- 1Plant Ecology and Nature Conservation, Institute of Biochemistry and Biology, University of Potsdam, Potsdam, Germany
- 2Biodiversity Research Centre, Agriculture and Natural Resources Sciences, Namibia University of Science and Technology, Windhoek, Namibia
- 3Department of Ecological Dynamics, Leibniz-Institute for Zoo and Wildlife Research, Berlin, Germany
Globally, migratory ungulates are affected by fences. While field observational studies reveal the amount of animal–fence interactions across taxa, GPS tracking-based studies uncover fence effects on movement patterns and habitat selection. However, studies on the direct effects of fences and fence gaps on movement behavior, especially based on high-frequency tracking data, are scarce. We used GPS tracking on three common African antelopes (Tragelaphus strepsiceros, Antidorcas marsupialis, and T. oryx) with movement strategies ranging from range residency to nomadism in a semi-arid, Namibian savanna traversed by wildlife-proof fences that elephants have regularly breached. We classified major forms of ungulate–fence interaction types on a seasonal and a daily scale. Furthermore, we recorded the distances and times spent at fences regarding the total individual space use. Based on this, we analyzed the direct effects of fences and fence gaps on the animals’ movement behavior for the previously defined types of animal–fence interactions. Antelope-fence interactions peaked during the early hours of the day and during seasonal transitions when the limiting resource changed between water and forage. Major types of ungulate–fence interactions were quick, trace-like, or marked by halts. We found that the amount of time spent at fences was highest for nomadic eland. Migratory springbok adjusted their space use concerning fence gap positions. If the small home ranges of sedentary kudu included a fence, they frequently interacted with this fence. For springbok and eland, distance traveled along a fence declined with increasing utilization of a fence gap. All species reduced their speed in the proximity of a fence but often increased their speed when encountering the fence. Crossing a fence led to increased speeds for all species. We demonstrate that fence effects mainly occur during crucial foraging times (seasonal scale) and during times of directed movements (daily scale). Importantly, we provide evidence that fences directly alter antelope movement behaviors with negative implications for energy budgets and that persistent fence gaps can reduce the intensity of such alterations. Our findings help to guide future animal–fence studies and provide insights for wildlife fencing and fence gap planning.
1 Introduction
In the Anthropocene, wildlife is unavoidably confronted with fences (Jakes et al., 2018). Interactions between animals and fences are ubiquitous and entail a high mortality risk. This risk manifests indirectly by either hindering animal access to crucial resources (e.g., Spinage, 1992) or artificially increasing the hunting success of predators near fence lines (e.g., Dupuis-Desormeaux et al., 2016b; Prugh et al., 2019), or directly through entanglement (e.g., Mbaiwa and Mbaiwa, 2006; Safner et al., 2021). Despite much emphasis on such potentially fatal consequences, the impact of most fence interactions is more nuanced: For instance, altered movement behavior leads to overexploitation of food resources [Loxodonta africana (elephant) in southern Africa, Vanak et al., 2010], narrowed or obstructed corridors during seasonal migrations [Odocoileus hemionus (mule deer) in North America, Sawyer et al., 2013], reduced foraging periods [Procapra przewalskii (Przewalski’s gazelle) in East Asia, You et al., 2013], or an alteration of space use in the proximity of fences [Antilocapra americana (pronghorn) in North America, Jones et al., 2022].
The preservation and restoration of ungulates’ migratory movements have been linked to important ecosystem processes (e.g., Kauffman et al., 2021b); however, new border fences increasingly impede wildlife (e.g., Linnell et al., 2016; Safner et al., 2021). Therefore, improved knowledge of the direct effects of fences and passable fence sections (from here on referred to as “fence gaps”) on ungulate movement becomes essential. Fence and fence gap planning, as well as policymaking, require a fundamental understanding of how ungulates interact with fences, how locomotion is affected, and what ultimate consequences emerge, especially concerning the energetic loss faced by the animals (e.g., Hering et al., 2022; Jones et al., 2022) and consequences for the populations (e.g., Van Moorter et al., 2020). One step towards disentangling the complexity of fence effects on wildlife is to evaluate the behavior of ungulates at fences and fence gaps. For instance, understanding how different species interact with specific fence types will help design more wildlife-friendly fences (Segar and Keane, 2020). Identifying peak times of ungulate–fence interactions can prove crucial for efficient fence management to minimize the possible negative effects. Knowing the distances and times ungulates tend to travel along fences is key for fence gap positioning, and evaluating the effects of fences and fence gaps on the locomotion of ungulates could provide profound knowledge for future fence-related policies.
In particular, wildlife-proof fences effectively suppress the movements of mammals and many other non-flying vertebrates, possibly restricting their access to important resources such as forage and water. The latter applies especially to migratory species, which track resources on large spatial scales (e.g., Abrahms et al., 2021; Esmaeili et al., 2021) and hence are more likely to encounter such barriers. Since forms of wildlife-based land use constitute a common and growing practice in southern Africa (e.g., Lindsey et al., 2009; Taylor et al., 2020), wildlife-proof fences are prevalent and can confine wildlife to conservation areas (e.g., Hayward, 2012). Some of the most far-reaching wildlife-proof fences are the veterinary cordon fences (VCFs). Established to minimize wildlife–livestock disease transmission (e.g., foot-and-mouth disease, Caron et al., 2013), VCFs traverse thousands of kilometers across Zimbabwe, Botswana, and Namibia (Cumming et al., 2015). Presumably, breaches or passable sections appear in most wildlife-proof fences, often created by animals. For instance, Lupulella mesomelas (jackal), Mellivora capensis (honey badger), Hystrix cristata (porcupine), and Orycteropus afer (aardvark) undermine fences (Kesch et al., 2014), while elephants often directly damage them (e.g., Mutinda et al., 2014; Mogotsi et al., 2016; Naidoo et al., 2022), thereby eliminating the barrier for other species.
Fence gaps created by elephants are likely located on natural movement corridors. Thus, such gaps provide an ideal setup to study the direct effects of fences and fence gaps on animal movements across spatial and temporal scales. While large-scale migratory movements of ungulates triggered by seasonal changes in food availability lead to periodic peaks in wildlife–fence interactions (e.g., Xu et al., 2021; Hering et al., 2022), many interactions occur daily when home ranges contain fences (e.g., Laguna et al., 2022). At the home range scale, larger herbivores (>1 kg) are severely affected since many species travel long distances to reach forage and water resources (e.g., Bartlam-Brooks et al., 2011; Naidoo et al., 2022). This disturbance is likely more pronounced in semi-arid savannas since the erratic and patchy distributed resources (e.g., Owen-Smith, 2014; Ibrahim et al., 2021) increase migratory movements (Abrahms et al., 2021). Especially in such environments, removing long fence sections or introducing fence gaps can benefit important ecological processes associated with long-distance movements of ungulates, such as dispersed grazing pressure. For instance, Bartlam-Brooks et al. (2011) demonstrated that Equus burchelli (plains zebra) reestablished their migration routes after removing a VCF. Furthermore, Dupuis-Désormeaux et al. (2016a) showed that a selective fence gap design kept Rhinoceros sp. inside a reserve while allowing 37 mammal species to use the wider landscape matrix outside of that reserve. However, our knowledge of fence gaps’ effects on larger herbivores in semi-arid environments remains limited.
Studies on ungulate–fence interactions have focused on crossing behaviors such as passing under or jumping over (e.g., Palmer et al., 1985; Harrington and Conover, 2006; Burkholder et al., 2018), on time taken to cross (e.g., Segar and Keane, 2020), and on classifying interaction types (e.g., Wilkinson et al., 2021; Xu et al., 2021; Hering et al., 2022). For example, in a camera trap study at Lake Nakuru National Park, Kenya, Wilkinson et al. (2021) observed most ungulate–fence interactions during the day and distinguished four behaviors at fences: “cross”, “straddle” (individuals on opposite fence side), “grazing/drinking”, and “vigilance”. Xu et al. (2021) quantified pronghorn and mule deer fence interactions based on hourly GPS-telemetry data in the rangelands of Wyoming, USA, and identified primarily quick interactions (“cross” or “bounce”), even though “average movement”, “tracing”, “back-and-forth”, and “trapped” behaviors were also reported. While these advances contributed significantly to the fence ecology framework suggested by McInturff et al. (2020), studies examining the alteration of ungulate movements directly at fences are scarce (e.g., Goddard et al., 2001; Gulsby et al., 2011; Nandintsetseg et al., 2019; Laguna et al., 2022; Jones et al., 2022). Recently, high-resolution animal tracking data with more than two localizations per hour have become available, allowing the derivation of improved statistics on animal–fence interactions. These encompass movement speeds at fences, the number of crossing attempts, traveled distances along a fence, and times spent at a fence, among other indicators. Furthermore, such tracking data reveal fence gap positions.
In this study, we analyzed the direct effects of wildlife-proof fences and fence gaps on the movement behavior of three southern African ungulate species in a semi-arid savanna landscape in northwestern Namibia. The three species differ in their basic movement strategy: range residency [Tragelaphus strepsiceros (greater kudu)], partial migration [Antidorcas marsupialis (springbok)], and nomadism [Tragelaphus oryx (common eland)]. Within the study region, a private nature reserve borders the Etosha National Park. The areas are separated by Namibia’s VCF and other non-electrified and electrified wildlife-proof fences. The VCF is known to be damaged by elephants regularly, often creating gaps. Wildlife frequently uses these fence gaps to move between the National Park and the private land. Using this natural experimental setup, we identified fence interaction types and quantified the seasonal and daily dynamics of their occurrences for the three movement strategies and types of wildlife-proof fences. While we expected the seasonal dynamics to be more pronounced in the migratory and nomadic species, the daily dynamics should not differ across species but could be related to daily movement modes such as frequent traveling during the morning (e.g., Owen-Smith, 2014; Prokopenko et al., 2017). Based on this, we implicitly evaluated direct fence effects by assessing the intensity of ungulate–fence interaction. While distances traveled along fences relative to overall distance traveled indicated the amount of the locomotion capacity spent dealing with fences, time spent at fences indicated potential time losses relating to other behaviors such as feeding itself (e.g., You et al., 2013) or moving to foraging grounds. In particular, we focused on whether (i) the awareness of a fence gap’s position reduced the traveled distance along a fence. Furthermore, we were interested in the immediate effect of fences on the ungulates’ locomotion since this implies possible direct reductions in energy budgets. Therefore, we (ii) compared movement speeds before, during, and after a fence interaction.
2 Methods
2.1 Study area
The study area was located 80 km southwest of the Etosha pan (15.2235°E, 19.2576°S, Figure 1) at the boundary of the Kunene, Omusati, and Oshana regions in northern Namibia. The core area is a private nature reserve (“Etosha Heights”—EH, 460 km²) along the southern boundary of Etosha National Park (ENP, 22,941 km²). These two areas are separated by a 70-km-long section of Namibia’s VCF. The VCF is composed of two fence lines separated by 10 m. The northern fence line is a 2.8-m-high wildlife-proof fence with 21 strands and the lower 1.5 m is covered with wire mesh. The southern fence line is a 1.5-m-high stock-proof fence with seven strands. EH is separated from adjacent commercial livestock farms by a well-maintained, electrified, and wildlife-proof fence line (2.4 m high, 18 strands). On two of these farms, which are also enclosed with wildlife-proof fences (2.4 m high, multi-strand, lower half with wire mesh, not electrified), further movement data of wild ungulates were captured.
