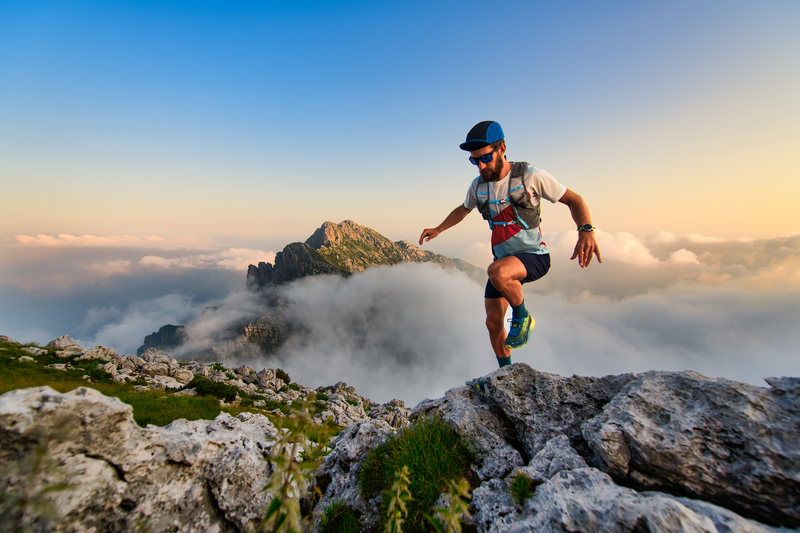
94% of researchers rate our articles as excellent or good
Learn more about the work of our research integrity team to safeguard the quality of each article we publish.
Find out more
ORIGINAL RESEARCH article
Front. Conserv. Sci. , 09 March 2023
Sec. Animal Conservation
Volume 4 - 2023 | https://doi.org/10.3389/fcosc.2023.976914
Introduction: The maintenance of habitat connections between fragmented habitat patches is vital for the conservation of wildlife populations and ecosystem functioning. The awareness of connectivity issues for species conservation has resulted in a growth of connectivity modeling and the application of these results in conservation planning. Such connectivity modeling efforts can include several decisions or data limitations, which could influence the connectivity results and ultimately a systematic conservation plan (SCP). However, there has been little investigation of how these different decisions on species, scale, and extent influence the ultimate conservation planning outcomes.
Methods: We modeled the connectivity of northern bobwhite (Colinus virginianus), North American river otter (Lontra canadensis), and black bear (Ursus americanus), three species with varying ecological requirements, through the Congaree Biosphere Region, South Carolina, USA. We modeled habitat suitability for each species using boosted regression trees and converted these results into resistance layers for the connectivity analyses. We compared models for each species at multistate regional and local extents using directional and omnidirectional circuit theory approaches. We then used the results from each modeling combination as conservation goals for three different SCPs to determine how connectivity modeling decisions may influence geographic conservation decisions.
Results: There was substantial positive spatial correlation between the three connectivity models of each species, and there appeared to be general agreement among mammals as to most important primary corridors. Across all species, the greatest agreement was between the omnidirectional and local directional models as compared with the regional directional plan, which highlighted a unique corridor. The omnidirectional conservation plan required the least amount of planning units to achieve its conservation goals, followed by the local and then regional directional plans that required over 200 km2 more land area to be conserved.
Discussion: Our results indicate that overall variations in connectivity modeling decisions may have only a moderate impact on the identification of important movement corridors for conservation at the local scale. Practitioners should base modeling decisions on the ecology of the study region, conservation question, and available computing resource.
Habitat fragmentation is widely recognized for its deleterious impacts on wildlife populations and ecosystem functions (Foley et al., 2005; Crooks et al., 2011). Fragmentation of habitat into isolated patches can cause a decrease in individual survival, isolation of subpopulations, and a decrease in genetic mixing of a species (Ruiz-Gutiérrez et al., 2008; Aguilar et al., 2019). It can also disrupt ecosystem services such as soil retention and pollination (Lennartsson, 2002; Bryan-Brown et al., 2020). Ecologists and regional planners have increasingly used different connectivity modeling methods to identify the important corridors and pinch points between these habitat fragments in an effort to plan for and maintain connections on the landscape (Spencer et al., 2010; Dutta et al., 2016; Sunny et al., 2022). Thus, increased clarity is needed in how different choices made during the analysis process may have impacts on the identification of important corridors and ultimately on systematic conservation planning.
Connectivity analysis has advanced over the last two decades through the lens of graph theory (Pascual-Hortal and Saura, 2006) from least-cost path and factorial least-cost path analysis (Baldwin et al., 2010; Beier et al., 2011; Rudnick et al., 2012), to resistance kernels, and circuit-theory models (McRae et al., 2008; Mohammadi et al., 2021). The rapid uptake by conservation professionals of landscape connectivity using the Circuitscape analysis (McRae and Shah, 2009), a directional application of circuit theory, has now led to the more recent introduction of Omniscape, an omnidirectional moving-window application of circuit theory (McRae et al., 2016; Dickson et al., 2019; Landau et al., 2021). These analyses often require several arguably subjective decisions about grain size (e.g., raster resolution), study extent, model parameter values, and, in the case of least-cost path methods and Circuitscape, locations of focal nodes (Beier et al., 2008; Beier et al., 2011; Zeller et al., 2018). Circuitscape simulates the directional movement of a pulse of electricity between a pair of focal nodes where increasing density of electrical current values can be interpreted as the increasing probability of individual wildlife movement or gene flow over time (McRae and Beier, 2007). This can especially aid in the identification of important connectivity corridors and pinch points, areas where there may be a substantial geographic narrowing or bottleneck of a corridor (McRae et al., 2008; Pelletier et al., 2014). However, the diffusion of electrical current density across the landscape may differ at different study extents and resolutions potentially highlighting different movement corridors and pinch points in the same study area. Omniscape differs by calculating electrical movement from all potential source pixels within a narrow moving window to a pixel or block of pixels in the center of that window. The moving window iterates across the entire study extent and cumulates electrical current density from all iterations (Landau et al., 2021). This omnidirectional calculation method could identify different corridors than the directional models, as animal movement can come from any direction. Thus, differences in the choice of study extent, data resolution, or model type may ultimately influence wildlife management decisions and conservation planning outcomes.
Systematic conservation planning (SCP) or spatial optimization aims to identify the most efficient means of conserving a combination of specific conservation goals for different features dispersed across a landscape (Margules and Pressey, 2000). Features can vary widely including critical habitat for endangered species, artisanal fishing grounds, or ecosystem services (Beger et al., 2010a; Adame et al., 2015). Conservation goals are then set as a proportion of that feature within the planning study area, proportions ideally set through the input from local stakeholders and conservation scientists. Programs such as Marxan, Zonation, and the “priortizr” package in program R use different modeling algorithms to select planning units (PUs) that best meet conservation goals in an efficient and potentially less costly manner (Ball et al., 2009; Hanson et al., 2020; Moilanen et al., 2022).
At the most basic level, connectivity is incorporated in these programs by the clumping of PUs to create connected intact habitat patches. In Marxan, this is adjusted via the boundary line modifier (BLM), which serves as penalty factor to reduce the edge-to-area ratio, in essence rewarding tighter and tighter groups of planning units the more one increases the BLM. It is also possible to directly incorporate connectivity results into a systematic plan either as a conservation goal for those areas with the highest current density as a connectivity cost (Daigle et al., 2020) or analyzed in a post-hoc fashion (Fajardo et al., 2014).
