–7. doi: 10.1016/j.it.2011.07.001
45. Hermansson A, Ketelhuth DFJ, Strodthoff D, Wurm M, Hansson EM, Nicoletti A, et al. Inhibition of T cell response to native low-density lipoprotein reduces atherosclerosis. J Exp Med. (2010) 207:1081–93. doi: 10.1084/jem.20092243
46. Sun J, Hartvigsen K, Chou M-Y, Zhang Y, Sukhova GK, Zhang J, et al. Deficiency of antigen-presenting cell invariant chain reduces atherosclerosis in mice. Circulation. (2010) 122:808–20. doi: 10.1161/CIRCULATIONAHA.109.891887
47. Koltsova EK, Garcia Z, Chodaczek G, Landau M, McArdle S, Scott SR, et al. Dynamic T cell-APC interactions sustain chronic inflammation in atherosclerosis. J Clin Invest. (2012) 122:3114–26. doi: 10.1172/JCI61758
48. Yan P, Xia C, Duan C, Li S, Mei Z. Biological characteristics of foam cell formation in smooth muscle cells derived from bone marrow stem cells. Int J Biol Sci. (2011) 7:937–46. doi: 10.7150/ijbs.7.937
49. Weyand CM, Berry GJ, Goronzy JJ. The immunoinhibitory PD-1/PD-L1 pathway in inflammatory blood vessel disease. J Leuk Biol. (2018) 103:565–75. doi: 10.1189/jlb.3MA0717-283
50. Lin A, Wei T, Meng H, Luo P, Zhang J. Role of the dynamic tumor microenvironment in controversies regarding immune checkpoint inhibitors for the treatment of non-small cell lung cancer (NSCLC) with EGFR mutations. Mol Cancer. (2019) 18:139. doi: 10.1186/s12943-019-1062-7
51. Dinh TN, Kyaw TS, Kanellakis P, To K, Tipping P, Toh B-H, et al. Cytokine therapy with interleukin-2/anti-interleukin-2 monoclonal antibody complexes expands CD4+CD25+Foxp3+ regulatory T cells and attenuates development and progression of atherosclerosis. Circulation. (2012) 126:1256–266. doi: 10.1161/CIRCULATIONAHA.112.099044
52. Kita T, Yamashita T, Sasaki N, Kasahara K, Sasaki Y, Yodoi K, et al. Regression of atherosclerosis with anti-CD3 antibody via augmenting a regulatory T-cell response in mice. Cardiovasc Res. (2014) 102:107–17. doi: 10.1093/cvr/cvu002
53. Wigren M, Björkbacka H, Andersson L, Ljungcrantz I, Fredrikson GN, Persson M, et al. Low levels of circulating CD4+FoxP3+ T cells are associated with an increased risk for development of myocardial infarction but not for stroke. Arterioscl Thromb Vasc Biol. (2012) 32:2000–4. doi: 10.1161/ATVBAHA.112.251579
54. Hermansson A, Johansson DK, Ketelhuth DFJ, Andersson J, Zhou X, Hansson GK. Immunotherapy with tolerogenic apolipoprotein B-100-loaded dendritic cells attenuates atherosclerosis in hypercholesterolemic mice. Circulation. (2011) 123:1083–91. doi: 10.1161/CIRCULATIONAHA.110.973222
55. de Boer OJ, van der Meer JJ, Teeling P, van der Loos CM, van der Wal AC. Low numbers of FOXP3 positive regulatory T cells are present in all developmental stages of human atherosclerotic lesions. PLoS ONE. (2007) 2:e779. doi: 10.1371/journal.pone.0000779
56. Ait-Oufella H, Salomon BL, Potteaux S, Robertson A-KL, Gourdy P, Zoll J, et al. Natural regulatory T cells control the development of atherosclerosis in mice. Nat Med. (2006) 12:178–180. doi: 10.1038/nm1343
57. Elhage R, Gourdy P, Brouchet L, Jawien J, Fouque M-J, Fiévet C, et al. Deleting TCR alpha beta+ or CD4+ T lymphocytes leads to opposite effects on site-specific atherosclerosis in female apolipoprotein E-deficient mice. Am J Pathol. (2004) 165:2013–8. doi: 10.1016/s0002-9440(10)63252-x
58. Han S, Liu P, Zhang W, Bu L, Shen M, Li H, et al. The opposite-direction modulation of CD4+CD25+ Tregs and T helper 1 cells in acute coronary syndromes. Clin Immunol. (2007) 124:90–7. doi: 10.1016/j.clim.2007.03.546
59. Taghavie-Moghadam PL, Waseem TC, Hattler J, Glenn LM, Dobrian AD, Kaplan MH, et al. STAT4 regulates the CD8(+) regulatory T Cell/T follicular helper cell axis and promotes atherogenesis in insulin-resistant Ldlr(-/-) Mice. J Immunol. (2017) 199:3453–65. doi: 10.4049/jimmunol.1601429
60. Gaddis DE, Padgett LE, Wu R, McSkimming C, Romines V, Taylor AM, et al. Apolipoprotein AI prevents regulatory to follicular helper T cell switching during atherosclerosis. Nat Commun. (2018) 9:1095. doi: 10.1038/s41467-018-03493-5
Keywords: immune infiltrates, atherosclerotic plaque, coronary artery disease, pathways, immune microenvironment
Citation: Han H, Du R, Cheng P, Zhang J, Chen Y and Li G (2021) Comprehensive Analysis of the Immune Infiltrates and Aberrant Pathways Activation in Atherosclerotic Plaque. Front. Cardiovasc. Med. 7:602345. doi: 10.3389/fcvm.2020.602345
Received: 03 September 2020; Accepted: 31 December 2020;
Published: 05 February 2021.
Edited by:
George W. Booz, University of Mississippi Medical Center School of Dentistry, United StatesReviewed by:
Suowen Xu, University of Science and Technology of China, ChinaAndriy Yabluchanskiy, University of Oklahoma Health Sciences Center, United States
Copyright © 2021 Han, Du, Cheng, Zhang, Chen and Li. This is an open-access article distributed under the terms of the Creative Commons Attribution License (CC BY). The use, distribution or reproduction in other forums is permitted, provided the original author(s) and the copyright owner(s) are credited and that the original publication in this journal is cited, in accordance with accepted academic practice. No use, distribution or reproduction is permitted which does not comply with these terms.
*Correspondence: Yang Chen, smartchengdu@136.com; Gang Li, lgmed2020@163.com
†These authors have contributed equally to this work