- 1Center for the Advancement of Spatial Informatics Research and Education, University of New Mexico, Albuquerque, NM, United States
- 2Department of Geography and Environmental Studies, University of New Mexico, Albuquerque, NM, United States
- 3Division of Migratory Bird Management, United States Fish and Wildlife Service, Orono, ME, United States
- 4Environmental Studies Program, Bureau of Ocean Energy Management, Sterling, VA, United States
- 5Department of Wildlife Ecology and Conservation, University of Florida, Gainesville, FL, United States
- 6Audubon Texas, National Audubon Society, Austin, TX, United States
- 7Division of Biological Sciences, United States Fish and Wildlife Service, Albuquerque, NM, United States
- 8Conservation Metrics, Inc., Santa Cruz, CA, United States
Technological and methodological advances in remote sensing and machine learning have created new opportunities for advancing wildlife surveys. We assembled a Community of Practice (CoP) to capitalize on these developments to explore improvements to the efficiency and effectiveness of aerial wildlife monitoring from a management perspective. The core objective of the CoP is to organize the development and testing of remote sensing and machine learning methods to improve aerial wildlife population surveys that support management decisions. Beginning in 2020, the CoP collaboratively identified the natural resource management decisions that are informed by wildlife survey data with a focus on waterbirds and marine wildlife. We surveyed our membership to establish 1) what management decisions they were using wildlife count data to inform; 2) how these count data were collected prior to the advent of remote sensing/machine learning methods; 3) the impetus for transitioning to a remote sensing/machine learning methodological framework; and 4) the challenges practitioners face in transitioning to this framework. This paper documents these findings and identifies research priorities for moving toward operational remote sensing-based wildlife surveys in service of wildlife management.
1 Introduction
Biologists and natural resource managers use aerial surveys to estimate abundance and trends of many wildlife species, providing essential data for informing management decisions (e.g. Norton-Griffiths, 1975; Caughley, 1977; Lyons et al., 2008). Historically, low-altitude aerial surveys conducted by human observers have been a resource-efficient method to collect data over extensive areas, ranging from hundreds to thousands of hectares (e.g. Fiori et al., 2017; Conroy et al., 2018; White and Veit, 2020). These surveys have been instrumental in studying taxa such as waterbirds (e.g. Smith, 1995), marine mammals (e.g. Fiori et al., 2017), and large ungulates (e.g. Caughley, 1977; Conroy et al., 2018). However, aerial surveys can suffer from significant limitations including observation biases and count variability (e.g. Boyd, 2000; Frederick et al., 2003; Conroy et al., 2018), difficulties with error enumeration (Davis et al., 2022), and safety risk to participants (Sasse, 2003).
Technological advancements in remote sensing approaches may alleviate some of these limitations. Automated object detection techniques applied to aerial imagery, such as deep learning using convolutional neural networks (CNNs), show promise for enhancing the frequency, efficiency, accuracy, and safety of aerial wildlife counts. Because aerial imaging missions typically produce data volumes that are time- and cost-prohibitive to manually process, automated methods are rapidly being incorporated into a variety of ecological and natural resource management workflows (Tuia et al., 2022).
To encourage collaboration on the development of remote sensing and machine learning approaches in wildlife monitoring and reduce redundant efforts, practitioners at federal and state natural resource management agencies and applications-based researchers at academic institutions in the United States working on these issues in parallel began to meet virtually in 2020 during the COVID-19 pandemic. Over time, the group formed the Remote Sensing/Machine Learning Community of Practice for Wildlife Survey (CoP). This paper documents the findings of the CoP regarding the management applications requiring wildlife count data, the methods traditionally used to collect these data, the challenges with traditional methods leading to the impetus to transition to a remote sensing/machine learning (RS/ML) survey framework, and identifies research priorities to ease the transition into implementing an affordable, operational, and statistically valid RS/ML workflow.
2 Background
Initially, the CoP was convened by the US Fish and Wildlife Service’s Division of Migratory Bird Management, the Bureau of Ocean Energy Management’s Environmental Studies Program, the US Geological Surveys’ Upper Midwest Ecological Science Center, and the Missouri Department of Conservation. In 2020, invitations were broadly distributed through agency and academic email lists to solicit participation by practitioners and researchers across the United States.
The CoP fosters information exchange and collaboration on shared challenges; guides technology development in alignment with agency requirements, resources, and obstacles; identifies research priorities; and advances community-wide progress through initiatives like data standardization and accessibility, recommendations on tools and workflows, and development of publicly available annotated image datasets for a variety of species. In a series of semi-structured workshops from 2021–2023, the CoP solicited insights from members regarding: 1) the management decisions practitioners were informing with wildlife count data; 2) the traditional methodologies employed for data collection; 3) motivations of members for transitioning to an RS/ML approach; and 4) perceived obstacles to implementing such an approach. Leadership synthesized these responses, identified common themes, and presented results to the broader group for further refinement and clarification. These results are presented in this paper.