We focused this study on the potential landscape connectivity of three species, namely, northern bobwhite (Colinus virginanus), North American river otter (Lontra canadensis), and eastern black bear (Ursus americanus). These focal species were chosen due to their varying ecological requirements and the interest from local conservation stakeholders within our study area. Single- and multi-species connectivity results on their own can directly inform land management decisions (Dilts et al., 2016; Wang et al., 2018); however, there is a growing usage of these connectivity results in concert with other conservation concerns in SCPs (Pliscoff et al., 2020). While there is an ongoing and important focus on focal species choice (Breckheimer et al., 2014; Meurant et al., 2018) and the calculation of resistance surfaces (Zeller et al., 2012; Peterman et al., 2019), less attention has been applied to how different circuit-theory modeling decisions can influence conservation decision-making. The ecological or political reasons for decisions such as spatial extent or data resolution may be different between a connectivity modeling exercise and that for a SCP; therefore, it is fundamental to ask how the decisions made during the connectivity modeling process may influence the ultimate inference from such conservation plans.
The objective of our study is to compare how spatial extent and modeling methodology influence connectivity results within the same study area and how this subsequently influences systematic conservation plans. We modeled connectivity for our three focal species in three different ways: directionally at a local and regional spatial extent and omnidirectionally at a regional extent. We then used these different results as conservation goals for three different SCPs. We hypothesized that northern bobwhite connectivity models would find similar results and concentrate in upland working lands away from impervious human land cover due to the species penchant for early-successional land covers. Conversely, we hypothesized that the three analysis types for bears and otters would all identify the same major bottomland habitat cores as important corridors given the extensive intact forested wetland land cover with very little human development concentrating modeled animal movement flow. However, we hypothesized that current densities in smaller secondary corridors would be significantly different between the three analysis types due to variation in the diffusion of current flow across two different extents and two connectivity model types. Given the general overlap between analysis types, we hypothesized that all SCP models would identify and prioritize planning units within the same major bottomland corridor but that planning units within secondary narrower riparian corridors and some uplands of the study area would vary between the SCPs.
Our study was focused on the UNESCO designated Congaree Biosphere Region (CBR) in the central midlands of South Carolina, USA. The CBR (4,910 km2) spans a five-county area of the Southeastern Coastal Plain ecoregion and includes the urban center of the city of Columbia, bottomland hardwood swamps, and upland piney woods (Figure 1). Bottomland habitat includes extensive tracts of cypress (Taxodium distichum)-tupelo (Nyssa aquatica) swamps along the Wateree and Congaree Rivers including the largest intact old-growth bottomland hardwood forest in the southeastern US within Congaree National Park. The park includes ~6,180 ha of federally designated wilderness that serves as the core-protected area of the biosphere reserve designation (UNESCO, 2021). This provides important habitat for a plethora of species including bald eagle (Haliaeetus leucocephalus), black bear, and American alligator (Alligator mississippiensis). Upland habitat within the biosphere includes urban and exurban development, extensive pine silviculture, agriculture, and US Army Base Fort Jackson. Ft. Jackson is ~21,000 ha of primarily pine woodlands including >2,800 ha of restored longleaf pine (Pinus palustris) habitat (Figure 1), important habitat for the endangered red-cockaded woodpecker (Leuconotopicus borealis), and the dominate upland ecosystem prior to human cultivation and fire suppression (Earley, 2004; Kinzer, 2017).
Figure 1 Connectivity modeling was conducted at a regional extent that included two ecoregions across most of four US states and at a local extent just surrounding the Congaree Biosphere Region (CBR) of South Carolina. The CBR includes the Cowasee Basin, a nearly contiguous expanse of bottomland hardwood swamps buffered by upland forests.
We chose three different focal species that represent a wide swath of ecosystems and species within the southeastern and mid-Atlantic regions of the United States, the northern bobwhite, North American river otter, and eastern black bear. The northern bobwhite is an upland game bird that prefers early successional habitat, agricultural ecotones, and warmer temperature regions (Evans et al., 2014). The species is classified as near threatened by the IUCN, and several states within their range have active conservation initiatives to recover declining populations (GDNR, 2013; Virginia Quail Recovery Initiative, 2015; Birdlife International, 2021). The river otter utilizes rivers and large creeks for food, shelter, and movement and is an important surrogate species for riparian habitat (Helon, 2006; Haskell et al., 2018). Finally, the black bear is an omnivorous generalist in habitat use; however, the species prefers large intact habitat cores and mixed forests (Tredick et al., 2017; Zeller et al., 2019). Both the black bear and the river otter are classified as species of least concern (Garshelis et al., 2016; Serfass, 2021), with varying levels of hunting and trapping activities allowed for both species across the regional study area. We used three focal species in an effort to balance a variation in species habitat preferences, with the computational intensity of connectivity models at a multi-state regional scale, and to produce products reasonable feasible for practitioners to replicate.
We used detection-only species records from the Global Biodiversity Information Facility (GBIF), the majority of these being citizen science data from confirmed “research-grade” records on iNaturalist or eBird (GBIF, 2020) (Figure 2). Due to potential clustering bias of presence-only data to higher human densities or public protected areas, we stratified detections using the SDM Toolbox stratification tool (Brown et al., 2017) to randomly cull points so that no points were within 5 km of each other (Boria et al., 2014; Karelus et al., 2018). We removed all records with >1 km spatial coordinate error distortion and removed records prior to 1980 for bear (n = 407) and otter (n = 312) detections due to coordinate accuracy prior to that period and the year 2000 for bobwhite (n = 1,566), as we were using disturbed lands to model bobwhite habitat use.
Figure 2 Analysis process taken for each focal species. Local circuitscape analysis was conducted at 30-m resolution, and regional circuitscape and omniscape analyses were conducted at 100-m resolution. Marxan systematic conservation plans included a conservation goal for each focal species.
We used two different spatial extents centered around the CBR study area and two different circuit-theory methods to conduct our connectivity analyses. The local spatial extent was contained within South Carolina and included the Southeastern Coastal Plain ecoregion and the northeastern portion of the state. The broader regional spatial extent was focused upon the Piedmont and Southeastern Coastal Plains level 3 ecoregions (U.S. Environmental Protection Agency, 2013) within the states of Georgia, North Carolina, South Carolina, and Virginia (Figure 1) and included at least a 20-km of buffer around the two ecoregions to reduce study boundary bias (Koen et al., 2010).
Given the bias in both presence-only data and the geolocations recorded within these databases, we chose to use proportional cover percentage (i.e., fractional cover) and distance to a covariate rather than the covariates at that exact detection point. We can generalize the covariates in the region around, for example, a roadkill detection by using proportional cover, which provides a continuous representation of spatial heterogeneity between land cover types rather than missing a potentially important habitat covariate entirely when using a categorical dataset (Cushman et al., 2013; Bevanda et al., 2014; Wu et al., 2021).
We hypothesized a suite of covariates that a review of the scientific literature indicated as potentially important for the habitat use of one or multiple focal species and generated 30-m resolution rasters of each covariate at the regional extent (Table 1). We used kernel density estimators with a 1-km moving window radius to estimate the proportional cover of wetlands using the National Wetland Inventory (Dertien et al., 2020) and mixed forests and human development using the National Land Cover Database 2016 (U.S. Fish and Wildlife Service, 2017; Jin et al., 2019). We combined all burned and disturbed landcover from the US Geological Survey’s Gap Analysis to create the distance from burned and disturbed land.
