- 1Department of Biology, University of Kentucky, Lexington, KY, United States
- 2School of Earth and Space Exploration, Arizona State University, Tempe, AZ, United States
- 3Max Plank Institute for Mathematics in the Sciences, Leipzig, Germany
- 4Genomica, Ecologia y Medio Ambiente (GEMA) Center for Genomics, Ecology and Environment, Universidad Mayor, Santiago, Chile
- 5Network Science Institute, Northeastern University, Boston, MA, United States
- 6Department of Computer Science, University of Arizona, Tucson, AZ, United States
- 7Department of Ecology and Evolutionary Biology, University of Arizona, Tucson, AZ, United States
- 8Department of Geography, Florida State University, Tallahassee, FL, United States
- 9School of Geographical Sciences and Urban Planning, Arizona State University, Tempe, AZ, United States
- 10School of Natural Resources and the Environment, University of Arizona, Tucson, AZ, United States
- 11Department of Epidemiology and Biostatistics, University of Arizona, Tucson, AZ, United States
- 12Santa Fe Institute, Santa Fe, NM, United States
Understanding scaling relations of social and environmental attributes of urban systems is necessary for effectively managing cities. Urban scaling theory (UST) has assumed that population density scales positively with city size. We present a new global analysis using a publicly available database of 933 cities from 38 countries. Our results showed that (18/38) 47% of countries analyzed supported increasing density scaling (pop ~ area) with exponents ~⅚ as UST predicts. In contrast, 17 of 38 countries (~45%) exhibited density scalings statistically indistinguishable from constant population densities across cities of varying sizes. These results were generally consistent in years spanning four decades from 1975 to 2015. Importantly, density varies by an order of magnitude between regions and countries and decreases in more developed economies. Our results (i) point to how economic and regional differences may affect the scaling of density with city size and (ii) show how understanding country- and region-specific strategies could inform effective management of urban systems for biodiversity, public health, conservation and resiliency from local to global scales.
200 word statement of contribution: Urban Scaling Theory (UST) is a general scaling framework that makes quantitative predictions for how many urban attributes spanning physical, biological and social dimensions scale with city size; thus, UST has great implications in guiding future city developments. A major assumption of UST is that larger cities become denser. We evaluated this assumption using a publicly available global dataset of 933 cities in 38 countries. Our scaling analysis of population size and area of cities revealed that while many countries analyzed showed increasing densities with city size, about 45% of countries showed constant densities across cities. These results question a key assumption of UST. Our results suggest policies and management strategies for biodiversity conservation, public health and sustainability of urban systems may need to be tailored to national and regional scaling relations to be effective.
Introduction
Urbanization has significantly increased in recent decades. Developing a science of cities is necessary to understand, predict and manage the future of an urbanizing planet (Acuto et al., 2018; Burger et al., 2019; Bettencourt, 2021; Uchida et al., 2021). A general theory of cities should predict how variation in city size, infrastructure, and governance are linked to influence biodiversity, urban ecosystems, resource use, social interactions, innovations, economic activity, public health, crime, and urban sustainability Bettencourt et al., 2007; Lobo et al., 2019; Bettencourt, 2020; 2021; Uchida et al., 2021). Indeed, while many socioeconomic, behavioral, ecological, and evolutionary processes are affected by urbanization, it is unclear how city size impacts these processes directly or indirectly via variation in human population density.
Several studies have recently extended scaling approaches from biology—where various organismal characteristics scale with size—to the study of cities (Bettencourt et al., 2007; Bettencourt and West, 2010; Fragkias et al., 2013; Bettencourt, 2013, 2020; Oliveira et al., 2014; West, 2018; Lobo et al., 2019; Ortman et al., 2020). Urban Scaling Theory (UST; a.k.a Urban Settlement Theory) is a general scaling framework that makes quantitative predictions for many urban attributes spanning physical, biological, and social dimensions, and how they scale with city size. Similar to the scaling of traits with body size in biology, various attributes of cities scale as a power-law:
where Y is an attribute for a given city at time (t), N is human population size or total number of inhabitants in the city, Y0 is the elevation and α is the exponent. The three main classes of scaling behavior are: (i) Sublinear (α < 1), (ii) linear (α = 1, aka isometric), and (iii) superlinear scaling (α > 1). Analysis of initial data from modern cities in a few countries suggest α < 1 for infrastructural quantities; α = 1 for measures of resource use and waste production; and α > 1 for social attributes including super-creative employment, crime, and infectious disease (Bettencourt et al., 2007). These empirical patterns have helped develop the mechanistic theory behind the scaling categories, with spatial filling networks and social interaction dynamics in growing urban systems as their determinants (Bettencourt, 2013).
A key underlying assumption of UST mechanistic theory is that densities increase with city size, which results in economies of scale (α < 1) in space use (Bettencourt, 2013) that influences the scaling of several characteristics of cities, particularly those related to social outcomes. This results in exponents of α > 1 for some urban features are due to efficiencies gained in communication and infrastructure networks in larger cities. More specifically, assuming optimal infrastructure and material use, UST proposes that circumscribing urban areas and the urban built area scale with population size as α = ⅔ and α = ⅚, respectively. However, it is essential to establish empirical foundations to advance general theory and applications for global urban management practices.
