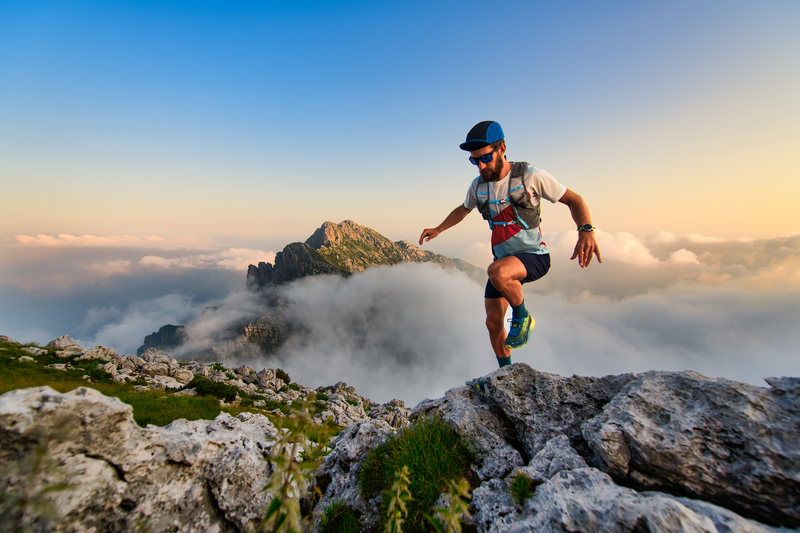
94% of researchers rate our articles as excellent or good
Learn more about the work of our research integrity team to safeguard the quality of each article we publish.
Find out more
ORIGINAL RESEARCH article
Front. Conserv. Sci. , 05 June 2024
Sec. Conservation Genetics and Genomics
Volume 5 - 2024 | https://doi.org/10.3389/fcosc.2024.1366248
Central African rainforests are predicted to be disproportionately affected by future climate change. How species will cope with these changes is unclear, but rapid environmental changes will likely impose strong selection pressures. Here we examined environmental drivers of genomic variation in the central African puddle frog (Phrynobatrachus auritus) to identify areas of elevated environmentally-associated turnover. We also compared current and future climate models to pinpoint areas of high genomic vulnerability where allele frequencies will have to shift the most in order to keep pace with future climate change. Neither physical landscape barriers nor the effects of past Pleistocene refugia influenced genomic differentiation. Alternatively, geographic distance and seasonal aspects of precipitation are the most important drivers of SNP allele frequency variation. Patterns of genomic differentiation coincided with key ecological gradients across the forest-savanna ecotone, montane areas, and a coastal to interior rainfall gradient. Areas of greatest vulnerability were found in the lower Sanaga basin, the southeastern region of Cameroon, and southwest Gabon. In contrast with past conservation efforts that have focused on hotspots of species richness or endemism, our findings highlight the importance of maintaining environmentally heterogeneous landscapes to preserve genomic variation and ongoing evolutionary processes in the face of climate change.
The tropical forests of the Congo Basin and Gulf of Guinea represent one of the most biologically diverse regions in the world. This region ranks third in plant, mammal, bird, and amphibian species richness after the Amazon and New Guinea (Mittermeier et al., 2003). With respect to amphibians, the Cameroon highlands are recognized as one of the world’s most important biodiversity hotspots (Herrmann et al., 2005; Stuart et al., 2008; Gvoždík et al., 2020). Several hypotheses have been advanced to explain the high biodiversity in this region. The more arid conditions during the Pleistocene resulted in fragmented forest habitat, and previous phylogeographic studies have shown that past Pleistocene refugia shaped population structure in several central African rainforest species, providing support for the role of Pleistocene forest refugia as potential engines of diversification (Quérouil et al., 2003; Plana, 2004; Anthony et al., 2007; Born et al., 2011; Nicolas et al., 2011; Murienne et al., 2013). Alternatively, the riverine barrier hypothesis has argued that rivers could have led to the isolation and diversification of tropical forest species (Colyn et al., 1991). Support for this hypothesis has been found in primates (Telfer et al., 2003; Anthony et al., 2007; Mitchell et al., 2015), birds (Aleixo, 2004) and rodents (Nicolas et al., 2011). Compared to these other taxa, far less attention has been paid to assessments of the impact of physical landscape barriers or past Pleistocene refugia on gene flow, or the effects of environmental variation (i.e. precipitation, temperature, and seasonality) on patterns of amphibian diversification in this region.
Environmental variation can act as a strong agent of diversifying selection, particularly in areas of high environmental heterogeneity (Endler, 1973), such as that observed across ecotones (Smith et al., 1997; Freedman et al., 2010; Termignoni-García et al., 2017) or across different levels of elevation (Thomassen et al., 2011). In central Africa, environmental variation has been shown to explain patterns of genetic differentiation in olive sunbirds (Smith et al., 2011), little greenbuls (Smith et al., 1997; Zhen et al., 2017), skinks (Freedman et al., 2010), chimpanzees (Mitchell et al., 2015), forest antelope (Ntie et al., 2017), soft-furred mice (Morgan et al., 2020), and reed frogs (Bell et al., 2017). These heterogeneous environments may capture ecological and evolutionary processes that are fundamental to maintaining and generating biological diversity (Moritz et al., 2000).
One major challenge is being able to effectively partition the effects of isolation by environment (IBE) from other potential drivers of population differentiation, namely: isolation by distance (IBD), isolation by resistance due to physical landscape barriers (IBB), and historical isolation due to past Pleistocene refugia (IBP). Advances in landscape genomics can be used to simultaneously assess the relative importance of competing ecological and historical drivers on genomic differentiation (Manthey and Moyle, 2015; Termignoni-García et al., 2017). Specifically, Fitzpatrick and Keller (2015) have shown that Generalized Dissimilarity Modelling (GDM (Ferrier et al., 2007)) and Gradient Forests (GF (Ellis et al., 2012)) can be powerful tools for analyzing gene–environment associations at the landscape level. Under a model of IBE, genetic differentiation increases with environmental differences between sites, independent of geographic distance (Shafer and Wolf, 2013; Wang and Bradburd, 2014). In contrast, under a model of IBD, genetic differentiation is predicted to increase as a function of geographic distance whereas genetic differentiation under IBB is driven by landscape barriers to animal or plant dispersal (Balkenhol et al., 2017; Cushman and Schwartz, 2006). Resistance distances due to barriers between populations can be based on landscape features that may inhibit gene flow, including physical barriers such as rivers (Mitchell et al., 2015) or changes in elevation (IBB). Lastly, resistance matrices can also be used to model the effects of past refugia (Ntie et al., 2017) (IBP), by hindcasting areas of suitable habitat during the last glacial maximum (LGM) (Nogués-Bravo, 2009). In many of these cases, circuit theory is used to incorporate IBE, IBB, and IBP into models of population connectivity and identify which variables are the most important predictors of gene flow (McRae and Beier, 2007).
