- 1Independent Researcher, Jonesboro, ME, United States
- 2Department of Biology, University of Central Florida, Orlando, FL, United States
- 3Department of Mathematics and Systems Engineering, Florida Institute of Technology, Melbourne, FL, United States
- 4Department of Biology, Colby College, Waterville, ME, United States
- 5Brevard County Environmentally Endangered Lands Program, Melbourne, FL, United States
- 6Ecological Services, United States Fish & Wildlife Service, St. Petersburg, FL, United States
Population dynamics and viability are driven by interactions among habitat and species biology. The Florida scrub-jay (Aphelocoma coerulescens) is a declining and Federally Threatened bird species that requires mid-succession habitat of partly open soil surface with mid-height vegetation. This habitat is created and sustained in a dynamic state of vegetation growth and periodic natural (e.g., fire) or managed (e.g., mechanical clearing) disturbances. Florida scrub-jays once occupied open oak scrub habitats across much of peninsular Florida but have been reduced to a few regional metapopulations and scattered isolated remnant populations. Many of these populations are undergoing continuing decline as open scrub is either converted to residential development or transitions into closed pine and oak forests due to fire suppression. Long-term field studies have shown that breeding and survival rates are determined by the quality of the scrub habitat, with the demography influenced by and in turn mediating the social structure. Prior Population Viability Analyses (PVA) that included dependencies of demographic rates on habitat and social structure indicated that the east coast Florida metapopulations were fragmented into remnant protected patches that were too small and isolated to support long-term persistence, even if the remaining habitat area and quality was sustained. Moreover, recent modeling of habitat transitions under various proposed management schemes, in conjunction with implementation of Adaptive Resource Management (ARM), projected that the proportion of optimal habitat will continue to decline. In this study, we integrated these habitat projections within the PVA and found that the Brevard County mainland metapopulation is projected to decline toward extinction unless habitat quality, extent, and connectivity can be improved. Land managers have recently implemented new innovative methods for restoring optimal scrub habitat, identifying potential improvements in habitat connectivity of nearby populations, and translocation methods to increase and reinforce the demographic and genetic integrity of local populations. Our linked habitat-population models project that the combination of such habitat and population management actions can stabilize the metapopulation and achieve long-term viability.
1 Introduction
The transitional habitat dynamics of species of conservation concern often need planning and active management. Active management is especially important for species in fragmented landscapes that once depended on lightning fires that can no longer spread naturally across landscapes. However, these managed replacements often differ greatly from natural fires in seasonality, intensity, and frequency and often need supplemental mechanical treatments to achieve the same outcome as natural disturbances (Duncan et al., 2009, Duncan et al., 2015).
There is uncertainty in adaptive management of such systems where actions and models of predicted effects need to be repeatedly revisited and adjusted (Nichols et al., 2024). Adaptive Resource Management (ARM) models support management by estimating the most appropriate management actions based on habitat (e.g., successional states) and occupancy (e.g., territory occupancy) states (Eaton et al., 2021), abundance, or vital rates (Johnson et al., 2011). In addition, endangered species recovery planning entails population analyses to estimate time for species recovery and potential population strategies required for long-term sustainability (Population Viability Analyses, PVA).
However, both habitat and population dynamics are often characterized too simply for guiding effective management. Habitat is sometimes described only as types of landcover where a species is found, but habitat is not likely to depend on a single property of the environment, nor will it often be spatially uniform. Habitat should refer to conditions relevant to both occupancy of species and the demographics (recruitment, survival) that support sustainable populations (Van Horne, 1983; Hall et al., 1997). Most PVA make projections based on demographic rates recorded over a series of years with implicit or explicit assumptions that the birth rates, death rates, and habitat quality will remain the same as in years when data were collected. To account for ongoing or projected changes to the environment, a PVA should include both the projected changes to the habitat and links of habitat to demography (fecundity, survival, dispersal, and carrying capacity). The extent to which the PVA considers the key features and dynamics of habitat will determine the usefulness of the PVA for projecting trends, assessing threats, and evaluating management options.
The Florida scrub-jay (Aphelocoma coerulescens, FSJ) is a declining and Federally Threatened bird species endemic to Florida, USA. Much about FSJ sociobiology, population parameters, and habitat requirements has been the result of long-term studies of two distinct metapopulations, one study now exceeding 45 years (Woolfenden and Fitzpatrick, 1984; Fitzpatrick and Bowman, 2016) and the other over 35 years (Breininger et al., 2009, Breininger et al., 2023). These studies show that FSJs generally mate for life and stay within the same territory once they become breeders (Woolfenden and Fitzpatrick, 1984; Fitzpatrick and Bowman, 2016). Florida scrub-jays are cooperative breeders where young usually remain with their parents for several years helping feed new offspring, defend territories, and spot and mob predators. Nonbreeders quickly fill nearby breeder vacancies and rarely disperse more than a few kilometers from their natal territory (Breininger et al., 2006, Breininger et al., 2023). Florida scrub-jays require oak scrub (Quercus spp.) that occurs on dry sandy ridges often within a landscape of mesic flatwoods (saw palmetto) and ephemerally flooded marshes within areas subject to fires caused by lightning. Transitions between habitat states vary depending on vegetation assemblages, climatic conditions, habitat edge effects, fire history, and mechanical cutting in manners that influence territory occupancy, abundance, reproductive success, and survival (Breininger et al., 2010, Breininger et al., 2018; Johnson et al., 2011; Eaton et al., 2021). The 45-year study (at Archbold Biological Station) focused on relatively stable, optimal habitat, whereas the 35-year study (on Kennedy Space Center, Merritt Island National Wildlife Refuge, and nearby mainland Brevard County) occurred in conservation areas degraded by reductions in the fire regime and where restoration has been a difficult process. Degradation resulted in spatially and temporally dynamic patterns in habitat quality where territory quality varied greatly within landscapes.
A revised U.S. Fish and Wildlife Service species recovery plan was approved in 2019 (U.S. Fish and Wildlife Service, 2019a) based on a triage strategy identifying areas known as “focal landscapes” that have the potential for long-term population viability. Three criteria for delisting the species are: (1) stable or increasing populations in each of 7 focal landscapes (one of which, the East Coastal Genetic Unit, coincides with the South & Central Mainland Brevard County metapopulation that is the focus of this study); (2) subpopulations “connected to the extent that natural genetic diversity can be naturally maintained without translocations”; and (3) threats “addressed such that sufficient habitat remains for the species to remain viable for the foreseeable future.” The first criterion requires that the decline over the last few decades be halted or even reversed. The second criterion implies minimal loss of genetic diversity, but it does not define what low level of loss might be accepted. Retaining at least 95% of genetic diversity would be approximately equivalent to avoiding inbreeding at the level of first-cousin matings (F = 0.0625) or closer. The third criterion directly addresses the need for habitat protection and management, but it does not define what is considered to be “viable” (other than the first two criteria), nor does it define the duration of the “foreseeable future”. A large majority of the U.S. Fish & Wildlife Service recovery plans that provide explicit definitions of viability have used a criterion of keeping the probability of extinction below 5% for 100 years (Carroll et al., 2019).
This paper focuses on the South and Central Mainland FSJ metapopulation (as denoted by Stith, 1999; and shown in Figure 1) in Brevard County, Florida, USA. This metapopulation occupies habitats that are mostly protected and managed by the county Environmentally Endangered Lands program. Prior Population Viability Analyses (Lacy and Breininger, 2021) assessed the viability of this and also the North Mainland and Canaveral metapopulations in Brevard County, but without consideration of the changing habitat conditions caused by vegetation succession, natural disturbances, and management actions. Several earlier PVA models assumed static habitat that was identical across populations, excluded genetics, and were not individual-based (e.g., Woolfenden and Fitzpatrick, 1991; Root, 1998; Breininger et al., 1999). Stith (1999) developed an individual-based model distinguishing suburban and conservation lands that were assumed to be optimal habitat from unsuitable urban areas. Florida scrub-jay territories within conservation lands often occur as mixtures of habitat that do not transition between states uniformly and where territories function as sources and sinks within individual populations (Breininger and Carter, 2003; Breininger and Oddy, 2004; Breininger et al., 2009, Breininger et al., 2023). These complexities inspired collaborations among stakeholders, land managers, and biologists to develop ARM projects to enhance species recovery (Johnson et al., 2011; Eaton et al., 2021).
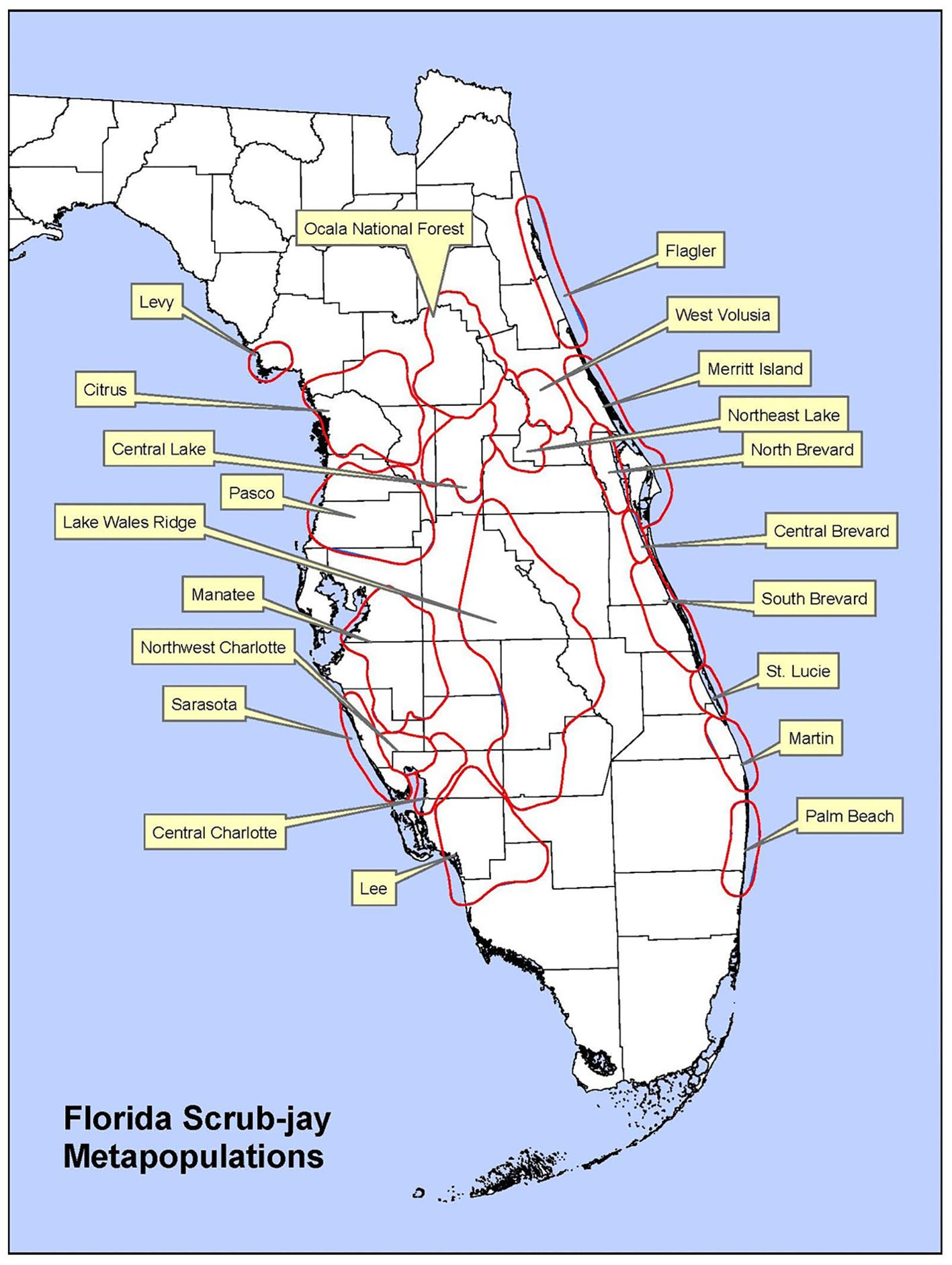
Figure 1. Florida scrub-jay metapopulations. From U.S. Fish and Wildlife Service (2019b).
Management of threatened species usually focuses either on habitat projections and options or on population viability assessments and actions to improve population demography. Even when both habitat and population dynamics are considered, the analyses are usually independent, and the resulting actions considered as alternatives rather than as an integrated strategy. The earlier PVA on this FSJ metapopulation concluded that it was comprised of populations that were all too small and isolated to support long-term persistence (Lacy and Breininger, 2021), while the habitat modeling presented a bleak picture of projected decline in the quality of the remaining patches of habitat (Eaton et al., 2021). Neither approach identified a strategy that was likely to restore adequate habitat and recover this metapopulation. This study integrates updated habitat projections from the ARM project within the PVA to account for ongoing or projected changes to the environment and links habitat to demography. This linked habitat-population model provides new insights into how the combination of habitat and population management actions can stabilize the metapopulation and achieve long-term viability.
2 Methods
Many of the methods here are based on population sizes (tallied as number of breeding groups), estimates of demographic rates, and dispersal rates parameterized and assembled in a recent PVA (Lacy and Breininger, 2021) based on >30 years research and monitoring that has occurred in the east coast Florida metapopulations of the FSJ. These previous studies are well characterized in dozens of published articles (e.g., Breininger et al., 2009, Breininger et al., 2023; Breininger and Oddy, 2004; and see Introduction). Key features are summarized below.