Population density is also a critical variable of study for other areas of fundamental urban science and practical purposes. Variation in human population density reflects the unique ecology of hyper-dense urban societies existing at several orders of magnitude denser (Burger et al., 2017; Burger and Fristoe, 2018). Density fundamentally affects social interactions, the built environment, open/green spaces, demand for natural resources and impacts on landscapes. The null expectation is that population density is invariant of city size if city area scales isometrically with population size. However, UST predicts that city area scales sublinearly with city population size and, therefore, human population density increases with city size. Increases in population density suggests important underlying interactions and dynamics and has fundamental implications for the emergent behavior of many urban social attributes.
Despite the apparent importance of understanding variation in urban densities, there is limited understanding, however, of how the scaling of area with population size vary globally. Some studies have supported sublinear scaling of areas with population size based on ordinary least-square regression and limited data that is highly unrepresentative of cities especially in the tropics and “Global South”. For example, one seminal study was based on data for just four highly-developed nations: USA, Sweden, United Kingdom, and Canada (Bettencourt, 2013). Another study was based on historical societies with small city sizes compared to modern cities (Lobo et al., 2019). A recent global analysis of the scaling of area with population size using data from the Organisation for Economic Co-operation and Development (OECD) showed mixed results where some countries and regions scaled linearly and even superlinear, while others scaled sublinearly at 5/6 (Ortman et al., 2020). This study used Functional Urban Areas (FAU) defined as “spatial units whose outlines encapsulate daily flows of people, goods, and information” and hypothesized that countries conforming to UST predictions with α ≈ ⅚ are countries developing incrementally with homogeneous social-mixing, whereas countries where α ≠ ⅚ are experiencing rapid development and land expansion. So, advancing urban science and UST necessitates assessing if urban area scales sublinearly to what exponent. Doing so is the first step in developing a general scaling framework for managing urban biodiversity and sustainable cities.
Here, we examine the scaling behavior of city areas with population size. We test the assumption of increasing densities, people per area, and city size by using a publicly available global database that fills important gaps in urban scaling knowledge (Acuto et al., 2018), especially in tropical regions. In addition, we explored whether factors such as regional differences, economic development status, and time influence the value of the scaling relations of the log population vs log area relationship. We end by discussing the important implications of these findings for managing urban ecosystems and biodiversity at local, regional and global scales.
Materials and methods
The data
We assessed the scaling of urban area and population size for 933 cities in 38 countries globally. We used the publicly available Global Human Settlement (GHS; Melchiorri, 2022) Urban Center Database (https://ghsl.jrc.ec.europa.eu/ghs_stat_ucdb2015mt_r2019a.php; Pesaresi et al., 2019) and the associated Global Human Settlement Layer (GHSL) project (https://ghsl.jrc.ec.europa.eu/index.php; Florczyk et al., 2019). The GHS classifies the physical extent of human settlements – from large megacities to villages and towns using a consistent methodology across the globe with satellite remote sensing as a primary source of information. Supervised automatic data classification is used to build the GHS built-up area grid (GHS-BUILT) using open decametric-resolution satellite imagery collected by the Landsat and Sentinel missions. Using census data through spatial modeling techniques combined with GHS-BUILT, the GHSL project produces global population density grids (GHS-POP). The data integration process foresees the downscaling of the information from the national census district level to a regular, finer-scaled, gridded, built-up density information layer at the resolution of 250m2 and 1 km2. Importantly, the GHS does not assign cities and populations by political boundaries (e.g., MSAs, countries, counties), but rather by the clustering and proximity of the built-up environment classified as urban.
The combination of GHS-BUILT and GHS-POP produces the GHS settlement model (GHS-SMOD), building on the “Degree of Urbanization” concept (Dijkstra and Poelman, 2014). Distinguishing between three main typologies of human settlements based on population density cut-off values: Urban Centers, Urban Clusters, and Rural Settlements. The Urban Centers, which represent the most densely inhabited part of human settlements, are defined as “the spatially-generalized high-density clusters of contiguous grid cells of 1 km2 with a density of at least 1,500 inhabitants per km2 of land surface or at least 50% built-up surface share per km2 of the land surface, and a minimum population of 50,000”, and are the core of the GHS-UCDB dataset. It considers all “Urban Centers” data from the GHS-SMOD 2015, 1 x 1 km2 resolutions, leading to a database containing over 10,000 individual cities and characterizing each by a number of variables describing the geography and the environment of the place, as well as socio-economic parameters and the potential exposure of an Urban Center to natural disasters. Initial analysis of all the data produced erroneous results due to variation in the resolution of the combined datasets in GHS. Further inspection of ‘outlier’ cities by our international team of scientists further identified problems with small area cities in the dataset in Bangladesh, Nepal, and other countries. The GHS was notified and agreed this was problematic and should be corrected in future versions. So, we excluded cities with total urban areas of <100 km2 similar to Ortman et al. (2020), reducing the number in our analysis to 933 cities from countries with at least five cities with >50,000 people.
Statistical analysis
We conducted both Reduced Major Axis (RMA) and Ordinary Least Squares (OLS) regression of log population size versus log area to estimate the scaling exponent (α) and elevation (β) for countries by years available in the GHS database (yrs. 1975, 1990, 2000, 2015) following Fuller and Gaston (2009) and Gudipudi et al. (2019). We report OLS and RMA regression statistics and scaling parameters in Appendix. We used the 95% confidence intervals (CIs) of scaling exponents to evaluate whether within-in country scaling relations were statistically indistinguishable from linear scaling (CIs bounding 1) versus providing support for allometric scaling (CIs not containing 1), including overlapping with α = 5/6 as is common practice in the city scaling literature (e.g., Finance and Swerts, 2020). The general nature of the results were similar between RMA and OLS, so we report only RMA analyses in the text because it is the standard method for allometric scaling studies (see Appendix and Supplemental Material for more information). We assessed the effect of the development status of the countries over scaling exponents and elevation through an analysis of covariance (ANCOVA), where log(population) was the dependent variable and population and development status of the country and their interaction were the predictor variables.