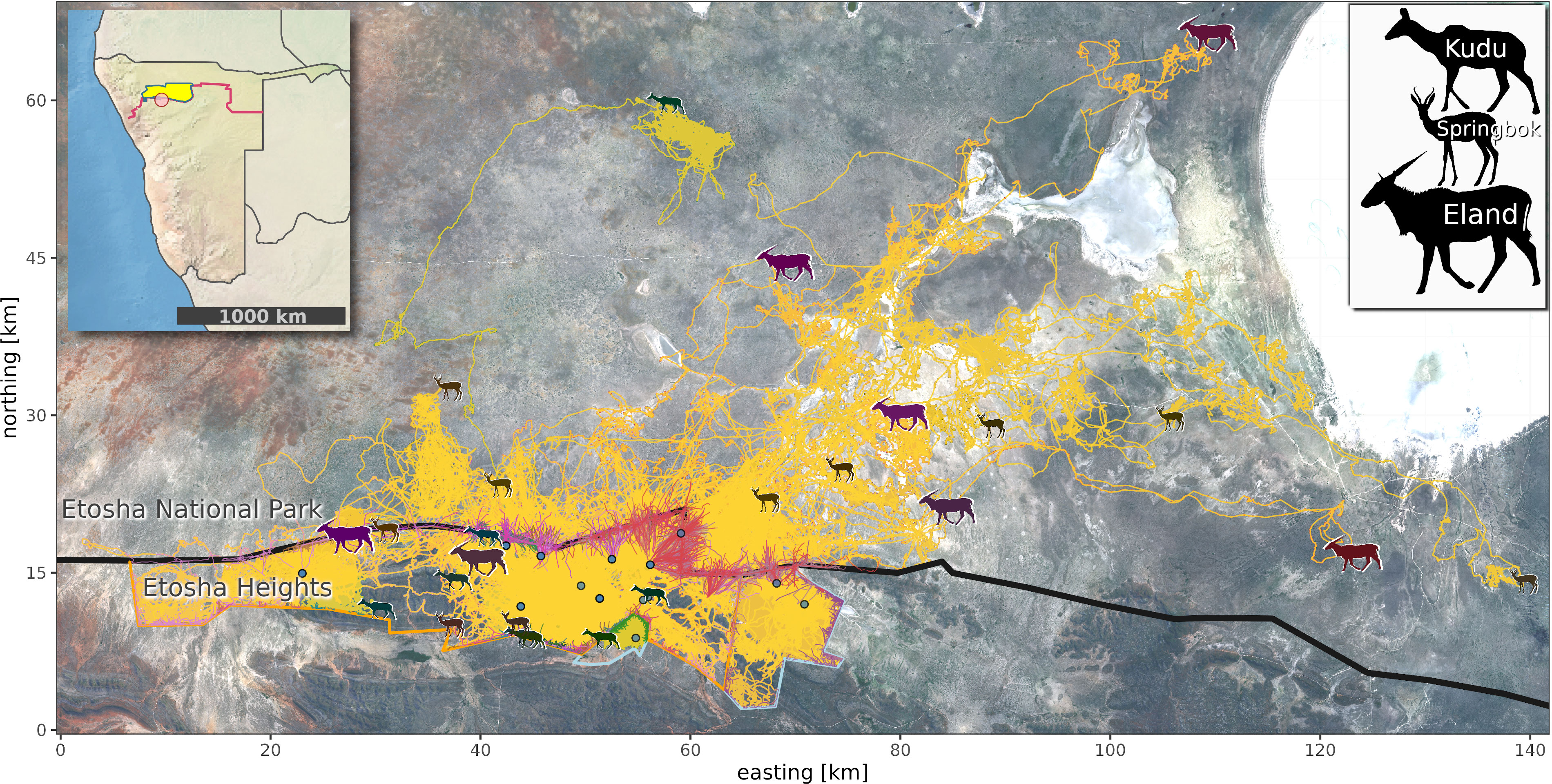
Figure 1 Study area with fence lines (black line—veterinary cordon fence, orange line—electrified fence, blue lines—wildlife-proof fences), movement trajectories (in yellow), and sub-trajectories 45 min before, during, and after fence interactions (colored lines). Species symbols (see legend) depict the farthest position of an individual from its most frequently visited water site (blue points). Background Sentinel 2 (Bands 3,4,5) image, March 2020 (contains modified Copernicus Sentinel data [2020]). Upper left Namibia map shows the veterinary cordon fence (VCF, red line) and focal wildlife conservation areas (yellow area—Etosha National Park, red point—Etosha Heights).
Elephants and other wildlife frequently damaged the VCF to access the well-maintained water points on EH. Damaged sections remained unrepaired for extended periods and, due to personnel and economic limitations, were only sporadically maintained. These sections were frequently damaged again within a few days after maintenance work. The damage characteristics varied from a few broken strands to completely down-trampled strands and wire mesh.
The climate is semi-arid, with highly variable rainfall mainly occurring from September to April (green season; mean temperature: 26°C). The colder dry season lasts from May to August (mean temperature: 18°C). Mean annual precipitation increases from southwest to northeast and is between 250 and 350 mm (averaged from 1981 to 2017 with CHIRPS data, Funk et al., 2015).
Natural surface water is limited to the green season on pans and in some depressions. Artificial water points are the only source of water during the dry season. While such water sources are frequent and well maintained on EH and the livestock farms, they are scarce in the southern parts of ENP.
Vegetation is heterogeneous with mountainous mixed woodlands (dominated by Terminalia prunioides, Commiphora glandulosa, and Combretum apiculatum), woodlands (Colophospermum mopane), shrublands (C. mopane, Catophractes alexandri, and Vachellia nebrownii), or grasslands (dominated by Enneapogon desvauxii, Aristida adscensionsis, and Eragrostis nindensis). During the dry season, the ground is mainly bare. While woody species foliate more periodically (Archibald and Scholes, 2007; Ibrahim et al., 2021) with slight differences in phenology caused by local hydrological conditions, the greening of herbaceous plants is mainly triggered by local rainfall events. Rainfall occurs erratically in both space and time.
2.2 Study species
We studied herd females of three common southern African antelope species with differing movement strategies (i.e., kudu, eland, and springbok) as we were particularly interested in differences among large herbivores (Bunnefeld et al., 2011; Kauffman et al., 2021b). The focal species are of consumptive and non-consumptive economic importance (Lindsey, 2011). Kudu and springbok are widespread and occur naturally on wildlife, mixed, and many cattle farms. Eland have largely been re-established on private reserves and game farms, although they naturally occur in ENP. On EH, eland were relocated from the Waterberg Plateau Park (250 km southeast of the study area) in the early 2000s (A. Nel, personal communication). While springbok primarily crawl under fences and, consequently, are already impeded by cattle fences with closely spaced strands or wire mesh (Bigalke, 1972), eland and kudu can jump over fences up to a height of 2 m (Owen-Smith, 1985). All three species are non- or low-water reliant (Hempson et al., 2015), but during the dry season, they visit water holes frequently, if available.
The greater kudu is a large [weight of females: 152 kg (Skinner and Chimimba, 2005)], social, low-water-dependent browser living in small, relatively constant groups of less than 14 females (Skinner and Chimimba, 2005). We observed group sizes of three to six individuals per collared individual during field observation in the dry season. Kudu are sedentary with rare dispersals from one group to another (Owen-smith, 1990). Except for one, all collared kudus’ movement patterns showed range residency (Figure 2).
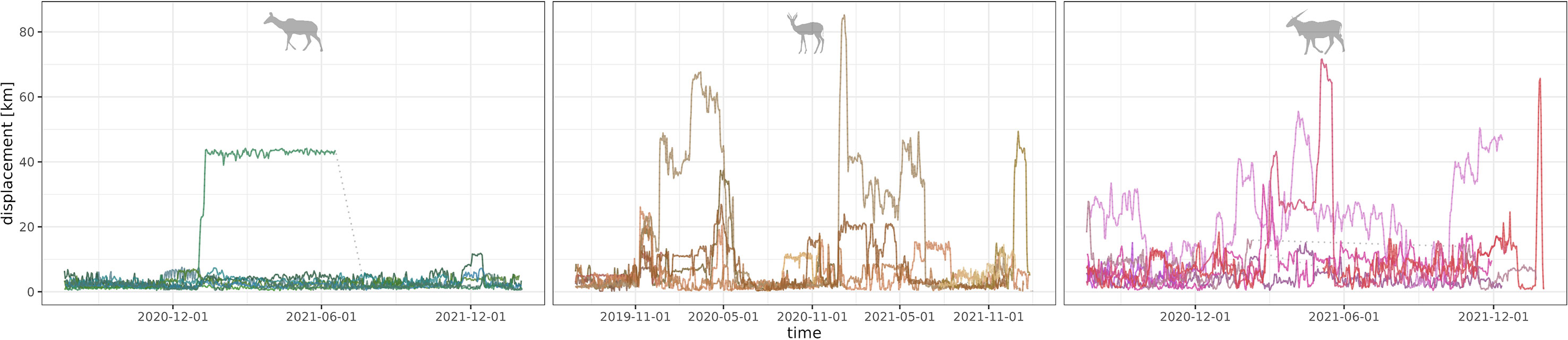
Figure 2 Overview of the individuals’ GPS-based movement patterns. Within species-wise panels (from left to right: kudu, springbok, eland) displacement is shown on the y-axis, measured as distance in km to the most visited waterpoint, along the observation time (x-axis, note the differing observation times). Individual patterns are shown in different colors.
The springbok is a medium-sized [weight of female: 37 kg (Skinner and Chimimba, 2005)], non-water-reliant mixed feeder (Hempson et al., 2015) living in small groups during the dry season and aggregates in large groups during the green season (Skinner and Chimimba, 2005). We observed group sizes of 12 to 45 individuals per collared individual during field observation in the dry season. Migratory movements of springbok vary largely throughout the years and between individuals. For instance, the historical “treks” of hundreds of thousands of springbok observed in the 19th and 20th centuries (e.g., Scully, 1913; Cronwright-Schreiner and Wessels, 1931; Skinner, 1993) in southern Africa are attributed to an interplay between successive years of exceptionally high rainfall, high population growth, drought, and overgrazing, eventually causing mass movements (e.g., Roche, 2005). The movement patterns of the collared individuals varied from range residency to migration (Figure 2).
We further chose the nomadic eland, which is the largest African antelope (weight of females: 305 kg). Eland is classified as a social, non-water-reliant mixed feeder (Hempson et al., 2015) with varying group sizes. We observed group sizes of 7 to 14 individuals per collared individual during field observation in the dry season. Eland are mainly nomadic, but range residency and migratory movements occur (Hillman, 1988). The collared individuals’ movement patterns were predominantly nomadic (as defined in Teitelbaum and Mueller, 2019), with partly irruptive sequences of long-distance movements (Figure 2).
2.3 Tracking data
Individuals were collared with e-Obs GPS devices (e-Obs GmbH, Germany; springbok: Collar 1D weighing 320 g, eland and kudu: Collar Big 3D weighing 840 g or Collar Big 4D weighing 960 g). We selected individuals from different dry season groups to cover the movement of many individuals per tracking device. Details on the capturing procedure can be found in Hering et al. (2022) (a summary is provided in the Supplementary Material Section 1.1). Animal handling permits were issued by the Namibian National Commission on Research Science and Technology, certificate number RCIV00032018 (authorization numbers: 20190602 and AN20190808).
GPS localizations were taken in sequences at 15-, 7.5-, or 5-min intervals depending on the battery size of the respective collar (see Table S1). A sequence consisted of at least three consecutive GPS localizations (one-second interval), with the average of these used for subsequent analysis.
2.4 Identification and definition of fence interactions
All analyses were performed with R (version 4.2.0, R Core Team, 2022) in R-Studio (v. 2022.02.2). We used fence interaction identification and categorization as described in detail in Hering et al. (2022) (a summary is provided in the Supplementary Material Section 1.3). Note that we assigned consecutive localization to a fence interaction event when they were not more than 50 m away from a fence line.
We distinguished basic interactions into cross or non-cross events. We further classified those depending on the movement behavior and the time spent at a fence into four interaction types: (i) quick, as immediate interaction, (ii) trace (no changes in direction), (iii) back-and-forth (changes in direction, at least one relative angle larger than 120°), and (iv) stay (at minimum one event of consecutive speeds below 0.05 m/s lasting longer than 15 min, independent of directional change). We used the naming of types introduced by Xu et al. (2021).
These interaction types may relate to specific behaviors. While quick interaction indicates short fence encounters, e.g., for a straight crossing or a short feeding event in close proximity to a fence line, trace interactions display a possible search for a gap or a detour caused by the fence which blocked the direct way. Back-and-forth interactions likely relate to situations during which the animals have been trapped and changed direction in order to escape. Stay interactions could display resting or ruminating events close to a fence.
2.5 Frequency of fence interaction types
We derived counts per fence interaction type and fence type for each individual in order to derive information on how the species interacted with the different fence types [VCF, electrified, wildlife-proof fence (EF), and non-electrified, wildlife-proof fences (NEF)] and what the major forms of animal–fence interactions were. We grouped the results by species. We accounted for the different extents of the fence types by calculating the actual length of each fence type within each individual’s space use area (for details, see the Fence interaction intensity section).
2.6 Temporal dynamics of fence interactions
In order to disentangle the seasonal dynamics of wildlife–fence interactions, we used descriptive statistical methods with regard to the season, the identified events, and possible changes in foraging preferences. In the first step, we assigned seasons as 2-month periods to the identified interaction events. We used the first recorded rainfall event in 2019 (above 10 mm, measured by our local weather station) as a starting point to define the onset of the first early green season. From there on, we defined further seasons (early, mid, and late green seasons, followed by early, mid, and late dry seasons), each lasting 61 days. Subsequently, we calculated the number of events (per species, season, and interaction type) and averaged it by dividing it by the total number of collared individuals per species and season. The numbers of collared individuals per species and season ranged from two (during the last recording season) to eight (Table S2). In a second step, we derived above-ground woody biomass from Bouvet et al. (2018) (25 m resolution) for each localization and calculated the daily average of visited woody biomass per species. We related these daily averages to the respective season and the recorded rainfall events to provide information on potential changes in forage preference.
We analyzed fence interaction dynamics in relation to the “time of day” using smoothed kernel density estimates (Wickham et al., 2022). We scaled the “time of day” to times after the “start of twilight” (nautical dawn, starting when the sun reaches 12° below the horizon) using the suncalc package (Thieurmel and Elmarhraoui, 2019). We identified the “start of twilight” in relation to the geographical position of each localization. We calculated density estimates for fence interaction events (per species and fence type) based on the first localization of each event. Furthermore, we calculated density estimates for directed movements, which we defined as such if the relative angle between two movement-track segments (around a localization) was between −90° and 90° and the speed (leading to this localization) was above 0.5 m/s.
2.7 Fence interaction intensity
We compared the intensity of animal–fence interactions to individual space use. We used weekly distance traveled along fences and time spent at fences as indicators of the intensity of animal–fence interactions. These were derived from the identified fence interaction events per week and for each individual. We chose week as a suitable time interval to include at least two fence encounters on average (the minimum average of encounters per day was 0.3 for springbok). To simplify the interpretation, we calculated the percentages of weekly distances traveled along fences and times spent at fences in relation to the total distance traveled or time per week. We, then, linked this with the individual core area of used space and the fence density within that area.
