Information guided adaptation of complex biological systems
- Hillman Cancer Center, Radiation Oncology, University of Pittsburgh Medical Center, Williamsport, PA, United States
A Corrigendum on
Information guided adaptation of complex biological systems
by Herndon RC (2023). Front. Complex Syst. 1:1208066. doi: 10.3389/fcpxs.2023.1208066
In the published article, the Supplementary Appendix was mistakenly not included in the publication. The missing material appears below:
Information guided adaptation of complex systems is extensible to quantum gravity. The analytic framework provided by the application of functional information to complex biological systems has direct application to the problem of uniting general relativity (GR) and quantum theory (QT). Functional information, If, provides an exchange mechanism between these two highly complex, successful, and currently incompatible models by creating a GR-QT information loop based on the assumption that there exists a mathematical connection between these two complex models that will serve as an information conduit, i.e., the models are describing the same property or thing (Supplementary Appendix Figure A1). This means the functional information change in a QT scenario can be used to quantify the corresponding change in GR information, which in turn can be used to quantify changes in a specific parameter(s)-of-interest.
Example Scenario: An investigator wants to know how a change in their QT model affects a parameter-of-interest in their corresponding GR model. Functional information is calculated from baseline GR (step I) and QT (step II) model data, IGR and IQT respectively. The blue arrows of Figure A1 convey the assumption that these two information data sets correspond to the same property or thing, thus permitting inter-model comparison. The investigator implements the QT model change-of-interest and determines the updated functional information, IQT′ (step III). To quantify a parameter-of-interest in the GR model that corresponds to a specific QT model change, the investigator defines the change in GR information as equivalent to the change in QT information, i.e., intra-model information change is IGR′/IGR = IQT′/IQT (Figure A1 yellow arrows). The updated GR functional information, IGR′, is calculated (step IV) using the baseline information, IGR and IQT, and the updated QT model information, IQT′. The updated GR functional information, IGR′, is then used to determine the GR parameter-of-interest. The cycle is repeated if additional dynamic data is needed.
This example illustrates how functional information may offer an analytic tool applicable to scenarios where GR and QT theories overlap and contend.
The author apologizes for this error and states that this does not change the scientific conclusions of the article in any way. The original article has been updated.
Publisher’s note
All claims expressed in this article are solely those of the authors and do not necessarily represent those of their affiliated organizations, or those of the publisher, the editors and the reviewers. Any product that may be evaluated in this article, or claim that may be made by its manufacturer, is not guaranteed or endorsed by the publisher.
Supplementary Appendix
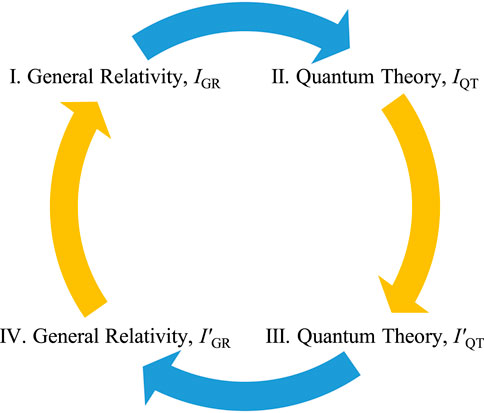
FIGURE A1. The functional information cycle, steps I–IV, provides a mechanism to analyze changes in a general relativity (GR) model data set that correspond to changes in a quantum theory (QT) model data set. Functional information can cross the knowledge chasm between compatible GR and QT models (blue arrows). Comparable GR and QT intra-model information change (yellow arrows) completes the cycle loop and permits analysis of the general relativity model, GR, using quantum theory, QT, model data or vice versa.
Keywords: information theory, complexity, bioinformatics, functional information, complex adaptive systems, computational biology
Citation: Herndon RC (2023) Corrigendum: Information guided adaptation of complex biological systems. Front. Complex Syst. 1:1254070. doi: 10.3389/fcpxs.2023.1254070
Received: 06 July 2023; Accepted: 25 July 2023;
Published: 04 August 2023.
Edited and reviewed by:
Marta C. Gonzalez, University of California, Berkeley, United StatesCopyright © 2023 Herndon. This is an open-access article distributed under the terms of the Creative Commons Attribution License (CC BY). The use, distribution or reproduction in other forums is permitted, provided the original author(s) and the copyright owner(s) are credited and that the original publication in this journal is cited, in accordance with accepted academic practice. No use, distribution or reproduction is permitted which does not comply with these terms.
*Correspondence: R. Craig Herndon, herndonrc@upmc.edu