2.1 Population delineation and habitat classification
Fourteen populations were delimited by identifying those habitat areas that were separated by at least 667 m at the closest points or are closer but separated by barriers to dispersal. Florida scrub-jays occasionally disperse across greater distances, but observed inter-population dispersal is insufficient to lead to genetic panmixia or regular demographic reinforcement (Lacy and Breininger, 2021). The metapopulation in south and central Brevard County mainland has not been observed to exchange individuals with either of two adjacent metapopulations – a much smaller north Brevard metapopulation or a Canaveral/Merritt Island metapopulation.
Established breeding pairs rarely move their territory, other than adjusting boundaries in response to opportunities and inter-group conflicts, and breeding pairs have not been observed to disperse between populations (Breininger et al., 2006). Dispersal rates of helper jays between each pair of populations were determined from the number of observed movements of banded helper jays from the natal population to a destination population, where they usually attempted to become breeders. Frequencies of movements relative to the total bird-years of banded helpers were used to create a matrix of inter-population dispersal rates (Lacy and Breininger, 2021).
As a component of the ARM program, FSJ habitat across the subpopulations in southern and central mainland Brevard County has been assessed by land managers and local biologists annually since 1997 (Eaton et al., 2021). The extent of accessible habitat in each population was overlaid with a total of 249 grid cells of 10 ha, approximating the size of scrub-jay territories. These grid cells will be referred to and treated in our model as “potential territories” (Breininger et al., 2006). The number of grid cells of suitable habitat was used to estimate the carrying capacity (K) for breeding groups in each population, except that three populations received supplemental feeding from park visitors resulting in smaller breeding territories, increasing local population sizes. For these food-supplemented populations, carrying capacity was estimated to be the current number of breeding groups because they showed elevated fecundity and no evidence of density-dependent reductions in survival (Breininger et al., 2022, Breininger et al., 2023). The resulting total metapopulation size (K) was 266 potential territories, with 168 of these occupied by breeding groups in 2023.
Analysis of wildlife habitat requires dual consideration of the characteristics of the environment that are changing and potentially modified through management and the consequent quality of that environment as habitat for the species (Nichols et al., 2024). Potential territories have been classified into five habitat states defined by vegetation height and extent of ground cover (Breininger et al., 2014; Eaton et al., 2021). Habitat states were consolidated and renamed to describe quality with respect to FSJ demography (Breininger et al., 2010; U.S. Fish and Wildlife Service, 2019b) and these simplified categories were used in the PVA (Lacy and Breininger, 2021). “Optimal Open” (OpO) habitat had medium-height (1.2 to 1.7 m) oak scrub and open sandy areas interspersed between scrub patches. This habitat state was named “Strong” with respect to its suitability for FSJs when it was determined that this habitat supported the best reproduction and survival (Breininger et al., 2009, Breininger et al., 2014, Breininger et al., 2023), with an estimated 7.0% potential population growth per year (Table 1). “Optimal Closed” (OpC) habitat had similar height oak scrub but with few open sandy areas. It was named “Weak” owing to the lower reproduction and survival in this habitat, resulting in projected population decline of 2.9% per year. “Short” (Sh) sites had vegetation that was mostly below 1.2 m. “Tall-mixed” (TM) had short or medium-height scrub with patches of oak > 1.7 m in height and > 0.4 ha in size. “Tall” (T) had patches of oak > 1.7 m in height and > 0.4 ha in area, and no short or medium-height scrub or open areas. Sh, TM, and T vegetation were named collectively as “Sink” habitat, as they have lower reproduction and survival (Breininger et al., 2014), resulting in a projected 10.4% decline per year. We will use the five vegetation labels (Sh, OpO, OpC, TM, and T) when describing projections of vegetation dynamics, and the three habitat quality labels (Strong, Weak, and Sink) when describing consequences for FSJ demography.
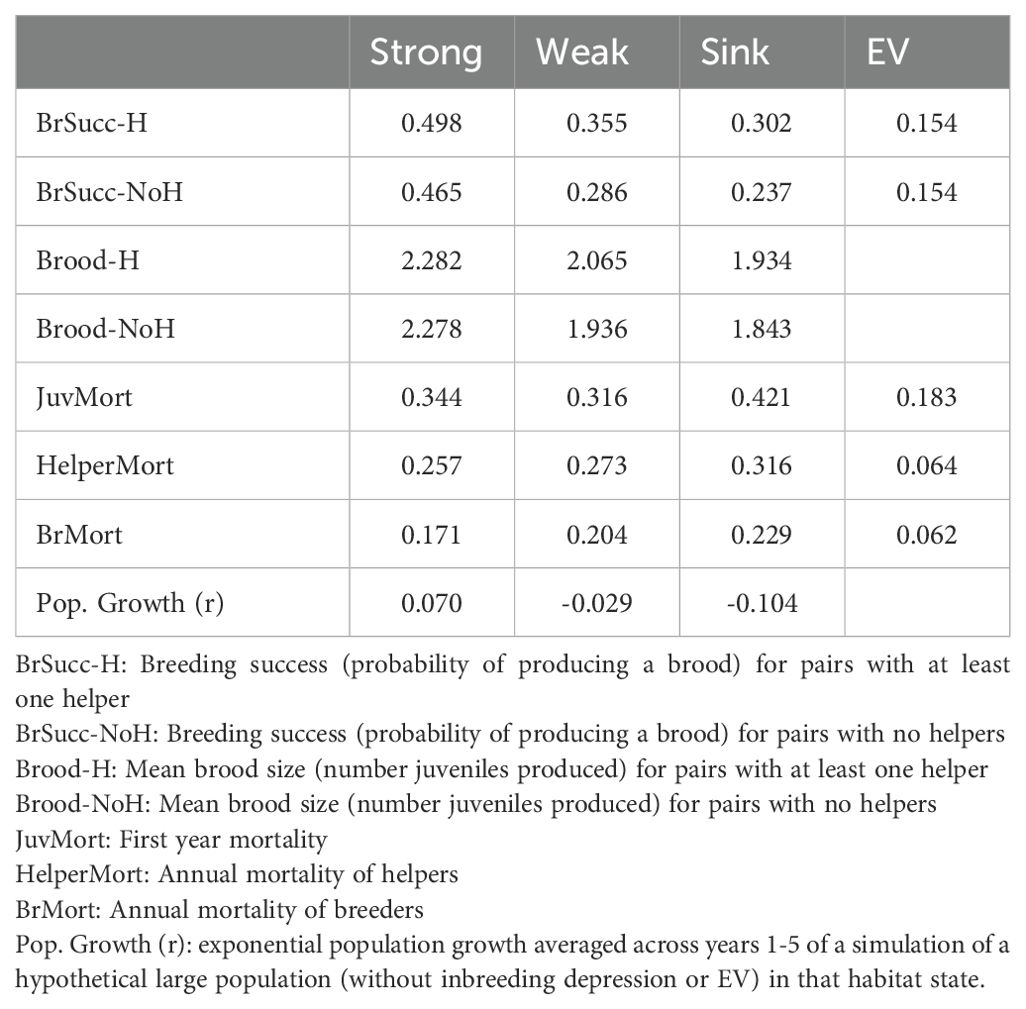
Table 1. Mean demographic rates and annual fluctuations (EV) in those rates for Strong, Weak, and Sink FSJ habitat.
Population projections were made for both hypothetical isolated populations (for testing effects of model parameters) and for the actual configuration of 14 populations in the metapopulation, with occasional inter-population dispersal as estimated from a distance function derived from data on inter-population movement frequencies (see below). For the analyses of isolated populations varying from 50 to 300 potential territories, the initial distribution of habitat states was set to the observed distribution across the metapopulation (0.492 OpO, 0.053 OpC, 0.094 Sh, 0.353 TM, 0.008 T) tallied in 2023 surveys by local biologists and land managers, resulting in 0.492 Strong, 0.053 Weak, and 0.455 Sink habitat with respect to the quality affecting the FSJ demography. The initial proportion of potential territories in each state occupied by breeding groups was similarly set to the metapopulation means observed in 2023 (0.847 of Strong, 0.786 of Weak, 0.380 of Sink; and 0.632 of all potential territories occupied). In analyses of the actual metapopulation, for each local population the initial number of potential territories in each of the five habitat states and the numbers occupied by breeding groups was set to the observed 2023 counts, using methods described by Eaton et al. (2021).
Due to successful implementation of new habitat management methods, the number of OpO potential territories increased dramatically from 2018 (n = 80) to 2023 (n = 131), accompanied by a parallel increase in breeding groups (111 to 168; 8.6% growth per year) in the metapopulation. To provide comparisons to the projections of habitat distributions and occupancy made by Eaton et al. (2021) based on data as of 2018, we also examined scenarios that started the distribution of habitat states (0.330 OpO, 0.116 OpC, 0.067 Sh, 0.446 TM, 0.041 T) and the occupancies of the states for each local population to the values that were observed in 2018.
2.2 Population demography
Components of reproductive success (% pairs producing juveniles, number of juveniles produced per pair) and survival rates were tallied from the long-term studies beginning in 1998 that include a census every month (Breininger et al., 2006, Breininger et al., 2009, Breininger et al., 2014, Breininger et al., 2023; Carter et al., 2023). Demographic rates were tallied each year from 1988 through 2021, separately for Strong, Weak, and Sink habitats, and separately for breeding pairs with no helpers vs with helpers (Table 1). Monthly detection probabilities for the field surveys that contributed to the estimation of demographic rates are usually greater than 0.90 using mark-recapture methodologies (Breininger et al., 2009, Breininger and Carter, 2003; Carter et al., 2023), so we used direct calculations of monitored groups rather than estimating rates from mark-recapture studies for a subset of years, as described by Lacy and Breininger (2021). For each level of habitat quality (Strong, Weak, and Sink), we estimated the annual variation in demographic rates due to fluctuations in the environmental conditions (the “environmental variation”, EV), by removing the expected binomial sampling variation from the total inter-annual variation in the data (Akçakaya, 2002; Lacy et al., 2023). Differences among Strong, Weak, and Sink habitats in EV for each demographic rate were non-significant, so each EV was pooled across habitats.
It was assumed that each local population was panmictic, other than for the avoidance of close inbreeding (see below), as the distances across each population can easily and readily be traversed by FSJs. However, FSJs most often establish new territories adjacent to (or sometimes budded off from) their natal breeding group (Breininger et al., 2006, Breininger et al., 2023), so it is possible that some localized inbreeding occurs above that generated in the simulation based on local population sizes and within-population breeding opportunities.
Inter-population dispersal rates were estimated from observed movements of FSJs from 1997 to 2008 (Breininger et al., 2006). Dispersal is almost exclusively departures of helper jays from their natal territories, after which they attempt to become established as breeders. Regression models were used to determine what function best fit the relationship of distance between nearest points for each pair of populations (D, in km) to dispersal rate (R) as the probability of a helper moving to the other population. The observed frequency of inter-population dispersal was found to be best fit with an inverse square-root model, with a regression equation of R = 0.0275/(√D), (SE(slope) = 0.0051; P < 0.00001, R2 = 0.1845) (Lacy and Breininger, 2021).
Florida scrub-jays are assumed to avoid mating with parents, offspring, siblings, maternal half-siblings, or paternal half-siblings (i.e., members of their breeding group), and we included this restriction on matings in the PVA model. We modeled any impacts of lower levels of inbreeding or accumulated inbreeding as a decrease in juvenile survival for inbred offspring, with the severity of inbreeding depression as estimated by Chen et al. (2016) (7.478 Lethal Equivalents for impacts on survival from 11-day nestling to yearling).
The prior PVA emphasized that local populations are currently too small and too isolated to ensure metapopulation viability, due to both demographic and genetic instability (Lacy and Breininger, 2021). Inter-population dispersal can be increased via habitat improvements, including both expansion of suitable habitat accessible to some populations and creation of corridors between some local populations, and via new experimental managed translocation of FSJs. To explore the value of increased connectivity, we examined some scenarios that included 3-fold or 5-fold more dispersal between each pair of populations.
The effect of habitat on overall population growth and persistence depends on both the extent of available habitat (number of potential breeding territories) and the distribution of habitat quality (Strong, Weak, and Sink). Florida scrub-jays respond to the availability of optimal habitat both in the likelihood of helpers leaving a natal group and attempting to become breeders in their own territory and in the likelihood that such new breeders will establish their new territory in optimal vs suboptimal habitat. The probability of a female helper transitioning to be a breeder was modeled as a logistic function: Pr[HtoBr] = exp(-1+B*pAvail)/[1 + exp(-1+B*pAvail)], with pAvail being the proportion of Strong potential territories unoccupied, and the slope parameter B defining the strength of the relationship. Male helpers were assumed to leave their natal groups to respond to opportunities to pair with females that were either transitioning from helpers to occupy new territories or were established breeders whose mates had died.
Next, the likelihood that a new breeder will establish her territory in Strong habitat was modeled by assuming that the female would choose a territory with Strong habitat with probability = pStrong^(1/Pref), with pStrong being the proportion of unoccupied territories that were Strong, and Pref being a parameter fitted to generate the observed proportion of occupied territories in Strong habitat. If a new breeder does not select a Strong territory in the simulation, then she is assigned to a Weak territory (rather than Sink) with probability equal to a similar exponential function of the proportion of Weak available territories among those that are not Strong: [pWeak/(1 – pStrong)]^(1/Pref). If neither Strong nor Weak are selected, then a new breeder is assigned to a Sink territory. Note that the likelihood of a new breeder establishing a territory in available Strong habitat might be not due only to the strength of behavioral preference for Strong habitat. The selection of a new territory might also be determined by the ability of the FSJs (and the researchers) to identify what is and will remain as the best habitat, and the accessibility of unoccupied Strong habitat. (E.g., even if Strong would be preferred, females transitioning to breeders might search for vacant habitat only close to their natal territory, and thereby end up settling in Weak habitat.)