As a space use metric, we calculated the utility distribution for each individual using the kernelUD function in R (Calenge and contributions from Scott Fortmann-Roe, 2021) on a 30 m × 30 m grid with the ad hoc method for smoothing parameter estimation. Subsequently, we extracted 95% isopleth for the space use area and 50% isopleth for the core area of used space. Finally, we calculated the fence density within the core area by extracting all fence lines within it and dividing their total length by the core area.
To assure comparability while linking the core area to fence interaction intensity, we focused on the period between the late dry season 2020 and 2021 and calculated all values for this time interval. For general, averaged descriptive metrics, we calculated values based on all available data to account for seasonal and individual variations.
2.8 Gap fidelity as an indicator of awareness
We tested for a correlation between the distance traveled along a fence line and the animals’ cognition of a fence gap. For the latter, we calculated gap utilization as an indicator of awareness. We based this analysis on the assumption that if the individuals are aware of gaps, these gaps are used more frequently than others.
We calculated gap utilization in two steps. First, we divided the fence area (by 50 m buffered fence line) into 100 m × 100 m squares. Then, we identified the gap used for a cross fence interaction event by intersecting the line segment at which the crossing occurred with the squares and recorded the unique identifier of that square. Adjacent crossed squares were consolidated as a single gap. The gap identifier was attributed to the respective event. Second, for each individual, we calculated the total number of crossings and related this to the number of times every single gap was crossed. We focused only on individuals with at least 10 crossings. Finally, we used the frequency a single gap was crossed as a proportion of all crossings as gap utilization.
We fitted generalized linear mixed-effects models (GLMM) on the distance an individual traveled along a fence line as the response variable with logarithmic link function and Gamma-distributed response data (Bates et al., 2022) to identify a potential relationship with an individual’s gap utilization. Since we expected general differences between the species due to their different space use and movement, we fitted a GLMM for each species. To account for differences between individuals, we included the individual as a random factor (random slope). We tested model importance by comparing it to a reduced model, containing only the fixed effect, and a null model, solely containing the random effect, via a likelihood-ratio test (anova function). As an indicator for the model quality, we used pseudo R² provided by the r.squaredGLMM function (Bartoń, 2022).
2.9 Direct fence effects on movement speed
We were especially interested in the potential direct effects of fences on the animals’ locomotion. Hence, we compared movement speeds during the identified animal–fence interaction events with those immediately before and after the respective events. In addition, we evaluated the possible effect of distance to the fence and of crossing or not crossing the fence.
We selected a time frame of 45 min to define speeds before or after a fence interaction, resulting from at least three measurements due to the maximum GPS fix rate of 15 min. We did not choose a more extended time window to exclude alternative movement behaviors as best as possible.
We fitted GLMMs (Gamma-distributed response data) per species and fence interaction type. Thus, we accounted for variance differences in speed among the species and interaction types, improving calculations of the estimates. We used speed as a response variable in a logarithmic link function (Bates et al., 2022). As fixed effects, we included a lag-speed variable with the former speed of each point to account for temporal autocorrelation and the fence interaction sub-type (cross/non-cross). We added an interaction term between distance to the closest fence and temporal group (before, during, and after). We included the event ID as a random factor (random intercept) to account for differences among single interaction events. We used speeds before and non-cross events as reference groups. We tested model importance by comparing it to a reduced model, containing only the fixed effects, and a null model, solely containing the random effect, via a likelihood-ratio test (anova function).
We used estimated marginal means (Lenth, 2022) to evaluate the relative change in speed during a crossing. For this, we set the reference group to before cross. We estimated response values for a 50-m fence distance (threshold between before and during definition, see the Identification and definition of fence interactions section) for each combination of species and interaction type.
3 Results
3.1 Frequency of fence interaction types
We identified a total of 3,582 fence interaction events. Seventy-two percent were observed at the VCF, 21.5% at the EF, and 6.5% at NEFs. Three out of eight kudu encountered the VCF (VCF within space use area of three individuals), six encountered the EF (EF within space use area of five), and two were found at a NEF (NEF within space use area of one individual). Nine out of 10 collared springbok encountered the VCF (VCF within space use area of nine individuals), seven encountered the EF (EF within space use area of nine individuals), and one encountered a NEF (NEF within space use area of four individuals). All of the seven eland encountered the VCF as well as the EF (VCF and EF within all individuals’ space use area), while for five of them, interactions with a NEF were identified (NEF within space use area of six individuals). The lowest number of total encounters was recorded for a kudu (ID: 7296), with six encounters during 559 days of observation. The highest number of total encounters was recorded for another kudu (ID: 7295), with 436 encounters during 526 days of observation. Average daily encounters ranged from 0.3 (95% CI: 0.1, 0.5) for kudu and 0.3 (95% CI: 0.2, 0.4) for springbok up to 0.5 (95% CI: 0.4, 0.6) for eland.
At the VCF, most fence crossings of springbok and eland were quick (springbok: 29% of all 1,050 fence encounters, eland: 26% of all 1,445 fence encounters), while for kudu, trace cross was observed the most (12% of all 1,087 encounters by kudu). Most fence interactions of the sub-type non-cross were quick for springbok (15%) and stay for eland (12%) at the VCF, while for kudu, stay non-cross at the EF occurred mostly (23%). Further frequent fence interactions were of type trace for all species (Figure 3).
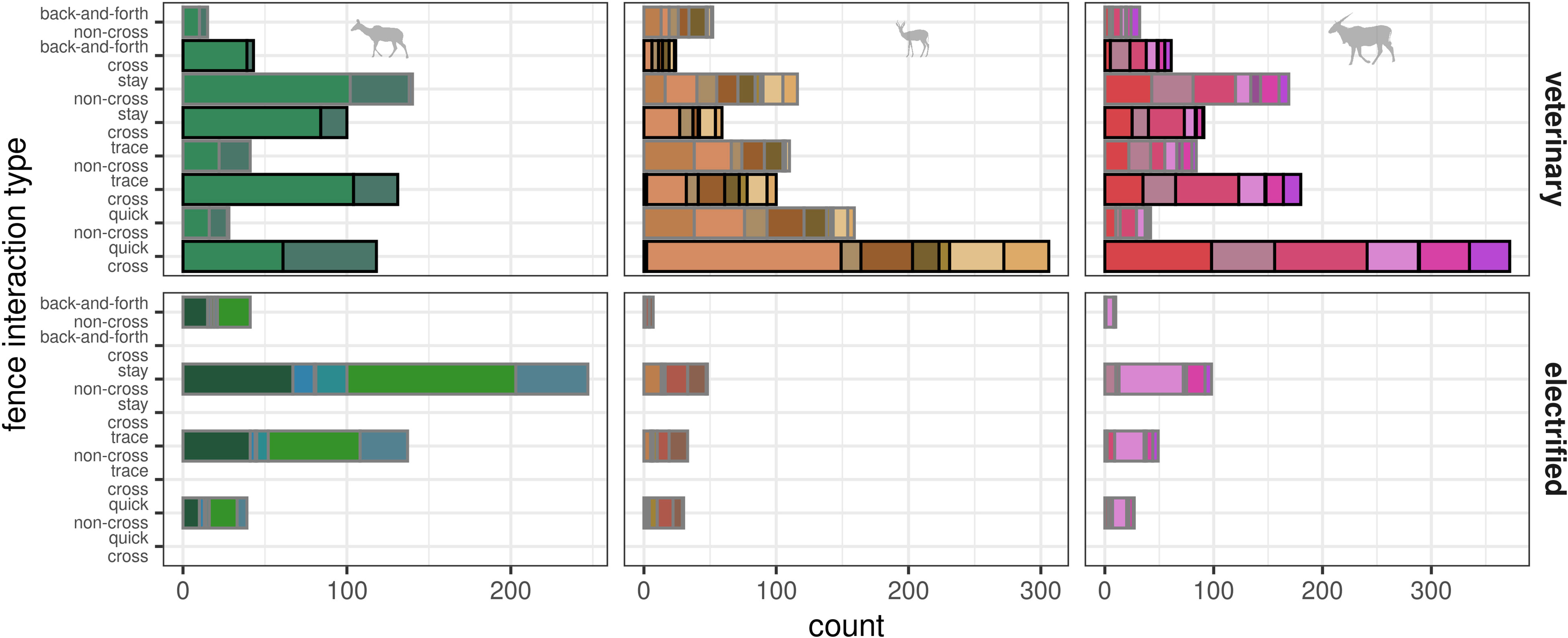
Figure 3 Total numbers of fence encounters grouped row-wise by fence type [upper row: veterinary cordon fence (VCF); lower row: electrified fence (EF)]. Number of encounters (x-axis) shown per fence interaction type (y-axis) with individual numbers indicated by color. Outer lines of bars indicate crossing success (black—cross; gray—non-cross). Species-wise numbers shown per column (see species symbol).
3.2 Temporal dynamics of fence interactions
Most fence interactions occurred during the day between dawn and dusk (kudu: 75%, springbok: 92%, eland: 69%). At the VCF, interactions occurred mainly in the morning (Figure 4), with peaks differing between species (kudu: 2 to 3 h after “start of twilight”, springbok: 2.5 to 3.5 h, eland: 1.5 to 2.5 h). We observed a secondary peak for all species during the evening (Figure 4). The temporal patterns of fence interactions at the VCF were similar to overall patterns of directed movements (Figure 4).

Figure 4 Temporal peaks in numbers of fence interactions for 24 h starting at twilight. Shown are density distributions of observed fence interactions (violet lines) and of directed movements (gray dotted lines, speeds larger than 0.5 m/s and relative angles less than 90°) per species (columns of panels, see symbol). Density (y-axis) for times after “start of twilight” (x-axis, sun 12° below horizon). Vertical lines indicate the time of sunrise (left line) and the times of sunset (earliest sunset indicated by dotted line, latest sunset shown by right line). Statistical population is either species-wise fence encounters (violet density estimates) or all directed GPS localizations (gray density estimates, 8% of all kudu localizations, 13% of all springbok localizations, and 18% of all eland localizations).
All species showed clear seasonal peaks in the number of fence interactions during the transition from dry to green season (Figure 5). At this time, the average above-ground woody biomass of visited locations increased for all species, indicating a temporal shift in forage resources (for details see Supplementary Material Section 2.1). The lowest numbers of fence interaction for eland and kudu were recorded during the transition from green to dry season and for springbok during the middle of the dry season.
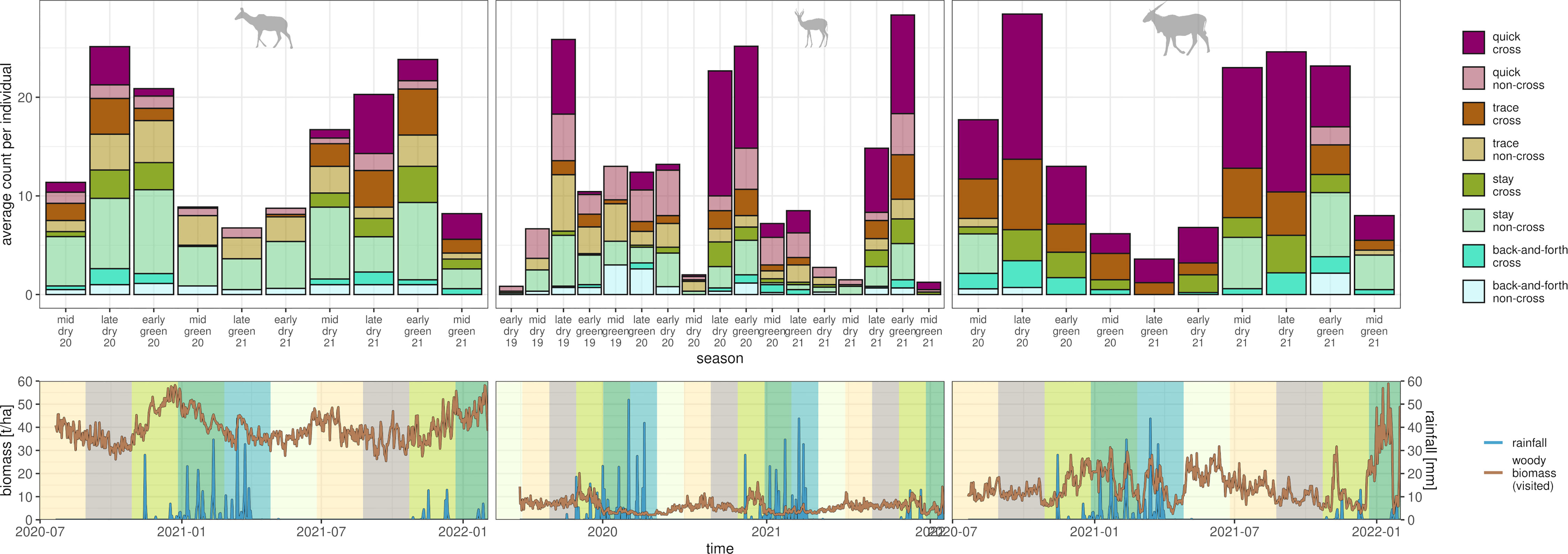
Figure 5 Temporal peaks in numbers of fence interactions along seasons and in relation to environmental conditions. Upper row of panels shows species-wise (panels, see icon) and interaction-type specific (see legend) counts of identified interactions per season (60-day periods). Counts were averaged by the number of observed individuals per season. Lower row of panels shows daily precipitation (blue lines) and daily average of above-ground woody biomass of the locations that were visited by all individuals of a species (brown line). Low values of woody biomass indicate open habitats, high values indicate shrub and woodlands. Colored rectangles in background depict the corresponding season used for the analysis in the upper row of panels. Note that for the last season (mid green 21), tracking data were available for a few individuals only.