2.1 Management applications
Wildlife monitoring surveys provide spatially explicit count data for informed decision-making based on population assessments, habitat characterization, and identification of long-term trends, providing valuable data for various regulatory and non-regulatory applications in the United States. The management decisions requiring wildlife count data identified by the CoP are summarized in Table 1 and can be divided into two broad types: 1) decisions focused on removal of wildlife (harvest), and 2) decisions focused on population change, for aiding species recovery and assessing the effects of resource manipulation (e.g., relationships between abundance, habitat management, anthropogenic modifications and climate change).
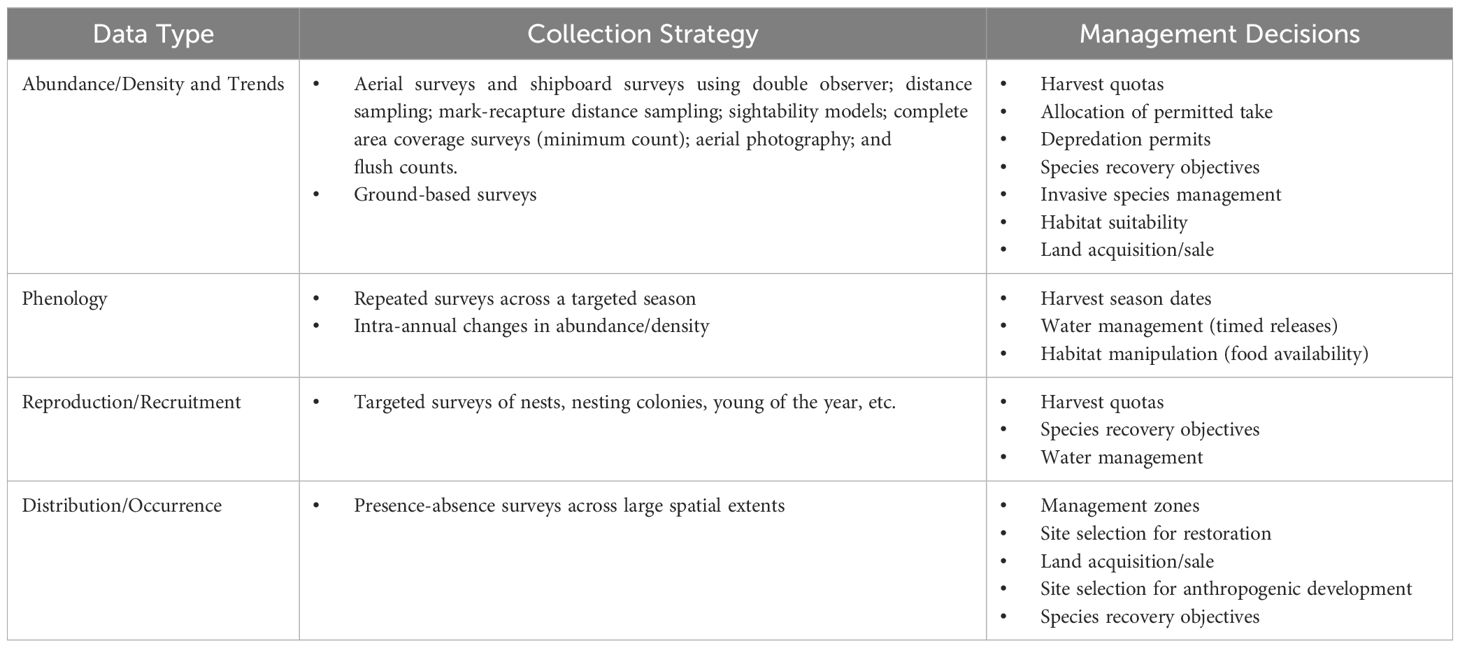
Table 1 Management decisions informed through data obtained by monitoring wildlife populations across local- to continental-scales.
Management decisions involving the harvest of wildlife typically focus on ensuring the long-term sustainability of the affected wildlife populations. For harvest, decision-making occurs in the context of hunting quotas, establishment of hunting seasons and locations that hunting occurs (Table 1) (Proffitt et al., 2020). Other related management goals include commercial or subsistence harvest, incidental take, and permitted take for scientific collecting or other activities. In some cases, management activities target population reduction or redistribution (e.g., permitted take related to crop depredation or invasive species control).