Central Africa faces a variety of threats from human activities and is especially vulnerable to climate change (Oates et al., 2004; Laporte et al., 2007; Abernethy et al., 2013; James et al., 2013). Temperatures are expected to rise along with potential shifts in rainfall patterns, including more intense dry seasons that could result in forest retreat (James et al., 2013). Species in this region, if they are to survive, would therefore be forced to respond to climate change either through dispersal, evolutionary adaptation or phenotypic plasticity (Holt, 1990; Davis et al., 2005). Given the threat that climate change poses to many species, there is now an increasing need to identify current and historical drivers of evolutionary diversification and recognize key areas for future conservation where species capacity to adapt is greatest (Anthony et al., 2015). Mapping landscape-level predictions of environmentally-associated genomic variation under both current and projected future environments can shed light on both the ability of populations to persist in their current state as well as their future capacity to respond to change through evolutionary adaptation (Gunderson, 2000; Thrush et al., 2009; Sgrò et al., 2011). In this regard, the term “genomic vulnerability” (Bay et al., 2018; Ruegg et al., 2018) has been used as a measure of the degree of “mismatch”, or “offset”, between current and future projections of environmentally-associated genomic variation and can be used as a proxy for population vulnerability to environmental change (Ruegg et al., 2018). Genomic vulnerability estimates how much allele frequencies would have to change to keep track with the environmental changes predicted to occur at a certain location. Thus, the locations with the greatest vulnerability are those that are predicted to have to undergo the greatest changes in allele frequencies to keep pace with environmental change.
In the present study, we used a combination of statistical methods to determine the potential drivers of genomic diversification in the widespread African puddle frog, Phrynobatrachus auritus across its range in the west Central African countries of Cameroon, Equatorial Guinea, and Gabon. We used geospatial modeling to map patterns of genomic turnover (i.e. the change in allele frequencies with geographic distance) and predict areas of elevated genomic vulnerability. P. auritus serves as an ideal model for examining the effects of environmental heterogeneity because it occurs in a variety of forest types (Zimkus and Schick, 2010) and occupies a wide range of environmental conditions. Findings from these methods were then used to address the following: 1) Does IBE influence genomic differentiation more than IBB or IBP? 2) Are areas of greatest environmentally-associated genomic turnover associated with strong environmental gradients across the landscape? 3) Where is genomic vulnerability predicted to be highest across the study area in response to future climate change?
Frogs were sampled between the months of March and July in 2013, 2014, and 2015. We collected a total of 191 P. auritus from four sites in Cameroon (Campo Ma’an (CM), N = 29; Ebo forest (EF), N = 24; Ndikiniméki (ND), N = 13; and Takamanda (TM), N = 15), and five sites in Gabon (Gamba Complex (GC), N = 24; Kessala (KS), N = 21; Lopé (LP), N = 18; Monts de Cristal (MC), N = 22; and Minkébé (MK), N = 25) (Figure 1A). These sites encompassed a range of forest types, namely: lowland rainforest (CM, MK), sub-montane rainforest (EF, TM), forest-savanna ecotone (KS, MC), coastal rainforest (GC), and mixed lowland-agricultural forest (ND). After frogs were euthanized with MS222 solution, muscle and kidney tissue was placed in 95% ethanol prior to DNA extraction. Male frogs were differentiated from female frogs by the presence of a developed vocal sac, indicated by a dark throat and vocal folds. Frogs that could not be sexed by these secondary sexual characteristics were dissected and sexed by either the type of reproductive organ or by the presence of eggs. All animal handling procedures were carried out according to an approved University of New Orleans Institutional Animal Care and Use Committee protocol 12-008. Specimens were deposited at the Museum of Comparative Zoology, Harvard University.
Figure 1 Field sampling and inferred population structure of P. auritus. (A) Map of nine sampling locations: Takamanda (TM), Ndikiniméki (ND), Ebo Forest (EF), Campo Ma’an (CM), Monts de Cristal (MC), Minkébé (MK), Lopé (LP), Kessala (KS), and Gamba Complex (GC). Each point is a sampling site and the colors correspond to the assigned population. The green shading corresponds to forest cover. (B) PCA of 1631 SNPs. Each point presents a sample, and samples are colored by site with similar color shades corresponding to their assigned populations. (C) FastStructure results of 1631 SNPs. Site abbreviations are labeled within each population.
We used five environmental variables to predict the influence of environmental variation on genomic differentiation given their relevance to amphibian ecology and biology as well as their relevance in previous studies (Ficetola and Maiorano, 2016; Morgan et al., 2020). The five bioclimatic variables were: annual temperature, temperature seasonality, annual precipitation, precipitation seasonality, and precipitation of the coldest quarter from the WorldClim database (Fick and Hijmans, 2017) (www.worldclim.org). Precipitation of the coldest quarter was selected because it represents a unique characteristic of precipitation for this region. It effectively reflects the seasonal inversion that occurs approximately across the equator such that the dry season in central Cameroon coincides with the rainy season in northern Gabon and vice versa (Heuertz et al., 2014; Morgan et al., 2020). We then tested for potential correlations between environmental variables to ensure no two variables had a Pearson’s correlation coefficient > 0.8. All climate variables had a resolution of 30 arc-seconds (approximately 1 km2). Although estimates of vegetation cover may also be important determinants of genomic differentiation, the lack of climate projection maps prevents the use of these variables for modeling under future conditions and were therefore not included in the current study. Future projections of bioclimatic variables were taken from aggregated global climate models (Clee, 2017) for two representative concentration pathways (RCPs) 2.6 and 8.5, projected for year 2080 based on the Intergovernmental Panel on Climate Change (IPCC) 5th assessment report. Together, these two RCPs span the greatest range in predicted radiative forcing under climate change and represent “best” and “worst” case scenarios regarding global mean temperature increases.
We extracted genomic DNA from either kidney or muscle tissue using Qiagen DNEasy Blood and Tissue kit (Qiagen, CA), following the manufacturer’s protocol. A total of 164 individuals had genomic DNA of sufficient quantity (> 50 ng) and quality needed for restriction site-associated sequencing (RAD-seq) as determined through gel electrophoresis (Davey et al., 2011). RAD-seq library preparation followed the BestRad protocol for Illumina sequencing as described in Ali et al. (2016). Briefly, genomic DNA (100 ng) was digested with 4.8 units of SbfI-HF restriction enzyme (New England Biolabs NEB, R3632L) at 37°C for 1 h in a 12 µl reaction volume. Samples were heated to 65°C for 20 min and 4 µl of the indexed BestRad SbfI P1 RAD adapter (10 nM) was added to each sample. Ligation of inline barcoded P1 adaptors to digested genomic DNA was performed overnight at 20°C with 640 units of T4 DNA ligase (NEB, M0202M), then 65°C for 20 min. Following ligation, 10 µl of each sample in each 48 well plate was pooled into a single tube and cleaned using 1x Agencourt AMPure XP beads (A63881; Beckman Coulter). Pooled DNA for each plate was then resuspended in 100 µl low TE and sheared to an average fragment size of 500 base pairs using a Bioruptor NGS sonicator (Diagenode). Sheared DNA was then concentrated to 55.5 µl using Ampure XP beads and used as the template in the NEBNext Ultra DNA Library Prep Kit for Illumina (NEB E7370L; v.1.2). The standard NEBNext protocol for library preparation was followed except that we used custom P2 adaptors which were created by annealing a NEBNext Multiplex Oligo for Illumina (NEB, E7335L) to the oligonucleotide GATCGGAAGAGCACACGTCTGAACTCC AGTCACIIIIIIATCAGAACA*A (where * represents a phosphorothioate DNA base). In addition, instead of the USER® enzyme step, we used a universal P1 RAD primer (AATGATACGGCGACCACCGAGATCTAC ACTCTTTCCCTACACGAC*G) and a universal P2 RAD primer (CAAGCAGAAGACGGCATACG*A) during final amplification. The final RAD library was cleaned using AMPure XP beads and sequenced at the UC Berkeley QB3 Vincent J Coates Genome Sequencing Laboratory (GSL) on an Illumina HiSeq2500: Rapid Run Mode (Illumina, San Diego, CA, USA) using paired-end 100-bp sequence reads.