3.3 Fence interaction intensity
The time spent and distances traveled at fences did not yield relationship to the core area’s size (Figure 6), but they differed among species. Species-specific maxima of the average weekly proportion of time and distance at fence lines were identified for individuals with an intermediate space use area between 232 km² and 290 km² (Table 1). The longest traveled distance along fences per week was recorded for an eland (32 km out of 86 km total distance within that week, Figure 7), followed by a springbok (12.8 km out of 102.0 km total distance) and a kudu (11.9 km out of 43.3 km total distance). The longest time spent at fences during a week was recorded for another eland (48.4 h, Figure 7), followed by a kudu (40.1 h) and a springbok (20.3 h). Species-specific averages of weekly traveled distances along fence lines ranged from 0.7 to 2.6 km/week (Table 2 with further details).
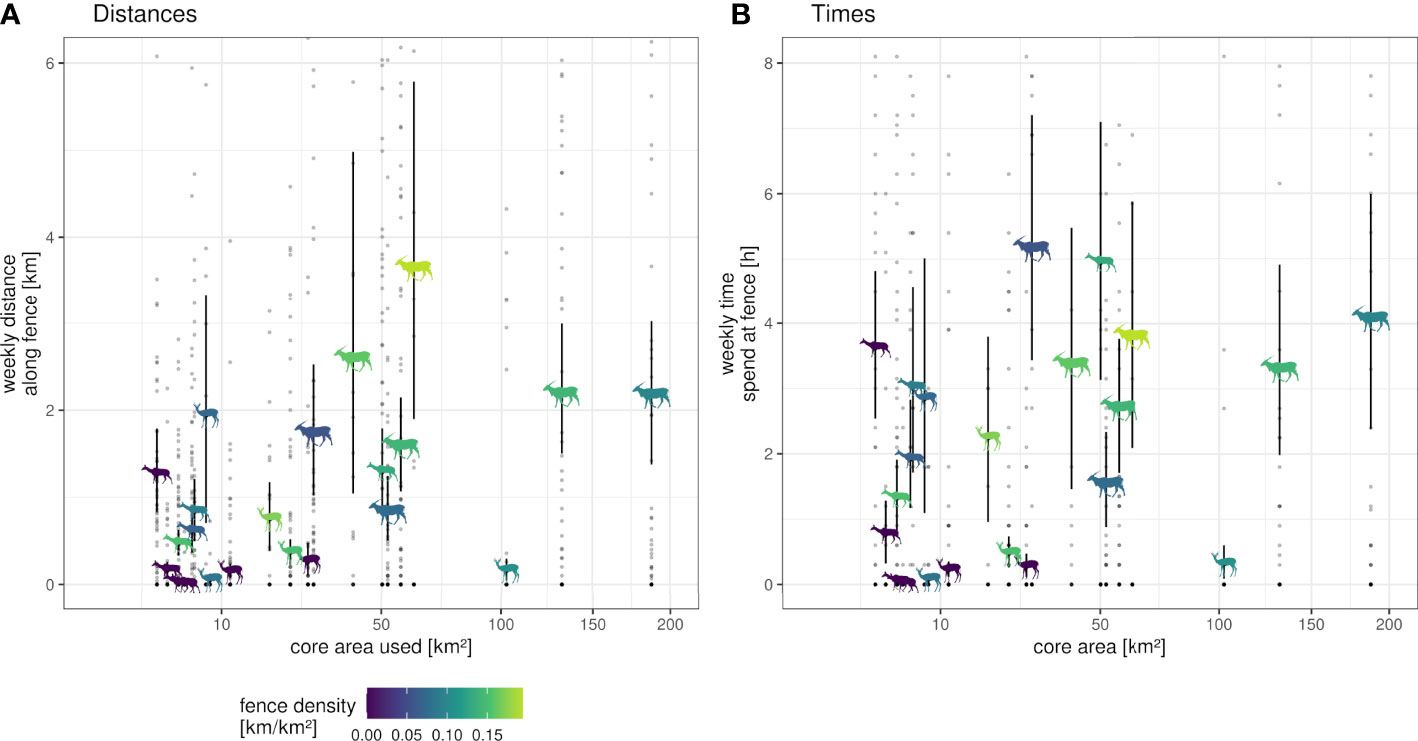
Figure 6 Weekly distances (A) and times (B) individuals spent at fence lines projected on the core area they used from end of dry season 2020 to end of dry season 2021. Shown are mean values (icon position) on the y-axis separated by individual and species with lower (0.025) and upper (0.975) confidence intervals from bootstrapping (1,000 iterations) shown by black, vertical lines. Fence density within each individuals’ core area indicated by color (see legend). Individuals are ordered by their used core area (x-axis, area based on 50% of kernel density estimates with ad hoc method for smoothing parameter calculation). Single points depict values of single weeks of observation. Note: the y-axis is limited to upper CI; data are not. The x-axis is square root-transformed; data are not.
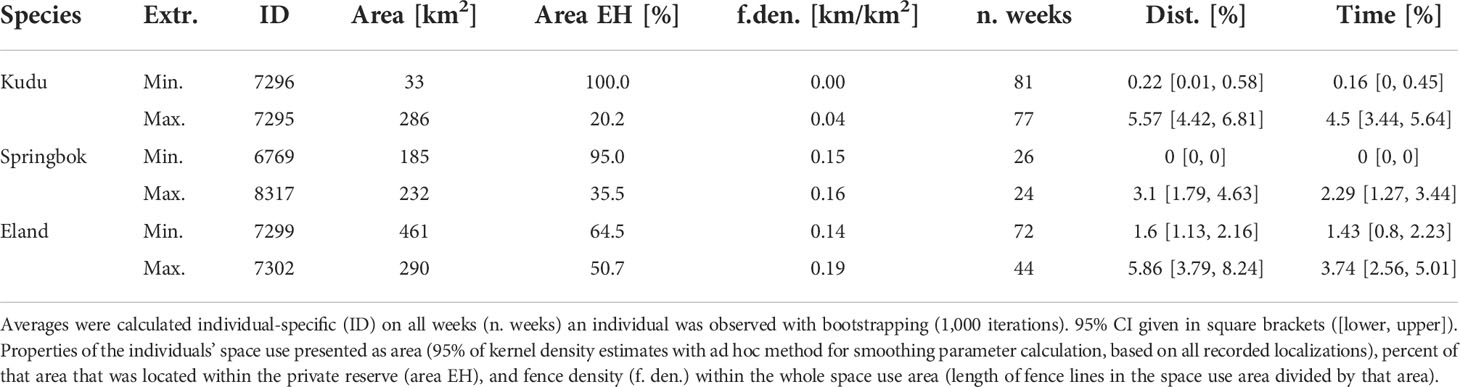
Table 1 Species-specific extrema (extr. with minimum—min. and maximum—max.) of average weekly percentage of distance (dist.) and time at fence lines.
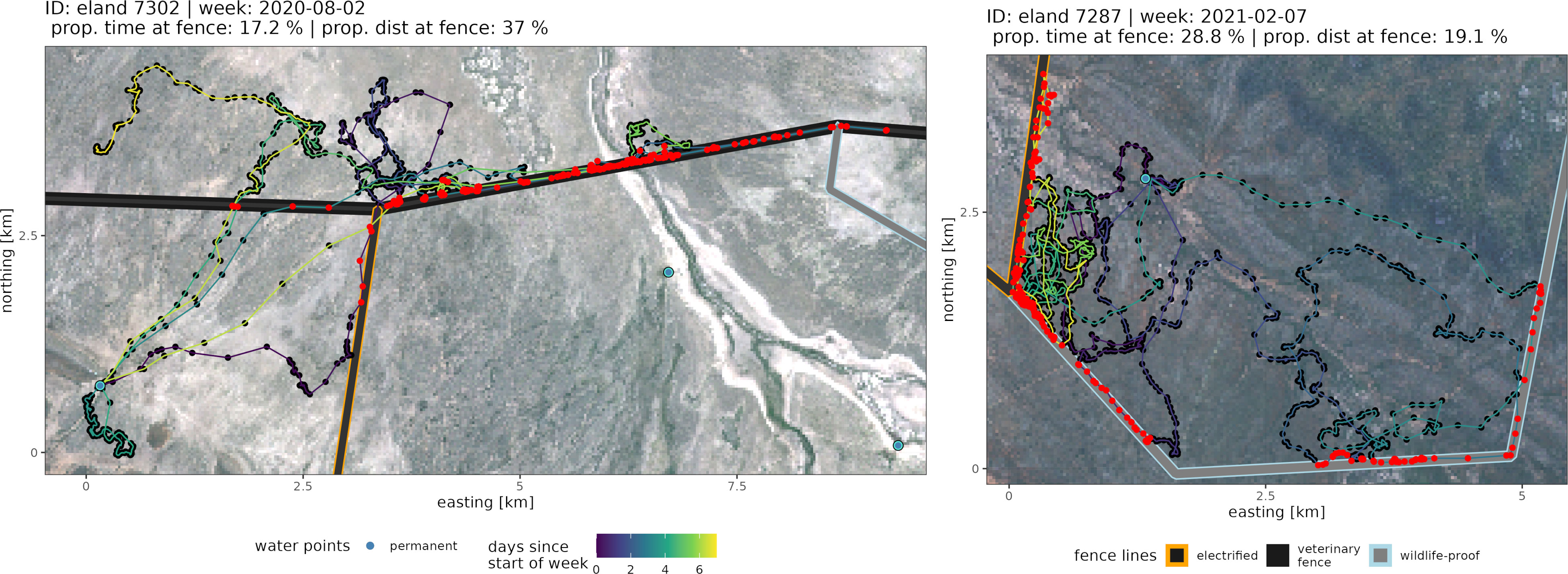
Figure 7 Example weekly movement paths of weeks with either very long distances moved along fence lines (left) or times spent at fence lines (right). Red points depict localizations related to a fence interaction event. Further details are shown in panel titles or within the legends. Background Sentinel 2 (Bands 3,4,5) image, March 2020 (contains modified Copernicus Sentinel data [2020]).

Table 2 Species-specific averages of weekly distances (mean dist.) as absolute values [km/week] and percentage of total weekly distance [%] and averages of percentages of time (mean time) at fence lines.
3.4 Gap fidelity as an indicator of awareness
Traveled distances along fence lines decreased with gap utilization, which may serve as a proxy for the awareness of fence gap position (Figure 8). The GLMM with the individual as a random factor was superior for eland only. For springbok, the GLM (without random intercept) was the best parsimonious model. Both models identified the effect of gap utilization on the distances traveled along fence lines (p < 0.001; effect size for eland: 0.02, for springbok: 0.23; full model results Table S3 and Table S4). No model differed from the null model for kudu.
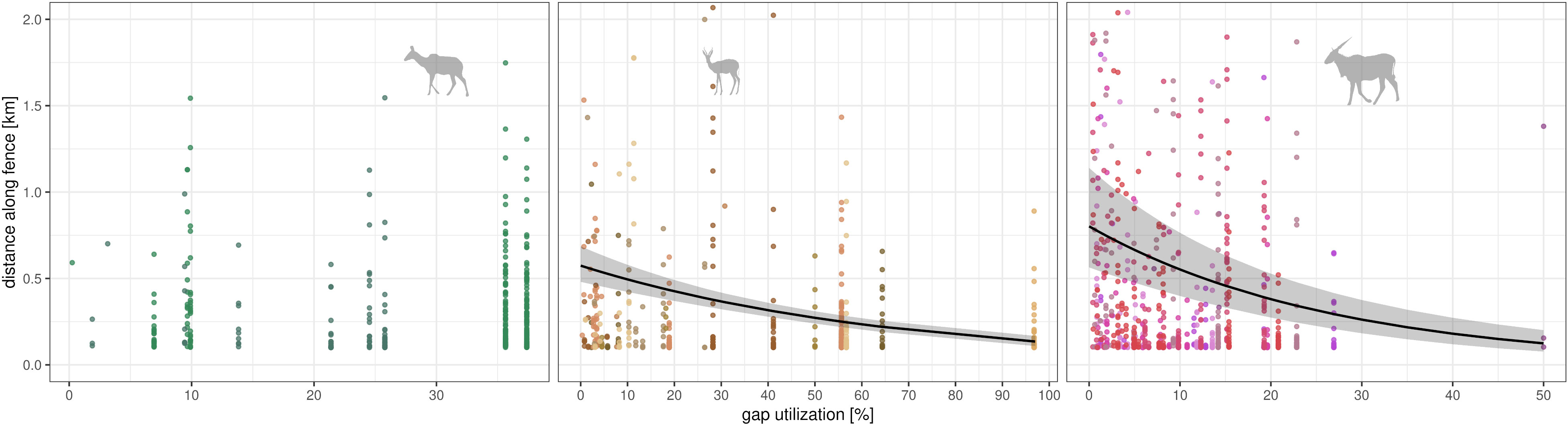
Figure 8 Relation between distance moved along fence line and gap utilization. Gap utilization (x-axis, limited to data) as individual proportion a single gap was used in comparison to the use of all gaps. Single points show distances along fence of each crossing event (y-axis). Species-wise (in panels, see symbol) prediction from GLMM (black lines, Gamma distribution with logarithmic link function) with confidence intervals (gray areas) for fixed effect.