After becoming breeders in new territories, jays were assumed not to revert to helper status and to remain in territories with the same habitat quality until they died.
Carrying capacity was imposed via a ceiling that removed helpers randomly if the population size exceeded 4 times the number of potential territories. The mean number of helpers per breeding group almost never exceeded 2 in our simulations, so this ceiling had no effect on population projections. However, a functional carrying capacity arises in our model mechanistically, in that as Strong territories become more fully occupied, fewer helpers transition to breeding status, and any new breeders are forced into suboptimal habitat with reproduction and survival rates that result in population decline in those Weak and Sink habitats.
2.3 Sensitivity analyses
Several of the variables that describe the behaviors of the scrub-jays in our models could not be estimated directly from available field observations, including the parameters that describe the probability that a helper will disperse from its natal group and attempt to become a breeder (parameter B) and the probability that such new breeders will establish territories in Strong vs Weak vs Sink habitat (Pref) – with both used in functions of the availability of vacant territories in each habitat state (see above). The baseline values of these parameters (B = 6, Pref = 6) were obtained by testing a range to see what values would result in populations with the helper:breeder ratio and proportional occupancies of Strong, Weak, and Sink habitat that approximate ratios observed in the field (Lacy and Breininger, 2021). For both parameters, we tested a range of 2 to 10 to determine how these aspects of FSJ social behaviors influence population viability and how sensitive the population projections are to the specific values chosen.
The sensitivity of results to estimated values of other model parameters (lethal equivalents, breeding success, juvenile and adult mortalities, and EV in reproductive and mortality rates) were examined in the prior PVA (Lacy and Breininger, 2021). While some (especially adult mortality) impacted population growth or probability of local extinction, within ranges considered plausible they were not found to have large effects on the relative comparisons among scenarios testing population structure and management options, and precise values assumed for each variable did not affect general conclusions about metapopulation viability and management recommendations.
2.4 Habitat projections
We projected changes in the number of Sh, OpO, OpC, TM, and T potential territories in each population over up to 100 years, starting with the distribution of habitat types observed in 2023, by applying the transition probabilities derived by Eaton et al. (2021) for each of four habitat management regimes (BURN, LMB, HMB, and NONE) applied in Oak woodland sites. The BURN regime used prescribed burning to maintain scrub vegetation. Light mechanical cutting followed by burning (LMB regime) employed cutting of taller vegetation to increase its flammability followed by a prescribed burn. Heavy mechanical cutting followed by burning (HMB regime) employs more extensive cutting of taller vegetation that would otherwise escape burning, followed by a controlled burn. The NONE regime applied no management to a site, allowing natural succession to occur. We applied BURN, LMB, and HMB regimes every 5 years, with intervening years subjected to no management. Each population, in each iteration, was assigned a random year from 1 to 5 as the first year for management to be applied. We also examined some scenarios with application of management actions on 3-year rotations. See Eaton et al. (2021) for more details on the management regimes that were explored and Nichols et al. (2024) for detailed discussion of the estimation of state transitions and the use of the projections in adaptive habitat management. We modeled transitions between habitat states probabilistically, with the fate of each potential territory sampled from the multinomial distribution determined for transition from its present state to its state in the subsequent year.
Managers have been exploring new techniques to improve habitat quality, and one new method (which we label DINO) uses an excavator with a forestry mulching attachment, commonly referred to as a brontosaurus mulcher (https://brownforestryproducts.com/), that reduces tall scrub to optimal height. This method has been most successful at transitioning TM to OpO in an annual time step resulting in a doubling of FSJ local population sizes in 2-3 years in several sites (Breininger, unpublished data). To represent the plausible effects of this new management regime, we tested scenarios that have much greater probabilities of transition from TM to OpO, given that this approach has been found to be much more successful for creating optimal habitat than was projected for any of the four previous management regimes (Eaton et al., 2021). The DINO method is meant to supplement the use of fire because mechanical cutting of tall scrub is often first needed prior to burning (Schmalzer and Boyle, 1998). For the DINO regime, we use a set of habitat transition probabilities that plausibly represents the new system being used by land managers (Supplementary Table S1).
2.5 Vortex PVA model
The FSJ population dynamics were modeled using the Vortex (version 10.7.0) population viability analysis software (Lacy, 2000a; Lacy et al., 2023; Lacy and Pollak, 2024; software and manual available at https://scti.tools/vortex/). Vortex is an individual-based model that simulates the fate of each individual through an annual cycle of breeding, mortality, increment of age, dispersal among subpopulations, removals (or emigration from the population), supplements (managed or natural immigration), translocations, and truncation if the population exceeds the carrying capacity (ceiling density dependence). Stochasticity in demographic processes is modeled as annual variation in each demographic rate at the population level (environmental variation) and random sampling variation in the fates of individuals (demographic stochasticity) (Lacy, 2000a, Lacy, 2000b). Individual fates are summed to provide projected population sizes, population growth rates, population age and sex structure, and probabilities and times to local extinction. Vortex projects the effects of population size and structure on loss of genetic diversity, and in turn the impacts of loss of diversity on fecundity and survival (inbreeding depression). Vortex provides the flexibility to specify demographic parameters as functions of environmental (e.g., climate, prey base), population (e.g., density, social structure), or individual (e.g., age, social status, inbreeding, genotype) properties through the use of state variables to track properties of the system (Global State Variables), each local population (Population State Variables), and each individual animal (Individual State Variables).
Details of the Vortex model structure and parameter values used to model the complex demographic, social, and other population processes driving FSJ population trajectories are given in a report of the earlier PVA that did not include linking to dynamic projections of habitat, but instead tested scenarios with various fixed values for the distribution of habitat quality in each population (Lacy and Breininger, 2021). Projections were run for 100 years, with 500 independent iterations of the population trajectories in each scenario. Tests with more iterations showed that 500 iterations provided adequate precision of means and variances to allow for comparison among scenarios.
2.6 Measures of metapopulation viability
We assessed metapopulation viability by the population size (number of breeding groups), probability of metapopulation persistence, median time to extinction (when extinction probabilities exceed 50%), quasi-extinction (probability that the population declined below 10 breeding groups), and the proportion of initial gene diversity remaining and the inbreeding coefficient averaged across those iterations in which the metapopulation was not extinct. We present population trends as the number of breeding groups, quasi-extinction, and mean genetic metrics over the first 50 years. The 50-year time frame is likely longer than the planning and management focus, but such projections can be useful because longer term consequences might not be apparent for decades, even if processes that will lead to demographic and genetic decline are underway earlier. The final extinction of a population in decline can take even longer, so the probabilities that the population will be extinct (reduced to no animals or just one sex) are presented over the full 100-year projections.
2.7 Integrating habitat transitions into the PVA
The inclusion of habitat projections as drivers of demography in the Vortex population model was implemented by specifying the habitat transitions each year within macros. The macros step through each potential territory, each simulation year, and determine its next habitat state by sampling the multinomial transition probabilities. In addition to the scenarios simulating five management regimes (BURN, LMB, HMB, NONE, and DINO), a “NoHab” scenario was modeled in which habitat remained constant at the distribution of states observed in 2023 (with neither management nor natural transitions of vegetation). The Vortex project files, data files accessed in the Vortex scenarios, and macros used in the Vortex model to implement habitat projections are available in a Zenodo repository at 10.5281/zenodo.13850866.
2.8 Population management options
Four population management options and combinations of them were explored to determine if they would slow, prevent, or reverse decline of the breeding population and ensure long-term viability of the metapopulation. These options for managing the FSJs and the configuration of populations were applied on top of an assumption that the improved management of habitat, represented by the DINO scenario, would be used into the future.
A “Connect” scenario assumes that several sets of populations can be functionally merged through protection and restoration of corridors of habitat. The connected populations are Jordan + Valkaria + Malabar (creating a single population of currently 42 breeding groups in an area that can support 78 territories), N Sebastian Conservation Area + Coraci + N Fork (creating a population of 43 breeding groups in an area that can support 50 territories), and Carson Platt + Corrigan (creating a population of currently 24 breeding groups in an area that can support 46 territories). These merged populations would be larger than any of the existing populations which have a maximum size of 34 potential territories (see Supplementary Table S2).
Two scenarios, “Dx3” and “Dx5”, assume that inter-population dispersal can be increased 3-fold or 5-fold relative to the distance-dependent estimates from prior observed dispersal rates. Such increased dispersal might be achieved through increased protection of intervening landscapes or might occur as a result of populations becoming more saturated causing more birds to disperse from natal populations.
Two translocation scenarios tested the impact of translocation of FSJs among populations. A total of 15 or 30 FSJs were translocated each year in scenarios “Trans15” and “Trans30”, with the number of jays removed from each donor population proportional to the number of occupied Strong territories in that population, and the number of FSJs moved into each recipient population proportional to the number of unoccupied Strong territories in the recipient population. For example, if one population has 20 occupied Strong territories out of 100 total in the metapopulation, then 6 of the 30 FSJs selected to be translocated in the Trans30 scenario would be taken from that population. If a second population had 10 of 60 unoccupied Strong territories, then it would receive 5 of the 30 translocated FSJs. Fractional numbers were stochastically rounded, and the scrub-jays to be moved from each population were chosen at random on the assumption that capture of FSJs for translocation would not be able to target specific birds.
A “Feed” scenario assumes that 1.5x more breeding groups can occupy a given area of Strong habitat if they are provided with supplemental feeding. Increases in numbers above what can be supported in 10 ha of habitat (the estimated mean territory size in the absence of feeding) have been observed in three populations (Viera, N Sebastian Conservation Area, and Wabasso) that do receive supplemental food from visitors, partly because of increased fecundity and density without evidence of decreased survival (Breininger et al., 2022; Breininger et al., 2023).
All combinations of Connect, Trans15 or Trans30, and Feed options were also assessed (Supplementary Table S4).
3 Results
3.1 Baseline projections in the absence of habitat transitions
Simulations of hypothetical isolated populations in habitat that can support 100 territories (i.e., 1000 ha of contiguous habitat), initially 50% occupied by breeding groups, confirmed that the demographic rates observed in territories with Optimal Open habitat (OpO, deemed “Strong”) result in positive population growth (mean exponential growth over first 5 years of simulations: r = 0.060). Optimal Closed habitat (OpC, deemed “Weak”) does not support positive growth (r = -0.043). Short, Tall Mix, and Tall (collectively deemed “Sink”) results in faster population declines (r = -0.120) and extinction usually occurred within 15 to 30 years (Figure 2). These growth rates are all lower than projected in Table 1, because of stochastic effects in the smaller populations. Larger populations in Weak and Sink habitat persisted a few years longer, but ultimately suffered the same fate, with median times to extinction of 52 and 29 years for populations with 300 potential territories (3000 ha) of Weak or Sink habitat, respectively (Supplementary Figure S1). Although the separation among populations in Strong, Weak, and Sink habitat is clear for the mean population trajectories, there was considerable stochastic variation among the independent iterations (as shown by the +/- 1 SD dashed lines in Figure 2A).
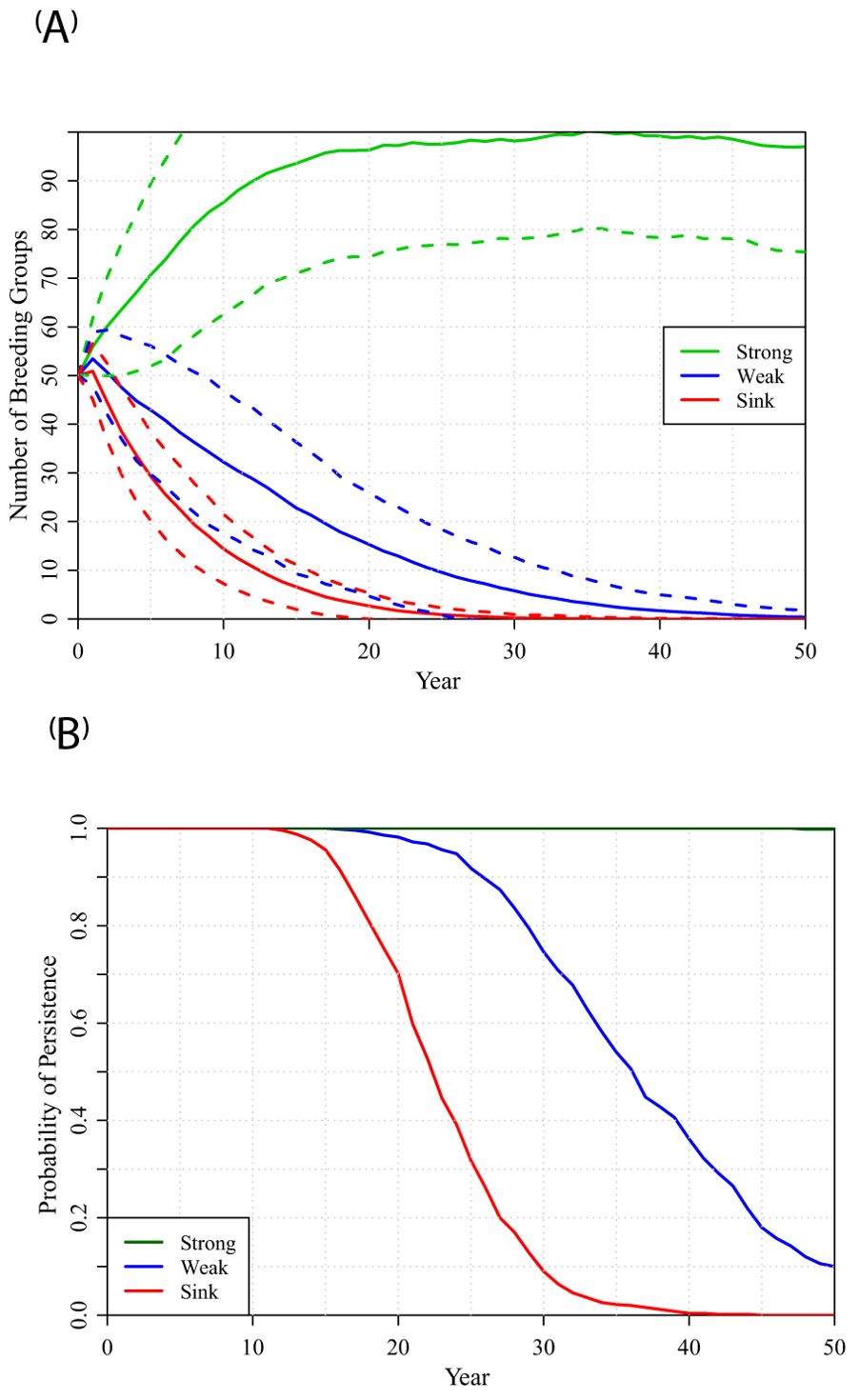
Figure 2. Mean trajectories projected over 50 years for populations in 100 potential territories, with initially 50 occupied, of all Strong, Weak, or Sink habitat (top to bottom, respectively): (A) number of breeding groups, with +/- 1 SD range across iterations shown as dashed lines; (B) probability that the population persists.