We used the bioinformatics software pipeline, STACKS v.1.44 (Catchen et al., 2011; Catchen et al., 2013) to process the restriction-site-associated DNA markers (RAD-tags) and generate single nucleotide polymorphism (SNP) datasets. First, we executed the “process_radtags” program in STACKS to demultiplex and trim sequence reads by the P1 barcodes and remove low quality reads (Phred quality score less than 20). After removing PCR duplicates with the “clone_filter” script, the processed reads were used to generate RAD loci without a reference genome using “denovo_map.pl” (parameter settings: m = 3 M = 5 n = 4). We empirically determined these parameters to limit the impact of over-splitting loci (Ilut et al., 2014; Harvey et al., 2015). This involved running the de novo assembly over a wide range of values of M (1–8) with “ustacks”. From these runs, we selected a value of M = 5 since we observed that the percentage of homozygous and heterozygous loci reached a stable value and thus minimized over-splitting of alleles for the final SNP calling.
Stacks calls SNPs (“sstacks”) within RAD loci using a multinomial-based likelihood model that estimates the likelihood of the two most frequently observed genotypes at each site and performs a standard likelihood ratio test using a chi-square distribution (Hohenlohe et al., 2010; Catchen et al., 2011). For SNP inference, we used the default alpha significance level of 0.05. Paralogous loci that stacked together were identified and removed by subsequent quality control steps built into STACKS (max number of stacks per loci (m) = 3 (Ilut et al., 2014; Harvey et al., 2015)). After the preliminary assembly of catalog loci using “denovo_map.pl”, we ran the STACKS correction mode (rxstacks-cstacks-sstacks) using the bounded SNP model with a 0.05 upper bound for the error rate. The “rxstacks” program made corrections to genotype and haplotype calls based on population information, rebuilt the catalog loci and filtered out loci with average log likelihood ratio of < 8.0.
We used three additional filtering steps to generate a set of high-quality RAD loci for downstream population genetic analysis. First, we retained only RAD loci that were present in 80% of all samples. Second, we removed RAD loci that contained more than 40 SNPs, as these likely represented sequencing errors or over-clustering of paralogous loci. Lastly, we used the BLAT alignment algorithm (Kent, 2002) to de novo align the RAD loci and remove those that aligned to multiple positions. The final consensus set of RAD loci comprised SNP data from a total of 139 individuals. Genotypes were called, filtered, and bi-allelic SNPs were exported in VCF format using the STACKS “populations” program. SNPs from the last seven bp of the RAD loci were removed as this part of the locus is likely to contain sequence errors at the 3’ end of the reads. The SNP dataset was further filtered with VCFtools v.0.1.14 (Danecek et al., 2011) to remove SNPs below a minor allele frequency (MAF) of 0.05 cutoff to reduce artifacts of sequence and assembly error. Finally, the dataset was filtered to include only one random SNP per RAD locus for use in all downstream analyses in order to avoid linkage disequilibrium between SNPs within RAD loci.
We performed a principal component analysis (PCA) using the Bioconductor package SNPRelate (Zheng et al., 2012) (https://www.bioconductor.org/) to summarize population genomic structure. We used the program FastStructure (Raj et al., 2014) to estimate the number of genetically distinct populations within the sampled P. auritus range. We tested a range of K values (where K denotes the number of inferred populations) from 1 to 10. The script “chooseK.py” included in the FastStructure package was used to determine the best estimate of K that maximizes the marginal likelihood. We also calculated pairwise estimates of FST (Weir and Cockerham, 1984) among sites and among K populations inferred from FastStructure using VCFtools. To test for an IBD effect, a Mantel test was used to assess the correlation between pairwise FST values and geographic distance. Mantel tests were run with 999,999 permutations using the vegan package (Oksanen et al., 2022) in R and are reported using both raw FST and transformed FST/(1-FST) distances, as well as both raw Euclidian geographic distance and log-transformed Euclidean distances (Slatkin, 1995; Rousset, 1997).
We used GDM to compare the importance of IBE to isolation by landscape barriers (IBB) or Pleistocene refugia (IBP) on patterns of genomic differentiation. GDM is a matrix regression technique that evaluates the relationship between site-site dissimilarities in environmental or landscape ‘predictor’ variables and a biotic ‘response’ variable (e.g. pairwise genetic distances). A major advantage of GDM over other modeling methodologies is that it can fit non-linear relationships between environmental variables and the biological response variable through the use of I-spline basis functions (Ferrier et al., 2007). This approach includes straight-line geographic distance as a predictor variable and can also incorporate a range of environmental data layers, and matrices derived from resistance surfaces as different predictors.
Pairwise dissimilarity in genomic composition between sites was modeled using two measures: 1) pairwise FST values and 2) a pairwise Bray-Curtis dissimilarity index based on the presence or absence of a SNP at each locus referred to as the Bray-Curtis allele frequency difference or AFD (Sherwin, 2022). IBE was represented by the set of five environmental variables described previously. In addition to these environmental variables, a set of predictor variables were generated to model the effect of IBB and IBP under the Last Glacial Maximum approximately 21,000 years ago (i.e. IBP). IBB represents physical barriers (elevation and rivers) to gene flow. Pairwise resistance distances for IBB were generated by creating raster layers of resistance surfaces based on landscape features, elevation and rivers, using the raster calculator available in QGIS v.2.18. We then calculated pairwise resistance distances from these raster layers with CIRCUITSCAPE 4.0 (McRae et al., 2013). Two IBB matrices were generated, IBB1 and IBB2. For IBB1, resistance values increased with increasing elevation and major rivers were treated as impenetrable. For IBB2, resistance increased with increasing elevation and also with Strahler order, which reflects size and strength of perennial river systems. IBP represents the historical landscape based on modeled available habitat. For IBP, we first projected habitat suitability for P. auritus under climate conditions during the LGM using two global climate models (CCSM and MIROC). We then created resistance surfaces where resistance was considered to be inversely proportional to habitat suitability, and finally, calculated pairwise resistance distances from this raster layer with CIRCUITSCAPE. Further details on how these predictor variables were generated and the resultant distance matrices can be found in Appendix 1. I-spline turnover functions describing the relationship between the biological response variable (pairwise dissimilarity in genomic composition) and each of the predictor matrices were visualized using rug plots and their significance was tested using 1000 permutations.
We evaluated the importance of environmental variables as predictors of environmentally-associated genomic turnover and spatialized these patterns across the study region using GF modeling within the gradientForest package (Ellis et al., 2012) in R (Appendix 1). Response variables were individual SNP minor allele frequencies within each population. Predictor variables were represented by the same environmental variables that were included in the GDM along with latitude and longitude. GF uses a machine-learning algorithm to divide the biological data into different bins (i.e. different values of allele frequencies), with partitions occurring at several split values along each environmental variable. This binning is performed for every SNP, weighting each SNP individually according to its fit to the model (i.e. R2) before aggregating across all SNPs. GF determines the “split importance” by measuring the amount of biological variation explained by a given split value (e.g. between 26 and 27°C), which is then cumulatively summed along each gradient to construct turnover functions (Fitzpatrick and Keller, 2015). The top three environmental variables in modeling genomic turnover from a total of 2000 regression trees were used to predict and map environmentally-associated turnover across the study region using a random grid of 100,000 sample points. To ensure that our GF model was performing better than random, we shuffled the environmental-predictor matrix to generate 200 randomized datasets and compared the number of SNP loci with R2 positive values to the mean R2 value across SNP loci using GF models describing variation in the real versus randomized datasets.
Lastly, we predicted future environmentally-associated genomic variation based on projecting GF models under climate change for the years 2050 and 2080 for RCPs 2.6 and 8.5, representing “best” and “worst” cases, respectively. To map predicted changes of genomic variation associated with environment, we calculated the Euclidean distance between models based on current and future climate conditions. Areas where environmentally-associated genomic variation changes the least are considered to have low genomic vulnerability whereas areas where they change the most are considered to have high genomic vulnerability.