Forest edge was derived from the Gap Analysis’ Forest/Open + Woodland/Shrubland Dataset. This data layer is categorically binned with furthest interior and exterior core habitat having the lowest and highest values, respectively. We reclassified this layer into distance bins that emphasized the forested/non-forested ecotone for modeling of the bobwhite species distribution. We used the National Hydrological Dataset (NHD) High Resolution dataset in order to construct the distance to streams and rivers covariate. We removed streams that were lower than the sixth order to better isolate those streams and rivers that would likely be accessible to river otter and in order to reduce the variation in data detail discrepancies of the NHD dataset. Elevation was taken from the USGS database, and slope was derived from this layer. We used simple kriging of a National Center for Atmospheric Research mean annual temperature point dataset to create a continuous 30-m temperature raster of the eastern US. We included temperature and elevation for bobwhite and otters given the large spatial extent and the species complex relationship with upper and lower temperatures (Lusk et al., 2001; Martin et al., 2010; Olsen et al., 2018). We did not use temperature while modeling black bears given the differences in isolated black bear subpopulations across our study region being mostly relegated to either extreme of our temperature gradient. We developed gradient boosted regression tree models in R package “dismo” to estimate the habitat suitability of each focal species at the regional extent (R Core Development Team, 2020; Hijmans et al., 2020). We constructed global models of all covariates hypothesized to be important for a focal species habitat use and used 10-fold cross-validation procedure with a learning rate of 0.001 to determine the optimum number of trees to reduce residual deviance (Table 1). We iteratively removed variables that had<5% contribution to the model and report AUC values for our chosen model. Habitat suitability models were then projected at a resolution of 30 m for the local extent analysis and 100 m for the regional extent analyses.
Since we were interested in resistance to wildlife movement rather than habitat suitability, we used a negative exponential transformation function to transform each habitat suitability layer. This has been shown to be a more realistic representation of landscape resistance, since the probability of habitat use may not correlate perfectly with the permeability of landscape to wildlife movement (Keeley et al., 2016; Zeller et al., 2018).
where R is the ultimate resistance of a pixel, h is the habitat suitability measure for that pixel, and c is a factor determining the degree of curve in the negative exponential function. This reduces the resistance level of mid-range habitat suitability areas while maintaining high resistance in those areas with very low habitat suitability values. Keeley et al. (2016) found a trend towards the best performance at moderate c factors; therefore, we set c = 4 for all three transformations.
To include the representation of primary and secondary highways and high intensity human development on the landscape, we incorporated a reclassified raster of land use informed by McRae et al. (2016) and an extensive review of the primary literature (Supplementary Table S1). We then averaged together the transformed boosted regression tree model and reclassified expert opinion layers to produce the final resistance layers.
We conducted two directional and one omnidirectional connectivity analyses for each of the three focal species to simulate different approaches that could be taken by researchers in order to address our questions concerning variation in results between different spatial extents and connectivity modeling types. We labeled the three different analysis groupings: local circuitscape, regional circuitscape, and omniscape. Local circuitscape used our local extent within South Carolina (Figure 1) and 30-m resolution species resistance layers, regional circuitscape used our regional extent across the South Atlantic states and 100-m resolution species resistance layers, and omniscape utilized the newer omnidirectional application of circuit theory and the same extent and resistance layers as the regional circuitscape. As stated, omniscape uses a moving window approach to model omnidirectional connectivity. For each window calculation, this method simulates electrical movement from all potential source pixels within the window to a pixel or block of pixels in the center of the window and then cumulates across all the window calculations. Therefore, we did not suggest that it was as necessary at this time to determine if spatial extent produced differences in omniscape results. Fluctuating the moving window size, thus changing the available source points in each window calculation, is likely more of a determining factor for the identification of important corridors. We used Circuitscape v5.7 and Omniscape v0.30 for connectivity analyses (Hall et al., 2021; Landau et al., 2021). The local circuitscape analysis was run on 4 CPUs and 16 GB RAM (<2 h), the regional circuitscape analysis was run on 8 CPUs and 120 GB RAM (<1 h), and the omniscape analysis was run on 55 CPUs and 350 GB RAM (approximately 140 h), the latter two on Clemson University’s Palmetto Cluster supercomputer.
We used different focal nodes for the local and regional circuitscape analyses. For the local circuitscape, we established four focal nodes within South Carolina to simulate different potential population movements through the CBR. Nodes were situated >50 km from the CBR boundary to reduce the proximity of node effect (Koen et al., 2014). For the regional circuitscape, we established eight focal nodes, four within Georgia and four within Virginia (Figure 1). Bobwhite focal nodes in Georgia and Virginia were positioned in focal landscapes or priority counties of conservation significance (GDNR, 2013; Virginia Quail Recovery Initiative, 2015). Bear and otter shared focal nodes given the overlap of some habitat requirements. Sites were selected in forested riparian areas where bears are well established in the state (Virginia Department of Game and Inland Fisheries, 2013). Focal nodes in Georgia were placed in river basins in counties with established or directly adjacent to counties with established bear populations, since otters are distributed across the state (Balkcom et al., 2019). Finally, we used a 25-km radius moving window with a 7 × 7 pixel central block size as parameters for the three omniscape analyses to balance analysis detail versus computational intensity (McRae et al., 2016). For purposes of this paper, resolution refers to the pixel size of the raster (e.g., 30 m), and extent refers to the total land area that is included in the connectivity analysis.
Finally, we used the “SpatialPack” package in R to calculate the Pearson’s correlation coefficient within species analysis pairs (Osorio et al., 2020). Raster data were normalized and centered to ensure a comparison on the same scale. We produced 10,000 random points spaced >250 m apart in order to provide a representative sample of the study extent and extracted cell values for all nine rasters at the same points. Covariate processing and statistical analyses were conducted in Program R v3.6 and ArcPro v2.2 (ESRI, Redlands, CA, USA).
We created a grid of 60.7 ha hexagonal PUs across the CBR and summarized cumulative current flow densities for each PU. We isolated the PUs within the top 70th percentile of cumulative current flow values as the most important corridor areas for conservation (Jennings and Zeller, 2017). We locked out PUs in highly urbanized areas (i.e., areas to disregard from potential plans) of the CBR and used the Protected Areas Database of the United States (USGS, 2020) and a local conservation easement database to determine locked-in PUs (i.e., areas already under protection). We set conservation goals as 60% of those PUs within the top 70th percentile for each focal species and complied conservation goals by connectivity analysis type. Finally, we added together the inverse distance from new human development derived from the NLCD 2016 change index (patches >1,800 m2 to minimize erroneous changes) and our proportional cover of human development covariate as a cost raster.
We conducted different SCP analyses for the local and regional circuitscape and omniscape connectivity analyses. We calibrated boundary line modifiers (BLMs), species penalty factors, and model iterations individually for each model using the ArcMarxan toolbox v.2.0 (Apropos Information Systems, Edmonton, AB, Canada). All SCP analyses were conducted in Marxan v.2.4.3 and ArcMap v.10.5.1.