Simulations of populations in all Strong habitat able to support from 25 to 250 potential territories, initially 50% occupied, showed rapid initial population growth, followed by stability with most or all habitat occupied when there were 75 or more potential territories (Supplementary Figure S2A), and few or no extinctions occurred within 100 years if the total area of habitat was more than 75 potential territories (Supplementary Figure S2B).
Simulations of isolated populations of varying size, each with the current (2023) proportions of Strong (49.2%), Weak (5.3%), and Sink (45.5%) habitat and proportions initially occupied (84.7%, 78.6%, and 38.0%, respectively), showed that the populations with this mix of habitat states are projected to decline slowly, as the number of breeding groups in Sink and Weak habitats declines (Supplementary Figure S3A). A population with fewer than about 150 to 175 potential territories (74 to 86 in Strong habitat) is vulnerable to extinction within 100 years (Supplementary Figure S3B). Very small, isolated populations, with only 25 potential territories, have a median time to extinction of 46 years. Ten of the 14 existing local populations have fewer than 25 potential territories, and all 14 have fewer than 35, so larger areas of contiguous habitat or increased connectivity among the populations will be necessary for long-term conservation.
Simulation of the current (2023) configuration of 14 populations with a total of 266 potential territories, under an assumption that habitat will remain as it is (the NoHab scenario), projected steady decline of the metapopulation, with a few local populations remaining stable or growing (primarily as a result of immigration from nearby larger populations), and most populations declining (Figure 3A). All populations had some chance of extirpation within 50 years (Figure 3B). The probability of metapopulation extinction within 50 years was less than 1%, but was 67% over 100 years, as the mostly isolated populations become increasingly inbred.
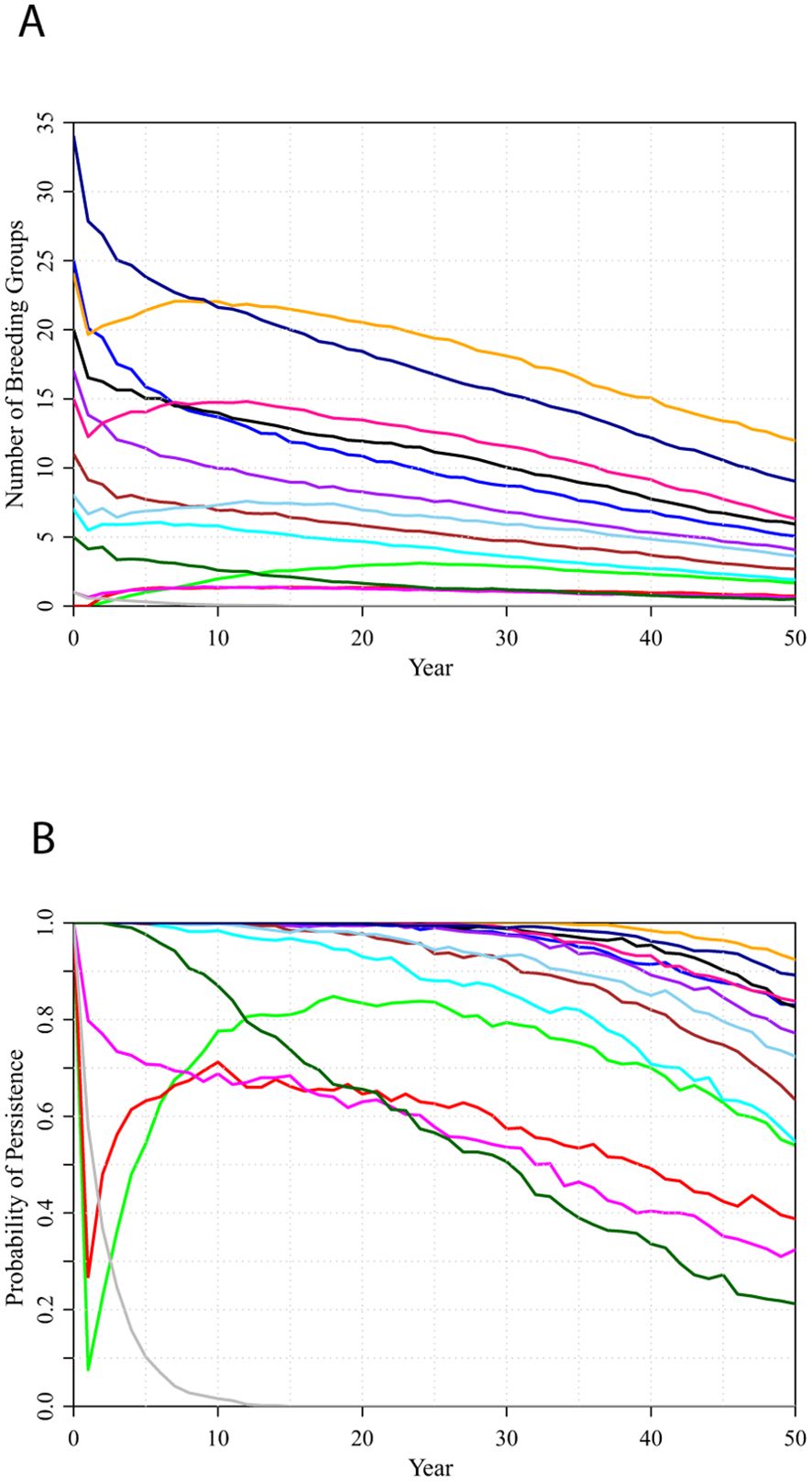
Figure 3. Trajectories projected over 50 years for the 14 populations comprising the metapopulation, under the assumption that proportions of Strong, Weak, and Sink potential territories remain constant (NoHab scenario): (A) number of breeding groups; (B) probability the population persists.
3.2 Habitat projections
Supplementary Figure S4 shows projected proportions of each habitat state under the five management regimes and the scenario of no habitat change. BURN management leads to more optimal (OpO) habitat and less sink habitat (predominantly TM) than do LMB or HMB, and each of these management regimes outperforms a lack of active management (NONE scenario). All four of these scenarios project long-term proportions of the habitat states that are similar to the steady state proportions projected by Eaton et al. (2021). However, these management regimes all predict that much of the currently OpO habitat will convert to TM (and to a lesser extent to OpC), with projections that the current nearly 50% OpO will decline to about 20% over the next 15 years (three cycles of active management). A lack of management (NONE) results in only about 11% remaining in optimal (OpO) state. In contrast, as a result of new management methods being applied since 2018, the metapopulation trend over the past 5 years has been the reverse, increasing from 33% to 49% OpO, while Weak (OpC) decreased from 12% to 5%, and Sink (Sh + TM + T) decreased from 55% to 45%. We do not yet have data sufficient to estimate transition probabilities for the newly implemented management, but sets of transition probabilities that increase OpO (e.g., the DINO scenario; Supplementary Table S1) can approximately mimic the changes that occurred in the past five years, and they result in a steady state distribution that maintains proportions of Strong, Weak, and Sink habitats close to those observed in 2023 (Supplementary Figure S4). For example, the decrease in TM projected from 2018 with the DINO transition probabilities almost exactly matched the projection starting with 2023 proportions beginning 5 years later (Supplementary Figure S5). The projections starting with the 2018 distributions of habitat drop in 5 years to the proportion observed in 2023, indicating that the DINO transition probabilities mimic the reduction in TM that was achieved by improved management methods.
3.3 Population projections in a dynamic habitat
The metapopulation is expected to decline to 23% occupancy (equivalent to about half of Strong habitat occupied) within 50 years if habitat is assumed to remain as it is in 2023 (Figure 4: top line, NoHab scenario), updating the analyses done previously (Lacy and Breininger, 2021). The metapopulation is expected to persist for 50 years, but then be at increasing probability of extinction over the following 50 years (Figure 4B). Under no active management (NONE scenario: bottom, blue lines), population decline is more rapid and there is a 47% probability of extinction within 50 years. HMB, LMB, or BURN management slows decline only a small amount relative to no active management and delays extinction by up to about 10 years. Under a scenario with habitat state transition probabilities that more closely project the habitat improvements achieved in the past five years (DINO scenario: black lines), population viability is considerably improved relative to the other four management scenarios, although the metapopulation trajectory still falls below the projection that assumes no change in habitat. All viability metrics for the habitat management scenarios are provided in Supplementary Table S3.
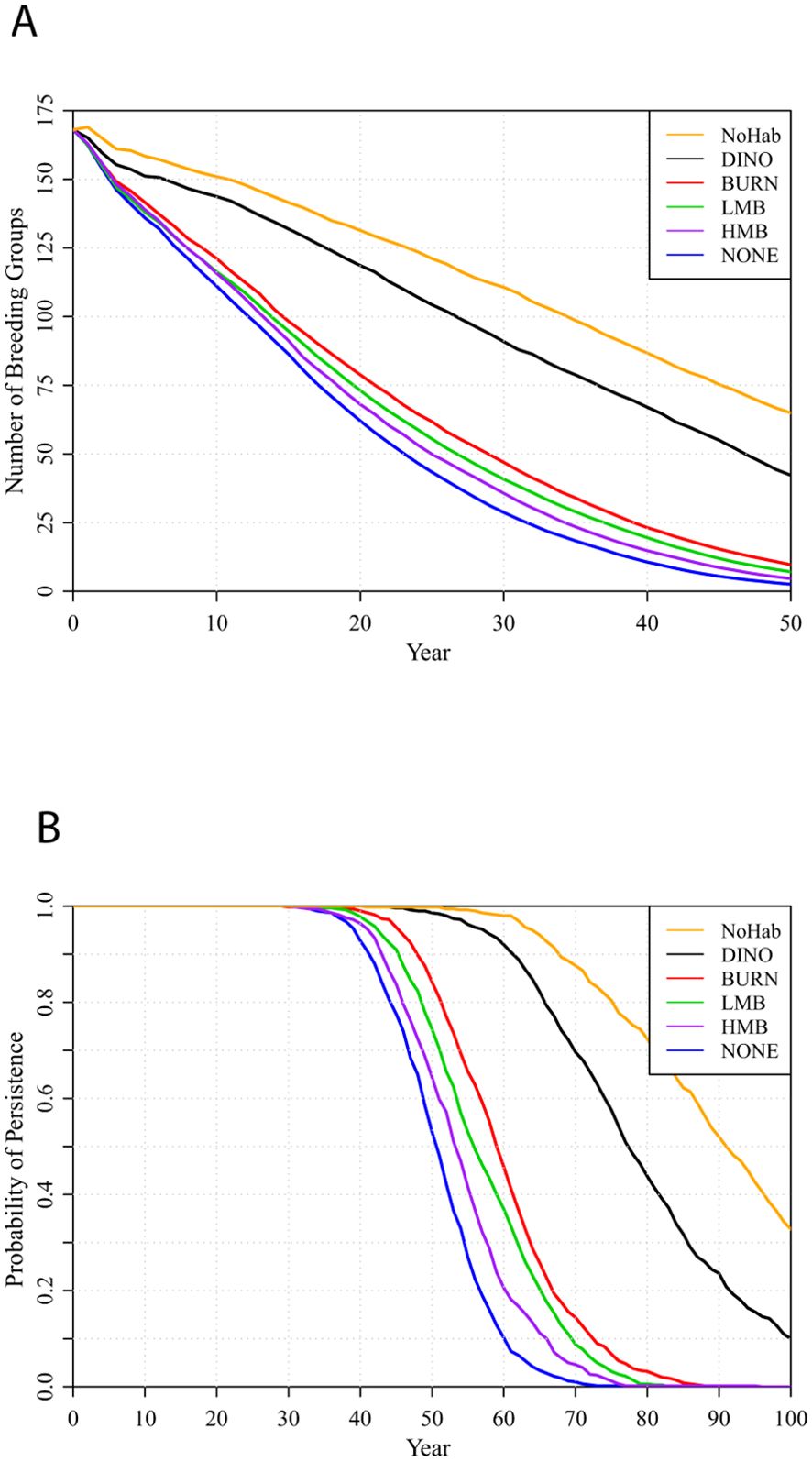
Figure 4. Metapopulation projections when habitat change under the several management scenarios is included in the PVA (top to bottom: NoHab, DINO, BURN, LMB, HMB, NONE) (A) number of breeding groups and (B) probabilities of metapopulation persistence. Probabilities of persistence are graphed through 100 years, because most extinctions occur between 40 and 100 years.