3.5 Direct fence effects on movement speed
For all of the three species, movement speed increased with cross fence interactions compared to non-cross interaction of the same interaction type (Table 3, details in Tables S5–S16). We frequently recorded effects on the movement speeds after the actual fence interaction. In relation to speeds before a crossing interaction, the strongest positive effects were identified for all species during quick cross events (Table 3). The strongest negative effects were identified for eland and springbok during stay cross events and for kudu after quick cross events. Generally, movement speed decreased the closer the animals moved towards a fence (e.g., Figure 9, full model results in Supplementary Material). This effect weakened after the fence interaction, leading to only slight increases in speed when the animals moved away from a fence (e.g., Figure 9).
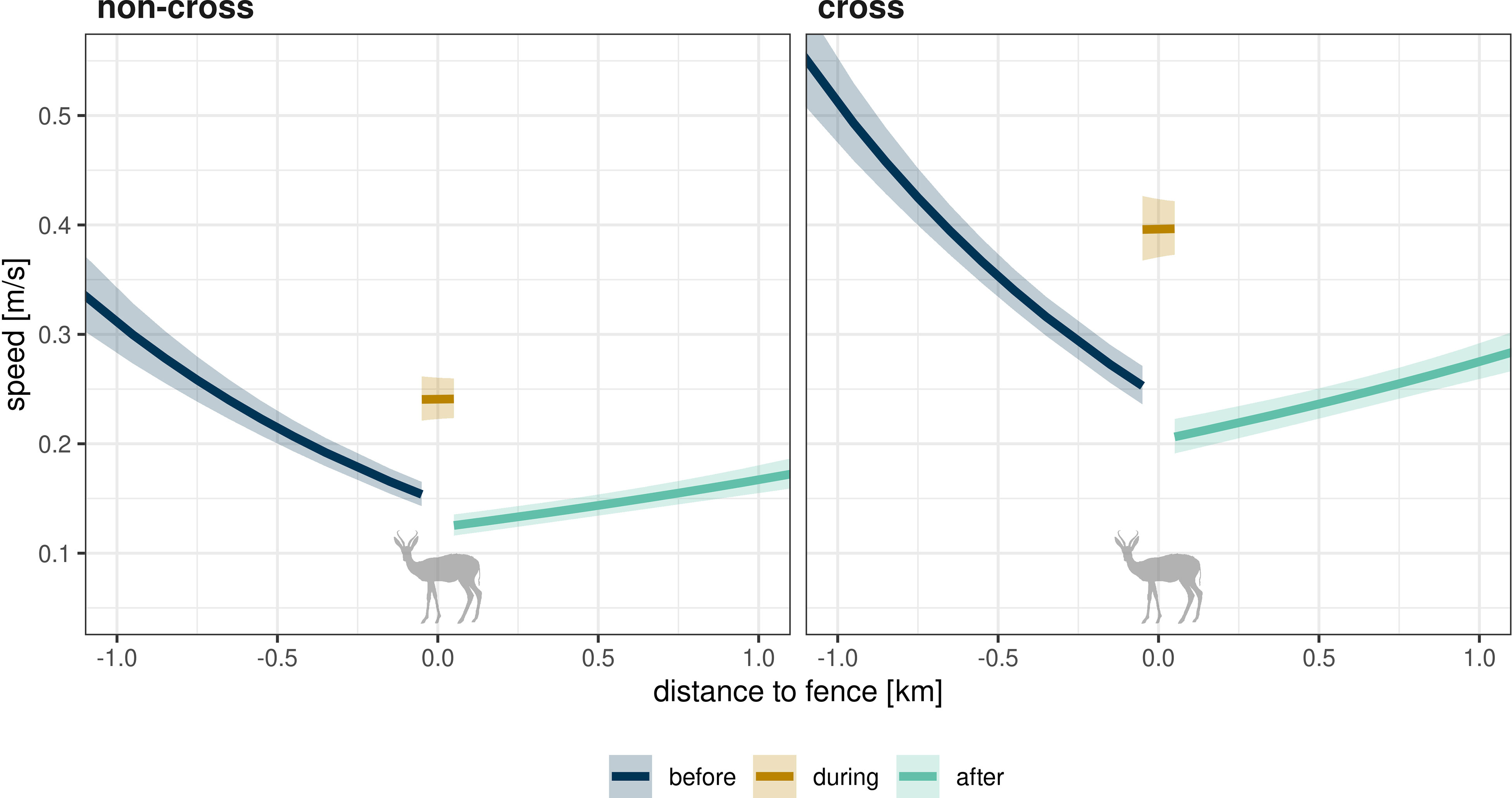
Figure 9 With fence encounters associated change in movement speed (y-axis) along the distance to a fence (x-axis). Shown is an example prediction for springbok and a quick fence interaction (lag-speed variable kept constant, random effects on population level). Colors depict the prediction for the temporal group (before, during, and after the interaction; see legend). Panels refer to the sub-type of fence interaction (left—non-cross interaction, right—cross interaction). For this presentation, x-values before the interaction were multiplied by −1, so that the temporal course of the event starts on the left of the axis and ends on the right.
During quick cross events, all species increased speed relative to the speed before the crossing (Figure 10). Compared to speeds before the crossing, speeds during the interaction were 1.4 times greater for kudu, 1.5 times greater for eland, and 1.6 times greater for springbok. After the crossing, speed was often lower than before the crossing. Speeds after the fence interaction were about 15% lower for all species.
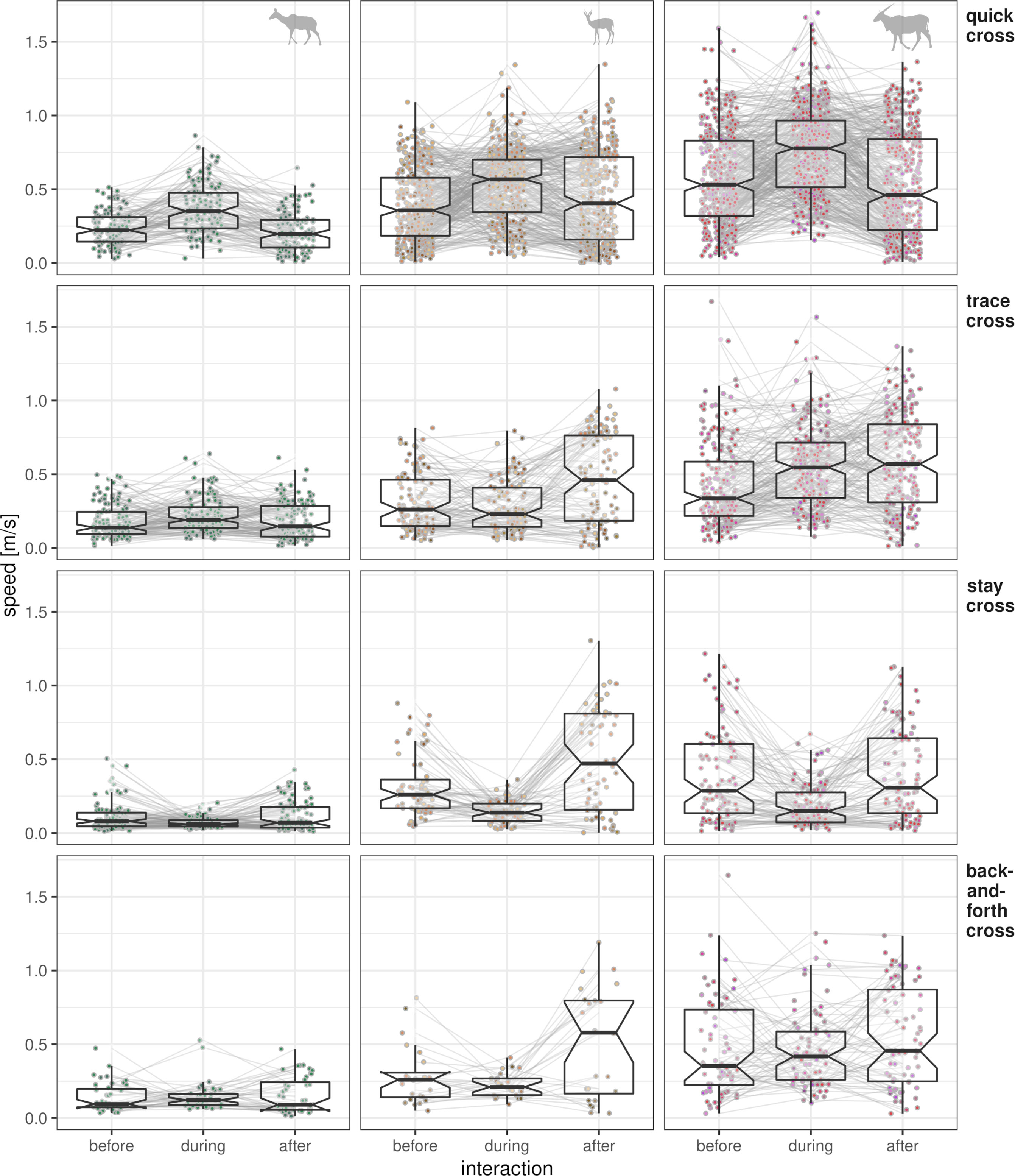
Figure 10 Average speeds of crossing fence interactions. Speeds are averaged per interaction event and temporal group (all speeds 45 min before, 45 min after and all speeds during the interaction) with box–whisker plots (no outliers shown, confidence region indicated by notches). Speeds are on the y-axis and temporal groups are on the x-axis. Single events are connected by a line. Columns of panels refer to species (see symbol in the first row); rows of panels refer to fence interaction type (see row titles on the right).
Kudu and eland speeds increased similarly during trace cross events (speed 1.4 times greater), while springbok speed increased slower (1.3 times greater). While no clear effects of the temporal group “after” on speeds were identified for kudu and eland, springbok increased speed after crossing (1.2 times greater than before).
When staying at the VCF before crossing it (stay cross), movement speeds were low by definition of this interaction type. Speeds during the interaction were significantly less for eland and springbok and only slightly lower for kudu. The temporal group “after” had no effect on speed for all species.
During back-and-forth cross, all species increased speed. For kudu and springbok, fewer than 50 single events were identified (Figure 10). Springbok increased speeds after crossing the fence—again reaching greater speeds than before.
4 Discussion
The movement data of kudu, springbok, and eland indicate that animals regularly encountered fences in the study area and frequently used fence gaps and that this affected the ungulates’ locomotion directly and, in parts, remarkably. Our dataset, consisting of 2.1 million GPS positions from 25 individuals and representing movements over two and a half years, enabled us to identify more than 2,500 km of movement tracks along fence lines. More than half of this distance was traveled by nomadic eland (7 individuals), while partial migratory springbok (10 individuals) traveled a third, and sedentary kudu (8 individuals) a fifth of it. This finding is corroborated by other studies showing similar records of animal–fence interactions (on 74% of all tracking days, Laguna et al., 2022) or generally frequent observations of interactions across taxa (e.g., Pirie et al., 2017; Wilkinson et al., 2021). These insights demonstrate that such large numbers are common and not an exception. Furthermore, our findings illustrate that the animals were restricted from reaching valuable foraging grounds since they spent significant parts of their movement capacities traveling along fences. The animals spent substantial amounts of time at fences and frequently increased their movement speed when crossing the VCF at gaps. These findings demonstrate that wildlife-proof fences, despite being intermittently passable, directly affect the locomotion of ungulates and may ultimately cause energy loss. This impact is most likely accompanied by increased stress levels (Gentsch et al., 2018) and negative fitness consequences (e.g., Sawyer et al., 2013; Segar and Keane, 2020). Nevertheless, our results also suggest that the awareness of specific gaps reduces the intensity of fence interaction, and hence fence gaps might mitigate the negative effects of fences. Although our results are limited in their spatial extent and the spectrum of ungulate species, the number of observations and the level of detail, in combination with findings of other studies, provide insights for future fence-related research and future fence management planning.
4.1 Frequency of fence interaction types
Fence interactions by springbok and eland were dominantly quick, which aligns well with other studies analyzing animal–fence interactions based on GPS tracking (Xu et al., 2021; Laguna et al., 2022). In contrast, kudu mostly stayed longer at fences either during stay or trace interactions. The number of trace events identified from our high-frequency GPS resolution (5–15 min) was greater than in studies using hourly localizations. We explain this difference by the duration of the tracing along a fence. We observed that trace cross interactions lasted 22 min on average (95% CI: 20, 24) for all three antelope species. In our study, a GPS localization frequency coarser than 15 min would have resulted in a different classification, or we would have missed the interaction altogether. Notably, stay cross fence interactions lasted 159 min (average with 95% CI: 143, 176). During such interactions, the animals were stationary at fences for 68 min (average with 95% CI: 57, 79). This dependency demonstrates not only the potential but also the limitations of such analysis regarding the temporal resolution of GPS tracking data (e.g., Bischof et al., 2019; Nathan et al., 2022). For instance, classifying trace interactions by hourly localizations may overlook that animals often perform halts at fences and not purely trace the fence line. Our results highlight that a systematic review of animal–fence interaction type classification in terms of the tracking data’s temporal resolution, as well as a consistent and appropriate nomenclature, is needed to improve the comparability of studies.