Results
Country-specific scalings
Our analysis revealed that the scaling exponents (α) varied widely across countries (Figures 1, 2), from 0.63-to 1.08 (Appendix); 17 countries (47%) supported UST predictions of a sublinear scaling exponent of ⅚ for built urban areas. In contrast, 18 countries (48%) had scaling relations indistinguishable from linear (α = 1) including 8 countries with 10 or more cities of similar densities (Table 1). See Appendix for country-level statistics for all years.
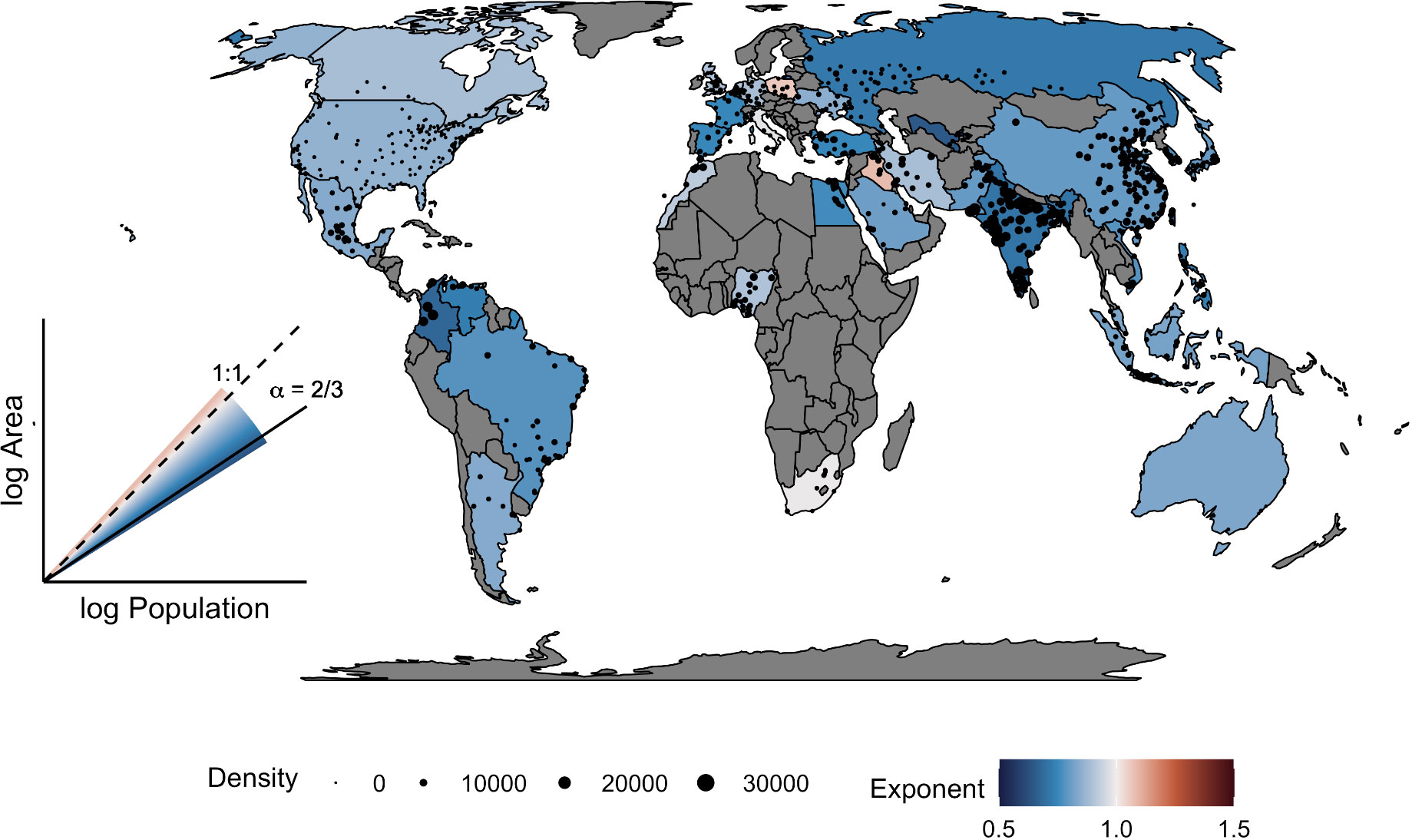
Figure 1 Global map of city scaling exponents for 38 countries for year 2015 from the GHS. See Appendix 1 for scaling statistics by country. Note that colors represent exponents for countries that contain more than 5 cities with an area >100km2 and >50,000 population. Gray countries do not satisfy these criteria; point size represents city density, which varies from <1,000 to >30,000.