3.4 Sensitivity analyses
3.4.1 Baseline model parameters
The parameter (B) in the logistic function that was used to describe the probability that a helper would disperse from its natal group and attempt to become a breeder was varied from B = 2 (relatively weak dependency on the proportion of Strong habitat that is vacant) to B = 10 (very strong dependency). Within this range, the projections of population size and times to extinction varied relatively little, with slightly better population trajectories when dispersal of helpers to become breeders was more strongly dependent on the availability of Strong habitat. Supplementary Figure S6 shows the comparisons for DINO management. Comparisons across values of B for HMB, LMB, BURN, and NONE management were similar, but with less spread among lines.
The parameter (Pref) in the exponential function that was used to describe the probability that a new breeder would establish a territory in Strong habitat was varied from Pref = 2 (relatively weak dependency on the proportion of vacant potential territories that was Strong habitat) to Pref = 10 (very strong dependency). Within this range, the projections of population size varied relatively little, although median time to extinction was delayed by up to 20 years when the preference for (or accessibility of) Strong habitat was increased. Supplementary Figure S7 shows comparisons for DINO management. Comparisons for HMB, LMB, BURN, and NONE management were similar, but with less spread among lines. Thus, as expected, if new breeders are more likely to establish territories in the best available habitat, population viability is improved, although not to an extent that would cause us to question the selection of Pref = 6 in our model.
3.4.2 Inter-population dispersal and connectivity
Population projections and times to extinction under each management scenario are significantly improved if dispersal is more frequent than we initially estimated, or dispersal is increased by expansion of local population boundaries or creation of corridors between populations. Figure 5 shows the effect of 3-fold or 5-fold increase in inter-population dispersal and the projection if the metapopulation is fully interconnected and panmictic for the BURN management scenario. Under panmixia, the metapopulation would consist of 70 occupied territories at 50 years (while the management sustained about 62 Strong, 35 Weak, and 169 Sink potential territories) with 93% probability of persistence through 100 years.
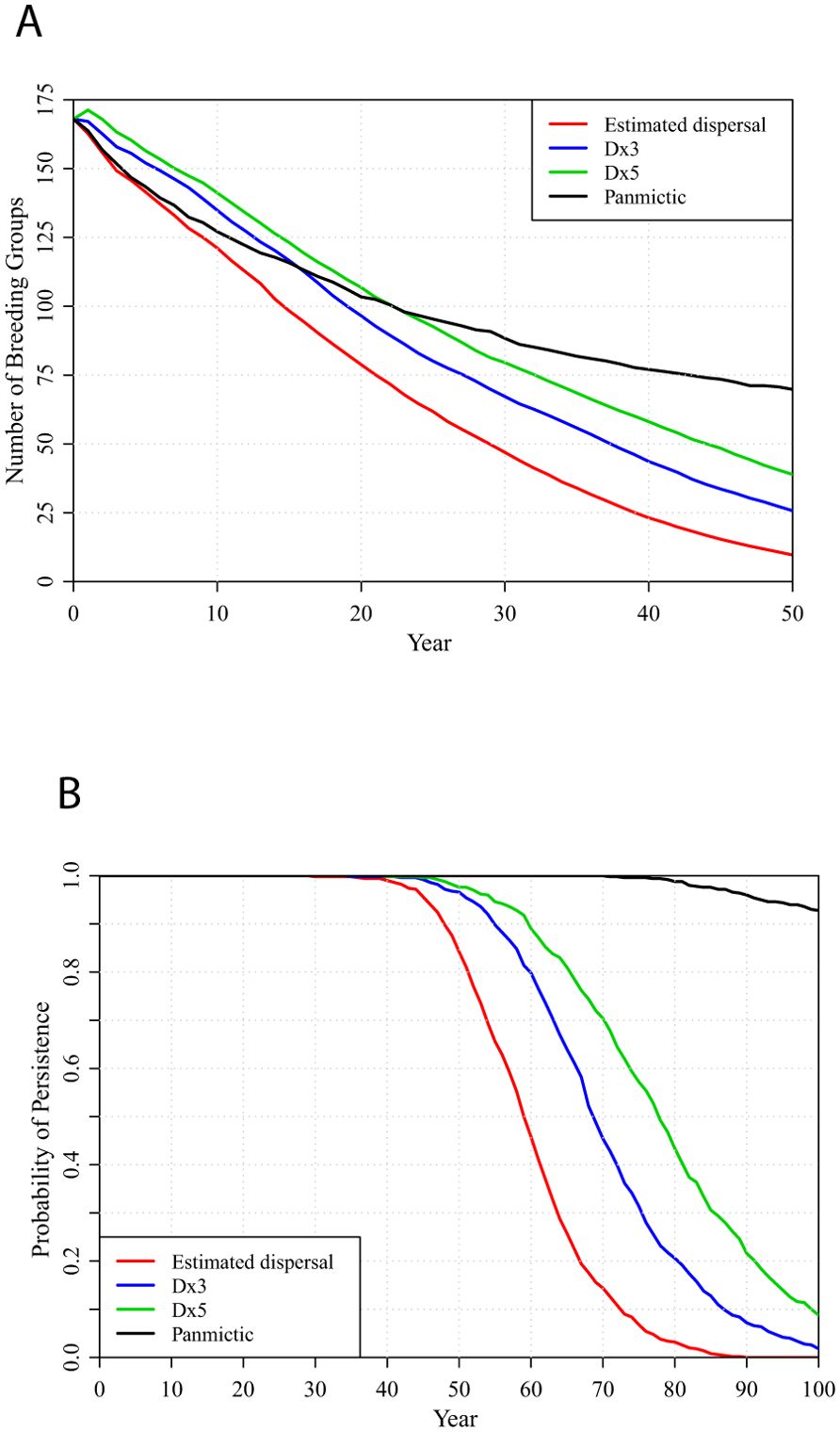
Figure 5. Metapopulation projections under the BURN management scenario, with the estimated, 3-fold increase (Dx3 scenario), 5-fold increase (Dx5) inter-population dispersal or complete panmixia (bottom to top): (A) number of breeding groups and (B) probabilities of metapopulation persistence over 100 years.
If management can continue to replicate the improvements to habitat that were obtained since 2018 (e.g., with the DINO management scenario), the benefits of increased connectivity are even greater (Figure 6). Under panmixia, the metapopulation would consist of 143 occupied territories at 50 years (while the management sustained about 119 Strong, 36 Weak, and 111 Sink potential territories) with 99% probability of persistence through 100 years. NONE, LMB, and HMB management scenarios also perform better if there is panmixia than otherwise, but they do not sustain the numbers of breeding groups as well as the BURN strategy nor assure metapopulation persistence (Supplementary Figure S8).
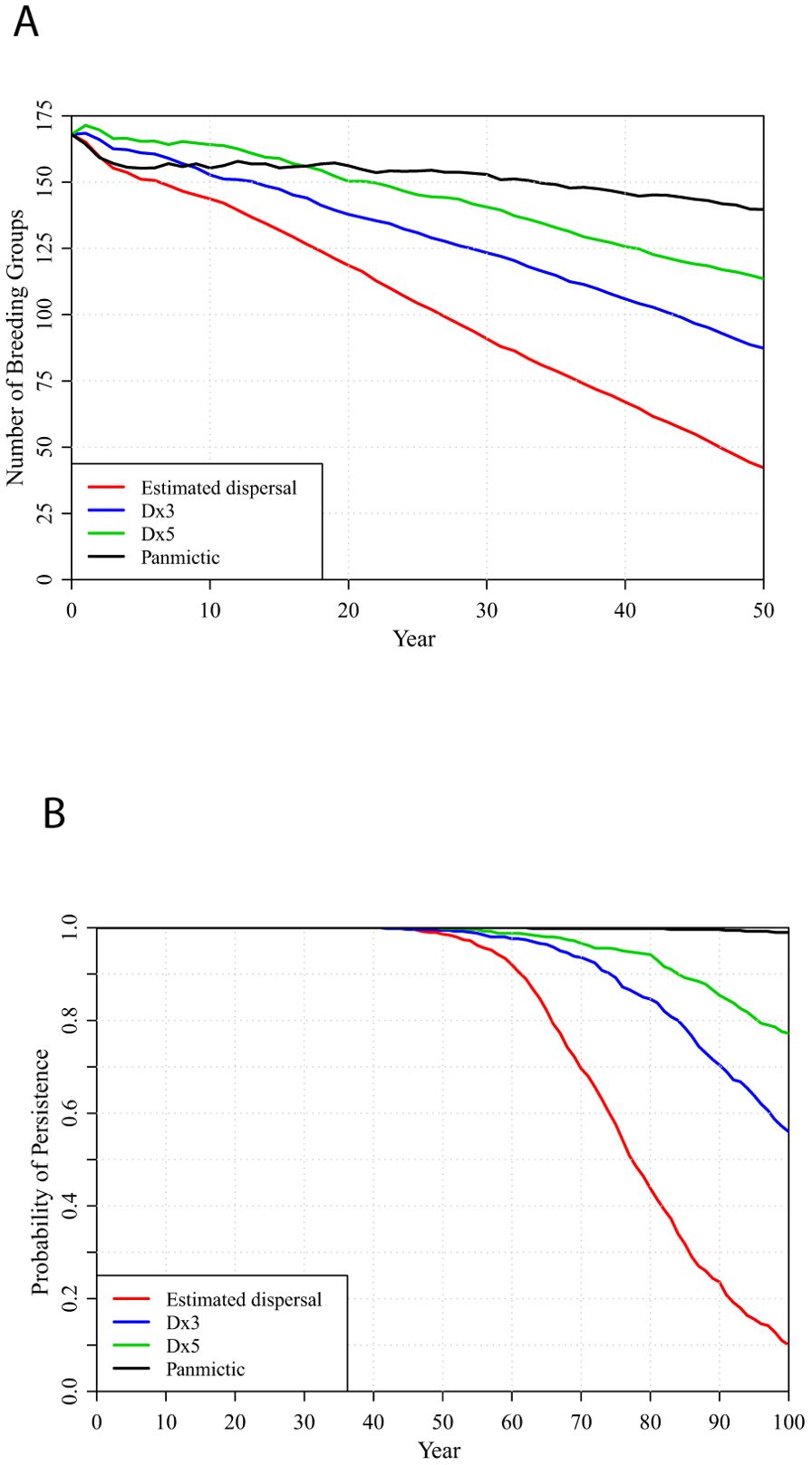
Figure 6. Metapopulation projections under the DINO management scenario, with the estimated, 3-fold (Dx3 scenario), 5-fold (Dx5) inter-population dispersal or complete panmixia (bottom to top): (A) number of breeding groups and (B) probabilities of metapopulation persistence over 100 years.
3.4.3 Management frequency
Part of the reason that the BURN, LMB, and HMB management regimes do not lead to much better metapopulation viability is because the active management is applied only once per 5 years (with the starting year randomized across local populations), and the other 4 years in each cycle experience habitat state transitions that occur with the NONE management. To test if management might be significantly more successful if it were applied more often to each population, we examined scenarios with each management action applied every 3 years (Figure 7).
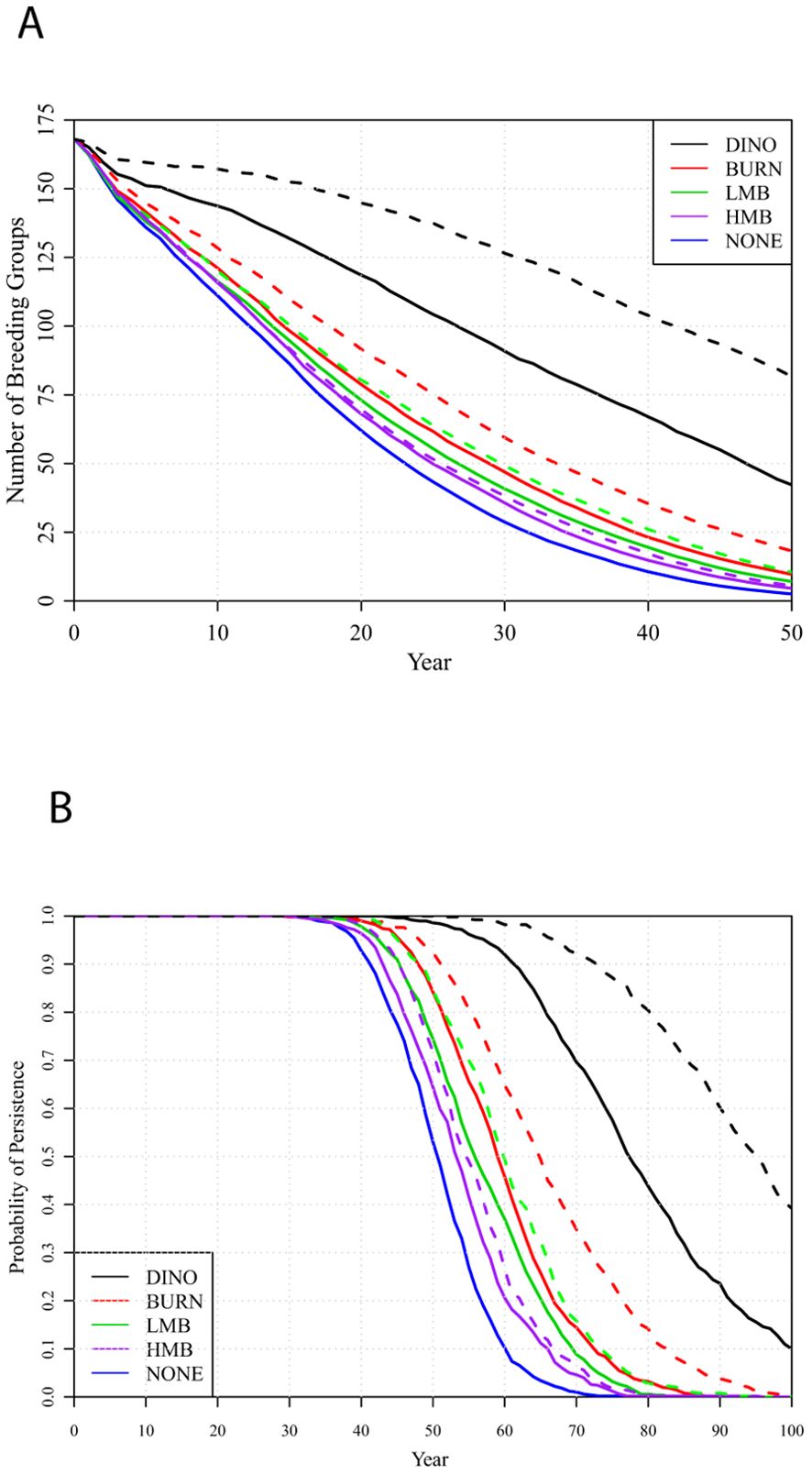
Figure 7. Metapopulation projections under each management scenario (top to bottom: DINO, BURN, LMB, HMB), applied every 5 years (solid lines) or every 3 years (dashed lines) or with no active management (bottom line): (A) number of breeding groups and (B) probabilities of metapopulation persistence over 100 years.