RAD-sequencing of 139 P. auritus samples generated a total of 838,425,400 paired-end reads across both plates (308,255,191; 530,170,209) after filtering out low-quality samples and reads. The number of raw sequencing reads per sample ranged from 133,185 to 16 million. The mean coverage depth ranged from 5x to 26x across individual samples (mean = 8x, median = 7x, Appendix 2). From these reads, we assembled 2,979 high-quality RAD loci and a total of 32,966 SNPs that were present in 80% or more samples. Using a minor allele frequency cutoff of 5%, we retained 1631 RAD loci encompassing 3,092 SNPs, and from which we selected one random SNP per RAD locus.
The PCA identified significant population structure across the sampled range of P. auritus. PC1 explained 25.89% of the variation and separated the three northern sites (EF, ND, TM) from the remaining six sites (CM, MC, MK, LP, KS, GC). PC2 explained 9.26% of variation and separated GC, the southernmost coastal site, from all other sites (Figure 1B). FastStructure analyses also revealed a pattern of population structure that is organized latitudinally into five distinct populations: 1) EF, ND, and TM, 2) CM, 3) MC and MK, 4) KS and LP, and 5) GC (Figure 1C, Appendix 3).
Pairwise FST values between sites ranged from 0 to 0.438 (mean = 0.229; Appendix 4), indicating low to moderate levels of genomic differentiation between sites. We found a significant correlation between pairwise FST and geographic distances between the sites (Mantel r = 0.6728; mantel simulated p-value = 0.001), suggesting a strong pattern of IBD.
The GDM based on pairwise FST values and AFD explained 80% and 81% of the variation in the data, respectively. Precipitation of the coldest quarter was the only significant variable (p = 0.02) and the most important variable in the model with FST values. Geographic distance was the only significant variable in the model based on AFD (p = 0.01), however precipitation of the coldest quarter was the most important variable, followed by geographic distance (Table 1). The I-spline plot for precipitation of the coldest quarter shows that observed compositional turnover in genomic differentiation increases with greater levels of precipitation (Supplementary Figure S1). Importantly, neither of the two resistance matrices (IBB1, IBB2) modeling the effects of riverine barriers nor the two matrices modeling the distribution of suitable habitat since the Pleistocene (IBP-CCSM, IBP-MIROC) had any significant effect on the model (i.e. all matrices had a coefficient = 0 except IBP-MIROC).
We used a GF approach to determine associations between SNPs and environmental variables and map environmentally-associated genomic turnover across the total study area. A total of 458 SNPs (28% of all SNPs) had R2 values > 0 (average = 0.28) (Figure 2A). When testing model performance, the number of SNPs with R2 values > 0 for all of the randomized datasets fell below the number observed for the real data (Supplementary Figure S2) and the mean R2 value generated for the real dataset fell within the upper 95% quartile of values generated for the randomized datasets (Supplementary Figure S2), both indicating that the GF model shows a stronger association between environmental and genomic variation for our dataset relative to the set of randomized datasets. Precipitation of the coldest quarter, latitude, and precipitation seasonality were the most important environmental predictors of genomic turnover (Figure 2B). Projected associations between allele frequencies and these three predictor variables revealed areas of pronounced genomic turnover throughout the Cameroonian highlands (green to orange), forest-savanna ecotone of south-central Cameroon (orange to green), across the equator (green to blue), and from the coast to the interior of Gabon (purple to blue) (Figure 2C).
Figure 2 GF results with maps of genomic variation and genomic vulnerability. (A) PC plot with labeled vectors indicating the direction and relative magnitude of environmental variables with the greatest contribution to the predicted patterns of SNP allele frequency differentiation. Vectors for precipitation of the coldest quarter and latitude are overlapping. Each point is a SNP and the color gradient corresponds to the map in panel C with circles indicating sampling sites. (B) Environmental and geographic variables ranked by their importance in explaining SNP allele frequency variation. (C) Map of the GF model of environmentally-associated SNPs for P. auritus. Larger color differences between any two areas in the landscape correspond to larger genetic differences. Circles indicate sampling sites. (D) Genomic vulnerability under climate projection RCP 8.5 for the year 2080. Red indicates greater changes in allele frequencies and higher genomic vulnerability, while blue indicates smaller changes in allele frequencies and less genomic vulnerability.
Predictions of environmentally-associated genomic turnover under future climate change projections showed similar patterns of genotype-environment associations across the landscape relative to current predictions. When we subtracted the current prediction of environmentally-associated genomic turnover from the future predictions under each of the four climate change projections (RCP 2.6 & RCP 8.5 for the years 2050 and 2080), we found a number of areas with high genomic vulnerability across the landscape (Figure 2D; Supplementary Figure S3). Areas with high genomic vulnerability (i.e. greater than 50% difference in environmentally-associated allele frequencies) occur to the north and south of the Cameroon highlands and throughout the southwest region of Gabon.
We adopted a comprehensive statistical approach to disentangling the effects of geographic distance, environmental variation, landscape barriers, and Pleistocene refugia on patterns of genomic differentiation in the African puddle frog P. auritus. Overall, we found that environmental variation plays an important role in shaping patterns of genomic differentiation. This is in addition to, but independent of, geographic distance. In particular, seasonal patterns of precipitation appear to be key in driving patterns of diversification in this tropical region, in keeping with a recent meta-analysis conducted of environmentally-mediated selection across the tropics (Siepielski et al., 2017). Through future modeling approaches, we also find that heterogeneous landscapes overlap with patterns of high environmentally-associated genomic variation, suggesting that they may play an important role in promoting and maintaining biodiversity.
First, we addressed whether IBE will influence genomic differentiation more than IBB or IBP with GDM. Both precipitation of the coldest quarter (FST model) and geographic distance (AFD model) are significant predictors of genomic differentiation. Precipitation of the coldest quarter was also important as an explanatory variable in the AFD model, but despite its importance was not found to be significant. Contrary to many phylogeographic studies that have been carried out previously in central Africa, we did not find evidence for an effect of landscape barriers or Pleistocene refugia on population genomic differentiation. These findings are in stark contrast to many previous studies that have placed emphasis on the role of Pleistocene refugia and/or rivers (Eriksson et al., 2004; Anthony et al., 2007; Nicolas et al., 2011; Bohoussou et al., 2015) with the exception of Bell et al. (Bell et al., 2017) where rivers were not important in reed frog diversification.
Second, we investigated if areas of greatest environmentally-associated genomic turnover are associated with strong environmental gradients across the landscape with GF. Areas of elevated genomic turnover in P. auritus appear to correspond to known ecological gradients. Genomic turnover is predicted to be high throughout the forest-savanna ecotone region south of the montane region in Cameroon where rainforest habitat in the south gradually transitions to savanna in the north. These findings are consistent with patterns of high intraspecific genomic diversity across this ecotonal region in the rainforest bird Andropadus virens (Zhen et al., 2017) and soft-furred mouse Praomys misonnei (Morgan et al., 2020). There is also high genomic turnover in P. auritus across the Cameroon highlands, reflecting both elevation and distance from the coast. The Cameroon highlands are a known biodiversity hotspot, especially for amphibian richness and endemism (Herrmann et al., 2005; Pauwels and Rodel, 2007; Stuart et al. 2008; Zimkus and Gvoždík, 2013) so that elevated genomic turnover in this region is to be expected. Mountain ranges and elevational gradients are often recognized as important drivers of genetic heterogeneity and, as is the case here, are important for the conservation of evolutionary potential.
