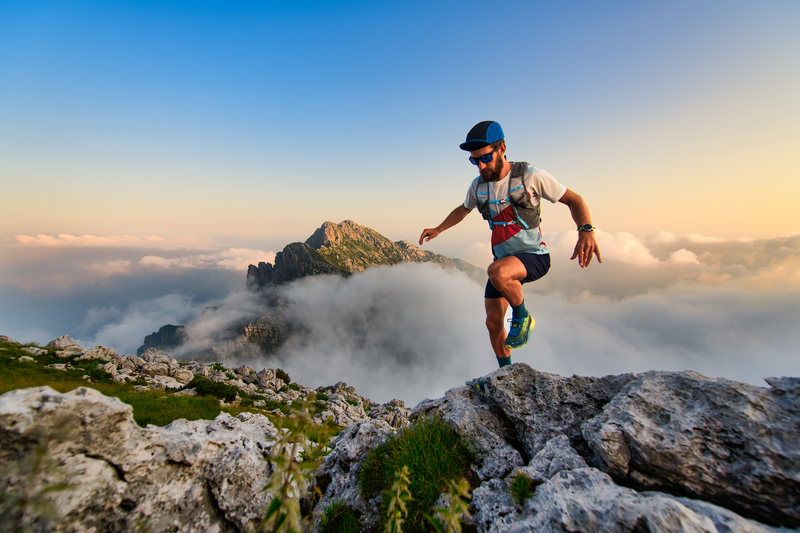
95% of researchers rate our articles as excellent or good
Learn more about the work of our research integrity team to safeguard the quality of each article we publish.
Find out more
EDITORIAL article
Front. Conserv. Sci. , 18 February 2025
Sec. Animal Conservation
Volume 6 - 2025 | https://doi.org/10.3389/fcosc.2025.1566673
This article is part of the Research Topic Linking Habitat Quality to Population Dynamics for Conservation Decision Making View all 10 articles
Editorial on the Research Topic
Linking habitat quality to population dynamics for conservation decision making
Natural and anthropogenic factors alter habitats so that trends, random sampling, or single snapshots of habitat conditions often do not predict future species abundance (Kunegel-Lion et al., 2022; Conquet et al., 2023). Habitat dynamics are measured at different spatial scales (e.g., landscape, management units, patch, territory) and are asynchronous and driven by climate change, disturbances, invasive species, and habitat management.
Endangered species recovery plans and species status assessments have requirements to address time to population recovery, but they often do not adequately address habitat dynamics and factors that led to endangerment (Auld and Keith, 2009; Shirey et al., 2022). Understanding how habitat dynamics influence population dynamics is necessary for making sound conservation decisions.
Examples across a range of species, habitat and actions are important to facilitate decision making (Runge, 2011). From literature reviews, we found 160 individuals as potential authors and invited them to contribute, leading to 9 manuscripts. Below we summarize these studies and related literature to describe improvements to support conservation decision making.
Nichols et al. introduced Structured Decision Making (SDM) and Adaptive Resource Management (ARM) topics used in natural resource management and a framework to combine population and habitat variables in a statistical likelihood approach. Our view of habitat conditions was broad, for example including human disturbance as a factor that altered habitat suitability (e.g., Martin et al., 2011). Peterson and Duarte used integrated models to prioritize salmon habitat restoration in perennial versus ephemeral habitats depending on whether essential suitable perennial habitat was already present, but ephemeral habitats could produce greater salmon growth and survival during high-water years.
Eaton et al. use a portfolio of strategies for an endangered Puerto Rican frog where uncertainties could result in conservation failure due to climate changes, so that several strategies might be best. Stantial et al. describe the initial stage of the experimentalist school of adaptive management, emphasizing stakeholder involvement, shared understanding, and plans for experimentation that eventually could reduce the uncertainty around the use of prescribed fire for salt marsh bird species.
Early work on linking habitat and population dynamics used the Landis Forest succession models (e.g., Akçakaya, 2001; He, 2009), which led to other approaches such as state transition models (e.g., Raphael et al., 2013). Later metamodeling approaches used separate disease, predator-prey dynamics, and habitat dynamics models to pass information to population viability analysis (PVA) models (Lacy et al., 2013). In this Research Topic, Lacy et al. provide an example linking a predator (polar bears), prey (pinnipeds), and habitat change (declining Arctic sea ice).