The second class of decisions centers on species recovery and manipulating wildlife habitat or resources. Population count data and derived estimates are useful for understanding wildlife-habitat relationships to recover species, and prioritize, plan, or evaluate the effectiveness of habitat conservation or direct management actions (e.g., potential risk of long-term disturbance to communities of marine wildlife due to offshore energy installations; growing crops to support migratory waterfowl populations). Furthermore, these data inform strategic planning of activities to mitigate the potential impacts on wildlife and to evaluate post-construction effects. Spatial patterns and temporal trends of wildlife populations derived from survey data provide critical layers of information during project development, permitting processes, and support collaborative mitigation strategies between government, non-governmental organizations, and developers.
2.2 Traditional data collection methods
While the CoP identified several methods for collecting wildlife count data (Table 1), aerial survey was the most common technique in which remote sensing and machine learning might be applied. Aerial observer surveys have monitored the abundance, density and distribution of wildlife since the 1930s, when the first exploratory aerial surveys were conducted of wintering waterfowl (USFWS, 2004). Aerial observer surveys are typically favored over surveys conducted from the ground or water surface when large areas are covered over short timeframes, or in remote or rugged terrain where ground access is difficult or dangerous (Samuel et al., 1987; McRoberts et al., 2011; Conroy et al., 2018). The characteristics of the wildlife species and populations sampled, background terrain, land cover, distance to survey location, and operational costs dictate the applicability and choice of aircraft and flight altitude. Species difficult to observe may warrant crewed low-level flights (Sasse, 2003). For example, long-standing survey protocols for large ungulates (Conroy et al., 2014, 2018), Galliformes (Butler et al., 2007; McRoberts et al., 2011), cranes (Butler et al., 2019), waterfowl (Prenzlow and Lovvorn, 1996) and seabirds (Camphuysen et al., 2004) require flight altitudes of approximately 30–80 m above ground level.
3 Transition to a remote sensing/machine learning workflow
Practitioners in the CoP seek to understand the advantages and disadvantages of transitioning from traditional aerial observer surveys of wildlife to a remote sensing-based (aerial imaging) workflow, paired with automated image processing using machine learning methods. This conceptual approach is visualized in Figure 1 in the form of a remote sensing communication model (Lippitt et al., 2014) and will be referred to as the RS/ML workflow from here.
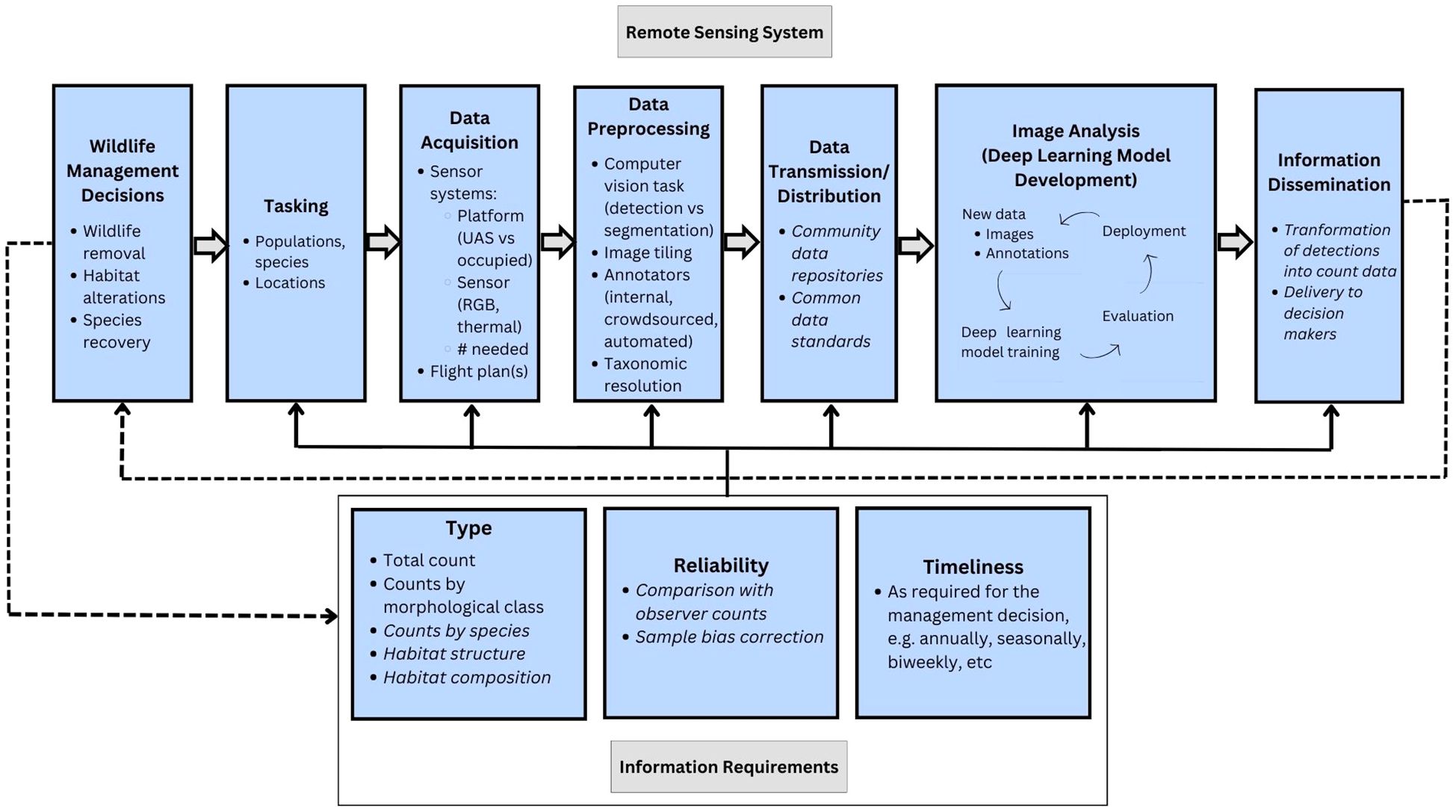
Figure 1 Remote sensing/machine learning workflow for aerial wildlife monitoring conceptual diagram, in the form of a remote sensing communication model. Italics indicate areas that have been identified as research priorities by the CoP, requiring further development. Regular font reflects the current workflow as practiced by a plurality of CoP membership.