Many studies show that linear, anthropogenic landscape features, such as roads and fences, affect the movements of terrestrial animals (e.g., Jones et al., 2022) and negatively influence space use (e.g., Robb et al., 2022). Individual movement strategies, the spatial density of such features, and their permeability determine the severity of the features’ effects on the animals. This general relation between landscape configuration and movement strategy is well reflected in our counts of animal–fence interactions. While most of the migratory springbok and all of the nomadic eland frequently crossed the permeable VCF, only a minority of sedentary kudu did so. Home ranges of these kudu individuals contained the VCF and its permeability enabled the home range formation around it, leading to frequent interactions. We found comparably large interaction numbers for many kudu with the non-permeable electrified fence (EF). The EF likely limited those individuals’ home range formations. In contrast, springbok and eland rarely interacted with the EF, although the fence intersected with their used space. Despite a possible area-specific avoidance caused by practices such as hunting or poaching outside the conservation areas, we see two main explanations to cause this finding. First, the general movement behavior of springbok and eland likely enabled them to discard areas beyond the EF and rather select areas around and beyond the permeable VCF. Indeed, e.g., Robb et al. (2022) showed that migratory pronghorn strongly avoids non-permeable features, whereas kudu’s range residency and feeding habits (De Garine-Wichatitsky et al., 2004) likely kept the individuals in proximity to the EF. Second, vegetation beyond the EF is dominated by woody species and therefore attractive for browsing kudu, while vegetation beyond the VCF is open and grass dominated and conclusively attractive for mixed-feeding (during green season grazing) springbok and eland (e.g., Hillman, 1988; Skinner, 1993). This habitat configuration might have led kudu, whose home ranges were close to the EF, more towards this fence during foraging movements.
Overall, our classification of animal–fence interaction types (see Xu et al., 2021; Laguna et al., 2022) allowed us to identify differences in fence interaction between species with implications for fence management and fence gap planning (cf. Hering et al., 2022). All species traced fence lines, indicating that they would be capable of finding new fence gaps. For sedentary kudu, which form smaller and constant home ranges, such gaps might be placed at locations connecting nearby wood- or shrublands. For springbok and eland, which travel longer distances, gaps connecting productive shrub- and grasslands would be beneficial. We found that the latter two species are likely to integrate gaps into their movement routines, which was expressed by many quick crossings. Also, other studies find fence gaps to be used relatively fast by many species (e.g., Dupuis-Desormeaux et al., 2018).
4.2 Temporal dynamics of fence interactions
We found clear seasonal trends in the number of fence interactions for all species. Peaks occurred during times of seasonal transitions, possibly attributed to changes in resource availability (forage and water) in combination with the spatial arrangement of the fences. During the colder dry season with limited forage availability, access to water is crucial to minimize the consequences of adaptations to dehydration, such as increased digestion periods and reduced metabolic rates (e.g., Skinner and Louw, 1996; Cain et al., 2006). In our study region, the private nature reserve south of the VCF maintains artificial water points at a high spatial density compared to the ENP, which are the only surface water sources in the dry season. During the beginning of the warm green season, natural surface water is not widely available yet, and during calving and lactation, access to productive foraging grounds is essential. At that time (late dry season–early green season), the first flushes of woody plants in ENP (north of the VCF) form the first viable and reliable source of nutrition (Archibald and Scholes, 2007; Ibrahim et al., 2021). Simultaneously, individuals still needed to drink at artificial water points on the private land. They often commuted between vital forage patches north of the VCF and the water points south of it. This spatial segregation most likely caused the observed seasonal peaks in animal–fence interactions (see Hering et al., 2022). In this manner, the VCF likely impedes access to valuable resources for many individuals since it runs across (west to east) a landscape-wide rainfall and soil moisture gradient (southwest to northeast). The latter causes areas northeastwards to be generally more productive during the green season, but the VCF often acts as a barrier (see Tinley, 1971; Naidoo et al., 2022). Similar seasonal dynamics of animal–fence interactions were found by, e.g., Visscher et al. (2016); Wilkinson et al. (2021), and Xu et al. (2021), which demonstrates the importance of the spatial arrangement of fences related to movement strategies (Jones et al., 2019).
“Time of day” of the animal–fence interactions depended on species-specific activity patterns (e.g., Visscher et al., 2016). For instance, at Lake Nakuru National Park, Kenya, Wilkinson et al. (2021) found that ungulates, which exhibit main activity times during the day (e.g., Owen-Smith and Goodall, 2014), were more likely to cross the fence during daylight. The same holds for ungulates crossing roads (e.g., Prokopenko et al., 2017). We also found the majority of fence interactions to occur during daylight (1.5–3.5 h after sunrise). This observation is likely related to the natural movement times of the animals, possibly related to the combination of light to detect predators and cooler temperatures compared to the afternoons. However, this also indicates that fences impede ungulates at specific times of the day and when they move towards a certain resource (e.g., Owen-Smith et al., 2010).
Our findings on the temporal dynamics of animal–fence interactions provide important implications for future fence and fence gap management. Suppose it is intended to install new fence gaps. In that case, gaps should be in place before the growing season starts since animals increase movements during seasonal transitions, likely enabling them to identify passages faster. Potential wildlife management practices such as population counts or health control, which could be efficiently undertaken at fence gaps (e.g., Hering et al., 2022), should focus on these times. Costs of elaborated practices could be reduced if performed during the early hours of the day. Fence control practices could also focus on these seasonal and daily times to be more efficient.
4.3 Fence interaction intensity and gap utilization
We assume that we found no correlation between individual space use (area) and the intensity of their fence interaction because the fence density within our study area was relatively low (within the area covering all localizations: 0.1 km/km²). High fence densities can severely affect habitat quality, as seen, e.g., for pronghorn by narrowing their winter habitats (10% decrease per 1 km/km², Robb et al., 2022) or for elephants by increasing their space use intensity (Vanak et al., 2010; Osipova et al., 2018). Fence densities in some studies exceeded ours by up to an order of magnitude (fence density in grasslands of 489 Alberta, USA: 1.1 km/km², Jones et al., 2019) and corroborate the trend of decreased wildlife mobility in human-modified landscapes (Tucker et al., 2018). However, we identified a general relation of movement strategies to the intensity of fence interaction. Springbok seemed less affected by fences, despite their known tendency to migrate (Skinner, 1993). Most individuals’ temporal foraging grounds were either a long way from a fence or located around a permeable section of the VCF with persistent gaps (see the next paragraph). Fence interaction of sedentary kudu was at an intermediate level resulting from the spatial composition of the constant and narrow home ranges. This composition ranged from home ranges without fences over some formed around the permeable VCF to one where the individual was fenced in on a small farm. Eland interacted with fences most intensively (e.g., see extreme examples in Figure 7 or Mbaiwa and Mbaiwa, 2006) because they seldom used areas for long periods and traveled long distances between them. Our findings suggest that the key factors for understanding and predicting the intensity of animal–fence interaction are the spatial composition of the habitat (e.g., Cushman et al., 2010; Sawyer et al., 2013; Jones et al., 2019), the movement strategies on individual and species level (e.g., Berg et al., 2019; Xu et al., 2021), and the seasonality (e.g., Wilkinson et al., 2021). The average distances traveled along fences and times spent at fences were below 5%. Although this might appear low, the associated reduction of locomotion capacity and the time lost for feeding could be detrimental to individual fitness, especially in times of drought, which will increase in the future (Iturbide et al., 2020).
We could show that traveled distances along the VCF decreased with increasing gap utilization (measured as the proportion a single gap was used relative to all used gaps). This relationship demonstrates that persistent gaps in wildlife-proof fences could reduce possible negative effects. From a short-term perspective, immediate reductions in distances traveled along fences will cause interactions to be relatively short, thus reducing risks of entanglements (e.g., Mbaiwa and Mbaiwa, 2006) or lowering energy waste since individuals can incorporate such gaps into their daily routines (e.g., Dupuis-Desormeaux et al., 2018; Jones et al., 2020). From our results, we conclude that this is especially important for highly mobile species since they are affected most by fences (e.g., Nandintsetseg et al., 2019). Gaps, persistent over more extended periods, might even reduce the risk of drought-linked population collapses (e.g., Spinage, 1992; Roche, 2008) or local overuse of resources that could lead to degradation of the vegetation (e.g., Vanak et al., 2010; Pirie et al., 2017). Herds would be less hindered while undertaking migratory movements to cope with the patchy and erratic availability of forage and water resources (e.g., Bartlam-Brooks et al., 2011). Many options for permeable or selectively permeable fence gap designs (e.g., Weise et al., 2011; Gates et al., 2012; Dupuis-Desormeaux et al., 2018) and semi-automated surveillance (e.g., Janzen et al., 2017) exist already; however, establishing persistent and managed gaps requires an adjustment of conservation aims and legal regulations to promote large-scale ungulate movements (e.g., Hoare, 1992; McInturff et al., 2020; Kauffman et al., 2021a). Nevertheless, ungulates performing migratory movements deal with semi- and permeable fences (Sawyer et al., 2013), although the benefits of long-distance movement might be reduced (Monteith et al., 2018). Persistent and managed gaps might be the first step toward successfully conserving ungulate migrations (Kauffman et al., 2021a; Hering et al., 2022).
4.4 Direct fence effects on movement speed
All species changed movement speeds when interacting with a fence. For all situations, we found movement speeds to decrease the closer to a fence the animals were. These decreases may relate to raised vigilance behavior for detecting predators near a fence. Indeed, ungulates adjust movement rates to predator activity (e.g., Gaynor et al., 2019; Prugh et al., 2019), and many African predators are known to include fences in their hunting strategies (e.g., van Dyk and Slotow, 2003). Antelopes were faster when crossing the VCF during the fence interaction types quick and trace. This increase could be attributed to the crossing strategy itself, which may reflect a natural flight reaction (e.g., Prokopenko et al., 2017; Lewis et al., 2021). Interestingly, a similar increase in movement speed (our study: 0.1 to 0.2 m/s) was observed for wolves concerning fence encounters [Laskin et al. (2020): a plus of 0.1 m/s]. Migratory Cervus canadensis (elk), which are more comparable to our African antelopes, showed changes in movement speed depending on the fence design (Laskin et al., 2020), and these changes fall into the same range as ours. Furthermore, all species’ speed decreased after crossing and slightly increased again when moving away from the fence. This initial reduction might be linked to a certain recovery time needed after the crossing and highlights the effects of fence interactions on the ungulates’ energy expenditure (Hering et al., 2022).
That crossing a fence was associated with generally higher speeds aligns with the findings of Prokopenko et al. (2017), who showed that elk avoided road crossing less when they were faster. Possibly, crossing a linear structure led the animals to a specific resource, and feeding bouts only increased after reaching the resource. Consequently, more time was invested in actual locomotion, leading to higher speeds before reaching the resource (e.g., Owen-Smith and Goodall, 2014). In the case of non-cross encounters, a crossing might not have been intended, and the animals performed more feeding bouts already, leading to lower speeds. Nevertheless, in both cases, the increased speed during the interaction suggests an apparent alteration of the ungulates’ movement behavior, which has been demonstrated in other studies as well (e.g., You et al., 2013; Prokopenko et al., 2017; Jones et al., 2022). Fence interactions of the type stay included sequences of low speeds by definition; hence, we found decreased speeds at fences during such events in many cases. Associated behaviors likely are resting, ruminating, or standing alert (Owen-Smith et al., 2010). Wilkinson et al. (2021) also found such events common at fences. We could not detect clear differences when graphically comparing the time of the day of stay events at or not at fences, indicating that fences did not directly initiate the halt. Still, the duration of these halts tended to be shorter at fences, indicating a negative effect of fences on these behaviors (see Supplementary Material Section 2.4). Notably, fence-related alterations of behavior, e.g., reduced feeding and resting times due to raised walking times, imply negative effects on energy budgets, possibly reducing individual fitness. This alteration can scale up to changes in movement strategies of wildlife populations, eliminating the advances of migratory movements and, especially under changing environmental conditions, leading to population collapses (e.g., Van Moorter et al., 2020).
5 Conclusion and perspectives
In the Anthropocene, accompanied by a growing human impact on ecosystems (Ellis et al., 2021), migratory movements of ungulates need to be restored and protected to conserve the ecosystem functions they provide (Kauffman et al., 2021a). However, this conservation relies on access to vast areas endangered by barriers such as fences. Consequently, measures to mitigate the negative effects of fences on ungulates are urgently needed for future nature conservation planning.
Here, we provide insights from a natural experimental setup wherein elephants regularly breached a wildlife-proof fence, and three GPS-collared African ungulate species regularly used these gaps. While fences directly altered the movement behavior of the antelopes, the fence gaps enabled them to track resources over vast areas. Furthermore, our results suggest that fence interaction intensity decreases if gaps are incorporated into individual movement routines. For possible future fence gap planning and wildlife management practices, we outline that considering species-specific movement characteristics and daily and seasonal dynamics will be crucial (see also Burkholder et al., 2018; Dupuis-Desormeaux et al., 2018; Segar and Keane, 2020). Although we found fence gaps to affect the movement behavior of the ungulates, we see them as a possible compromise that enables long-distance movements, allows for wildlife control measures, and, simultaneously, grants the immediate reconstruction of an impermeable barrier if needed. We emphasize that the persistence of passable fence sections is crucial to reduce possible negative effects of fences on the animals (e.g., Naidoo et al., 2022), the habitat itself (e.g., Vanak et al., 2010; Pirie et al., 2017) and, consequently, the whole ecosystem (Kauffman et al., 2021a), especially regarding the increase of (wildlife-proof) border fences (e.g., Linnell et al., 2016; Safner et al., 2021).