Lacy et al. provide a PVA that includes habitat dynamics at the territory scale exploring both habitat and population management options to provide for sustainable Florida scrub-jay populations in a fragmented landscape. This modeling relied on long-term research of populations and habitat dynamics and learning from ARM that linked habitat, population data, and decision making, and brought stakeholders, biologists, and managers together. Forero-Sanchez et al. use a PVA of an endangered tamarin with a plant-based energetic model to estimate carrying capacity for subpopulations, incorporating climate change, fire risk, and habitat connectivity through forest corridors to estimate inter-population dispersal and metapopulation persistence.
Bohnett et al. show how a combination of connectivity models for focal species with complementary and opposing habitat requirements can better inform landscape design to prioritize conservation areas in landscapes with rapid human development. Schumaker shows a landcover map and movement simulator for an endangered butterfly to explore the concepts and mechanics behind a novel connectivity assessment methodology.
Integrated population models (IPM) can combine population data and habitat to incorporate habitat and population dynamics in both breeding and wintering areas (Osnas et al., 2021). Information about many species of conservation concern with different requirements has been integrated into IPMs to make decisions about fire management (Conlisk et al., 2015).
The examples above demonstrate that a broad variety of approaches are available for modeling ecosystems and species to serve conservation decision-making, providing a large toolbox that includes both canned and investigator-adapted coding. Both modeling and monitoring are generally needed to resolve uncertainties (Armstrong and Reynolds 2012, Converse and Armstrong 2016). Monitoring provides fantastic opportunities for learning and is often a regulatory requirement used in negotiation, but its implementation to make better decisions is often not well developed (Yoccoz et al., 2001, Nichols and Williams 2006, Nichols and Armstrong 2012). We suggest monitoring should address the 4 major reasons for monitoring to support decision-making described in this volume Nichols et al. We suggest applications increase collaboration among population biologists, geneticists, field biologist, managers, stakeholders, and habitat modeling experts.
DB: Writing – original draft, Writing – review & editing. DA: Writing – original draft, Writing – review & editing. JN: Writing – original draft, Writing – review & editing. RL: Writing – original draft, Writing – review & editing.
We thank the authors who submitted manuscripts in this Research Topic, guest reviewers and editors, and the Frontiers team.
The authors declare that the research was conducted in the absence of any commercial or financial relationships that could be construed as a potential conflict of interest.
The author(s) declared that they were an editorial board member of Frontiers, at the time of submission. This had no impact on the peer review process and the final decision.
All claims expressed in this article are solely those of the authors and do not necessarily represent those of their affiliated organizations, or those of the publisher, the editors and the reviewers. Any product that may be evaluated in this article, or claim that may be made by its manufacturer, is not guaranteed or endorsed by the publisher.
Akçakaya H. (2001). Linking population-level risk assessment with landscape and habitat models. Sci. Total Environ. 274, 283–291. doi: 10.1016/S0048-9697(01)00750-1
Armstrong D. P., Reynolds. M. H. (2012). Modelling reintroduced populations: the state of the art and future directions. Reintroduction biology: integrating Sci. Manage., Oxford, UK: Wiley-Blackwell. 165–222.
Auld T. D., Keith D. A. (2009). Dealing with threats: integrating science and management. Ecol. Manage. Restor. 10, S79–S87. doi: 10.1111/j.1442-8903.2009.00447.x
Conlisk E., Syphard A. D., Franklin J., Regan. H. M. (2015). Predicting the impact of fire on a vulnerable multi-species community using a dynamic vegetation model. Ecol. Model. 301, 27–39. doi: 10.1016/j.ecolmodel.2015.02.004
Conquet E., Ozgul A., Blumstein D. T., Armitage K. B., Oli M. K., Martin J. G., et al. (2023). Demographic consequences of changes in environmental periodicity. Ecology 104, e3894. doi: 10.1002/ecy.v104.3
Converse S. J., Armstrong. D. P. (2016). Demographic modeling for reintroduction decision-making. Reintroduction fish wildlife populations, University of California Press. 123–146.