Considering all analyses, results show a strong role for both distance and environment in shaping genomic differentiation in P. auritus. The role of IBD was supported by findings from GDM, Mantel tests, and the significance of latitude (but not longitude) in predicting genomic turnover in GF analyses, whereas IBE was supported by both GDM and GF. GDM and GF identified precipitation of the coldest quarter as a key driver in genomic differentiation, and GF also identified precipitation seasonality as a top predictor variable. As further support for the role of environment as an important factor, we see those areas of elevated genomic turnover span regions of strong ecological transition, corresponding primarily with patterns of seasonal variation in precipitation. This suggests the role of environmental gradients and ecotones in shaping adaptive environmentally-associated diversification.
Patterns of environmentally-associated genomic differentiation reported here are consistent with previous investigations of gene-environmental associations in this region. For example, precipitation has been shown to be an important predictor of patterns of genetic variation in central African lizards (Freedman et al., 2010), chimpanzees (Mitchell et al., 2015), birds (Smith et al., 2011), and forest antelope (Ntie et al., 2017). In the present study, precipitation of the coldest quarter is highest in the Cameroon highlands and decreases progressively throughout central Cameroon and Gabon (Supplementary Figure S4), mirroring shifts in genomic turnover observed in P. auritus. Conversely, precipitation seasonality is more consistent across the study region with subtle increases in seasonality moving from the Gabon-Cameroon border into northern Cameroon. There are relatively sharper shifts in seasonality with increasing elevation in the Cameroon highlands. Both precipitation patterns demonstrate shifts in genomic differentiation throughout the highlands, across the equator, and subtly from coastal to inland Gabon. Gradients in rainfall not only shape the distribution of forest cover but also present potentially strong selection pressures on the phenology of P. auritus since the timing and duration of amphibian reproductive events are very sensitive to rainfall levels (Corn, 2005; Ficetola and Maiorano, 2016).
Precipitation of the coldest quarter is also indicative of seasonal patterns in rainfall availability that are inverted across the Equator separating Cameroon and Gabon. Rainforests on either side of the equator have their own distinct seasonal patterns of rainfall (Heuertz et al., 2014) such that the dry season in central Cameroon coincides with the rainy season in northern Gabon and vice versa. This seasonal inversion could be responsible for the shift in genomic variation observed in P. auritus across the equator. It has been hypothesized that these contrasting patterns of seasonal rainfall could lead to reproductive isolation and speciation across this region (Heuertz et al., 2014). A life history study of P. auritus found that females lay eggs several times in the year with breeding peaking during the rainy season (Tasse Taboue and Fokam, 2016). Thus, if populations breed at different times either side of the equator, this could result reproductive isolation and account for some of the patterns in genetic differentiation we find here. Future work should look more closely at the seasonal inversion hypothesis and how heterogeneous annual patterns of rainfall influence genomic differentiation in other rainforest species.
Finally, we examined the range of genomic vulnerability across the study region given predicted climate change. We identified multiple areas of high genomic vulnerability where populations may be more susceptible to climate change under future projections. In Cameroon, there are patches of high genomic vulnerability alongside the Cameroon highlands and within the Sanaga basin. Southwest Gabon also encompasses a large area of elevated genomic vulnerability that contains a matrix of forest and savanna ecosystems. Genomic vulnerability may be an important metric to incorporate into conservation prioritization as it may also indicate areas where populations are already susceptible to present-day environmental pressures. For example, Bay et al. (Bay et al., 2018) have recently shown that yellow warbler (Setophaga petechia) populations with the highest genomic vulnerability were also experiencing the largest population declines. Therefore, areas of high genomic turnover and vulnerability may be important targets for future conservation efforts since the former serves as centers of high adaptive potential whereas the latter signal susceptibility to environmental change.
Although we adopted a genome-wide approach in the present study, our SNP dataset is only likely to capture a fraction of the total number of loci in the genome that constitute targets for selection and/or regions of the genome that may be linked loci under selection. Further research should focus on linking genotypic variation to phenotypic traits under selection to understand the evolutionary significance of divergence more fully across ecological gradients as well as examine the relative importance of genetic versus environmental factors that may influence morphological variation. This could involve assembling and annotating a reference genome for this species, and sequencing and SNP genotyping candidate genes that could be targets of selection.
Understanding the ecological and historical processes involved in diversification is important not only for increasing our knowledge of evolutionary mechanisms, but also for making evolutionarily informed conservation decisions to protect biodiversity and prioritize new areas for preservation in the light of rapid climate change. By taking a robust statistical approach to disentangling competing drivers of differentiation, we show that environmental factors are largely responsible for patterns of genomic differentiation and genomic turnover in our study species. In contrast, landscape barriers (rivers and elevation) and historical barriers (Pleistocene refugia) to gene flow have little influence on genomic differentiation. These findings, therefore, highlight the importance of preserving heterogeneous environments, such as environmental gradients, in maintaining species potential to respond to future environmental change and underline the importance of considering evolutionary processes in the design of future protected areas.
The original contributions presented in the study are publicly available. This data can be found here: GitHub, https://github.com/cmiller504/p_auritus.
The animal study was approved by University of New Orleans Institutional Animal Care and Use Committee protocol 12-008. The study was conducted in accordance with the local legislation and institutional requirements.
CM: Conceptualization, Formal analysis, Investigation, Writing – original draft, Project administration. GT: Conceptualization, Methodology, Writing – review & editing. EF: Writing – review & editing, Project administration. KM: Conceptualization, Methodology, Writing – review & editing. YZ: Resources, Software, Writing – review & editing. RH: Methodology, Resources, Software, Writing – review & editing. VLU: Resources, Writing – review & editing. KR: Resources, Software, Writing – review & editing. PS: Methodology, Resources, Software, Writing – review & editing. SN: Project administration, Writing – review & editing. PM: Funding acquisition, Project administration, Supervision, Writing – review & editing. JM: Supervision, Writing – review & editing. TF: Writing – review & editing. BZ: Writing – review & editing. TS: Funding acquisition, Supervision, Writing – review & editing. NA: Conceptualization, Funding acquisition, Project administration, Supervision, Writing – review & editing.
The author(s) declare financial support was received for the research, authorship, and/or publication of this article. This work was supported by National Science Foundation grant no. OISE 1243524.
We thank the Agence Nationale des Parcs Nationaux, ANPN (permit #AE130012), Centre National de la Recherche Scientifique et Technologique, CENAREST (permit #AR0010/13, AR0024/14), Ministère des Forêts et de la Faune, MINFOF (permit #153/AO/MINFOF/PNCM, 008/A/MINFOF/R), and Ministère de la Recherche Scientifique et de l’Innovation, MINRESI, as well as all our valuable field guides for helping organize field collections and processing samples for exportation. We also thank University of California, Berkeley’s Vincent J. Coates Genomic Sequencing Laboratory (GSL), for sequencing services.
The authors declare that the research was conducted in the absence of any commercial or financial relationships that could be construed as a potential conflict of interest.
The author(s) declared that they were an editorial board member of Frontiers, at the time of submission. This had no impact on the peer review process and the final decision.
All claims expressed in this article are solely those of the authors and do not necessarily represent those of their affiliated organizations, or those of the publisher, the editors and the reviewers. Any product that may be evaluated in this article, or claim that may be made by its manufacturer, is not guaranteed or endorsed by the publisher.
The Supplementary Material for this article can be found online at: https://www.frontiersin.org/articles/10.3389/fcosc.2024.1366248/full#supplementary-material
Supplementary Figure 1 | GDM I-spline plots. Fitted functions of observed compositional turnover in genomic differentiation for the five significant predictor variables. The maximum height of each curve indicates the total amount of compositional turnover associated with that variable, while holding all other variables constant. The slope of each function indicates the rate of compositional turnover and how this rate varies along the gradient of the predictor variable. Variables with all coefficients=0 are not shown because they have no relationship with the modeled biological pattern.