The RS/ML workflow is flexible for incorporating data from a variety of airborne sensor systems; while the typical paradigm incorporates very high spatial resolution (<1 inch/2.5 cm per pixel) and natural-color imagery (i.e., RGB), some CoP members use thermal imagery in certain contexts (e.g., Luz-Ricca et al., 2023). Traditional pixel-based automated image processing methods (e.g., spectral thresholding) are typically effective only in scenarios with high visual contrast between the animal of interest and its background, such as snow geese on dark water (Laliberte and Ripple, 2003). Otherwise, the heterogeneity of pixel values within singular objects (individual animals, in this instance) tends to lead to unreliable classification results. Machine learning methods in computer vision, particularly deep learning using CNNs, can more flexibly incorporate complex image information by transitioning the unit of analysis from the pixel to the objects formed by groups of pixels, and sampling pixel neighborhoods that provide contextual patterns often used for visual interpretation by humans (e.g., size, shape, pattern). Machine learning models must be “trained” using hundreds to thousands of human-annotated examples of each class or object of interest.
3.1 Impetus for transitioning to an RS/ML workflow
The CoP membership identified the following general themes in their interest in transitioning to an RS/ML workflow:
3.1.1 Efficiency
Participants intend that after an initial investment for development and validation of machine learning models for image processing, efficiency gains in field operations and data workflows will manifest. Imaging with UAS and higher-altitude occupied aircraft should reduce flight times, human labor in the field, and carbon emissions while increasing safety to participants. After development and validation of a machine learning model for image processing, time to review errors should drop to a fraction of the time investment required to manually count and identify animals in imagery (Chabot et al., 2018).
3.1.2 Data quality
Aerial imaging should lead to better data quality via lower count variance compared to observer surveys (Hodgson et al., 2018), as well as new opportunities to assess bias/error compared with traditional methods (Augustine et al., 2023). Additionally, it was anticipated that the preservation of the survey frame via the image data would enable future revisions to counts/identifications if error is suspected and/or as methods continue to improve.
3.1.3 New opportunities
Preserving the survey frame and environmental context captured in aerial images informs new types of research involving the relative spatial arrangements of animals (e.g., Henriksen et al., 2015). Novel research can also explore the environmental conditions observed during an image collection, such as vegetation structure and composition, wetland conditions, and other habitat features. Re-analysis for non-target animal species could also be possible.
3.2 Barriers and bridges to implementation
The CoP membership identified the following general obstacles that they or their organization had experienced or were experiencing while attempting to implement an RS/ML workflow for wildlife monitoring:
3.2.1 Financial
The infrastructure required to support an RS/ML workflow necessitates large upfront costs in the acquisition of hardware (e.g., sensor systems, data storage, computing power) and specialized personnel for data collection, management, and development of machine learning models, which require technical skill sets generally not possessed by natural resource managers. Sustained partnerships between technically trained individuals and natural resource managers are needed to advance systematic data collection, processing, and reporting efforts when transitioning to an RS/ML workflow. In addition, greater investment on a community level in open-source model development and data sharing to reduce redundant efforts can improve model performance and reduce financial burdens on individual teams.