He H. S. (2009). “A review of LANDIS and other forest landscape models for integration with wildlife models,” in Models for planning wildlife conservation in large landscapes (Elsevier Science, San Diego, California, USA), 321–338.
Kunegel-Lion M., Neilson E. W., Mansuy N., Goodsman. D. W. (2022). Habitat quality does not predict animal population abundance on frequently disturbed landscapes. Ecol. Model. 469, 109943. doi: 10.1016/j.ecolmodel.2022.109943
Lacy R. C., Miller P. S., Nyhus P. J., Pollak J., Raboy B. E., Zeigler. S. L. (2013). Metamodels for transdisciplinary analysis of wildlife population dynamics. PloS One 8, e84211. doi: 10.1371/journal.pone.0084211
Martin J., Fackler P. L., Nichols J. D., Runge M. C., McIntyre C. L., Lubow B. L., et al. (2011). An adaptive-management framework for optimal control of hiking near golden eagle nests in Denali national park. Conserv. Biol. 25, 316–323. doi: 10.1111/j.1523-1739.2010.01644.x
Nichols J. D., Armstrong. D. P. (2012). Monitoring for reintroductions. Reintroduction biology: integrating Sci. Manage., Oxford, UK: Wiley-Blackwell. 223–255.
Nichols J. D., Williams. B. K. (2006). Monitoring for conservation. Trends Ecol. Evol. 21, 668–673. doi: 10.1016/j.tree.2006.08.007
Osnas E. E., Boomer G. S., DeVries J. H., Runge M. C. (2021). Decision-support framework for linking regional-scale management actions to continental-scale conservation of wide-ranging species. US Geological Survey. Reston, Virginia, USA. doi: 10.3133/ofr20201084
Raphael M. G., Hessburg P., Kennedy R., Lehmkuhl J., Marcot B. G., Scheller R., et al. (2013). Assessing the compatibility of fuel treatments, wildfire risk, and conservation of northern spotted owl habitats and populations in the eastern cascades: A multi-scale analysis Vol. 31 (JFSP Research Project Reports). Available at: http://digitalcommons.unl.edu/jfspresearch/31 (Accessed Feb 12 2025).
Runge M. C. (2011). An introduction to adaptive management for threatened and endangered species. J. Fish Wildlife Manage. 2, 220–233. doi: 10.3996/082011-JFWM-045
Shirey P. D., Colvin S. A., Roulson L. H., Bigford. T. E. (2022). A review of recovery plan criteria for threatened and endangered fish taxa managed by the US fish and wildlife service. Fisheries 47, 256–261. doi: 10.1002/fsh.10743
Keywords: adaptive management, endangered species recovery, restoration, population modelling, habitat modelling
Citation: Breininger DR, Armstrong DP, Nichols JD and Lacy RC (2025) Editorial: Linking habitat quality to population dynamics for conservation decision making. Front. Conserv. Sci. 6:1566673. doi: 10.3389/fcosc.2025.1566673
Received: 25 January 2025; Accepted: 04 February 2025;
Published: 18 February 2025.
Edited and Reviewed by:
Ronald R. Swaisgood, San Diego Zoo Wildlife Alliance, United StatesCopyright © 2025 Breininger, Armstrong, Nichols and Lacy. This is an open-access article distributed under the terms of the Creative Commons Attribution License (CC BY). The use, distribution or reproduction in other forums is permitted, provided the original author(s) and the copyright owner(s) are credited and that the original publication in this journal is cited, in accordance with accepted academic practice. No use, distribution or reproduction is permitted which does not comply with these terms.
*Correspondence: David R. Breininger, ZGF2aWQuYnJlaW5pbmdlckB1Y2YuZWR1
Disclaimer: All claims expressed in this article are solely those of the authors and do not necessarily represent those of their affiliated organizations, or those of the publisher, the editors and the reviewers. Any product that may be evaluated in this article or claim that may be made by its manufacturer is not guaranteed or endorsed by the publisher.
Research integrity at Frontiers
Learn more about the work of our research integrity team to safeguard the quality of each article we publish.