In order to continue the disentanglement of the complexity of animal–fence interactions, we see a high potential for studies using accelerometer data to identify specific behaviors at fences and their possible alterations. Our natural experimental setup further suggests that animal movement modeling studies (e.g., Cushman et al., 2010), which evaluate best practices for fence arrangements, e.g., along and not across important environmental gradients, could help minimize the fences’ negative effects on animals. This minimization is particularly pertinent in arid environments since moving far distances is obligatory in landscapes of spatiotemporal scattered resources (e.g., Skinner, 1993; Abrahms et al., 2021).
Data availability statement
The raw data supporting the conclusions of this article will be made available by the authors on reasonable request.
Ethics statement
This study was reviewed and approved by Namibian National Commission On Research Science and Technology.
Author contributions
RH, MH, SK-S, and NB contributed to conception and design of the study. RH organized the database. RH and JS performed the statistical analysis. RH wrote the first draft of the manuscript. JS, MH, NB, and SK-S wrote sections of the manuscript. All authors contributed to manuscript revision, read, and approved the submitted version.
Funding
This work was part of the ORYCS project within the SPACES II program, supported by the German Federal Ministry of Education and Research [grant number FKZ 01LL1804A]. The publication of this work was funded by the Deutsche Forschungsgemeinschaft (DFG, German Research Foundation)—project number 491466077.
Acknowledgments
We thank Andre Nel, the team of Etosha Heights Private Reserve, and Dr. Mark Jago for full support during field research. We thank Dr. Philipp Meyer, Dr. Taylor Smith, Dr. Manuel Roeleke, and Dr. Jennifer Pohle for scientific advise and Dr. Alexios Synodinos for valuable comments on the manuscript. We acknowledge the support of the Ministry of Environment, Tourism and Forestry, Namibia and the Namibian National Commission On Research Science and Technology, who permitted this research (certificate number RCIV00032018 with authorization numbers 20190602 and 20190808). Further, we thank the reviewers of this publication for their valuable work which helped to improve the overall quality.
Conflict of interest
The authors declare that the research was conducted in the absence of any commercial or financial relationships that could be construed as a potential conflict of interest.
Publisher’s note
All claims expressed in this article are solely those of the authors and do not necessarily represent those of their affiliated organizations, or those of the publisher, the editors and the reviewers. Any product that may be evaluated in this article, or claim that may be made by its manufacturer, is not guaranteed or endorsed by the publisher.
Supplementary material
The Supplementary Material for this article can be found online at: https://www.frontiersin.org/articles/10.3389/fcosc.2022.959423/full#supplementary-material
References
Abrahms B., Aikens E. O., Armstrong J. B., Deacy W. W., Kauffman M. J., Merkle J. A. (2021). Emerging perspectives on resource tracking and animal movement ecology. Trends Ecol. Evol. 36, 308–320. doi: 10.1016/j.tree.2020.10.018
Archibald S., Scholes R. (2007). Leaf green-up in a semi-arid African savanna -separating tree and grass responses to environmental cues. J. Vegetation Sci. 18, 583–594. doi: 10.1111/J.1654-1103.2007.TB02572.X
Bartlam-Brooks H. L., Bonyongo M. C., Harris S. (2011). Will reconnecting ecosystems allow long-distance mammal migrations to resume? a case study of a zebra equus burchelli migration in Botswana. ORYX 45, 210–216. doi: 10.1017/S0030605310000414
Bates D., Maechler M., Bolker B., Walker S. (2022). “lme4: Linear mixed-effects models using eigen and S4,” in R package version 1.
Berg J. E., Hebblewhite M., St. Clair C. C., Merrill E. H. (2019). Prevalence and mechanisms of partial migration in ungulates. Front. Ecol. Evol. 7. doi: 10.3389/fevo.2019.00325
Bigalke R. (1972). Observations on the behaviour and feeding habits of the springbok, antidorcas marsupialis. Zool Africana 7, 333–359. doi: 10.1080/00445096.1972.11447448
Bischof R., Gjevestad J. G. O., Ordiz A., Eldegard K., Milleret C. (2019). High frequency GPS bursts and path-level analysis reveal linear feature tracking by red foxes. Sci. Rep. 9, 1–13. doi: 10.1038/s41598-019-45150-x
Bouvet A., Mermoz S., Le Toan T., Villard L., Mathieu R., Naidoo L., et al. (2018). An above-ground biomass map of African savannahs and woodlands at 25 m resolution derived from ALOS PALSAR. Remote Sens. Environ. 206, 156–173. doi: 10.1016/j.rse.2017.12.030
Bunnefeld N., Börger L., Van Moorter B., Rolandsen C. M., Dettki H., Solberg E. J., et al. (2011). A model-driven approach to quantify migration patterns: Individual, regional and yearly differences. J. Anim. Ecol. 80, 466–476. doi: 10.1111/j.1365-2656.2010.01776.x
Burkholder E. N., Jakes A. F., Jones P. F., Hebblewhite M., Bishop C. J. (2018). To jump or not to jump: Mule deer and white-tailed deer fence crossing decisions. Wildlife Soc. Bull. 42, 420–429. doi: 10.1002/wsb.898
Cain J. W., Krausman P. R., Rosenstock S. S., Turner J. C. (2006). Mechanisms of thermoregulation and water balance in desert ungulates. Wildlife Soc. Bull. 34, 570–581. doi: 10.2193/0091-7648(2006)34[570:motawb]2.0.co;2
Calenge and contributions from Scott Fortmann-Roe (2021). “adehabitatHR: Home range estimation. ” in R package version 0.4.19.
Caron A., Miguel E., Gomo C., Makaya P., Pfukenyi D. M., Foggin C., et al. (2013). Relationship between burden of infection in ungulate populations and wildlife/livestock interfaces. Epidemiol. Infection 141, 1522–1535. doi: 10.1017/S0950268813000204
Cronwright-Schreiner S. C., Wessels E. (1931). The migratory springbucks of south Africa (The trekbokke): Also an essay on the ostrich and a letter desciptive of the Zambezi falls (London: T. Fisher Unwin).
Cumming D. H. M., Osofsky S. A., Atkinson S. J., Atkinson M. W. (2015). “Beyond fences: Wildlife, livestock and land use in southern Africa,” in One health: the theory and practice of integrated health approaches (CABI), 243–257 (CABI International: CABI Books). doi: 10.1079/9781780643410.0243
Cushman S. A., Chase M., Griffin C. (2010). “Mapping landscape resistance to identify corridors and barriers for elephant movement in southern Africa,” in Spatial complexity, informatics, and wildlife conservation, vol. 9784431877. (Tokyo: Springer), 349–367. doi: 10.1007/978-4-431-87771-4_19
De Garine-Wichatitsky M., Fritz H., Gordon I. J., Illius A. W. (2004). Bush selection along foraging pathways by sympatric impala and greater kudu. Oecologia 141, 66–75. doi: 10.1007/s00442-004-1630-3
Dupuis-Désormeaux M., Davidson Z., Mwololo M., Kisio E., MacDonald S. E. (2016a). Usage of specialized fence gaps in a black rhinoceros conservancy in Kenya. Afr. J. Wildlife Res. 46, 22–32. doi: 10.3957/056.046.0022
Dupuis-Desormeaux M., Davidson Z., Pratt L., Mwololo M., MacDonald S. E. (2016b). Testing the effects of perimeter fencing and elephant exclosures on lion predation patterns in a Kenyan wildlife conservancy. PeerJ 2016, e1681. doi: 10.7717/peerj.1681
Dupuis-Desormeaux M., Kaaria T. N., Mwololo M., Davidson Z., MacDonald S. E. (2018). A ghost fence gap: Surprising wildlife usage of an obsolete fence crossing. PeerJ 2018, e5950. doi: 10.7717/peerj.5950
Ellis E. C., Gauthier N., Goldewijk K. K., Bird R. B., Boivin N., Díaz S., et al. (2021). People have shaped most of terrestrial nature for at least 12,000 years. Proc. Natl. Acad. Sci. United States America 118, e2023483118. doi: 10.1073/pnas.2023483118
Esmaeili S., Jesmer B. R., Albeke S. E., Aikens E. O., Schoenecker K. A., King S. R. B., et al. (2021). Body size and digestive system shape resource selection by ungulates: A cross-taxa test of the forage maturation hypothesis. Ecol. Lett. 00, ele.13848. doi: 10.1111/ele.13848
Funk C., Peterson P., Landsfeld M., Pedreros D., Verdin J., Shukla S., et al. (2015). The climate hazards infrared precipitation with stations - a new environmental record for monitoring extremes. Sci. Data 2, 1–21. doi: 10.1038/sdata.2015.66
Gates C. C., Jones P., Suitor M., Jakes A., Boyce M. S., Kunkel K., et al. (2012). “The influence of land use and fences on habitat effectiveness, movements and distribution of pronghorn in the grasslands of north America,” in Fencing for conservation: Restriction of evolutionary potential or a riposte to threatening processes? (NY: Springer New York), 277–294. doi: 10.1007/978-1-4614-0902-1_15
Gaynor K. M., Brown J. S., Middleton A. D., Power M. E., Brashares J. S. (2019). Landscapes of fear: Spatial patterns of risk perception and response. Trends in Ecol. and Evol. 34, 355–368. doi: 10.1016/j.tree.2019.01.004
Gentsch R. P., Kjellander P., Röken B. O. (2018). Cortisol response of wild ungulates to trauma situations: hunting is not necessarily the worst stressor. Eur. J. Wildlife Res. 64, 1–12. doi: 10.1007/S10344-018-1171-4/FIGURES/3
Goddard P. J., Summers R. W., Macdonald A. J., Murray C., Fawcett A. R. (2001). Behavioural responses of red deer to fences of five different designs. Appl. Anim. Behav. Sci. 73, 289–298. doi: 10.1016/S0168-1591(01)00150-2
Gulsby W. D., Stull D. W., Gallagher G. R., Osborn D. A., Warren R. J., Miller K. V., et al. (2011). Movements and home ranges of white-tailed deer in response to roadside fences. Wildlife Soc. Bull. 35, 282–290. doi: 10.1002/WSB.38
Harrington J. L., Conover M. R. (2006). Characteristics of ungulate behavior and mortality associated with wire fences. Wildlife Soc. Bull. 34, 1295–1305. doi: 10.2193/0091-7648(2006)34[1295:coubam]2.0.co;2
Hayward M. W. (2012). “Perspectives on fencing for conservation based on four case studies: Marsupial conservation in Australian forests; bushmeat hunting in south africa; large predator reintroduction in south africa; and large mammal conservation in Poland,” in Fencing for conservation: Restriction of evolutionary potential or a riposte to threatening processes? (NY: Springer New York), 7–20. doi: 10.1007/978-1-4614-0902-1_12
Hempson G. P., Archibald S., Bond W. J. (2015). A continent-wide assessment of the form and intensity of large mammal herbivory in Africa. Science 350, 1056–1061. doi: 10.1126/science.aac7978
Hering R., Hauptfleisch M., Jago M., Smith T., Kramer-Schadt S., Stiegler J., et al. (2022). Don’t stop me now: Managed fence gaps could allow migratory ungulates to track dynamic resources and reduce fence related energy loss. Front. Ecol. Evol. 10. doi: 10.3389/fevo.2022.907079
Hillman J. (1988). Home range and movement of the common eland (Taurotragus oryx pallas 1766) in Kenya. Afr. J. Ecol. 26, 135–148. doi: 10.1111/j.1365-2028.1988.tb00964.x
Hoare R. E. (1992). Present and future use of fencing in the management of larger African mammals. Environ. Conserv. 19, 160–164. doi: 10.1017/S0376892900030642
Ibrahim S., Kaduk J., Tansey K., Balzter H., Lawal U. M. (2021). Detecting phenological changes in plant functional types over West African savannah dominated landscape. Int. J. Remote Sens. 42, 567–594. doi: 10.1080/01431161.2020.1811914
Iturbide M., Gutiérrez J. M., Alves L. M., Bedia J., Cerezo-Mota R., Cimadevilla E., et al. (2020). An update of IPCC climate reference regions for subcontinental analysis of climate model data: definition and aggregated datasets. Earth System Sci. Data 12, 2959–2970. doi: 10.5194/ESSD-12-2959-2020
Jakes A. F., Jones P. F., Paige L. C., Seidler R. G., Huijser M. P. (2018). A fence runs through it: A call for greater attention to the influence of fences on wildlife and ecosystems. Biol. Conserv. 227, 310–318. doi: 10.1016/j.biocon.2018.09.026
Janzen M., Visser K., Visscher D., MacLeod I., Vujnovic D., Vujnovic K. (2017). Semi-automated camera trap image processing for the detection of ungulate fence crossing events. Environ. Monit. Assess. 189, 1–13. doi: 10.1007/s10661-017-6206-x
Jones P. F., Jakes A. F., MacDonald A. M., Hanlon J. A., Eacker D. R., Martin B. H., et al. (2020). Evaluating responses by sympatric ungulates to fence modifications across the northern great plains. Wildlife Soc. Bull. 44, 130–141. doi: 10.1002/wsb.1067
Jones P. F., Jakes A. F., Telander A. C., Sawyer H., Martin B. H., Hebblewhite M. (2019). Fences reduce habitat for a partially migratory ungulate in the northern sagebrush steppe. Ecosphere 10, e02791. doi: 10.10.1002/ecs2.2791
Jones P. F., Jakes A. F., Vegter S. E., Verhage M. S. (2022). Is it the road or the fence? influence of linear anthropogenic features on the movement and distribution of a partially migratory ungulate. Movement Ecol. 10, 37. doi: 10.1186/s40462-022-00336-3
Kauffman M. J., Aikens E. O., Esmaeili S., Kaczensky P., Middleton A., Monteith K. L., et al. (2021a). Causes, consequences, and conservation of ungulate migration. Annual Review of Ecology, Evolution, and Systematics 52, 453–478. doi: 10.1146/annurev-ecolsys-012021-011516
Kauffman M. J., Cagnacci F., Chamaillé-Jammes S., Hebblewhite M., Hopcraft J. G. C., Merkle J. A., et al. (2021b). Mapping out a future for ungulate migrations. Science 372, 566–569. doi: 10.1126/science.abf0998
Kesch K. M., Bauer D. T., Loveridge A. J. (2014). Undermining game fences: Who is digging holes in Kalahari sands? Afr. J. Ecol. 52, 144–150. doi: 10.1111/aje.12096
Laguna E., Barasona J. A., Carpio A. J., Vicente J., Acevedo P. (2022). Permeability of artificial barriers (fences) for wild boar (Sus scrofa) in Mediterranean mixed landscapes. Pest Manage. Sci 78 (6), 2277–2286. doi: 10.1002/ps.6853
Laskin D. N., Watt D., Whittington J., Heuer K. (2020). Designing a fence that enables free passage of wildlife while containing reintroduced bison: A multispecies evaluation. Wildlife Biol 4, wlb.00751 doi: 10.2981/wlb.00751
Lenth R. V. (2022). “Emmeans: Estimated marginal means, aka least-squares means. ” in R package version 1.7.5.