Shortening the cycle of active management improves efficacy of the DINO management (top two lines), almost doubling the breeding population at year 50 and increasing the probability of metapopulation persistence for 100 years from 10% to 39%. The improvement in the BURN management (next two lines) was more modest, increasing breeding population at year 50 from 10 to 18 and not avoiding the almost certain extinction within 100 years. LMB (next two lines down) improved population size only from 7 to 11 breeding groups after 50 years and delayed median time to extinction only from 56 to 60 years. A 3-year cycle of HMB (next two lines) provided almost no improvement over the 5-year cycle, and HMB provided only a little better protection for the metapopulation than did no active management (bottom line). Further viability metrics for 5-year and 3-year intervals of habitat management are given in Supplementary Table S3. There was substantial variation among independent iterations of the simulations, as shown by the SDs in Supplementary Table S3, but averaged across the 500 iterations the differences among mean trajectories for different scenarios was consistent.
3.5 Population management options
Projections with 3-fold or 5-fold increases in interpopulation dispersal demonstrated the benefits of increased connectivity. Possible options for more direct management of the FSJ populations were therefore coupled with the DINO improved habitat management. The individual options for population management (Connect, Feed, Trans15, and Trans30) were not sufficient to prevent ongoing decline in population size, resulting in a substantial risk of metapopulation extinction within 50 to 100 years (Figure 8, Supplementary Table S4). An increase in the number of breeding pairs that can be accommodated within a given extent of Strong habitat, represented by the Feed option, improved the population relative to not implementing any of the population management options, but inbreeding still accumulates to levels (mean = 0.062, equivalent to first-cousin matings) that would depress first-year survival and thereby result in the metapopulation size eventually falling below most other management options. Connecting the three sets of nearby populations (Connect option) reduces the accumulation of inbreeding and reduces the probability of extinction relative to the Feed option, but results in a similar decline in metapopulation size. Translocating 15 FSJs per year moves almost as many FSJs between populations as occurs with a 3-fold increase in natural dispersal (Trans15: mean = 21.4 scrub-jays move annually via natural dispersal plus the translocations, in the first 5 years before population decline; Dx3: mean = 22.8 move annually), but managed translocations move FSJs selectively from crowded populations to more sparsely occupied ones, rather than moving mostly to adjacent populations as occurs with enhanced natural dispersal, and this results in slower accumulation of inbreeding with managed translocations (Supplementary Table S4). The Trans30 management strategy approaches the benefits projected with 5x dispersal.
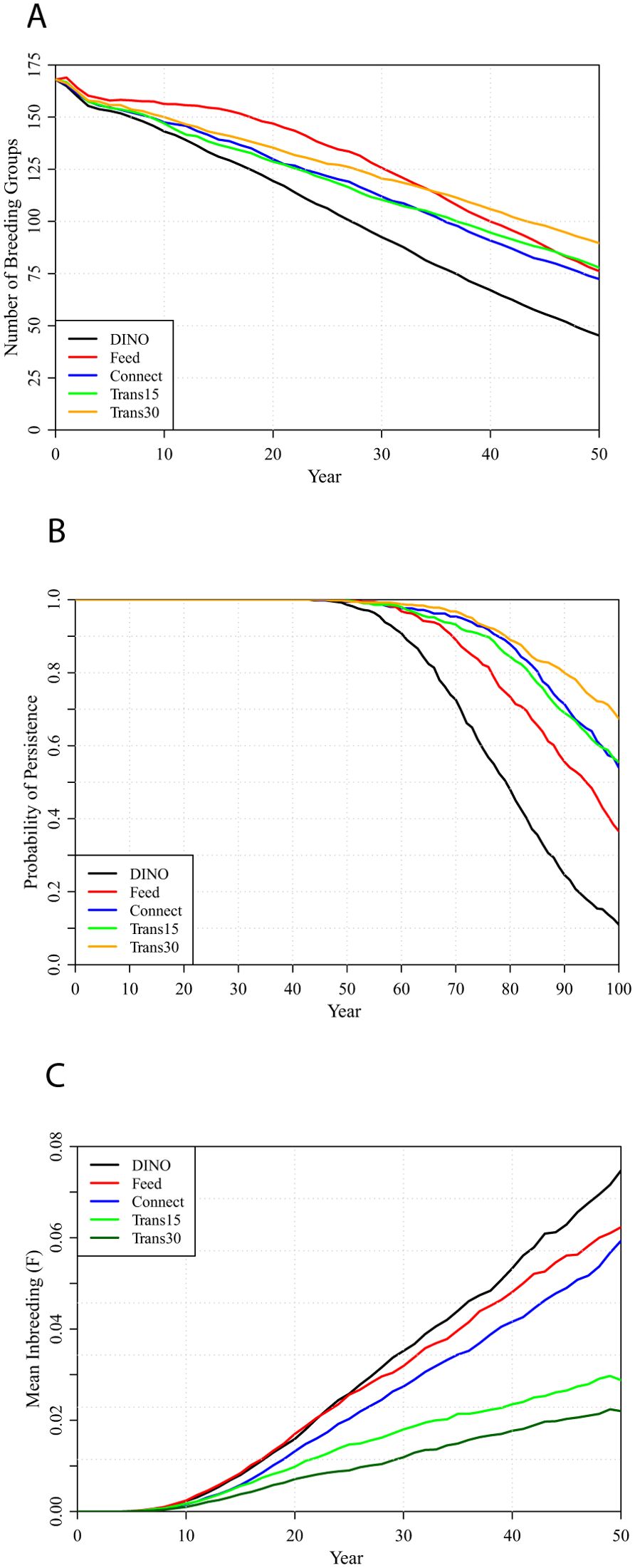
Figure 8. Metapopulation projections under several population management scenarios, each applied with DINO habitat management applied every 5 years: DINO habitat management only (bottom line), or with population management connecting 3 sets of adjacent populations (Connect), translocating 15 or 30 FSJs per year (Trans15 and Trans30), or supplemental feeding (Feed). (A) number of breeding groups projected over 50 years, (B) probabilities of metapopulation persistence over 100 years, and (C) inbreeding accumulated over 50 years.
Population management options that combine the Feed amplification of Strong territories with translocations were found to nearly stabilize the population size, minimize inbreeding, and with 30 FSJs translocated per year could keep the likelihood of extinction below 10% over 100 years (Figure 9, Supplementary Table S4). Combinations of population management that increase dispersal but do not increase Strong territories (Trans15 + Connect, Trans30 + Connect) result in greater population declines and do not keep the long-term probability of extinction below 10%. Combining all of Feed, Connect, and Translocations can result in population stability and less than 5% probability of extinction (Supplementary Table S4).
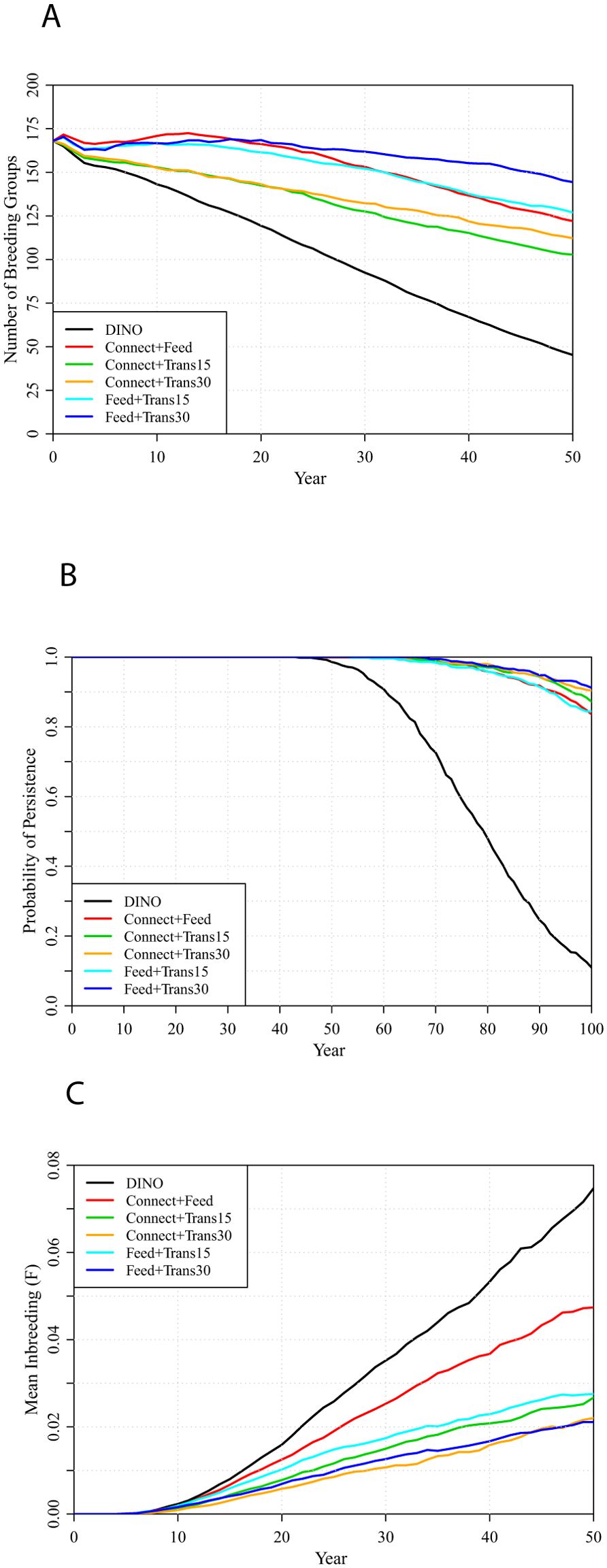
Figure 9. Metapopulation projections under several combinations of population management scenarios, each applied with DINO habitat management applied every 5 years: (A) number of breeding groups projected over 50 years, (B) probabilities of metapopulation persistence over 100 years, and (C) inbreeding accumulated over 50 years.
4 Discussion
Our updating of prior PVAs reaffirms that the populations of FSJs in the mainland portion of Brevard County, Florida are currently too small and isolated for long-term viability. Moreover, even management of the existing habitat, with the currently low level of connectivity among the set of 14 individually small populations, will not be adequate to prevent further decline and ensure long-term viability. Neither the earlier projections of habitat management options without further manipulation of the population structure (Eaton et al., 2021) nor population management options without further management of the transitional habitat states (Lacy and Breininger, 2021) showed paths forward that would ensure long-term persistence and growth of the metapopulation. A combination of habitat management and direct population management is needed to ensure viability.
The first delisting criterion of the U.S. Fish & Wildlife Service Recovery Plan, that of stable or increasing numbers, can be achieved, but only through a combination of continued aggressive and effective restoration and management of scrub habitat, much greater connectivity among subpopulations, and perhaps also increasing the density of breeding groups through supplemental feeding or other supportive actions. The second criterion, that of maintained genetic diversity, can be achieved at a level of 95% retention over 50 years, but only with increased connectivity that would likely require managed translocations. This would be counter to a requirement for genetic diversity being maintained naturally without translocations. The broadest criterion, that of sustaining habitat sufficient to ensure viability for the foreseeable future, at the level of keeping the likelihood of metapopulation extinction below 5% for 100 years, can be met only if all aspects of habitat and population management are effectively addressed: habitat management that increases optimal scrub habitat; increased connectivity; and further enhancing the sizes of populations that can be sustained in the existing habitat through supplemental feeding or other supportive actions.
Each of these forms of active population management has been shown to be at least plausible biologically and logistically. Translocation is in the early learning stages where family groups or helpers have been moved and translocated individuals often stay in the recipient sites and produce young (Mumme and Below, 1999). Currently all supplemental feeding is unauthorized, but it might increase as conservation sites get more human visitors. Experimental results by Schoech et al. (2008) suggested supplemental feeding might encourage initial FSJ population growth; supplemental feeding has increased populations of other jay species (Derbyshire et al., 2015). Generally, supplemental feeding needs additional research to confirm benefits outweigh risks (Benmazouz et al., 2021).