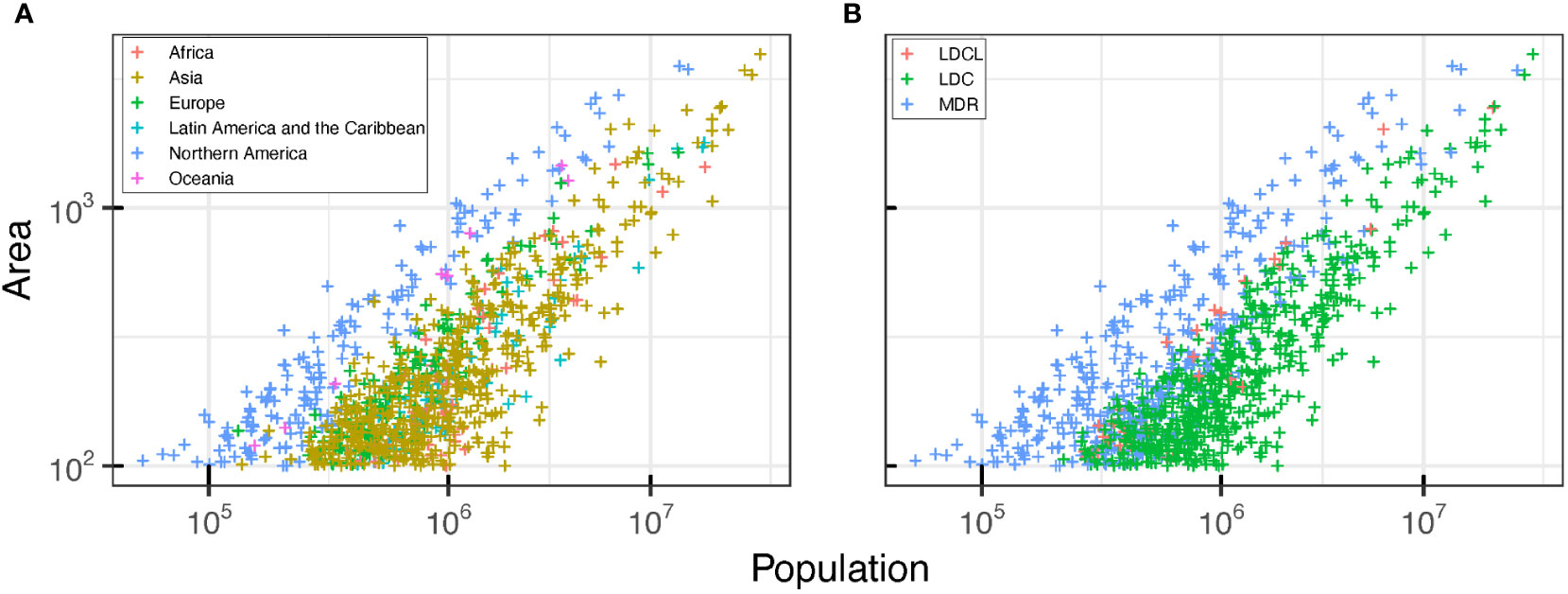
Figure 2 A scatter plot of 933 cities in 2015 used in this study colored by geographic region (A) and level of economic development status: LDC = low development countries, LDCL = least developed countries, and MDR = more developed countries (B). Note that the x and y axes are log10 transformed.

Table 1 The number and percentage of countries in 2015 with scaling exponents from containing isometry (α = 1) and/or the UST predicted values (α = 5/6) based on 95% Confidence Intervals.
We found greater variation in the elevation Y0 between countries than within (Appendix). Development status had no detectable effect on scaling exponents (ANCOVA: F = 1.929, p = 0.146), but affected Y0 (ANCOVA: F = 311.254, p < 0.001), being highest in the More Developed countries and lower in the Less and Least Developed countries. Those results indicate that cities in less and least developed countries are denser than the more developed countries for any given city size. For example, the highest density cities in the curated GHS dataset occur in countries of Asia (Surat = 24,117 ind km-2), Africa (Cairo = 12,451 ind km-2) and tropical Latin America (Salvador = 9,839 ind km-2) and are considerably higher for a given city size than densities in the USA (NYC = 2,963 ind km-2), Australia (Sydney = 2,762 ind km-2), Canada (Toronto = 2,990 ind km-2). Maximum densities for each country range from ~2,000-20,000 ind km-2, and tend to be higher close to the tropics (Figure 1). See appendix for full statistics by country and years.
Temporal analysis
We found scaling exponents to vary over time for some countries and regions and remain invariant in others (Figures 3, 4; Appendix). Notably, the 95% CIs for the exponents of 13 countries overlap with one (linear) over three decades (Belgium, Colombia, Germany, Iran, Malaysia, Nigeria, Pakistan, Poland, Saudi Arabia, South Africa, Ukraine, and Venezuela) whereas 9 countries stayed sublinear (Brazil, Egypt, France, India, Japan, Mexico, Russia, Taiwan, and Uzbekistan). In contrast, Italy changed from superlinear to linear, and 12 countries changed from linear to sublinear (Argentina, Australia, Bangladesh, Canada, China, Indonesia, South Korea, Spain, Turkey, United States, United Kingdom).