3.2.2 Technical
State-of-the-art machine learning methods incorporate substantial technical requirements that can be exacerbated by the specialized needs of wildlife monitoring programs. For instance, a common barrier identified by CoP membership was the acquisition of a sufficient quantity of annotated imagery for training machine learning models. Little pre-existing, publicly available data of this type exists for aerial wildlife monitoring; thus, new data must be collected by practitioners for each species and area of interest. Imagery collection must be based on the goals and measurable objectives of the survey and timed carefully (e.g., phenology of migratory species). Image annotation can be particularly time-consuming for aerial images, which can potentially contain dozens to hundreds of individuals in a given image. Securing a sufficient variety of examples of a given species to annotate may also require sorting through dozens to hundreds of “empty” images with no animals. Additionally, practitioners expressed that it was often difficult to collect imagery of quality sufficient for trained observers to differentiate similar-looking species, or to achieve the practitioners’ desired taxonomic resolution.
Some difficulties with the annotation process can be mitigated by interventions such as crowdsourcing and reducing observer effort by tiling images to reduce search area and the number of animals per image tile (Converse et al.)1. Data sharing and community alignment of priorities to avoid duplication would help reduce the necessity for individual groups to collect vast volumes of imagery and annotations.
Some CoP members expressed uncertainty about how to validate the RS/ML approach, including articulating errors stemming from the aerial imaging process (e.g., double counting of individual animals in overlapped aerial images) as well as the machine learning classification of imagery; how these errors compare with those from traditional observer surveys; and how to conduct quality assurance and quality control on data products produced through an RS/ML workflow. Furthermore, the typical lack of “true” counts against which to compare the results of either manual or machine interpretation of imagery complicates the validation process, though this difficulty is shared with traditional aerial surveys.
The poor generalizability of machine learning models was another common obstacle to the implementation of an RS/ML workflow. Machine learning models tend to perform poorly when exposed to data differing from the training data in some aspect– for instance, a new habitat, sensor, time of day, species assemblage, etc (Beery et al., 2018). Integration of more data into machine learning models by fusing similar datasets from a variety of sources may help mitigate this problem, while having the benefit of lowering data collection and annotation requirements for individual practitioners (Weinstein et al., 2022).
CoP membership indicated difficulties with data curation and archive management required by the volumes of imagery data produced via an RS/ML approach. This problem is partly due to resource limitations (see above), as well as a lack of referenceable standards.
4 Discussion
Practitioners identified limitations of traditional wildlife population survey methods, particularly aerial observer surveys, leading to interest in transitioning to an RS/ML workflow. We acknowledge that the RS/ML workflow does not solve all of the limitations of aerial observer surveys, including susceptibility to availability bias (if an animal cannot be seen/photographed, it cannot be counted), and the typical lack of independently verified counts against which survey data may be validated. Despite this, the RS/ML workflow shows promise in advancing participant safety, reducing costs, improving data quality, and offering new research avenues. Methodological and logistical challenges, as outlined above, currently hinder widespread adoption of this framework. To address current challenges, the CoP has identified an agenda including new research avenues and establishing a set of best practices supporting the workflow.
4.1 Research agenda
In the collaborative workshop process, the CoP identified the following research priorities:
4.1.1 Characterizing bias from aerial imaging
To provide a fair point of comparison with existing observer-based methods, research will be required to characterize the types of bias stemming from an aerial imaging approach as well as strategies for correcting these biases. Aerial imaging is conducted at higher altitudes and sometimes with different sensor platforms, producing varying levels of noise or flying in different patterns from observer-based surveys, which may impact detection and availability biases of wildlife species. An emerging research area examines the ability of human observers to detect and identify different species of animals from aerial imagery, whether there are characteristics of the image/sensor system, habitat, or species of interest that impact this ability, and what data collection parameters can be established to maximize observers’ ability to correctly count and identify animals (e.g., Converse et al)1. Developing methods for coping with errors and artifacts from an aerial imaging approach, such as double counts of animals present in multiple frames of imagery, will also be necessary.
4.1.2 Improving the machine learning pipeline
Research and development that improves the machine learning pipeline at all points– from image annotation to model selection and accuracy assessment– will be critical to rendering an RS/ML workflow more feasible for practitioners to implement. Better understanding of the factors that impact the efficiency of human effort in the annotation process may produce important insights into structuring field data collections and allow for producing better models from fewer training samples. Improving the accuracy of machine learning models to reduce reliance on human review in terms of annotating inputs and in correcting errors will also be critical. This will necessitate foundational computer vision research into avenues such as active learning techniques (e.g., Norouzzadeh et al., 2021), data augmentations (e.g., Chen et al., 2022), new model architectures, and more consistent image processing procedures (e.g., Kellenberger et al., 2021). Increasing confidence in machine learning outputs through improved statistical rigor will be necessary, as current methods do not adequately characterize multiple sources of error and bias including the downstream propagation of uncertainty in image labels. In tandem, this will also require developing a better understanding of the count precision required for different wildlife management applications.