We constructed boosted regression tree SDMs to model our focal species habitat suitability across the regional extent. Black bear models included a positive relationship between the proportional cover (%) of mixed forest and wetlands as two of the highest contributors to the model (AUC = 0.808; SE, 0.015). The best bobwhite model included a positive relationship with increasing temperature and grass/hay cover and a negative relationship with elevation and the distance to burned or recently disturbed habitat. The bobwhite model and other species models did not appear to perform (AUC = 0.686 SE 0.004). The otter model included a negative relationship with distance from a stream or river and forest cover and a positive relationship with temperature (AUC = 0.851; SE, 0.008) (Table 2).
The three different analysis types, despite differences in spatial extent, raster resolution, and connectivity modeling type, identified the same general important corridor areas for each species. The bear and otter analyses pinpointed the bottomland hardwood swamps along the Congaree and Wateree rivers (Figure 3A). These bottomlands are mostly unfragmented in comparison to the surrounding highly modified rural and exurban uplands. The otter analyses also identified several of the same major riparian zones throughout the CBR as important movement corridors for the semi-aquatic species (Figure 3B). The three bobwhite analyses all identified restored long-leaf pine and recently disturbed land on the army base given the species’ penchants for open and early-successional habitats and large agriculture and silviculture upland areas in the center of the CBR between Congaree National Park and Ft. Jackson, as the most important corridors within the Biosphere Region (Figure 3C). Overall, bobwhite current densities were far more diffuse within the CBR as compared to the other two focal species. Pearson’s correlations were all relatively high and positive, especially for bears and otters (r > 0.78; Table 3). Bobwhite correlations were relatively lower, especially between the regional circuitscape and the two other analyses (r < 0.68; Table 3).
Figure 3 Cumulative current density maps for three focal species from three connectivity analysis types within the Congaree Biosphere Region of South Carolina. The three analysis types all displayed similar patterns across the three different species. Corridors were identified for bears and otters in the (A) bottomland hardwoods of the Cowasee Basin and narrower (B) riparian areas for otters. Current densities in bobwhite models were especially concentrated in (C) restored longleaf pine, disturbed army lands, and areas of mixed agriculture.
Table 3 Pearson correlation coefficient values between the three different connectivity current rasters per species using a pairwise modified t-test that accounts for spatial autocorrelation.
We modeled three SCPs using the three analysis types. Boundary-line modifier calibrations were similar for the three plans; therefore, we used the same BLM value across the plans for ease of comparison between plans. As with similarities of within-species analyses, the SCPs had general similarities in the blocks of PUs selected (Figure 4). The bottomland habitat of the Cowasee Basin (the notable “Y” shaped landscape) with its dense riparian habitat and large contiguous core areas was consistently selected as priority areas for conservation. Due to these structural traits, these habitats were the largest corridors from the bear and otter connectivity models and have several protected areas that the Marxan models connected. All three plans selected a large block of planning units in the southeast section of the CBR where planning unit costs were lower and where a bottleneck of open, primarily agricultural, habitat between bottomlands and open water concentrated bobwhite current density values (Figures 3C, 4).
Figure 4 Systematic conservation plans created using inputs from three different connectivity analysis: (A) local circuitscape, (B) regional circuitscape, and (C) omniscape. Each conservation plan includes conservation goals from black bear, bobwhite, and river otter connectivity models. Overlaying the three plans (D) shows that the local circuitscape and omniscape model had the most agreement, while the regional circuitscape model included a secondary corridor in the center of the Congaree Biosphere Region, South Carolina, USA. Planning units that overlapped with protected areas were locked in to each conservation plan.
There were some differences in the exact PUs selected for corridor areas and the number of PUs necessary to achieve conservation goals. The regional circuitscape plan deviated from the local and omniscape plan by prioritizing a second corridor in the center of the biosphere traversing across the uplands between Congaree NP and Ft. Jackson (Figure 4B). This corridor was associated with the directional movement of current in the regional connectivity models from the southwest in Georgia to the northeast in Virginia. The omniscape plan selected the fewest planning units (PU = 3,324 [2,019.33 km2]) necessary to achieve its conservation goals, followed by the local (PU = 3,438 [2,088.59 km2]) and then regional (PU = 3,653 [2,234.39 km2]) circuitscape plans. Thus, compared to the omniscape conservation plan, the local and the regional circuitscape plans required 69.26 and 215.06 km2 more land area, respectively.
We examined if different decisions in spatial extent and model type in the connectivity modeling process influence the ultimate conservation planning inference. Overall, we found that the omniscape analysis produced a plan with the most limited spatial requirements, approximately 10.6% less land area to achieve its goals compared to the regional circuitscape plan. Across connectivity analyses, there was greater spatial correlation among black bear and among otter connectivity analyses, compared to among bobwhite analyses likely due to the more structural diffuse layout of the upland habitat. Despite some of these differences, our findings illustrate that different spatial extents, resolutions, and methodologies may not greatly impact the ultimate conservation recommendations and that broad regional connectivity analyses can justifiably be used for conservation planning at a local extent.
There is extensive evidence for the need to model wildlife connectivity and species distributions at broad spatial extents (Macdonald et al., 2019; Kaszta et al., 2020). Similar studies have been able to compare the influence of spatial grain and compare different methods in nested spatial extents on connectivity results (Krosby et al., 2015; Zeller et al., 2017). As with previous literature, our study has found that different spatial extents and methods can have an impact on the identification of potentially important wildlife corridors (Krosby et al., 2015). However, we have until only recently been able to explore the influence that vastly different spatial extents may have on the same connectivity methodology (Koen et al., 2019). Unlike the limited number of studies that have made these comparisons, we found that while methodologies and study extent may vary, the final conservation recommendations can show general convergence (Figure 4).
Differences between the results of our analyses are partially related to the differences in the directional biases of the different models. The local circuitscape and omniscape connectivity analyses and subsequent conservation plans were more similar than the regional circuitscape analysis in part because they were both modeling the movement of animals coming from multiple directions. Prior to the development of Omniscape, a way to construct omnidirectional circuit-theory analyses was by surrounding the study area of interest with systematically or randomly distanced nodes to model hundreds of pairwise connections (Koen et al., 2014; Pitman et al., 2017). Our local circuitscape analysis followed a similar but simpler method, by using four focal nodes examining how wildlife from multiple directions may move through the study area. In comparison, the regional circuitscape analysis is asking a more bidirectional question of connectivity across a subcontinent, a method that would more likely be used in connectivity studies of future climate scenarios or regional land use change (Terando et al., 2014; Leonard et al., 2016).
Within-species pairwise comparisons between methodologies showed relatively high correlation coefficient values, especially for bear and otter analyses (Table 3), which followed our hypotheses that the different analyses would generally display the same relationships and previous work, which found that a directional and omnidirectional approach identified similar results (Pelletier et al., 2014). However, bobwhite correlations were notably lower than the other two focal species, especially when considering the regional circuitscape analysis (Table 3). Preferred bobwhite upland habitat in our study area is much more diffuse and fragmented in comparison to the well-defined habitat core of bottomland hardwoods preferred in bear and otter models; this likely increased the deviation in how the different circuit-theory models behaved within our study area.