Supplementary Figure 2 | GF model performance testing results from comparing association between environmental and genomic variation for our dataset relative to the set of randomized datasets. (A) The number of SNPs with R2 values > 0 for all of the randomized datasets fell below the number observed for the real data. (B) The mean R2 value generated for the real dataset fell within the upper 95% quartile of values generated for the randomized datasets.
Supplementary Figure 3 | Map of genomic vulnerability across the landscape for each of the four climate change projections (RCP 2.6 & RCP 8.5 for the years 2050 and 2080). Red indicates greater changes in allele frequencies and thus, higher genomic vulnerability, while blue indicates smaller changes in allele frequencies and less genomic vulnerability.
Supplementary Figure 4 | Map of precipitation of the coldest quarter across the study region. Darker shades of green correspond to more precipitation, measured in millimeters (one millimeter of rainfall is the equivalent of one liter of water per square meter).
Appendix 2 | Output from Stacks including raw sequencing reads and mean coverage depth per sample.
Appendix 3 | FastStructure results including marginal likelihood for all values of K.
Appendix 4 | Pairwise FST values and Mantel test results.
Abernethy K. A., Coad L., Taylor G., Lee M. E., Maisels F. (2013). Extent and ecological consequences of hunting in Central African rainforests in the twenty-first century. Philos. Trans. R. Soc. B: Biol. Sci. 368:20120303. doi: 10.1098/rstb.2012.0303
Aleixo A. (2004). Historical diversification of a terra-firme forest bird superspecies: A phylogeographic perspective on the role of different hypotheses of amazonian diversification. Evol. (N Y) 58, 1303–1317. doi: 10.1111/j.00143820.2004.tb01709.x
Ali O. A., O’Rourke S. M., Amish S. J., Meek M. H., Luikart G., Jeffres C., et al. (2016). RAD capture (Rapture): flexible and efficient sequence-based genotyping. Genetics 202 (2), 389–400. doi: 10.1534/genetics.115.183665
Anthony N. M., Atteke C., Bruford M. W., Dallmeier F., Freedman A., Hardy O., et al. (2015). Evolution and conservation of central African biodiversity: priorities for future research and education in the Congo basin and Gulf of Guinea. Biotropica 47 (1), 6–17. doi: 10.1111/btp.12188
Anthony N. M., Johnson-Bawe M., Jeffery K., Clifford S. L., Abernethy K. A., Tutin C. E., et al. (2007). The role of Pleistocene refugia and rivers in shaping gorilla genetic diversity in central Africa. Proc. Natl. Acad. Sci. U.S.A. 104 (51), 20432–20436. doi: 10.1073/pnas.0704816105
Balkenhol N., Dudaniec R. Y., Krutovsky K. V., Johnson J. S., Cairns D. M., Segelbacher G., et al. (2017). Landscape genomics: understanding relationships between environmental heterogeneity and genomic characteristics of populations. In: Rajora O. (eds) Population Genomics. Population Genomics. Springer, Cham., 261–322. doi: 10.1007/13836_2017_2
Bay R. A., Harrigan R. J., Underwood V. L., Gibbs H. L., Smith T. B., Ruegg K. (2018). Genomic signals of selection predict climate-driven population declines in a migratory bird. Sci. (1979) 361, 83–86. doi: 10.1126/science.aan4380
Bell R. C., Parra J. L., Badjedjea G., Barej M. F., Blackburn D. C., Burger M., et al. (2017). Idiosyncratic responses to climate-driven forest fragmentation and marine incursions in reed frogs from Central Africa and the Gulf of Guinea Islands. Mol. Ecol. 26 (19), 5223–5244. doi: 10.1111/mec.14260
Bohoussou K. H., Cornette R., Akpatou B., Colyn M., Kerbis Peterhans J., Kennis J., et al. (2015). The phylogeography of the rodent genus Malacomys suggests multiple Afrotropical Pleistocene lowland forest refugia. J. Biogeogr 42 (11), 2049–2061. doi: 10.1111/jbi.12570
Born C., Alvarez N., McKey D., Ossari S., Wickings E. J., Hossaert-McKey M., et al. (2011). Insights into the biogeographical history of the Lower Guinea Forest Domain: evidence for the role of refugia in the intraspecific differentiation of Aucoumea klaineana. Mol. Ecol. 20 (1), 131–142. doi: 10.1111/mec.2010.20.issue-1
Catchen J., Hohenlohe P. A., Bassham S., Amores A., Cresko W. A. (2013). Stacks: An analysis tool set for population genomics. Mol. Ecol. 22, 3124–3140. doi: 10.1111/mec.12354
Catchen J. M., Amores A., Hohenlohe P., Cresko W., Postlethwait J. H. (2011). Stacks: Building and genotyping loci de novo from short-read sequences. G3: Genes Genomes Genet. 1, 171–182. doi: 10.1534/g3.111.000240
Clee P. R. S. (2017). Distributions, Drivers, and Risks of Wildlife Infectious Diseases across Africa: using geospatial analyses to elucidate disease occurrence in biodiversity hotspots. Drexel University.
Colyn M., Gautier-Hion A., Verheyen W. (1991). A Re-Appraisal of Palaeoenvironmental History in Central Africa: Evidence for a Major Fluvial Refuge in the Zaire basin. J. Biogeogr 18, 403–407. doi: 10.2307/2845482
Corn P. S. (2005). Climate change and amphibians. Anim. Biodivers Conserv. 28, 59–67. doi: 10.32800/abc
Cushman S. A., McKelvey K. S., Hayden J., Schwartz M. K. (2006). Gene flow in complex landscapes: testing multiple hypotheses with causal modeling. The American Naturalist. 168 (4), 486–499. doi: 10.1086/506976
Danecek P., Auton A., Abecasis G., Albers C. A., Banks E., DePristo M. A., et al. (2011). The variant call format and VCFtools. Bioinformatics 27 (15), 2156–2158. doi: 10.1093/bioinformatics/btr330
Davey J. W., Hohenlohe P. A., Etter P. D., Boone J. Q., Catchen J. M., Blaxter M. L.. (2011). Genome-wide genetic marker discovery and genotyping using next-generation sequencing. Nat. Rev. Genet. 12 (7), 499–510. doi: 10.1038/nrg3012
Davis M. B., Shaw R. G., Etterson J. R. (2005). Evolutionary responses to changing climate. Ecology 86, 1704–1714. doi: 10.1890/03-0788
Ellis N., Smith S. J., Roland Pitcher C. (2012). Gradient forests: Calculating importance gradients on physical predictors. Ecology 93, 156–168. doi: 10.1890/11-0252.1
Endler J. A. (1973). Gene flow and population differentiation. Sci. (1979) 179, 243–250. doi: 10.1126/science.179.4070.243
Eriksson J., Hohmann G., Boesch C., Vigilant L. (2004). Rivers influence the population genetic structure of bonobos (Pan paniscus). Mol. Ecol. 13, 3425–3435. doi: 10.1111/j.1365-294X.2004.02332.x
Ferrier S., Manion G., Elith J., Richardson K. (2007). Using generalized dissimilarity modelling to analyse and predict patterns of beta diversity in regional biodiversity assessment. Divers. Distrib 13, 252–264. doi: 10.1111/j.1472-4642.2007.00341.x
Ficetola G. F., Maiorano L. (2016). Contrasting effects of temperature and precipitation change on amphibian phenology, abundance and performance. Oecologia 181, 683–693. doi: 10.1007/s00442-016-3610-9
Fick S. E., Hijmans R. J. (2017). WorldClim 2: new 1-km spatial resolution climate surfaces for global land areas. Int. J. Climatology 37, 4302–4315. doi: 10.1002/joc.5086
Fitzpatrick M. C., Keller S. R. (2015). Ecological genomics meets community-level modelling of biodiversity: Mapping the genomic landscape of current and future environmental adaptation. Ecol. Lett. 18, 1–16. doi: 10.1111/ele.12376
Freedman A. H., Thomassen H., Buermann W., Smith T. B. (2010). Genomic signals of diversification along ecological gradients in a tropical lizard. Mol. Ecol. 19, 3773–3788. doi: 10.1111/j.1365-294X.2010.04684.x
Gunderson L. H. (2000). Ecological resilience-in theory and application. Annu. Rev. Ecol. Syst. 31, 425–439. doi: 10.1146/annurev.ecolsys.31.1.425
Gvoždík V., Nečas T., Dolinay M., Zimkus B. M., Schmitz A., Fokam E. B. (2020). Evolutionary history of the Cameroon radiation of puddle frogs (Phrynobatrachidae: Phrynobatrachus), with descriptions of two critically endangered new species from the northern Cameroon Volcanic Line. PeerJ 8, e8393. doi: 10.7717/peerj.8393
Harvey M. G., Judy C. D., Seeholzer G. F., Maley J. M., Graves G. R., Brumfield R.T. (2015). Similarity thresholds used in DNA sequence assembly fromshort reads can reduce the comparability of population histories across species. PeerJ 3, e895. doi: 10.7717/peerj.895
Herrmann H.-W., Böhme W., Euskirchen O., Hermann P. A., Schmitz A. (2005). African biodiversity hotspots: the amphibians of Mt Nlonako, Cameroon. Salamandra 41, 61–81.