4.1.3 From detections to counts
Developing approaches for transforming the outputs of machine learning detections of wildlife into population data will be critical. An important starting point will be developing strategies for survey design to ensure the correct count data is collected, including parameters for imagery acquisition. Integrating RS/ML outputs into existing population management frameworks will require characterizing the similarities and differences between population inference from RS/ML approaches and traditional surveys.
4.2 Best practices
In addition to setting a research agenda, CoP membership agreed on the need for establishing a set of best practices to guide practitioners. These standards will include information needs aimed at technical improvements, including annotation tools, data and metadata formats, and structures/locations for data storage. Establishing best practices for standard data formatting will aid projects in releasing their data publicly and thereby enhance progress towards improving machine learning models for wildlife detection more generally. Membership also agreed on the need for referenceable standards for data collection and validation, including specifications for sensor systems to capture the desired taxonomic resolution, structuring field data collections for training and validation data, and developing a set of quality assurance and control practices.
5 Conclusion
Technological and methodological advancements offer new opportunities in aerial wildlife monitoring. Aerial observer surveys have traditionally been deployed to collect wildlife survey data to inform decisions on wildlife removal and alterations to habitat. While some limitations are shared with traditional methods, the RS/ML workflow shows promise to improve participant safety, provide more rigorous data, and allow for new research opportunities given the preservation of additional spatial distribution data and environmental context. Significant logistical and technical obstacles remain, hindering the widespread implementation of an RS/ML workflow for wildlife monitoring. In setting a research agenda and establishing a set of best practices, we hope to move toward a vision of coordinating efforts among practitioners in North America in developing a continental-scale machine learning model for acquiring and processing aerial wildlife survey imagery, while establishing an emulative workflow for other practitioners worldwide.
Data availability statement
The original contributions presented in the study are included in the article/supplementary material. Further inquiries can be directed to the corresponding author.
Author contributions
RC: Conceptualization, Investigation, Visualization, Writing – original draft, Writing – review & editing. CL: Conceptualization, Investigation, Supervision, Visualization, Writing – original draft, Writing – review & editing. MK: Conceptualization, Investigation, Writing – original draft, Writing – review & editing. TW: Conceptualization, Investigation, Visualization, Writing – original draft, Writing – review & editing. BW: Investigation, Writing – original draft, Writing – review & editing. RG: Investigation, Writing – original draft. DS: Investigation, Visualization, Writing – original draft, Writing – review & editing. AF: Investigation, Writing – review & editing. MB: Investigation, Visualization, Writing – original draft, Writing – review & editing. SS: Investigation, Visualization, Writing – original draft, Writing – review & editing. GH: Investigation, Writing – original draft, Writing – review & editing.
Funding
The author(s) declare that no financial support was received for the research, authorship, and/or publication of this article.
Acknowledgments
With deep thanks to the broader membership of the Remote Sensing/Machine Learning Community of Practice for Wildlife Survey group for providing their insights over the course of the collaborative workshop sessions.
Conflict of interest
Author AF was employed by the company Conservation Metrics, Inc.
The remaining authors declare that the research was conducted in the absence of any commercial or financial relationships that could be construed as a potential conflict of interest.
Publisher’s note
All claims expressed in this article are solely those of the authors and do not necessarily represent those of their affiliated organizations, or those of the publisher, the editors and the reviewers. Any product that may be evaluated in this article, or claim that may be made by its manufacturer, is not guaranteed or endorsed by the publisher.
Footnotes
- ^ 1Converse, R. L., Lippitt, C. D., Sesnie, S. E., Harris, G. M., Butler, M. J., and Stewart, D. R. Observer variability in manual-visual interpretation of UAS imagery of wildlife, with insights for deep learning applications. (In review)
References
Augustine B. C., Koneff M. D., Pickens B. A., Royle J. A. (2023). Towards estimating marine wildlife abundance using aerial surveys and deep learning with hierarchical classifications subject to error. bioRxiv. doi: 10.1101/2023.02.20.529272
Beery S., Van Horn G., Perona P. (2018). “Recognition in terra incognita,” in Computer Vision – ECCV, vol. 11220 . Eds. Ferrari V., Hebert M., Sminchisescu C., Weiss Y. (Springer International Publishing: Cham, Switzerland), 472–489.