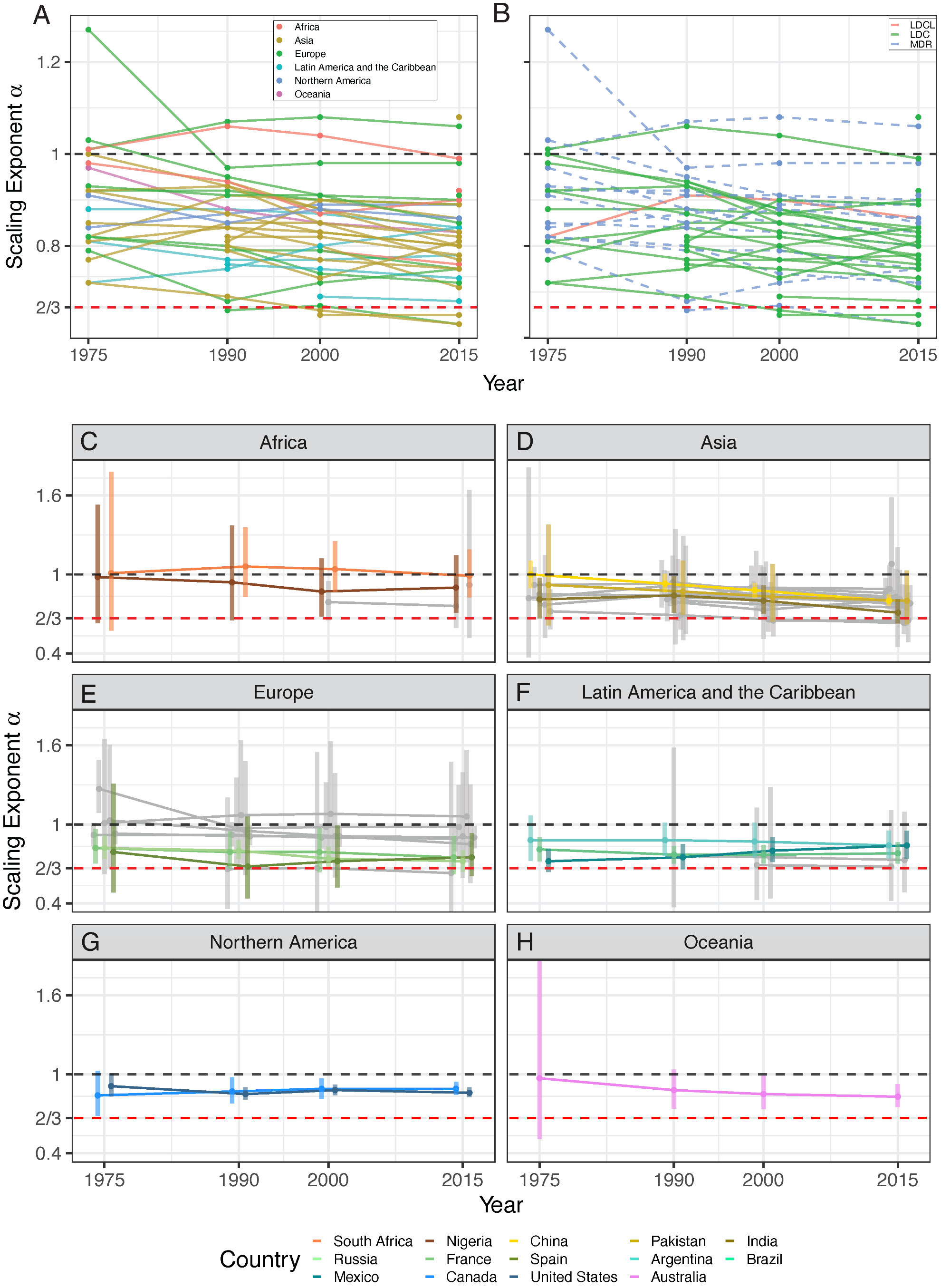
Figure 3 Scaling exponents over time by: (A) all regions, (B) 2015 economic development status, (C) Africa, (D) Asia, (E) Europe, (F) Latin America and the Caribbean, (G) Northern America, (H) Oceania. Note each line represents the exponent for a country over time and vertical lines 95% Confidence Intervals. Select countries listed in the legend are highlighted.
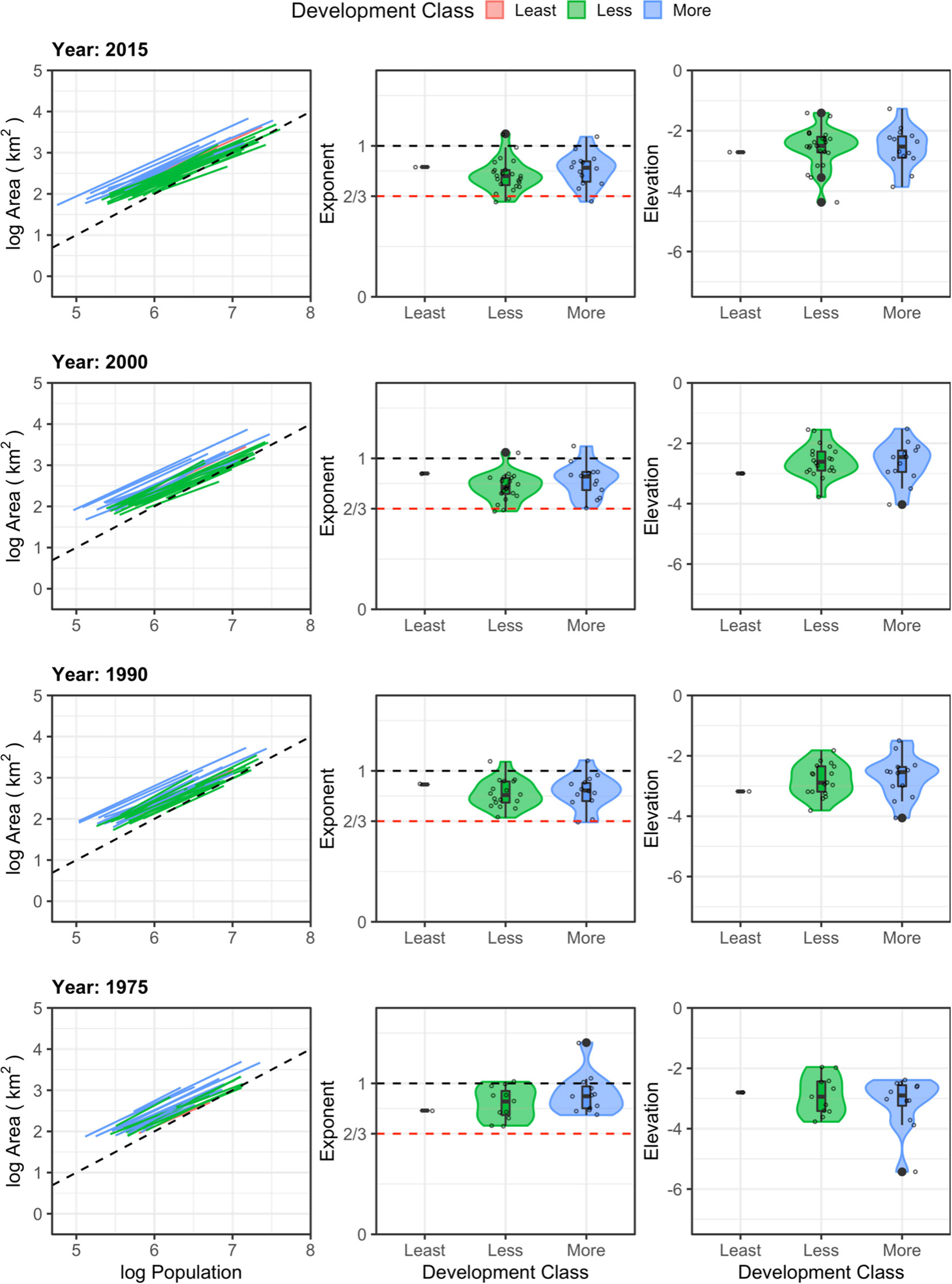
Figure 4 Comparison of log area~ log population scaling for GHS dataset for different decades. Black dashed lines represent the 1/1 line (e.g., α = 1). All regressions (Reduced Major Axis) are country-level with at least 5 cities of > 50,000 people and >= 100 km2 for the specific year.
Discussion
Using a new multi-decadal global-scale dataset, our results fill a gap in the literature by evaluating how commonly density increases with urban population size across the globe, leading to sublinear scaling of area with population size. Our results show mixed support for the UST consistent with previous studies (Batty and Furgeson, 2011; Ortman et al., 2020). Sublinear scaling of area with population (increasing density) with an exponent of ⅚ is generalizable for only ~47% of countries globally. In contrast, ~48% of countries showed scaling relations statistically indistinguishable from constant densities across cities. Some countries (N = 13) exhibited constant densities across cities for four time periods spanning three decades. Thus, we conclude that sublinear scaling as predicted by the UST is not the norm.
Why do we see variation in scaling parameters and a lack of support for UST in many countries? It is unlikely a statistical artifact since several of the countries not supporting UST predictions have sufficient (>10) cities. Our results are similar to Ortman et al., 2020, which used a different methodology in the OECD “Functional Urban Areas” data resulting in substantially different sample sizes. Our results are additionally incomparable because their results are reported mostly for regions instead of countries. However, Ortman et al. (2020) hypothesize countries that do not support UST predictions have experienced rapid growth and land expansion in recent decades, whereas UST assumes social-mixing and incremental development. It is possible that the countries that do not exhibit scaling to the ⅚ UST prediction fail to meet these assumptions. In UST, the proposed mechanisms underlying increasingly denser larger cities are based on the capacity of the transport and communication system to connect all of its inhabitants and are assumed self-similar, maintaining their properties regardless of scale (Bettencourt, 2013; Bettencourt, 2020; Ortman and Lobo, 2020). However, such communication systems are costly, and the “ideal” (theoretical) connection