4.1 Improving PVA through links to habitat models
For many species, especially those in transitional habitats, habitat is not static, but PVA itself does not provide the methods for assessing changes to habitat (Akçakaya et al., 2004a, Akçakaya et al., 2005). Habitat characteristics can affect most population processes that are modeled in a PVA: reproduction and survival, carrying capacity, dispersal, connectivity, and social structure. However, PVAs rarely assess how habitat characteristics drive the vital rates, and rarely do they rely upon quantitative models of habitat change and the functional links of habitat to demographic rates, as conducted herein.
The observed demographic rates for FSJs in Brevard County confirm that the habitat states as classified by the ARM team do have direct impact on reproduction and survival of the FSJs, resulting in positive population growth in the most optimal habitat (OpO), decline in weaker (OpC), and the fastest population decline in habitat with vegetation that is either too short or too tall. The simulations with updated demographic rates confirmed the finding of earlier PVA (Lacy and Breininger, 2021) that isolated populations (or interconnected sets of populations with high rates of exchange) with fewer than about 80 potential territories in OpO habitat are vulnerable to extinction.
Carrying Capacity (K) is a key parameter of most population models, and yet the ecological carrying capacity of an area of habitat for a species is difficult to measure under all environmental conditions. Estimates of K are generally based on estimates of maximum observed prior population densities. However, it is uncommon for PVA models to implement population limitations that explicitly respond to the temporal and spatial variation in habitat quality. Carrying capacity arose in our models by virtue of the source-sink dynamics arising from habitat variability: Strong habitat patches allowed for positive population growth, while the poorer reproduction and survival in Sink habitat resulted in decline. Consequently, carrying capacity emerges from the distribution of habitat and responds to dynamic changes in the habitat. The linkage of habitat projections to population consequences via a mechanism driving carrying capacity in this system is an example of site-dependent population regulation (Kluyver and Tinbergen, 1954; Rodenhouse et al., 1997).
In our modeling, we also included the effect of habitat quality on social structure. We found that metapopulation viability is enhanced by the availability of Strong habitat influencing the likelihood that helper scrub-jays will disperse from natal groups and attempt to establish their own breeding group, and then establishing a territory in best available habitat. However, Sink habitats might play a stabilizing role via providing a buffer that absorbs excess birds when population densities get high, from which new breeders can be readily drawn when vacancies in better habitat arise (Pulliam et al., 1992). Conversely, Sink habitat might drain birds away from optimal habitat, if the tendency for dispersing jays to seek out the best habitat is not strong enough (Lacy and Breininger, 2021; Breininger et al., 2023). Within the ranges of parameter values that we tested, these competing forces apparently largely cancel: populations with a mix of Strong, Weak, and Sink habitat were projected to survive as well as did populations with only the Strong habitat. (E.g., about 80 Strong territories are required for population viability, regardless of whether there are also some Sink territories.) These components of the PVA model illustrate that the effects of dynamic habitat on populations can arise via effects on breeding patterns, dispersal, habitat choice, and likely other population processes that go beyond simple effects on fecundity and survival.
4.2 Effects of incorporating dynamic habitat projections into the PVA
The PVA model presented in Lacy and Breininger (2021) was a highly detailed, individual-based model that included components of population dynamics that are often ignored in simpler PVAs. Even with the inclusion of such complexity in the PVA, we now find that it makes a big difference to the PVA projections if we assume (as is done in many PVAs) that the current state of the habitat and the consequent demographic rates will persist (the “NoHab” scenarios that repeated the assumptions made in the prior PVA) vs projecting the habitat dynamics and the impacts on the population. The earlier PVA results were apparently too optimistic, in that they did not account for the expected transition of optimal oak scrub habitat to Tall and Tall-Mixed pine and oak woodland in the absence of very aggressive and ongoing management to regenerate scrub.
The pessimistic projections of population viability under prior levels of management helped to drive efforts to use much more aggressive management to restore optimal habitat. The DINO improvements to habitat suggest that managers can have greater control over habitat quality than previously considered using range-wide plant community management strategies relying mostly on fire alone as a management tool. Previous mechanical cutting methods relied on taking the tallest vegetation to the ground with hopes that fire thereafter could maintain the system, but this has not succeeded. Fires are difficult to start and then control, and cutting oaks to the ground results in dense sprouting vegetation that often burns poorly and results in few openings jays need. These early mechanical treatments were focused on the most degraded habitat, possibly explaining why they had worse results than fire alone and suggesting the need for continued innovation. Florida scrub-jay habitat quality is often considered an indicator of conditions for many unique plants and animals in scrub, and innovations for FSJs will need to be tested for other species of conservation concern that require frequent fire and also now reside in fragmented habitat (Kent and Kindell, 2010).
4.3 Management implications
Given the uncertainties in the habitat transition probabilities for different management regimes, this study should be seen as a presentation of a methodology and a demonstration of the potentially large impact on PVA results when projections of habitat change are included and integrated with the population model. Even so, the general trends revealed provide some guidance as to what management is necessary to sustain a viable metapopulation of FSJs in Brevard County.
The combined habitat and population modeling indicate that achieving the recovery goals of long-term stability, metapopulation persistence, and retaining adequate genetic diversity will require a combination of management of the scrub habitat, improving the capacity of even the optimal habitat to support breeding groups, and increasing connectivity among local populations. Attending to just one or two of these proposed actions, as might be suggested by a strictly habitat analysis or a strictly population analysis, will not be adequate. The recent history of success in restoring optimal scrub via mechanical removal of trees was represented by the DINO scenarios in our model, but the actual habitat transition probabilities will need to be monitored over time. Increased connectivity of local populations might be achieved by a combination of the means tested in our model: improving corridors that would allow for functional merging of some sets of local populations, actions that increase rate of inter-population dispersal through improved habitat management, or managed translocations. Given the importance of increasing connectivity, it will be important to monitor the effectiveness of whatever means are selected, either through tracking of movements of individual FSJs or through genetic monitoring to assess the consequences of increase connectivity or, ideally, both. The need also to manage for a higher density of breeding groups to be supported within available habitat might be achieved by supplemental feeding or other means to further improve the demographic performance of FSJs in each habitat state.
Our habitat-PVA model can provide the basis for testing more specific options for management, including determining: the number of optimal territories needed for a local population to provide benefit to the metapopulation, the necessary interval in years between habitat restoration actions, the schedule of translocations and best age classes and social composition to be moved, the number of exchanges of FSJs required for adjacent populations to function as fully connected units, the effect on the metapopulation of the loss of any of the populations, and the rate at which FSJs can be removed from healthy local populations to be used to reinforce or reestablish other populations or metapopulations.
4.4 Limitations and further needs
Adequately understanding the processes that threaten the viability of the FSJ metapopulation and the consequences of various proposed management actions required detailed data on population structure and demography, understanding of the relationships between demographic rates and habitat characteristics, knowledge of the behavioral responses to habitat quality and availability, habitat models for estimating projected changes in habitat quality, and comprehensive models for integrating habitat and population processes. For the FSJ such data have been generated over decades of extensive monitoring and research of both the Brevard County metapopulations and the metapopulation at Archbold Biological Station in south-central Florida (Woolfenden and Fitzpatrick, 1984; Fitzpatrick and Bowman, 2016). For many other species requiring habitat and population management, such data are not available and mechanistic models as detailed and complex as those we present would not be possible. Instead, PVA might be restricted to predictions of occupancy rates and mean growth rates, each based on empirical trends rather than on demographic modeling to generate those trends in mechanistic simulations (Nichols et al., 2024). Similarly, the modeling of habitat transitions might be limited to a dichotomous switching between suitable and unsuitable states or, conversely, might be based on a more mechanistic understanding of the factors driving habitat change that provides more refined projections than did our use of estimated transition probabilities.
The current and potential future values for many of the variables in our models are uncertain, so specific predictions might be overly optimistic or pessimistic. Extensive sensitivity analysis of key model parameters (e.g., natural dispersal rates, annual variability in demographic rates, effects of inbreeding) was provided in Lacy and Breininger (2021) and those analyses indicate that the general trends described above are sufficiently robust to parameter uncertainty. However, structural (process) uncertainty about the driving processes and the functional relationships is harder to assess because, by definition, it is the limitations in our knowledge about how the system works and what kinds of models can adequately represent it. Further exploration could omit or add component processes (habitat, demographic, social, or genetic) to the Vortex model that we used, in order to determine if our understanding of the system changes. In addition, alternative models of the habitat dynamics, such as can be done with the ST-Sim package for spatially explicit modeling of vegetation change (Daniel et al., 2016; docs.stsim.net), and for population dynamics, such as the HexSim spatially explicit, individual-based PVA (Schumaker and Brookes, 2018; hexsim.net), could be explored and predictions compared to those that we obtained with Vortex. Other available approaches include linking population models to Landis landscape models (Akçakaya et al., 2004b; Akçakaya et al., 2005).
We linked habitat and population models by including habitat transition probabilities that had been developed in the ARM project into macros that specified within the Vortex PVA the changing numbers of potential territories in each habitat state. Although we implemented the habitat transitions as probabilistic events, we did not include uncertainty in the transition probabilities in our modeling. Measures of uncertainty in the habitat transitions could be implemented within the macros by sampling the transition probabilities from distributions that describe their uncertainties. Another source of uncertainty that we were not able to include in our analyses is management uncertainty – the variability in application of and results from management actions that are recommended. We assumed that the management regimes we tested would be applied uniformly across the 14 local populations of the metapopulation, with the same consequences for the habitat. More complex models of habitat change might include additional habitat states or modifying characteristics, dependencies on spatial arrangement of habitat, or dependencies on the history of states rather than just the immediately prior habitat state. Such processes might need to be represented in a habitat model that is more complex than could be described in the simple macro language available within Vortex. The lack of certainty about both process (model structure) and parameter values, and the possibility that new methods of habitat and population management might be envisioned and tested, means that habitat and population responses to environmental change and management actions will need to be monitored, and data and models regularly updated, to allow for adaptive management of this threatened species.
Even if all the model parameters were correctly estimated, both the habitat transitions and the FSJ population dynamics include many stochastic processes, and consequently there was large variation among the iterations of any given scenario (see Figure 2 and Supplementary Tables S3, S4). Thus, although monitoring the fate of the habitat and populations will be an essential component of the adaptive management (Nichols et al., 2024), researchers and managers will need to recognize that the outcomes over just a few years of management might by chance be considerably better or worse than the expectation for the system. Well-informed management can increase the probability of success, but not guarantee it.
For some species, the wildlife population might impact its habitat in important ways, so that the habitat transitions are influenced by the population trajectory as well as the reverse. To capture these interactive dynamics between the population and its habitat might require that the habitat transition probabilities and the population changes be modeled simultaneously or interleaved on an annual or other timestep basis. This could be achieved by including in the Vortex scenario calls each simulation year to an external program written in R (R Core Team, 2020) that simulates habitat transitions based on the current state of both habitat and the population (and possibly other external drivers). Alternatively, a “metamodel” (Lacy et al., 2013) that includes both a model for estimating habitat transitions and a population model could step through both the habitat transition and population response one iteration and one year at a time.
Each level of complexity added to the linked analysis of habitat and population will increase potential for error in modeling the system or estimating parameters. Moreover, added complexity can make the causes of emergent trends more difficult to discern and to present to decision-makers, possibly without adding significant increase in accuracy of projections. Therefore, it will be important to determine how much realism is needed to capture the system dynamics adequately to guide successful management.
4.5 Value of integrated habitat and population modelling
Despite uncertainties, attempts at modeling such complexities bring together multidisciplinary collaborations (e.g., field and population ecologists, geneticists, land managers, and conservation land acquisition specialists) that learn to communicate more clearly with one another, reducing jargon and misunderstanding about geographical scale and time horizons. For this metapopulation of Florida scrub-jays, multi-disciplinary collaboration and integrated use of multiple modelling systems helped lead to recognition of the failure of past management practices to ensure long-term persistence, experimentation with new ways to manage both the scrub habitat and the FSJ populations, and identification of combinations of actions that are projected to result in population persistence and recovery.
Data availability statement
The datasets presented in this study can be found in online repositories. The names of the repository/repositories and accession number(s) can be found below: Zenodo repository: 10.5281/zenodo.13850866.
Author contributions
RL: Conceptualization, Formal analysis, Investigation, Methodology, Software, Visualization, Writing – original draft. DRB: Conceptualization, Data curation, Investigation, Project administration, Writing – review & editing. DJB: Data curation, Formal analysis, Writing – review & editing. AS: Investigation, Writing – review & editing. AF: Investigation, Writing – review & editing. EH: Investigation, Writing – review & editing. SM: Investigation, Resources, Writing – review & editing. DD: Investigation, Resources, Writing – review & editing. TM: Conceptualization, Funding acquisition, Project administration, Supervision, Writing – review & editing.
Funding
The author(s) declare financial support was received for the research, authorship, and/or publication of this article. Funding for the PVA was provided by a grant from The Nature Conservancy from funds allocated by U.S. Fish & Wildlife Service. Funding for field work was provided from the Florida Department of Transportation, Contract BDV24 #977-23.
Acknowledgments
We thank Sammy McGee, Mike Knight, Wendy Swindell, Ashley Lingwood, Beth Powell, Mike Legare, and Jim Lyon for their management of habitat and populations of the Florida scrub-jay and providing insights into our analyses. We thank Robbie Breininger, Michelle Smurl, Kerry Sweeny, Judy Elseroad, Geoff Carter, Stephanie Legare, and Jane Schnee for assistance with field studies. We thank Eric Stolen and Billy Payne for assistance in analyses. We thank Colin Daniel for his insights into vegetation modeling and links to population simulations. We thank Angela Klug for helping to secure and manage funds for the PVA work. Support from the sponsors of the Species Conservation Toolkit Initiative (https://scti.tools) enables the support and free distribution of the Vortex software.