Lewis M. A., Fagan W. F., Auger-Méthé M., Frair J., Fryxell J. M., Gros C., et al. (2021). Learning and animal movement. Front. Ecol. Evol. 9. doi: 10.3389/fevo.2021.681704
Lindsey P. A. (2011). An analysis of game meat production in Namibia: Linkages with food security. (East/Southern Africa: TRAFFIC).
Lindsey P. A., Romañach S. S., Davies-Mostert H. T. (2009). The importance of conservancies for enhancing the value of game ranch land for large mammal conservation in southern Africa. J. Zoology 277, 99–105 doi: 10.1111/j.1469-7998.2008.00529.x
Linnell J. D., Trouwborst A., Boitani L., Kaczensky P., Huber D., Reljic S., et al. (2016). Border security fencing and wildlife: The end of the transboundary paradigm in Eurasia? PloS Biol. 14, e1002483. doi: 10.1371/journal.pbio.1002483
Mbaiwa B. Y. J. E., Mbaiwa O. I. (2006). The effects of veterinary fences on wildlife populations in okavango delta, Botswana. Int. J. Wilderness 12, 17–24.
McInturff A., Xu W., Wilkinson C. E., Dejid N., Brashares J. S. (2020). Fence ecology: Frameworks for understanding the ecological effects of fences. BioScience 70, 971–985. doi: 10.1093/biosci/biaa103
Mogotsi K., Kgosikoma O. E., Lubinda K. F. (2016). Wildlife-livestock interface, veterinary cordon fence damage, lack of protection zones, livestock theft and owner apathy: Complex socio-ecological dynamics in foot and mouth disease control in southern Africa. Pastoralism 6, 21. doi: 10.1186/s13570-016-0068-7
Monteith K. L., Hayes M. M., Kauffman M. J., Copeland H. E., Sawyer H. (2018). Functional attributes of ungulate migration: landscape features facilitate movement and access to forage. Ecol. Appl. 28, 2153–2164. doi: 10.1002/eap.1803
Mutinda M., Chenge G., Gakuya F., Otiende M., Omondi P., Kasiki S., et al. (2014). Detusking fence-breaker elephants as an approach in human-elephant conflict mitigation. PloS One 9, e91749. doi: 10.1371/JOURNAL.PONE.0091749
Naidoo R., Beytell P., Brennan A., Kilian W., McCulloch G., Stronza A., et al. (2022). Challenges to elephant connectivity from border fences in the world’s largest transfrontier conservation area. Front. Conserv. Sci. 3. doi: 10.3389/fcosc.2022.788133
Nandintsetseg D., Bracis C., Olson K. A., Böhning-Gaese K., Calabrese J. M., Chimeddorj B., et al. (2019). Challenges in the conservation of wide-ranging nomadic species. J. Appl. Ecol. 56, 1916–1926. doi: 10.1111/1365-2664.13380
Nathan R., Monk C. T., Arlinghaus R., Adam T., Alós J., Assaf M., et al. (2022). Big-data approaches lead to an increased understanding of the ecology of animal movement. Science 375, eabg1780. doi: 10.1126/science.abg1780
Osipova L., Okello M. M., Njumbi S. J., Ngene S., Western D., Hayward M. W., et al. (2018). Fencing solves human-wildlife conflict locally but shifts problems elsewhere: A case study using functional connectivity modelling of the African elephant. J. Appl. Ecol. 55, 2673–2684. doi: 10.1111/1365-2664.13246
Owen-Smith N. (1985). The ecological potential of the kudu for commercial production in savanna regions. J. Grassland Soc. South. Afr. 2, 7–10. doi: 10.1080/02566702.1985.9648005
Owen-smith N. (1990). Demography of a Large herbivore, the greater kudu tragelaphus strepsiceros, in relation to rainfall. J. Anim. Ecol. 59, 893. doi: 10.2307/5021
Owen-Smith N. (2014). Spatial ecology of large herbivore populations. Ecography 37, 416–430. doi: 10.1111/j.1600-0587.2013.00613.x
Owen-Smith N., Fryxell J. M., Merrill E. H. (2010). Foraging theory upscaled: The behavioural ecology of herbivore movement. Phil. Trans. R. Soc. B 365, 2267–2278. doi: 10.1098/rstb.2010.0095
Owen-Smith N., Goodall V. (2014). Coping with savanna seasonality: Comparative daily activity patterns of African ungulates as revealed by GPS telemetry. J. Zool 293, 181–191. doi: 10.1111/jzo.12132
Palmer W. L., Payne J. M., Wingard R. G., George J. L. (1985). A practical fence to reduce deer damage. Wildlife Soc. Bull. 13, 240–245.
Pirie T. J., Thomas R. L., Fellowes M. D. (2017). Game fence presence and permeability influences the local movement and distribution of south African mammals. Afr. Zool 52, 217–227. doi: 10.1080/15627020.2017.1410074
Prokopenko C. M., Boyce M. S., Avgar T. (2017). Characterizing wildlife behavioural responses to roads using integrated step selection analysis. J. Appl. Ecol. 54, 470–479. doi: 10.1111/1365-2664.12768
Prugh L. R., Sivy K. J., Mahoney P. J., Ganz T. R., Ditmer M. A., van de Kerk M., et al. (2019). Designing studies of predation risk for improved inference in carnivore-ungulate systems. Biol. Conserv. 232, 194–207. doi: 10.1016/j.biocon.2019.02.011
R Core Team (2022). R: A language and environment for statistical computing. (Vienna, Austria: R Foundation for Statistical Computing).
Robb B. S., Merkle J. A., Sawyer H., Beck J. L., Kauffman M. J. (2022). Nowhere to run: Semi-permeable barriers affect pronghorn space use. J. Wildlife Manage 86, e22212. doi: 10.1002/jwmg.22212
Roche C. (2005). Springbok drink the rain’s blood’: Indigenous knowledge and its use in environmental history-the case of the /Xam and an understanding of springbok treks*. South Afr. Historical J. 53, 1–22. doi: 10.1080/02582470509464887
Roche C. (2008). ‘The fertile brain and inventive power of man’: Anthropogenic factors in the cessation of springbok treks and the disruption of the karoo ecosystem 1865–1908. Africa 78, 157–188. doi: 10.3366/e0001972008000120
Safner T., Gracanin A., Gligora I., Pokorny B., Flajšman K., Apollonio M., et al. (2021). State border fences as a threat to habitat connectivity: A case study from south-eastern Europe. Sumarski List 145, 269–278. doi: 10.31298/SL.145.5-6.6
Sawyer H., Kauffman M. J., Middleton A. D., Morrison T. A., Nielson R. M., Wyckoff T. B. (2013). A framework for understanding semi-permeable barrier effects on migratory ungulates. J. Appl. Ecol. 50, 68–78. doi: 10.1111/1365-2664.12013
Scully W. C. (1913). Further reminiscences of a south African pioneer (TF unwin). (London:T. Fisher Unwin).
Segar J., Keane A. (2020). Species and demographic responses to wildlife-friendly fencing on ungulate crossing success and behavior. Conserv. Sci. Pract. 2, e285. doi: 10.1111/csp2.285
Skinner J. D. (1993). Springbok (Antidorcas marsupialis) treks. Trans. R. Soc. South Afr. 48, 291–305. doi: 10.1080/00359199309520276
Skinner J. D., Chimimba C. T. (2005). The mammals of the southern African Sub-region (Cambridge:Cambridge University Press). doi: 10.1017/cbo9781107340992
Skinner G., Louw J. (1996). The springbok (Antidorcas marsupialis) - ecology and physiology. Transvaal Musuem Monogr. 10, 19–35.
Spinage C. A. (1992). The decline of the Kalahari wildebeest. Oryx 26, 147–150. doi: 10.1017/S0030605300023577
Taylor W. A., Lindsey P. A., Nicholson S. K., Relton C., Davies-Mostert H. T. (2020). Jobs, game meat and profits: The benefits of wildlife ranching on marginal lands in south Africa. Biol. Conserv. 245, doi:108561. doi: 10.1016/J.BIOCON.2020.108561
Teitelbaum C. S., Mueller T. (2019). Beyond migration: Causes and consequences of nomadic animal movements. Trends in Ecology and Evolution 34, 569–581. doi: 10.1016/j.tree.2019.02.005
Thieurmel B., Elmarhraoui A. (2019). “Suncalc: Compute sun position, sunlight phases, moon position and lunar phase.” in R package version 0.5.0.
Tucker M. A., Böhning-Gaese K., Fagan W. F., Fryxell J. M., Moorter B. V., Alberts S. C., et al. (2018). Moving in the anthropocene: Global reductions in terrestrial mammalian movements. Science 359, 466–469. doi: 10.1126/SCIENCE.AAM9712
Vanak A. T., Thaker M., Slotow R. (2010). Do fences create an edge-effect on the movement patterns of a highly mobile mega-herbivore? Biol. Conserv. 143, 2631–2637. doi: 10.1016/j.biocon.2010.07.005
van Dyk G., Slotow R. (2003). The effects of fences and lions on the ecology of African wild dogs reintroduced to pilanesberg national park, south Africa. Afr. Zool 38, 79–94. doi: 10.1080/15627020.2003.11657196
Van Moorter B., Engen S., Fryxell J. M., Panzacchi M., Nilsen E. B., Mysterud A. (2020). Consequences of barriers and changing seasonality on population dynamics and harvest of migratory ungulates. Theor. Ecol. 13, 595–605. doi: 10.1007/s12080-020-00471-wV
Visscher D. R., MacLeod I., Janzen M., Visser K., Lekas A. (2016). The impact of wildlife friendly fences on ungulate crossing behaviour at the wainwright dunes ecological reserve (Edmonton, AB: The King’s University).
Weise F. J., Wessels Q., Munro S., Solberg M. (2011). Using artificial passageways to facilitate the movement of wildlife on Namibian farmland. South Afri J Wildlife Res 44 (2), 161–166. doi: 10.3957/056.044.0213
Wickham H., Chang W., Henry L., Pedersen T. L., Takahashi K., Wilke C., et al. (2022). “ggplot2: Create elegant data visualisations using the grammar of graphics.” in R package version 3.3.6.
Wilkinson C. E., McInturff A., Kelly M., Brashares J. S. (2021). Quantifying wildlife responses to conservation fencing in East Africa. Biol. Conserv. 256, 109071. doi: 10.1016/j.biocon.2021.109071
Xu W., Dejid N., Herrmann V., Sawyer H., Middleton A. D. (2021). Barrier behaviour analysis (BaBA) reveals extensive effects of fencing on wide-ranging ungulates. J. Appl. Ecol. 58, 690–698. doi: 10.1111/1365-2664.13806
Keywords: fence ecology, veterinary cordon fence, ungulate, movement speed, fence interaction, GPS, Africa, wildlife conservation
Citation: Hering R, Hauptfleisch M, Kramer-Schadt S, Stiegler J and Blaum N (2022) Effects of fences and fence gaps on the movement behavior of three southern African antelope species. Front. Conserv. Sci. 3:959423. doi: 10.3389/fcosc.2022.959423
Received: 01 June 2022; Accepted: 21 September 2022;
Published: 20 October 2022.
Edited by:
Christine Wilkinson, University of California - Berkeley, United StatesReviewed by:
Norman Owen-Smith, University of the Witwatersrand, South AfricaEmily Bennitt, University of Botswana, Botswana
Copyright © 2022 Hering, Hauptfleisch, Kramer-Schadt, Stiegler and Blaum. This is an open-access article distributed under the terms of the Creative Commons Attribution License (CC BY). The use, distribution or reproduction in other forums is permitted, provided the original author(s) and the copyright owner(s) are credited and that the original publication in this journal is cited, in accordance with accepted academic practice. No use, distribution or reproduction is permitted which does not comply with these terms.
*Correspondence: Robert Hering, cm9iZXJ0LmhlcmluZ0B1bmktcG90c2RhbS5kZQ==