Our study system may be rather unique in the sharp contrast between two large linear intact habitat cores and a fragmented rural/exurban matrix, which could lead to more consensus in connectivity models compared to other study areas. Beyond the boundaries of our study area, at the regional extent, bear and bobwhite connectivity models had heavy diffusion of cumulative current densities in part because of the predominately rural forested or agricultural landscape of Georgia and South Carolina. The lower correlation among bobwhite analyses is an example of the importance of landscape context and how in landscapes with less obvious barriers or pinch points, there could be greater disagreement between different connectivity analysis types (Marrotte and Bowman, 2017; Marrotte et al., 2017). In areas where there is less diffusion of current density, our results point to the conservation importance of large tracts of bottomland hardwood forests in the southeastern US as movement corridors and habitat cores for black bears and otters and of the continued restoration of long-leaf pine and the creation of early successional habitat for northern bobwhite, especially within increasing pinch points near urban and exurban growth.
The three systematic conservation plans identified the same general areas for conservation protection including connections across the bottomland hardwoods to upland protected areas. However, there were some important differences in the number of planning units selected and the number of important corridors. We found that the omniscape plan selected the fewest planning units (i.e., land area) to achieve its conservation goals. The regional circuitscape plan required 215.06 km2 more land area to be protected than the omniscape plan and identified a secondary corridor for protection not present in other plans. This is a substantial difference for a region where most of the remaining land conservation will be done by private land conservation easement and the average land parcel is <35 ha.
While the omniscape plan ultimately required less land to achieve its conservation goals it came at the cost of time and computational resources. Executing the omniscape connectivity analysis required immensely more computing capacity through the use of a supercomputer executing the program for nearly 6 days per species, a level of high-throughput computing capacity likely not available to most conservation practitioners. This is compared to the local circuitscape analysis that was run on a single laptop in <2 h and resulted in a similar and nearly as efficient conservation plan. In addition, requiring less land in one plan does not necessarily mean that that plan will result in as good a conservation outcome as the more demanding plan, but rather that it meets that specific plan’s conservation goals using less area and potentially fewer monetary resources.
Landscape connectivity has been an important fixture in the suite of complementary conservation features since the beginning of systematic conservation planning implementation (Margules and Pressey, 2000; Baldwin et al., 2010). In that time, connectivity in SCPs has been able to transition from a general consideration of the spatial relationships between planning units to incorporating continuous data outputs from advanced ecological models (Beger et al., 2010b; Leonard et al., 2017; Pliscoff et al., 2020). Going forward, it will be important to continue to incorporate inputs that directly model connectivity, such as those from circuit-theory or resistance-kernel-based models, to increase the likelihood of success after conservation plan implementation.
There are multiple ways that circuit-theory model output can be incorporated as an SCP conservation goal. We implemented connectivity results as a simple proportion of planning units within the 70th percentile of current flow densities. While this captured much of the most important areas for the connectivity of our focal species, it may not adequately ensure functional connectivity in other study areas. Other options could include variable goals for different percentiles of current density (e.g., 100% of 90th percentile and 70% of 80th percentile) or conservation goals for different zones to ensure geographic dispersion.
Ultimately, the selection of connectivity modeling method, spatial extent, and resolution depends on the scientific question being asked, purpose of the conservation plan, and computing resources available to researchers. If the conservation goal is to maintain future corridors for genetic and population maintenance than the regional extent makes sense for incapsulating the scale of climatic and ecoregional change (Williams et al., 2005). If instead it is identifying the ideal local watershed for wildlife movement or wildlife crossing, then fine grain local extents using only a few focal nodes is likely robust and amenable to researchers that may not have access to high-throughput computing. What is clear from our results is that encapsulating a much broader extent may not influence the identification of the most important corridors for conservation at the local extent, which could be key for the application of regional connectivity studies at the local level (Figure 3).
Finally, this investigation demonstrates a broader issue as to the continuing focus on improved modeling and methodologies in the wildlife and conservation sciences. Great effort has been expended to the fine-tuning and incorporation of more data into and increased complexity of models to get at a less biased truth (Theobald et al., 2012; Lewis et al., 2018; Marcot and Penman, 2019). However, less attention is given to asking if that investment of energy in broader extents, smaller resolutions, or highly complex models is transforming into better conservation outcomes or if the same decisions or outcomes were possible with much less effort. We will be remiss in wildlife and conservation ecology if we do not continue to use resources to determine if the research that we produce is increasing conservation success and at what point there is diminishing returns from our focus on finer and finer intricacies.
The data presented in the study are deposited in figshare.org repository, accession number https://doi.org/10.6084/m9.figshare.21376140.v1.
JD and RB conceived the ideas and laid out the methodology. JD collected the data, created data products, analyzed the data, and led the writing of the manuscript. RB supervised the work and contributed to writing and editing of the manuscript. All authors contributed to the article and approved the submitted version.
Funding for this research was provided by the Margaret H. Lloyd-SmartState Endowment.
The authors declare that the research was conducted in the absence of any commercial or financial relationships that could be construed as a potential conflict of interest.
All claims expressed in this article are solely those of the authors and do not necessarily represent those of their affiliated organizations, or those of the publisher, the editors and the reviewers. Any product that may be evaluated in this article, or claim that may be made by its manufacturer, is not guaranteed or endorsed by the publisher.
The Supplementary Material for this article can be found online at: https://www.frontiersin.org/articles/10.3389/fcosc.2023.976914/full#supplementary-material
Adame M. F., Hermoso V., Perhans K., Lovelock C. E., Herrera-Silveira J. A. (2015). Selecting cost-effective areas for restoration of ecosystem services. Conserv. Biol. 29, 493–502. doi: 10.1111/cobi.12391
Aguilar R., Cristóbal-Pérez E. J., Balvino-Olvera F. J., de Jesús Aguilar-Aguilar M., Aguirre-Acosta N., Ashworth L., et al. (2019). Habitat fragmentation reduces plant progeny quality: a global synthesis. Ecol. Lett. 22, 1163–1173. doi: 10.1111/ele.13272
Baldwin R. F., Perkl R. M., Trombulak S. C., Burwell W. B. (2010). Modeling ecoregional connectivity. Eds. Trombulak S. C., Baldwin R. F. (New York: Springer). doi: 10.1007/978-90-481-9575-6
Balkcom G., Bond B., Day K., Frazier S., Hammond A., Larson D., et al. (2019). Strategic management plan for black bears in Georgia, (2019-2028) (Georgia Department of Natural Resouces: Wildlife Resources Division). Available from: https://georgiawildlife.com/sites/default/files/wrd/pdf/management/BearStrategicManagementPlan.pdf.
Ball I. R., Possingham H. P., Watts M. E. (2009). “Marxan and relatives: Software for spatial conservation prioritization,” in Spatial conservation prioritization: Quantitative methods and computational tools. Eds. Moilanen A., Wilson K. A., Possingham H. P. (Oxford, England: Oxford University Press), 185–195. Available at: www.ecology.uq.edu.au/marxan.