Conflict of interest
The authors declare that the research was conducted in the absence of any commercial or financial relationships that could be construed as a potential conflict of interest.
The author(s) declared that they were an editorial board member of Frontiers, at the time of submission. This had no impact on the peer review process and the final decision.
Generative AI statement
The authors declare that no Generative AI was used in the creation of this manuscript.
Publisher’s note
All claims expressed in this article are solely those of the authors and do not necessarily represent those of their affiliated organizations, or those of the publisher, the editors and the reviewers. Any product that may be evaluated in this article, or claim that may be made by its manufacturer, is not guaranteed or endorsed by the publisher.
Supplementary material
The Supplementary Material for this article can be found online at: https://www.frontiersin.org/articles/10.3389/fcosc.2024.1505016/full#supplementary-material
References
Akçakaya H. R. (2002). Estimating the variance of survival rates and fecundities. Anim. Conserv. 5, 333–336. doi: 10.1017/S1367943002004092
Akçakaya H. R., Burgman M. A., Kindvall O., Wood C. C., Sjogren-Gulve P., Hatfield J. S., et al. (2004a). Species conservation and management: case studies (New York: Oxford University Press). doi: 10.1093/oso/9780195166460.001.0001
Akçakaya H. R., Franklin J., Syphard A. D., Stephenson J. R. (2005). Viability of Bell’s sage sparrow (Amphispiza belli ssp. belli): altered fire regimes. Ecol. Appl. 15, 521–531. doi: 10.1890/03-5378
Akçakaya H. R., Radeloff V. C., Mlandenoff D. J., He H. S. (2004b). Integrating landscape and metapopulation modeling approaches: Viability of the sharp-tailed grouse in a dynamic landscape. Conserv. Biol. 18, 526–537.
Benmazouz I., Jokimäki J., Lengyel S., Juhász L., Kaisanlahti-Jokimäki M.-L., Kardos G., et al. (2021). Corvids in urban environments: A systematic global literature review. Animals 11, 3226. doi: 10.3390/ani11113226
Breininger D. R., Burgman M. A., Stith B. M. (1999). Influence of habitat quality, catastrophes, and population size on extinction risk of the Florida scrub-jay. Wildlife Soc. Bull. 27, 810–822.
Breininger D. R., Carter G. M. (2003). Territory quality transitions and source-sink dynamics in a Florida Scrub-Jay population. Ecol. Appl. 13, 516–529. doi: 10.1890/1051-0761(2003)013[0516:TQTASS]2.0.CO;2
Breininger D. R., Carter G. M., Legare S. A., Payne W. V., Stolen E. D., Breininger D. J., et al. (2022). Multistate modeling of Florida scrub-jay adult survival and breeding transitions. Ecosphere 13, e3991. doi: 10.1002/ecs2.v13.5
Breininger D. R., Foster T. E., Carter G. M., Duncan B. W., Stolen E. D., Lyon J. E. (2018). The effects of vegetative type, edges, fire history, rainfall, and management in fire-maintained habitat. Ecosphere 9, e02120. doi: 10.1002/ecs2.2018.9.issue-3
Breininger D. R., Nichols J. D., Carter G. M., Oddy D. M. (2009). Habitat-specific breeder survival of Florida Scrub-Jays: inferences from multistate models. Ecology 90, 3180–3189. doi: 10.1890/08-1123.1
Breininger D. R., Nichols J. D., Duncan B. W., Stolen E. D., Carter G. M., Hunt D. K., et al. (2010). Multistate modeling of habitat dynamics: factors affecting Florida scrub transition probabilities. Ecology 91, 3354–3364. doi: 10.1890/09-0964.1
Breininger D. R., Oddy D. M. (2004). Do habitat potential, population density, and fires influence scrub-jay source-sink dynamics? Ecol. Appl. 14, 1079–1089. doi: 10.1890/03-5002
Breininger D. R., Stolen E. D., Carter G. M., Legare S. A., Payne W. V., Breininger D. J., et al. (2023). Territory and population attributes affect Florida scrub-jay fecundity in fire-adapted ecosystems. Ecol. Evol. 13, e9704. doi: 10.1002/ece3.v13.1
Breininger D. R., Stolen E. D., Carter G. M., Oddy D. M., Legare S. A. (2014). Quantifying how territory quality and sociobiology affect recruitment to inform fire management. Anim. Conserv. 17, 72–79. doi: 10.1111/acv.2014.17.issue-1
Breininger D. R., Toland B., Oddy D. M., Legare M. L. (2006). Landcover characterizations and Florida scrub-jay (Aphelocoma coerulescens) population dynamics. Biol. Conserv. 128, 169–181. doi: 10.1016/j.biocon.2005.09.026
Carroll C., Lacy R. C., Frederickson R. J., Rohlf D. J., Hendricks S. A., Phillips M. K. (2019). Biological and sociopolitical sources of uncertainty in population viability analysis for endangered species recovery planning. Sci. Rep. 9, 10130. doi: 10.1038/s41598-019-45032-2
Carter G. M., Stolen E. D., Breininger D. R., Legare S. A., Hunt D. K., Schumann C. D., et al. (2023). Survival of juvenile Florida Scrub-Jays is positively correlated with month and negatively correlated with male breeder death. Ornithological Appl. 125, 1-13. doi: 10.1093/ornithapp/duad033
Chen N., Cosgrove E. J., Bowman R., Fitzpatrick J. W., Clark A. G. (2016). Genomic consequences of population decline in the endangered Florida Scrub-Jay. Curr. Biol. 26, 2974–2979. doi: 10.1016/j.cub.2016.08.062
Daniel C., Frid L., Sleeter B., Fortin M. J. (2016). State-and-transition simulation models: a framework for forecasting landscape change. Methods Ecol. Evol. 7, 1413-1423. doi: 10.1111/2041-210X.12597
Derbyshire R., Strickland D., Norris D. R. (2015). Experimental evidence and 43 years of monitoring data show that food limits reproduction in a food-caching passerine. Ecology 96, 3005–3015. doi: 10.1890/15-0191.1
Duncan B. W., Schmalzer P. A., Breininger D. R., Stolen E. D. (2015). Comparing fuels reduction and patch mosaic fire regimes for reducing fire spread potential: A spatial modeling approach. Ecol. Model. 314, 90–99. doi: 10.1016/j.ecolmodel.2015.07.013
Duncan B. W., Shao G., Adrian F. W. (2009). Delineating a managed fire regime and exploring its relationship to the natural fire regime in East Central Florida, USA: A remote sensing and GIS approach. For. Ecol. Manage. 258, 132–145. doi: 10.1016/j.foreco.2009.03.053
Eaton M. J., Breininger D. R., Nichols J. D., Fackler P. L., McGee S., Smurl M., et al. (2021). Integrated hierarchical models to inform management of transitional habitat and the recovery of a habitat specialist. Ecosphere 12, e03306. doi: 10.1002/ecs2.3306
Fitzpatrick J. W., Bowman R. (2016). Florida scrub-jays: oversized territories and group defense in a fire-maintained habitat. Cooperative breeding in vertebrates: studies of ecology, evolution, and behavior (Cambridge: Cambridge University Press), 77–96.
Hall L. S., Krausman P. R., Morrison M. L. (1997). The habitat concept and a plea for standard terminology. Wildlife Soc. Bull. 25, 173–182.
Johnson F. A., Breininger D. R., Duncan B. W., Nichols J. D., Runge M. C., Williams B. K. (2011). A Markov decision process for managing habitat for Florida Scrub-Jays. J. Fish Wildlife Manage. 2, 234–246. doi: 10.3996/012011-JFWM-003
Kent A., Kindell C. (2010). Scrub management guidelines for peninsular Florida: using the scrub-jay as an umbrella species. (Tallahassee, FL: Florida Fish and Wildlife Conservation Commission and Florida Natural Areas Inventory).
Kluyver H., Tinbergen L. (1954). Territory and the regulation of density in titmice. Arch. neerlandaises zoologie 10, 265–289. doi: 10.1163/036551653X00024
Lacy R. C. (2000a). Structure of the VORTEX simulation model for population viability analysis. Ecol. Bulletins 48, 191–203.
Lacy R. C. (2000b). Considering threats to the viability of small populations. Ecol. Bulletins 48, 39–51.
Lacy R. C., Breininger D. R. (2021). Population Viability Analysis (PVA) as a platform for predicting outcomes of management options for the Florida Scrub-Jay in Brevard County. NASA Technical Report. Document 20210022519, (Washington, DC: NASA).
Lacy R. C., Miller P. S., Nyhus P. J., Pollak J. P., Raboy B. E., Zeigler S. (2013). Metamodels for transdisciplinary analysis of population dynamics. PloS One 8, e84211. doi: 10.1371/journal.pone.0084211
Lacy R. C., Miller P. S., Traylor-Holzer K. (2023). Vortex 10 user’s manual. 4 may 2023 update (Apple Valley, MN: IUCN SSC Conservation Breeding Specialist Group, and Chicago Zoological Society).
Lacy R. C., Pollak J. P. (2024). VORTEX: A stochastic simulation of the extinction process. Version 10.7.0 (Brookfield, IL: Chicago Zoological Society). Available at: www.scti.tools/vortex.
Mumme R. L., Below T. H. (1999). Evaluation of translocation for the threatened Florida scrub-jay. J. Wildlife Manage. 63, 833–842. doi: 10.2307/3802796
Nichols J. D., Breininger D. R., Armstrong D. P., Lacy R. C. (2024). Habitat management decisions for conservation: A conceptual framework. Front. Conserv. Sci. 5. doi: 10.3389/fcosc.2024.1450000
Pulliam H. R., Dunning J. B. Jr., Liu J. (1992). Population dynamics in complex landscapes: a case study. Ecol. Appl. 2, 165–177. doi: 10.2307/1941773
R Core Team (2020). R: A language and environment for statistical computing. (Vienna, Austria: R foundation for statistical computing). Available at: https://www.R-project.org/.
Rodenhouse N. L., Sherry T. W., Holmes R. T. (1997). Site-dependent regulation of population size: a new synthesis. Ecology 78, 2025–2042. doi: 10.2307/2265942
Root K. V. (1998). Evaluating the effects of habitat quality, connectivity, and catastrophes on a threatened species. Ecol. Appl. 8, 854–865. doi: 10.1890/1051-0761(1998)008[0854:ETEOHQ]2.0.CO;2
Schmalzer P. A., Boyle S. (1998). Restoring long-unburned oak-mesic flatwoods requires mechanical cutting and prescribed burning. Restor. Manage. Notes 16, 96–97.
Schoech S. J., Bridge E. S., Boughton R. K., Reynolds S. J., Atwell J. W., Bowman R. (2008). Food supplementation: a tool to increase reproductive output? A case study in the threatened Florida Scrub-Jay. Biol. Conserv. 141, 162–173. doi: 10.1016/j.biocon.2007.09.009
Schumaker N. H., Brookes A. (2018). HexSim: a modeling environment for ecology and conservation. Landscape Ecol. 33, 197–211. doi: 10.1007/s10980-017-0605-9
Stith B. M. (1999). “Metapopulation dynamics and landscape ecology of the Florida Scrub-Jay,” in Aphelocoma coerulescens (Gainesville, FL: University of Florida).
U.S. Fish and Wildlife Service (2019a). Recovery plan for the florida scrub-jay (Aphelocoma coerulescens) (Atlanta GA: U.S. Fish and Wildlife Service).
U.S. Fish and Wildlife Service (2019b). “Species status assessment for the florida scrub-jay (Aphelocoma coerulescens),” in Version 1.0. U.S. Fish and wildlife service north florida ecological services(Jacksonville, FL).
Van Horne B. (1983). Density as a misleading indicator of habitat quality. J. Wildlife Manage. 47, 893–901. doi: 10.2307/3808148
Woolfenden G. E., Fitzpatrick J. W. (1984). The Florida scrub jay: demography of a cooperative-breeding bird (Princeton, New Jersey: Princeton University Press), 406.
Keywords: habitat modeling, habitat management, population viability analysis, Florida scrub-jay, population management, adaptive resource management, species recovery
Citation: Lacy RC, Breininger DR, Breininger DJ, Savage AE, Forsman AM, Hoffman EA, McGuffey SD, DeMeyer D and Mecklenborg T (2024) Linking habitat and population viability analysis models to account for vegetation dynamics, habitat fragmentation, and social behavior of a metapopulation of Florida scrub-jays. Front. Conserv. Sci. 5:1505016. doi: 10.3389/fcosc.2024.1505016
Received: 01 October 2024; Accepted: 12 November 2024;
Published: 03 December 2024.
Edited by:
João Pedro Barreiros, University of the Azores, PortugalReviewed by:
Barry Noon, Colorado State University, United StatesNathan Schumaker, US Environmental Protection Agency, United States
Copyright © 2024 Lacy, Breininger, Breininger, Savage, Forsman, Hoffman, McGuffey, DeMeyer and Mecklenborg. This is an open-access article distributed under the terms of the Creative Commons Attribution License (CC BY). The use, distribution or reproduction in other forums is permitted, provided the original author(s) and the copyright owner(s) are credited and that the original publication in this journal is cited, in accordance with accepted academic practice. No use, distribution or reproduction is permitted which does not comply with these terms.
*Correspondence: Robert C. Lacy, cmxhY3lAaXgubmV0Y29tLmNvbQ==