proposed by UST may not be achieved in some countries. Countries with low per capita GDP may face greater challenges in investing and maintaining the needed transportation and communication connectivity costs for their inhabitants, potentially leading to lower-performing cities economically (Ortman et al., 2020). However, our analysis did not reveal differences in exponents by economic development status as suggested by Ortman et al. (2020). More and better quality data across cities, especially from the “Global South,” will allow us to better understand the links between city scalings, economic development, and country-level outcomes in urban scaling parameters. Our results showing variation in urban density scaling for 38 countries is a step in that direction.
Our analysis did reveal differences in elevation, Y0 , by economic development status in the area-size scaling relations and only recently has this received attention in the UST literature (e.g., Bettencourt, 2020; Bettencourt et al., 2020; Ortman and Lobo, 2020). Our results show that Y0 varies ~1 order of magnitude between countries and was related to socioeconomic and geographic drivers, with more developed economies having lower urban densities and occurring at higher latitudes. Perhaps counterintuitive, countries with high density cities and less developed economies are the countries that have been slower to urbanize and transition to service-based economies from predominantly rural, resource-based economies (Burger et al., 2019). Countries with the highest density cities (highest Y0) and low economic development also have higher fertility relates and larger family sizes (Moses and Brown, 2003; DeLong et al., 2010; Burger, 2019) possibly related to high-density living. Interestingly, this latitudinal variation in urban densities and economic wealth mirrors gradients in biodiversity, with tropical areas having higher numbers of species of not only animals and plants (Brown, 2014) but also human pathogens and parasites compared to temperate regions (Guernier et al., 2004). Recently, urban scaling has been applied to understanding and managing the Covid-19 pandemic (Ribeiro et al., 2020; Stier et al., 2020). So, a possible practical avenue for future research is to understand the consequences of urban density scaling for contact rates, transmission and evolution of human pathogens and how to mitigate epidemics in countries with different scaling exponents and elevations. More investigation into the country- and region-specific drivers and consequences of variation in urban density scaling are thus clearly needed. Our results suggest that UST may need to be further refined and expanded to address this variation in scaling exponents and elevations theoretically with important practical applications.