Beger M., Grantham H. S., Pressey R. L., Wilson K. A., Peterson E. L., Dorfman D., et al. (2010a). Conservation planning for connectivity across marine, freshwater, and terrestrial realms. Biol. Conserv. 143, 565–575. doi: 10.1016/j.biocon.2009.11.006
Beger M., Linke S., Watts M., Game E., Treml E., Ball I., et al. (2010b). Incorporating asymmetric connectivity into spatial decision making for conservation. Conserv. Lett. 3, 359–368. doi: 10.1111/j.1755-263X.2010.00123.x
Beier P., Majka D. R., Spencer W. D. (2008). Forks in the road: Choices in procedures for designing wildland linkages. Conserv. Biol. 22, 836–851. doi: 10.1111/j.1523-1739.2008.00942.x
Beier P., Spencer W., Baldwin R. F., McRae B. H. (2011). Toward best practices for developing regional connectivity maps. Conserv. Biol. 25, 879–892. doi: 10.1111/j.1523-1739.2011.01716.x
Bevanda M., Horning N., Reineking B., Heurich M., Wegmann M., Mueller J. (2014). Adding structure to land cover - using fractional cover to study animal habitat use. Mov. Ecol. 2, 1–10. doi: 10.1186/s40462-014-0026-1
Birdlife International (2021). Colinus virginianus. The IUCN red list of threatened species (International Union for Conservation of Nature). Available at: https://www.iucnredlist.org/species/22728956/178045540.
Boria R. A., Olson L. E., Goodman S. M., Anderson R. P. (2014). Spatial filtering to reduce sampling bias can improve the performance of ecological niche models. Ecol. Modell. 275, 73–77. doi: 10.1016/j.ecolmodel.2013.12.012
Breckheimer I., Haddad N. M., Morris W. F., Trainor A. M., Fields W. R., Jobe R. T., et al. (2014). Defining and evaluating the umbrella species concept for conserving and restoring landscape connectivity. Conserv. Biol. 28, 1584–1593. doi: 10.1111/cobi.12362
Brown J. L., Bennett J. R., French C. M. (2017). SDMtoolbox 2.0: The next generation Python-based GIS toolkit for landscape genetic, biogeographic and species distribution model analyses. PeerJ 2017. doi: 10.7717/peerj.4095
Bryan-Brown D. N., Connolly R. M., Richards D. R., Adame F., Friess D. A., Brown C. J. (2020). Global trends in mangrove forest fragmentation. Sci. Rep. 10, 1–8. doi: 10.1038/s41598-020-63880-1
Crooks K. R., Burdett C. L., Theobald D. M., Rondinini C., Boitani L. (2011). Global patterns of fragmentation and connectivity of mammalian carnivore habitat. Philos. Trans. R. Soc B Biol. Sci. 366, 2642–2651. doi: 10.1098/rstb.2011.0120
Cushman S. A., Mersmann T. J., Moisen G. G., McKelvey K. S., Vojta C. D. (2013). “Using habitat models for habitat mapping and monitoring,” in A technical guide for monitoring wildlife habitat. Eds. Rowland M. M., Vojta C. D. (Washington, D.C: U.S. Department of Agriculture, Forest Service), 123–136. Available at: http://www.fs.fed.us/research/publications/gtr/gtr_wo89/gtr_wo89_05.pdf.
Daigle R. M., Metaxas A., Balbar A. C., McGowan J., Treml E. A., Kuempel C. D., et al. (2020). Operationalizing ecological connectivity in spatial conservation planning with marxan connect. Methods Ecol. Evol. 11, 570–579. doi: 10.1111/2041-210X.13349
Dertien J. S., Self S., Ross B. E., Barrett K., Baldwin R. F. (2020). The relationship between biodiversity and wetland cover varies across regions of the conterminous united states. PloS One 15, 1–18. doi: 10.1371/journal.pone.0232052
Dickson B. G., Albano C. M., Anantharaman R., Beier P., Fargione J., Graves T. A., et al. (2019). Circuit-theory applications to connectivity science and conservation. Conserv. Biol. 33, 239–249. doi: 10.1111/cobi.13230
Dilts T. E., Weisberg P. J., Leitner P., Matocq M. D., Inman R. D., Nussear K. E., et al. (2016). Multiscale connectivity and graph theory highlight critical areas for conservation under climate change. Ecol. Appl. 26, 1223–1237. doi: 10.1890/15-0925
Dutta T., Sharma S., McRae B. H., Roy P. S., DeFries R. (2016). Connecting the dots: mapping habitat connectivity for tigers in central India. Reg. Environ. Change 16, 53–67. doi: 10.1007/s10113-015-0877-z
Earley L. S. (2004). Looking for longleaf: The fall and rise of an American forest (Chapel Hill, NC, USA: University of North Carolina Press).
Evans K. O., Burger L. W., Riffell S. K., Smith M. D., Twedt D. J., Wilson R. R., et al. (2014). Avian response to conservation buffers in agricultural landscapes during winter. Wildl. Soc Bull. 38, 257–264. doi: 10.1002/wsb.405
Fajardo J., Lessmann J., Bonaccorso E., Devenish C. (2014). Combined use of systematic conservation planning, species distribution modelling, and connectivity analysis reveals severe conservation gaps in a megadiverse country (Peru). PloS One 9, 1–23. doi: 10.1371/journal.pone.0114367
Foley J. A., DeFries R., Asner G. P., Barford C., Bonan G., Carpenter S. R., et al. (2005). Global consequences of land use. Science 309, 570–574. doi: 10.1126/science.1111772
Garshelis D. L., Scheick B. K., Doan-Cride D. L., Beechman J. J., Obbard M. E. (2016). Ursus americanus. IUCN red list threat. species e.T41687A1 International Union for Conservation of Nature. doi: 10.2305/IUCN.UK.2016-3.RLTS.T41687A45034604.en
GBIF (2020). GBIF Occurrence Download. Available at: https://doi.org/10.15468/dl.bc1eoi (Accessed January 5, 2020).
GDNR (2013). Georgia’s bobwhite quail initiative 2013-2023. Georg. Dep. Nat. Resour. Wildl. Resour. Div. 1–4. Available at: https://georgiawildlife.com/sites/default/files/wrd/pdf/management/GA_BQI_13-23_ExecutiveSummary.pdf.
Hall K. R., Anantharaman R., Landau V. A., Clark M., Dickson B. G., Jones A., et al. (2021). Circuitscape in julia: Empowering dynamic approaches to connectivity assessment. Land 10, 1–24. doi: 10.3390/land10030301
Hanson J. O., Schuster R., Morrell N., Strimas-Mackey M., Watts M. E., Arcese P., et al. (2020) Prioritizr: Systematic conservation prioritization in r. R packag. version 5.0.1.6. Available at: https://cran.r-project.org/web/packages/prioritizr.
Haskell D. E., Webster C. R., Flaspohler D. J., Meyer M. W. (2018). Relationship between carnivore distribution and landscape features in the northern highlands ecological landscape of Wisconsin. Am. Midl. Nat. 169, 1–16. doi: 10.1674/0003-0031-169.1.1
Helon D. A. (2006). Summer home range, habitat use, movements, and activity patterns of river otters (Lontra canadensis) in the killbuck watershed, northeastern Ohio (Morgantown, WV: University of West Virginia). doi: 10.3390/ijms150916430
Hijmans R. J., Phillips S., Leathwick J., Elith J. (2020). dismo: Species distribution modeling in r. R package version 1.3. Available at: https://cran.r-project.org/package=dismo.
Jennings M., Zeller K. A. (2017). Comprehensive multi-species connectivity assessment and planning for the highway 67 region of San Diego county, California (San Diego, CA, U.S.A: San Diego State University). Available at: https://sdmmp.com/view_article.php?cid=CID_mjennings@mail.sdsu.edu_596fe044366a2.