Boyd W. S. (2000). A comparison of photo counts versus visual estimates for determining the size of snow goose flocks. J. Field Ornithol 71, 686–690. doi: 10.1648/0273-8570-71.4.686
Butler M. J., Ballard W. B., Wallace M. C., Demaso S. J., Mcgee B. K. (2007). Aerial surveys for estimating wild Turkey abundance in the Texas Rolling Plains. J. Wildlife Manage. 71, 1639–1645. doi: 10.2193/2006-254
Butler M. J., Strobel B. N., Eichhorn C., Moon J. A. (2019). Whooping crane winter abundance survey protocol: Aransas National Wildlife Refuge. Version 2.0. Survey Identification Number: FF02RTAR00–002. U.S. Fish and Wildlife Service: Austwell, Texas, USA
Camphuysen C. J., Fox A. D., Leopold M. F., Petersen I. K. (2004). Towards standardised seabirds at sea census techniques in connection with environmental impact assessments for offshore wind farms in the UK (Report No. COWRIE-BAM-02–2002). Report by Royal Netherlands Institute for Sea Research (NIOZ). Rep. Collab. Offshore Wind Res. Into Environ. (COWRIE). doi: 10.13140/RG.2.1.2230.0244
Caughley G. (1977). Sampling in aerial survey. J. Wildlife Manage. 41, 605–615. doi: 10.2307/3799980
Chabot D., Dillon C., Francis C. (2018). An approach for using off-the-shelf object-based image analysis software to detect and count birds in large volumes of aerial imagery. Avian Conserv. Ecol. 13, 15. doi: 10.5751/ACE-01205-130115
Chen L., Zheng Y., Xiao J. (2022). “Rethinking data augmentation for robust visual question answering,” in Computer Vision – ECCV. Eds. Avidan S., Brostow G., Cissé M., Farinella G. M., Hassner T. (Springer Nature, Switzerland), 95–112.
Conroy M. J., Harris G., Stewart D. R., Butler M. J. (2018). Evaluation of desert bighorn sheep abundance surveys, southwestern Arizona, USA. J. Wildlife Manage. 82, 1149–1160. doi: 10.1002/jwmg.21463
Conroy M. J., Henry R. S., Harris G. (2014). Estimation of regional sheep abundance based on group sizes. J. Wildlife Manage. 78, 904–913. doi: 10.1002/jwmg.731
Davis K. L., Silverman E. D., Sussman A. L., Wilson R. R., Zipkin E. F. (2022). Errors in aerial survey count data: Identifying pitfalls and solutions. Ecol. Evol. 12, e8733. doi: 10.1002/ece3.8733
Fiori L., Doshi A., Martinez E., Orams M. B., Bollard-Breen B. (2017). The use of unmanned aerial systems in marine mammal research. Remote Sens. 9, 543. doi: 10.3390/rs9060543
Frederick P. C., Hylton B., Heath J. A., Ruane M. (2003). Accuracy and variation in estimates of large numbers of birds by individual observers using an aerial survey simulator. J. Field Ornithol 74, 281–287. doi: 10.1648/0273-8570-74.3.281
Henriksen M. V. J., Hangstrup S., Work F., Krogsgaard M. K., Groom G. B., Fox A. D. (2015). Flock distributions of lesser flamingos Phoeniconaias minor as potential responses to food abundance-predation risk trade-offs at Kamfers Dam, South Africa. Wildfowl 65, 3–18.
Hodgson J. C., Mott R., Baylis S. M., Pham T. T., Wotherspoon S., Kilpatrick A. D., et al. (2018). Drones count wildlife more accurately and precisely than humans. Methods Ecol. Evol. 9, 1160–1167. doi: 10.1111/2041-210X.12974
Kellenberger B., Veen T., Folmer E., Tuia D. (2021). 21 000 birds in 4.5 h: Efficient large-scale seabird detection with machine learning. Remote Sens. Ecol. Conserv. 7, 445–460. doi: 10.1002/rse2.200
Laliberte A. S., Ripple W. J. (2003). Automated wildlife counts from remotely sensed imagery. Wildlife Soc. Bull. 31, 362–371.