Urban density and biodiversity
Understanding the scaling relations of urban densities has important consequences for green space and habitat for biodiversity (Callaghan et al., 2021; Uchida et al., 2021; Dunn et al., 2022). The country-specific parameter estimates in scaling exponents and Y0 (elevation) provided in the Appendix have implications for country-specific management and policy. For instance, population density and city area are both fundamental variables for biodiversity conservation, influencing human pressures on habitats and the fragmented structure of habitats. If density increases with city size for a given country, different incentives may be put in place to allow compensatory green space in larger, denser cities. The scaling of green space, therefore, may impact biodiversity.
Uchida et al. (2021) hypothesized that urban areas have higher biodiversity than similar size non-urban areas where the scaling exponent of the Species-Area-Relationship may be steeper with higher log-log intercepts. However, this relationship has largely been investigated in cities of the temperate Northern Hemisphere. For example, in European cities, bird species richness appears to increases with city area as power-law with exponent of 0.18. Given the shallow exponent, however, only a small number of species turnover is required in order for small cities to support more biodiversity per inhabitant. Indeed, for specific taxa like birds, species-area richness is found to be higher in urban areas compared to their rural counterparts (Ferenc et al., 2016; Callaghan et al., 2021; but see Fattorini et al., 2018 for Italy) and also depends on latitude (Murthy et al., 2016). However, this outcome may not necessarily be congruent with conservation priorities since urban areas often have greater numbers of species of both native and non-native generalist species resulting in part due increased species introductions and resource availability by humans in urban environments (Ferenc et al., 2016; Murthy et al., 2016). Our study highlights a latitudinal gradient in population density where the highest density cities in the world are located in the tropics, where there is generally higher terrestrial biodiversity (Brown, 2014). Further studies are needed to understand how variation in density scalings impacts the species-area relationship in cities in temperate versus tropics cities and in different biomes (Uchida et al., 2021).
More broadly, our results highlight the need to better understand how other large-scale geographic, political, and socioeconomic factors drive variation in population density and shape the area-size scaling exponent (α) and elevation (Y0) . In countries in which the scaling of area with population size is linear, the effect of the size distribution of cities should be invariant for regional biodiversity. Yet, other attributes of cities for urban biodiversity could also be important that do not scale linearly with city size. Additionally, whether or not multiple small cities can support more total urban species richness (gamma diversity) than a few large cities of the equivalent population depend on the rate of turnover in species composition between cities and the exponent of the species-area relationship of cities Uchida et al., 2021). Thus, resolving this dilemma requires a better understanding of the scaling of various biodiversity-relevant features of cities, such as how green space, biodiversity, net primary productivity, and habitat connectivity scale with city size. Additionally, the spatial distribution of cities of varying sizes undoubtedly affects the regional connectivity of habitats, influencing rates of colonization and dispersal between and within cities and rural areas. Our analysis of city area and population provide crucial information to advance more empirical and theoretical development to effectively understanding country-level scaling effects.
Extra-urban scalings
Cities are dependent on extra-urban interactions from regional and global trade networks (Burger et al., 2012) not currently considered in UST. The productivity of their boundaries does not constrain modern cities due to the industrial revolution, and the use of fossil fuels to now import vast quantities of goods and services from global trade networks into cities (Krausmann et al., 2008; Meyfroidt et al., 2011, Burger et al., 2012, 2019; Schramski et al., 2015, 2019, 2020). An increase in urbanization within countries over time shows (i) greater dependence on the net import of raw materials from abroad, increasing the actual footprint of the city that is not being taken into account (Rees and Wackernagl, 1996; Hidalgo, 2009; Meyfroidt et al., 2011; Burger et al., 2012; Schramski et al., 2019, 2020), (ii) a general trend of per capita increase in extra-metabolic and metabolic (i.e., food) energy sources and material consumption (Brown et al., 2011; Burger et al., 2017, 2019) (iii) an accelerated extinction of biodiversity and alterations of ecosystem function (e.g., Ceballos et al., 2015) that are so tightly related to the uncertainties behind the global change and the future Anthropocene era scenarios and our survival as species (Barnosky et al., 2012; Steffen et al., 2018). Future studies should advance linking urban scaling relations to extra-urban dynamics that are currently not accounted for in theoretical and empirical studies of the UST.
Conclusion
Using a global database of urban area and population size, we show the variation in population densities and scaling relationships between urban area and population size across the spectrum of socioeconomic development on Earth. Support for UST expectations of ⅚ scaling exponents was mixed, with nearly half of countries exhibiting scaling indistinguishable from linear and a surprising substantial number of countries maintaining this apparent linearity and invariance of population density over three decades. Further research is thus needed to understand the interactions among economics, demography, history, and culture that may underlie these context-specific relationships. While our study involves a wealth of data, including hundreds of cities and scaling analysis up to 38 countries over 4 time periods, we have only focused on area and population. Additional variables must be investigated at these large cross-country and cross-city scales. Whether or not a single general theory can manage urban biodiversity given the variation in urban density scaling uncovered here remains an open question.