Jin S., Homer C., Yang L., Danielson P., Dewitz J., Li C., et al. (2019). Overall methodology design for the united states national land cover database 2016 products. Remote Sens. 11. doi: 10.3390/rs11242971
Karelus D. L., Mccown J. W., Scheick B. K., Oli M. K. (2018). Microhabitat features in influencing habitat use by Florida black bears. Glob. Ecol. Conserv. 13, 1–14. doi: 10.1016/j.gecco.2017.e00367
Kaszta, Cushman S. A., Macdonald D. W. (2020). Prioritizing habitat core areas and corridors for a large carnivore across its range. Anim. Conserv. 23, 607–616. doi: 10.1111/acv.12575
Keeley A. T. H., Beier P., Gagnon J. W. (2016). Estimating landscape resistance from habitat suitability: effects of data source and nonlinearities. Landsc. Ecol. 31, 2151–2162. doi: 10.1007/s10980-016-0387-5
Kinzer M. (2017). Nature’s return: An environmental history of congaree national park (Columbia, SC, USA: University of South Carolina Press).
Koen E. L., Bowman J., Sadowski C., Walpole A. A. (2014). Landscape connectivity for wildlife: Development and validation of multispecies linkage maps. Methods Ecol. Evol. 5, 626–633. doi: 10.1111/2041-210X.12197
Koen E. L., Ellington E. H., Bowman J. (2019). Mapping landscape connectivity for large spatial extents. Landsc. Ecol. 34, 2421–2433. doi: 10.1007/s10980-019-00897-6
Koen E. L., Garroway C. J., Wilson P. J., Bowman J. (2010). The effect of map boundary on estimates of landscape resistance to animal movement. PloS One 5. doi: 10.1371/journal.pone.0011785
Krosby M., Breckheimer I., John Pierce D., Singleton P. H., Hall S. A., Halupka K. C., et al. (2015). Focal species and landscape “naturalness” corridor models offer complementary approaches for connectivity conservation planning. Landsc. Ecol. 30, 2121–2132. doi: 10.1007/s10980-015-0235-z
Landau V., Shah V., Anantharaman R., Hall K. (2021). Omniscape.jl: Software to compute omnidirectional landscape connectivity. J. Open Source Software 6, 2829. doi: 10.21105/joss.02829
Lennartsson T. (2002). Extinction thresholds and disrupted plant-pollinator interactions in fragmented plant populations. Ecology 83, 3060–3072. doi: 10.1890/0012-9658(2002)083[3060:ETADPP
Leonard P. B., Baldwin R. F., Hanks R. D. (2017). Landscape-scale conservation design across biotic realms: Sequential integration of aquatic and terrestrial landscapes. Sci. Rep. 7, 1–12. doi: 10.1038/s41598-017-15304-w
Leonard P. B., Sutherland R. W., Baldwin R. F., Fedak D. A., Carnes R. G., Montgomery A. P. (2016). Landscape connectivity losses due to sea level rise and land use change. Anim. Conserv. 20, 80–90. doi: 10.1111/acv.12289
Lewis K. P., Vander Wal E., Fifield D. A. (2018). Wildlife biology, big data, and reproducible research. Wildl. Soc Bull. 42, 172–179. doi: 10.1002/wsb.847
Lusk J. J., Guthery F. S., DeMaso S. J. (2001). Northern bobwhite (Colinus virginianus) abundance in relation to yearly weather and long-term climate patterns. Ecol. Modell. 146, 3–15. doi: 10.1016/S0304-3800(01)00292-7
Macdonald D. W., Bothwell H. M., Kaszta Ż., Ash E., Bolongon G., Burnham D., et al. (2019). Multi-scale habitat modelling identifies spatial conservation priorities for mainland clouded leopards ( neofelis nebulosa ). Divers. Distrib. 25, 1639–1654. doi: 10.1111/ddi.12967
Marcot B. G., Penman T. D. (2019). Advances in Bayesian network modelling: Integration of modelling technologies. Environ. Model. Software 111, 386–393. doi: 10.1016/j.envsoft.2018.09.016
Margules C. R., Pressey R. L. (2000). Systematic conservation planning. Nature 405, 243–254. doi: 10.1038/35012251
Marrotte R. R., Bowman J. (2017). The relationship between least-cost and resistance distance. PloS One 12, 1–19. doi: 10.1371/journal.pone.0174212
Marrotte R. R., Bowman J., Brown M. G. C., Cordes C., Morris K. Y., Prentice M. B., et al. (2017). Multi-species genetic connectivity in a terrestrial habitat network. Mov. Ecol. 5, 1–11. doi: 10.1186/s40462-017-0112-2
Martin D. J., Mcmillan B. R., Erb J. D., Gorman T. A., Walsh D. P. (2010). Diel activity patterns of river otters (Lontra canadensis) in southeastern Minnesota. J. Mammal. 91, 1213–1224. doi: 10.1644/09-MAMM-A-083.1
McRae B. H., Beier P. (2007). Circuit theory predicts gene flow in plant and animal populations. Proc. Natl. Acad. Sci. U. S. A. 104, 19885–19890. doi: 10.1073/pnas.0706568104
McRae B. H., Dickson B., Keitt T., Shah V. (2008). Using circuit theory to model connectivity in ecology, evolution, and conservation. Ecology 89, 2712–2724. doi: 10.1002/ecy.1674
McRae B., Popper K., Jones A., Schindel M., Buttrick S., Hall K., et al. (2016). Conserving nature’s stage: Mapping omnidirectional connectivity for resilient terrestrial landscapes in the pacific Northwest (Portland, Oregon, U.S.A: The Nature Conservancy). doi: 10.13140/RG.2.1.4158.6166
McRae B. H., Shah V. B. (2009) CIRCUITSCAPE user guide. Available at: http://www.circuitscape.org.
Meurant M., Gonzalez A., Doxa A., Albert C. H. (2018). Selecting surrogate species for connectivity conservation. Biol. Conserv. 227, 326–334. doi: 10.1016/j.biocon.2018.09.028
Mohammadi A., Almasieh K., Wan H. Y., Nayeri D., Alambeigi A., Ransom J. I., et al. (2021). Integrating spatial analysis and questionnaire survey to better understand human-onager conflict in southern Iran. Sci. Rep. 11, 1–12. doi: 10.1038/s41598-021-91921-w
Moilanen A., Kohonen I., Lehtinen P., Jalkanen J., Virtanen E. A., Kujala H. (2022) Zonation 5 v1.0 user manual. Available at: https://zenodo.org/record/5899003.
Olsen B. R. L., Fulbright T. E., Hernández F., Grahmann E. D., Wester D. B., Hehman M. W. (2018). Ground surface vs. black globe temperature in northern bobwhite resource selection. Ecosphere 9. doi: 10.1002/ecs2.2441
Osorio F., Vallejos R., Cuevas F., Mancilla D. (2020). SpatialPack: Tools for assessment the association between two spatial processes. R Packag. version 0.4. Available at: https://cran.r-project.org/package=SpatialPack.