Heuertz M., Duminil J., Dauby G., Savolainen V., Hardy O. J. (2014). Comparative phylogeography in rainforest trees from lower Guinea, Africa. PloS One 9 (1), e84307. doi: 10.1371/journal.pone.0084307
Hohenlohe P. A., Bassham S., Etter P. D., Stiffler N., Johnson E. A., Cresko W. A. (2010). Population genomics of parallel adaptation in threespine stickleback using sequenced RAD tags. PloS Genet. 6 (2), e1000862. doi: 10.1371/journal.pgen.1000862
Holt R. D. (1990). The microevolutionary consequences of climate change. Trends Ecol. Evol. 5, 311–315. doi: 10.1016/0169-5347(90)90088-U
Ilut D. C., Nydam M. L., Hare M. P. (2014). Defining loci in restriction-based reduced representation genomic data from nonmodel species: Sources of bias and diagnostics for optimal clustering. BioMed. Res. Int. 2014, 9. doi: 10.1155/2014/675158
James R., Washington R., Rowell D. P. (2013). Implications of global warming for the climate of African rainforests. Philos. Trans. R. Soc. B: Biol. Sci. 368, 20120298. doi: 10.1098/rstb.2012.0298
Kent W. J. (2002). BLAT—The BLAST-like alignment tool. Genome Res. 12, 656–664. http://www.genome.org/cgi/doi/10.1101/
Laporte N. T., Stabach J. A., Grosch R., Lin T. S., Goetz S. J. (2007). Expansion of industrial logging in Central Africa. Sci. (1979) 316, 1451. doi: 10.1126/science.1141057
Manthey J. D., Moyle R. G. (2015). Isolation by environment in white-breasted nuthatches (Sitta carolinensis) of the Madrean Archipelago sky islands: A landscape genomics approach. Mol. Ecol. 24, 3628–3638. doi: 10.1111/mec.13258
McRae B. H., Beier P. (2007). Circuit theory predicts gene flow in plant and animal populations. Proc. Natl. Acad. Sci. U.S.A. 104, 19885–19890. doi: 10.1073/pnas.0706568104
McRae B. H., Shah V. B., Mohapatra T. K., Anantharaman R. (2013). Circuitscape 4 (Seattle WA: The Nature Conservancy).
Mitchell M. W., Locatelli S., Sesink Clee P. R., Thomassen H. A., Gonder M. K. (2015). Environmental variation and rivers govern the structure of chimpanzee genetic diversity in a biodiversity hotspot. BMC Evol. Biol. 15, 1–13. doi: 10.1186/s12862-014-0274-0
Mittermeier R. A., Mittermeier C. G., Brooks T. M., Pilgrim J. D., Konstant W. R., Da Fonseca G. A., et al. (2003). Wilderness and biodiversity conservation. Proc. Natl. Acad. Sci. U.S.A. 100 (18), 10309–10313. doi: 10.1073/pnas.1732458100
Morgan K., Mboumba J.-F., Ntie S., Mickala P., Miller C. A., Zhen Y., et al. (2020). Precipitation and vegetation shape patterns of genomic and craniometric variation in the central African rodent Praomys misonnei. Proc. Biol. Sci. 287, 20200449. doi: 10.1098/rspb.2020.0449
Moritz C., Patton J. L., Schneider C. J., Smith T. B. (2000). Diversification of rainforest faunas: An integrated molecular approach. Annu. Rev. Ecol. Syst. 31, 533–563. doi: 10.1146/annurev.ecolsys.31.1.533
Murienne J., Benavides L. R., Prendini L., Hormiga G., Giribet G. (2013). Forest refugia in Western and Central Africa as ‘museums’ of Mesozoic biodiversity. Biol. Lett. 9, 20120932. doi: 10.1098/rsbl.2012.0932
Nicolas V., Missoup A. D., Denys C., Kerbis Peterhans J., Katuala P., Couloux A., et al. (2011). The roles of rivers and Pleistocene refugia in shaping genetic diversity in Praomys misonnei in tropical Africa. J. Biogeogr 38 (1), 191–207. doi: 10.1111/jbi.2010.38.issue-1
Nogués-Bravo D. (2009). Predicting the past distribution of species climatic niches. Global Ecol. Biogeography 18, 521–531. doi: 10.1111/j.1466-8238.2009.00476.x
Ntie S., Davis A. R., Hils K., Mickala P., Thomassen H. A., Morgan K., et al. (2017). Evaluating the role of Pleistocene refugia, rivers and environmental variation in the diversification of central African duikers (genera Cephalophus and Philantomba). BMC Evol. Biol. 17, 1–17. doi: 10.1186/s12862-017-1054-4
Oksanen J., Simpson G., Blanchet F., Kindt R., Legendre P., Minchin P., et al. (2022). Vegan: community ecology package. R package version 2. 6–4. Available at: https://CRAN.R-project.org/package=vegan.
Oates J. F., Bergl R. A., Linder J. M. (2004). Africa’s gulf of Guinea forests: biodiversity patterns and conservation priorities. Washington, DC: Conservation International. 90.
Pauwels O. S. G., Rodel M. (2007). Amphibians and National Parks in Gabon, western Central Africa Amphibien und Nationalparks in Gabun, westliches Zentralafrika. Herpetozoa 19, 135–148. Available at: https://pauwelsolivier.com/docs/AmphibiansAndGabonNP_2007.pdf.