Lippitt C. D., Stow D. A., Clarke K. C. (2014). On the nature of models for time-sensitive remote sensing. Int. J. Remote Sens. 35, 6815–6841. doi: 10.1080/01431161.2014.965287
Luz-Ricca E., Landolt K., Pickens B. A., Koneff M. (2023). Automating sandhill crane counts from nocturnal thermal aerial imagery using deep learning. Remote Sens. Ecol. Conserv. 9, 182–194. doi: 10.1002/rse2.301
Lyons J. E., Runge M. C., Laskowski H. P., Kendall W. L. (2008). Monitoring in the context of structured decision-making and adaptive management. J. Wildlife Manage. 72, 1683–1692. doi: 10.2193/2008-141
McRoberts J. T., Butler M. J., Ballard W. B., Whitlaw H. A., Haukos D. A., Wallace M. C. (2011). Detectability of lesser prairie-chicken leks: A comparison of surveys from aircraft. J. Wildlife Manage. 75, 771–778. doi: 10.1002/jwmg.116
Norouzzadeh M. S., Morris D., Beery S., Joshi N., Jojic N., Clune J. (2021). A deep active learning system for species identification and counting in camera trap images. Methods Ecol. Evol. 12, 150–161. doi: 10.1111/2041-210X.13504
Norton-Griffiths M. (1975). “Counting animals,” in Handbook 1, 2nd (African Wildlife Foundation, Nairobi).
Prenzlow D. M., Lovvorn J. R. (1996). Evaluation of visibility correction factors for waterfowl surveys in Wyoming. J. Wildlife Manage. 60, 286–297. doi: 10.2307/3802226
Proffitt K. M., Garrott R., Gude J. A., Hebblewhite M., Jimenez B., Paterson J. T., et al. (2020). Integrated carnivore-ungulate management: A case study in west-central Montana. Wildlife Monogr. 206, 1–28. doi: 10.1002/wmon.1056
Samuel M. D., Garton E. O., Schlegel M. W., Carson R. G. (1987). Visibility bias during aerial surveys of elk in Northcentral Idaho. J. Wildlife Manage. 51, 622–630. doi: 10.2307/3801280
Sasse D. B. (2003). Job-related mortality of wildlife workers in the United States 1937–2000. Wildlife Soc. Bull. 31, 1015–1020.
Smith G. W. (1995). A Critical Review of the Aerial and Ground Surveys of Breeding Waterfowl in North America. U.S. Department of the Interior, National Biological Service. Washington, DC, USA.
Tuia D., Kellenberger B., Beery S., Costelloe B. R., Zuffi S., Risse B., et al. (2022). Perspectives in machine learning for wildlife conservation. Nat. Commun. 13, 1. doi: 10.1038/s41467-022-27980-y
U.S. Fish and Wildlife Service (USFWS) (2004) The history of North American Waterfowl surveys: 50 years and still counting. Available online at: https://www.fws.gov/waterfowlsurveys/docs/history.pdf (Accessed April 4, 2024).
Weinstein B. G., Garner L., Saccomanno V. R., Steinkraus A., Ortega A., Brush K., et al. (2022). A general deep learning model for bird detection in high-resolution airborne imagery. Ecol. Appl. 32, e2694. doi: 10.1002/eap.2694
Keywords: wildlife inventory, wildlife monitoring, machine learning, deep learning, remote sensing, aerial imaging, artificial intelligence
Citation: Converse RL, Lippitt CD, Koneff MD, White TP, Weinstein BG, Gibbons R, Stewart DR, Fleishman AB, Butler MJ, Sesnie SE and Harris GM (2024) Remote sensing and machine learning to improve aerial wildlife population surveys. Front. Conserv. Sci. 5:1416706. doi: 10.3389/fcosc.2024.1416706
Received: 12 April 2024; Accepted: 21 May 2024;
Published: 05 June 2024.
Edited by:
Maxime Cauchoix, Université Toulouse III Paul Sabatier, FranceReviewed by:
Artur Andriolo, Juiz de Fora Federal University, BrazilCopyright © 2024 Converse, Lippitt, Koneff, White, Weinstein, Gibbons, Stewart, Fleishman, Butler, Sesnie and Harris. This is an open-access article distributed under the terms of the Creative Commons Attribution License (CC BY). The use, distribution or reproduction in other forums is permitted, provided the original author(s) and the copyright owner(s) are credited and that the original publication in this journal is cited, in accordance with accepted academic practice. No use, distribution or reproduction is permitted which does not comply with these terms.
*Correspondence: Rowan L. Converse, cm93YW5jb252ZXJzZUB1bm0uZWR1
†ORCID: Abram B. Fleishman, orcid.org/0000-0002-7209-0899
Rowan L. Converse, orcid.org/0000-0002-0847-0926
Christopher D. Lippitt, orcid.org/0000-0001-7979-7857
Mark D. Koneff, orcid.org/0000-0002-5674-8953
Benjamin G. Weinstein, orcid.org/0000-0002-2176-7935
David R. Stewart, orcid.org/0000-0003-3103-5372
Matthew J. Butler, orcid.org/0000-0001-9864-803X
Steven E. Sesnie, orcid.org/0000-0001-7939-0109
Grant M. Harris, orcid.org/0000-0002-7875-924X