Pascual-Hortal L., Saura S. (2006). Comparison and development of new graph-based landscape connectivity indices: Towards the priorization of habitat patches and corridors for conservation. Landsc. Ecol. 21, 959–967. doi: 10.1007/s10980-006-0013-z
Pelletier D., Clark M., Anderson M. G., Rayfield B., Wulder M. A., Cardille J. A. (2014). Applying circuit theory for corridor expansion and management at regional scales: Tiling, pinch points, and omnidirectional connectivity. PloS One 9, 1–11. doi: 10.1371/journal.pone.0084135
Peterman W. E., Winiarski K. J., Moore C. E., Carvalho C., da S., Gilbert A. L., et al. (2019). A comparison of popular approaches to optimize landscape resistance surfaces. Landsc. Ecol. 34, 2197–2208. doi: 10.1007/s10980-019-00870-3
Pitman R. T., Fattebert J., Williams S. T., Williams K. S., Hill R. A., Hunter L. T. B., et al. (2017). Cats, connectivity and conservation: incorporating data sets and integrating scales for wildlife management. J. Appl. Ecol. 54, 1687–1698. doi: 10.1111/1365-2664.12851
Pliscoff P., Simonetti J. A., Grez A. A., Vergara P. M., Barahona-Segovia R. M. (2020). Defining corridors for movement of multiple species in a forest-plantation landscape. Glob. Ecol. Conserv. 23, e01108. doi: 10.1016/j.gecco.2020.e01108
R Core Development Team. (2020). R: A language and environment for statistical computing. Available at: https://www.r-project.org.
Rudnick D. A., Ryan S. J., Beier P., Cushman S. A., Dieffenbach F., Epps C. W., et al. (2012). The role of landscape connectivity in planning and implementing conservation and restoration priorities. Issues Ecol. 16, 1–23. Available at: https://www.fs.usda.gov/research/treesearch/42229
Ruiz-Gutiérrez V., Gavin T. A., Dhondt A. A. (2008). Habitat fragmentation lowers survival of a tropical forest bird. Ecol. Appl. 18, 838–846. doi: 10.1890/07-1090.1
Serfass T. (2021). Lontra canadensis. IUCN red list threat species (International Union for Conservation of Nature). doi: 10.2305/IUCN.UK.2021-3.RLTS.T12302A164577078.en
Spencer W. D., Beier P., Penrod K., Winters K., Paulman C., Rustigian-Romos H., et al. (2010). California essential habitat connectivity project: A strategy for conserving a connected California. (California Department of Fish and Wildlife). Available at: https://wildlife.ca.gov/Conservation/Planning/Connectivity/CEHC.
Sunny A., López-Sánchez M., Ramírez-Corona F., Suárez-Atilano M., González-Fernández A. (2022). Genetic diversity and functional connectivity of a critically endangered salamander. Biotropica 54, 42–56. doi: 10.1111/btp.13025
Terando A. J., Costanza J., Belyea C., Dunn R. R., McKerrow A., Collazo J. A. (2014). The southern megalopolis: Using the past to predict the future of urban sprawl in the southeast U.S. PloS One 9. doi: 10.1371/journal.pone.0102261
Theobald D. M., Reed S. E., Fields K., Soulé M. (2012). Connecting natural landscapes using a landscape permeability model to prioritize conservation activities in the united states. Conserv. Lett. 5, 123–133. doi: 10.1111/j.1755-263X.2011.00218.x
Tredick C. A., Stauffer D. F., Kelly M. J., Vaughn M. R. (2017). Landscape-level habitat use and movement patterns of black bears in northeastern Arizona. Southwest. Nat. 62, 85–91. doi: 10.1894/0038-4909-62.1.85
UNESCO (2021). Technical Guidelines for Biosphere Reserves. Paris. Available at: https://en.unesco.org/sites/default/files/tgbr_en.pdf.
U.S. Environmental Protection Agency (2013) Level III ecoregions of the continental united states. Available at: https://www.epa.gov/eco-research/level-iii-a.
U.S. Fish and Wildlife Service (2017) National wetlands inventory. Available at: https://www.fws.gov/wetlands/data/mapper.html.
USGS (2020). PAD-US data download. Available at: https://www.usgs.gov/programs/gap-analysis-project/science/pad-us-data-download (Accessed February 6, 2020).
Virginia Department of Game and Inland Fisheries (2013) Black bear management plan 2012-2021. Available at: http://www.dgif.virginia.gov/wildlife/bear/blackbearmanagementplan.pdf.
Virginia Quail Recovery Initiative (2015). The bobwhite bulletin: An update on the Virginia quail recovery initative, (Virginia Quail Initiative). Available at: https://dwr.virginia.gov/wp-content/uploads/bobwhite-bulletin.pdf.
Wang F., McShea W. J., Li S., Wang D. (2018). Does one size fit all? a multispecies approach to regional landscape corridor planning. Divers. Distrib. 24, 415–425. doi: 10.1111/ddi.12692
Williams P., Hannah L., Andelman S., Midgley G., Araújo M., Hughes G., et al. (2005). Planning for climate change: Identifying minimum-dispersal corridors for the cape proteaceae. Conserv. Biol. 19, 1063–1074. doi: 10.1111/j.1523-1739.2005.00080.x
Wu L., Sun C., Fan F. (2021). Estimating the characteristic spatiotemporal variation in habitat quality using the InVEST model–a case study from guangdong–Hong Kong–Macao greater bay area. Remote Sens. 13, 1–24. doi: 10.3390/rs13051008
Zeller K. A., Jennings M. K., Vickers T. W., Ernest H. B., Cushman S. A., Boyce W. M. (2018). Are all data types and connectivity models created equal? validating common connectivity approaches with dispersal data. Divers. Distrib. 24, 868–879. doi: 10.1111/ddi.12742
Zeller K. A., McGarigal K., Cushman S. A., Beier P., Vickers T. W., Boyce W. M. (2017). Sensitivity of resource selection and connectivity models to landscape definition. Landsc. Ecol. 32, 835–855. doi: 10.1007/s10980-017-0489-8
Zeller K. A., McGarigal K., Whiteley A. R. (2012). Estimating landscape resistance to movement: A review. Landsc. Ecol. 27, 777–797. doi: 10.1007/s10980-012-9737-0
Keywords: systematic conservation planning, Circuitscape, Omniscape, Marxan, northern bobwhite, North American river otter, black bear, species distribution model
Citation: Dertien JS and Baldwin RF (2023) Does scale or method matter for conservation? Application of directional and omnidirectional connectivity models in spatial prioritizations. Front. Conserv. Sci. 4:976914. doi: 10.3389/fcosc.2023.976914
Received: 23 June 2022; Accepted: 22 February 2023;
Published: 09 March 2023.
Edited by:
Govindhaswamy Umapathy, Centre for Cellular & Molecular Biology (CCMB), IndiaReviewed by:
Alireza Mohammadi, University of Jiroft, IranCopyright © 2023 Dertien and Baldwin. This is an open-access article distributed under the terms of the Creative Commons Attribution License (CC BY). The use, distribution or reproduction in other forums is permitted, provided the original author(s) and the copyright owner(s) are credited and that the original publication in this journal is cited, in accordance with accepted academic practice. No use, distribution or reproduction is permitted which does not comply with these terms.
*Correspondence: Jeremy S. Dertien, amRlcnRpZUBnLmNsZW1zb24uZWR1
Disclaimer: All claims expressed in this article are solely those of the authors and do not necessarily represent those of their affiliated organizations, or those of the publisher, the editors and the reviewers. Any product that may be evaluated in this article or claim that may be made by its manufacturer is not guaranteed or endorsed by the publisher.
Research integrity at Frontiers
Learn more about the work of our research integrity team to safeguard the quality of each article we publish.