Plana V. (2004). Mechanisms and tempo of evolution in the African Guineo-Congolian rainforest. Philos. Trans. R. Soc. B: Biol. Sci. 359, 1585–1594. doi: 10.1098/rstb.2004.1535
Quérouil S., Verheyen E., Dillen M., Colyn M. (2003). Patterns of diversification in two African forest shrews: Sylvisorex johnstoni and Sylvisorex ollula (Soricidae, Insectivora) in relation to paleo-environmental changes. Mol. Phylogenet Evol. 28, 24–37. doi: 10.1016/S1055-7903(03)00027-7
Raj A., Stephens M., Pritchard J. K. (2014). FastSTRUCTURE: Variational inference of population structure in large SNP data sets. Genetics 197, 573–589. doi: 10.1534/genetics.114.164350
Rousset F. (1997). Genetic differentiation and estimation of gene flow from F-statistics under isolation by distance. Genetics 145, 1219–1228. doi: 10.1093/genetics/145.4.1219
Ruegg K., Bay R. A., Anderson E. C., Saracco J. F., Harrigan R. J., Whitfield M., et al. (2018). Ecological genomics predicts climate vulnerability in an endangered southwestern songbird. Ecol. Lett. 21 (7), 1085–1096. doi: 10.1111/ele.12977
Sgrò C. M., Hoffmann A. A., Lowe A. J. (2011). Building evolutionary resilience for conserving biodiversity under climate change. Evol. Appl. 4 (2), 326–337. doi: 10.1111/j.1752-4571.2010.00157.x
Shafer A. B. A., Wolf J. B. W. (2013). Widespread evidence for incipient ecological speciation: A meta-analysis of isolation-by-ecology. Ecol. Lett. 16, 940–950. doi: 10.1111/ele.12120
Sherwin W. B. (2022). Bray-Curtis (AFD) differentiation in molecular ecology: Forecasting, an adjustment (AA), and comparative performance in selection detection. Mol. Ecol. 12 (9), e9176. doi: 10.1002/ece3.9176
Siepielski A. M., Morrissey M. B., Buoro M., Carlson S. M., Caruso C. M., Clegg S. M., et al. (2017). Precipitation drives global variation in natural selection. Sci. (1979) 355 (6328), 959–962. doi: 10.1126/science.aag2773
Slatkin M. (1995). A measure of population subdivision based on microsatellite allele frequencies. Genetics 139, 457–462. doi: 10.1093/genetics/139.1.457
Smith T. B., Thomassen H. A., Freedman A. H., Sehgal R. N., Buermann W., Saatchi S., et al. (2011). Patterns of divergence in the olive sunbird Cyanomitra olivacea (Aves: Nectariniidae) across the African rainforest-savanna ecotone. Biol. J. Linn. Soc. 103 (4), 821–835. doi: 10.1111/bij.2011.103.issue-4
Smith T. B., Wayne R. K., Girman D. J., Bruford M. W. (1997). A role for ecotones in generating rainforest biodiversity. Sci. (1979) 276, 1855–1857. doi: 10.1126/science.276.5320.1855
Stuart S. N., Hoffmann M., Chanson J. S., Cox N. A., Berridge R. J., Ramani P., et al. (eds.) (2008). Threatened amphibians of the world. Lynx Edicions with IUCN—The World Conservation Union, Conservation International and NatureServe. (Lynx Edicions, Barcelona, Spain: IUCN, Gland, Switzerland; and Conservation International, Arlington, Virginia, USA), 16.
Tasse Taboue G. C., Fokam E. B. (2016). Life history of the golden puddle frog, phrynobatrachus auritus boulenger 1900 (Anura: phrynobatrachidae). Int. J. Biol. 8, 77. doi: 10.5539/ijb.v8n3p77
Telfer P. T., Souquiere S., Clifford S. L., Abernethy K. A., Bruford M. W., Disotell T. R., et al. (2003). Molecular evidence for deep phylogenetic divergence in Mandrillus sphinx. Mol. Ecol. 12 (7), 2019–2024. doi: 10.1046/j.1365-294X.2003.01877.x
Termignoni-García F., Jaramillo-Correa J. P., Chablé-Santos J., Liu M., Shultz A. J., Edwards S. V., et al. (2017). Genomic footprints of adaptation in a cooperatively breeding tropical bird across a vegetation gradient. Mol. Ecol. 26 (17), 4483–4496. doi: 10.1111/mec.14224
Thomassen H. A., Fuller T., Buermann W., Mila B., Kieswetter C. M., Jarrín-V P., et al. (2011). Mapping evolutionary process: a multi-taxa approach to conservation prioritization. Evol. Appl. 4 (2), 397–413. doi: 10.1111/j.1752-4571.2010.00172.x
Thrush S. F., Hewitt J. E., Dayton P. K., Coco G., Lohrer A. M., Norkko A., et al. (2009). Forecasting the limits of resilience: Integrating empirical research with theory. Proc. R. Soc. B: Biol. Sci. 276 (1671), 3209–3217. doi: 10.1098/rspb.2009.0661
Wang I. J., Bradburd G. S. (2014). Isolation by environment. Mol. Ecol. 23, 5649–5662. doi: 10.1111/mec.12938
Weir B. S., Cockerham C. C. (1984). Estimating F-statistics for the analysis of population structure. Evolution 38 (6), 1358–1370. doi: 10.2307/2408641
Zhen Y., Harrigan R. J., Ruegg K. C., Anderson E. C., Ng T. C., Lao S., et al. (2017). Genomic divergence across ecological gradients in the Central African rainforest songbird (Andropadus virens). Mol. Ecol. 26 (19), 4966–4977. doi: 10.1111/mec.14270
Zheng X., Levine D., Shen J., Gogarten S. M., Laurie C., Weir B.S. (2012). A high-performance computing toolset for relatedness and principal component analysis of SNP data. Bioinformatics 28 (24), 3326–3328. doi: 10.1093/bioinformatics/bts606
Zimkus B. M., Gvoždík V. (2013). Sky Islands of the Cameroon Volcanic Line: A diversification hot spot for puddle frogs (Phrynobatrachidae: Phrynobatrachus). Zool Scr 42, 591–611. doi: 10.1111/zsc.12029
Keywords: Central Africa, amphibians, RAD-seq, environmental gradients, genomic vulnerability, climate change
Citation: Miller CA, Tasse Taboue GC, Fokam EB, Morgan K, Zhen Y, Harrigan RJ, Le Underwood V, Ruegg K, Clee PRS, Ntie S, Mickala P, Mboumba JF, Fuller T, Zimkus BM, Smith TB and Anthony NM (2024) Environmental variation predicts patterns of genomic variation in an African tropical forest frog. Front. Conserv. Sci. 5:1366248. doi: 10.3389/fcosc.2024.1366248
Received: 05 January 2024; Accepted: 18 April 2024;
Published: 05 June 2024.
Edited by:
Jesus Eduardo Maldonado, Smithsonian Conservation Biology Institute (SI), United StatesReviewed by:
Katherine Andrea Solari, Stanford University, United StatesCopyright © 2024 Miller, Tasse Taboue, Fokam, Morgan, Zhen, Harrigan, Le Underwood, Ruegg, Clee, Ntie, Mickala, Mboumba, Fuller, Zimkus, Smith and Anthony. This is an open-access article distributed under the terms of the Creative Commons Attribution License (CC BY). The use, distribution or reproduction in other forums is permitted, provided the original author(s) and the copyright owner(s) are credited and that the original publication in this journal is cited, in accordance with accepted academic practice. No use, distribution or reproduction is permitted which does not comply with these terms.
*Correspondence: Courtney A. Miller, Y291cnRuZXltaWxsZXJAdWNsYS5lZHU=
Disclaimer: All claims expressed in this article are solely those of the authors and do not necessarily represent those of their affiliated organizations, or those of the publisher, the editors and the reviewers. Any product that may be evaluated in this article or claim that may be made by its manufacturer is not guaranteed or endorsed by the publisher.
Research integrity at Frontiers
Learn more about the work of our research integrity team to safeguard the quality of each article we publish.