Data availability statement
The original contributions presented in the study are included in the article/Supplementary Material. Further inquiries can be directed to the corresponding author.
Author contributions
JB led the conceptualization, execution, analysis, discussion, and writing with input from all authors. JB, JO, IH, VW, MS, KL, TB, AC, XF, CH-H, AK contributed to data analysis, interpretation of results and discussion. All authors contributed to editing the article and approved the submitted version.
Funding
This project was supported by funding from the Bridging Biodiversity and Conservation Science Program of the Arizona Institutes of Resilience at the University of Arizona, Postdoctoral funding from Northeastern University, a Lyman T Johnson Diversity Fellowship from the University of Kentucky’s Graduate School, and a Postdoctoral Fellowship from Agencia Nacional de Investigación y Desarrollo de Chile ANID 3190609.
Conflict of interest
The authors declare that the research was conducted in the absence of any commercial or financial relationships that could be construed as a potential conflict of interest.
Publisher’s note
All claims expressed in this article are solely those of the authors and do not necessarily represent those of their affiliated organizations, or those of the publisher, the editors and the reviewers. Any product that may be evaluated in this article, or claim that may be made by its manufacturer, is not guaranteed or endorsed by the publisher.
Supplementary material
The Supplementary Material for this article can be found online at: https://www.frontiersin.org/articles/10.3389/fcosc.2022.879934/full#supplementary-material
Supplemental 1 | Scaling parameters of all countries over time. Countries that changed from linear to sublinear: Argentina, Australia, Bangladesh, Canada, China, Indonesia, South Korea, Spain, Turkey, United States, United Kingdom. Countries that stayed linear: Belgium, Colombia, Germany, Iran, Malaysia, Nigeria, Pakistan, Poland, Saudi Arabia, South Africa, Ukraine, Venezuela. Countries that stayed sublinear: Brazil, Egypt, France, India, Japan, Mexico, Russia, Taiwan, Uzbekistan. Countries that changed from superlinear to linear: Italy.
Appendix 1 | Summary OLS and RMA statistics of scalings of log area with log population for countries >5 cities of >100 km2 and >50,000 population, per time frame (N = 933 cities in 2015, 738 in 2000, 591 in 1990, 416 in 1975).
References
Acuto M., Parnell S., Seto K. C. (2018). Building a global urban science. Nat. Sustain 1, 2–4. doi: 10.1038/s41893-017-0013-9
Barnosky A. D., Hadly E. A., Bascompte J., Berlow E. L., Brown J. H., Fortelius M., et al. (2012). Approaching a state shift in earth’s biosphere. Nature 486, 52–58. doi: 10.1038/nature11018
Batty M., Ferguson P. (2011). Defining city size. Environ. Plann. B: Plann. Design 38 (5), 753–756. doi: 10.1068/b3805ed
Bettencourt L. M. A. (2013). The origins of scaling in cities. Science 340, 1438–1441. doi: 10.1126/science.1235823
Bettencourt L. M. A. (2020). Urban growth and the emergent statistics of cities. Sci. Adv. 6, eaat8812. doi: 10.1126/sciadv.aat8812
Bettencourt L. M. A. (2021). Introduction to urban science: Evidence and theory of cities as complex systems (Boston, MA: MIT Press).
Bettencourt L. M. A., Lobo J., Helbing D., Kuhnert C., West G. B. (2007). Growth, innovation, scaling, and the pace of life in cities. Proc. Natl. Acad. Sci. 104, 7301–7306. doi: 10.1073/pnas.0610172104
Bettencourt L., West G. (2010). A unified theory of urban living. Nature 467, 912–913. doi: 10.1038/467912a
Bettencourt L. M. A., Yang V. C., Lobo J., Kempes C. P., Rybski D., Hamilton M. J. (2020). The interpretation of urban scaling analysis in time. J. R. Soc Interface 172019084620190846, 1–9. doi: 10.1098/rsif.2019.0846
Brown J. H. (2014). Why are there so many species in the tropics? J. Biogeogr. 41, 8–22. doi: 10.1111/jbi.12228
Brown J. H., Burnside W. R., Davidson A. D., DeLong J. P., Dunn W. C., Hamilton M. J., et al. (2011). Energetic limits to economic growth. BioScience 61, 19–26. doi: 10.1525/bio.2011.61.1.7
Burger J. R. (2019). Fueling fertility is emptying the planet’s resources. BioScience 69, 757–758. doi: 10.1093/biosci/biz084
Burger J. R., Allen C. D., Brown J. H., Burnside W. R., Davidson A. D., Fristoe T. S., et al. (2012). The macroecology of sustainability. PLoS Biol. 10, e1001345. doi: 10.1371/journal.pbio.1001345
Burger J. R., Brown J. H., Day J. W., Flanagan T. P., Roy E. D. (2019). The central role of energy in the urban transition: Global challenges for sustainability. Biophys. Econ Resour Qual 4, 5. doi: 10.1007/s41247-019-0053-z
Burger J. R., Fristoe T. S. (2018). Hunter-gatherer populations inform modern ecology. PNAS 115, 1137–1139. doi: 10.1073/pnas.1721726115
Burger J. R., Weinberger V. P., Marquet P. A. (2017). Extra-metabolic energy use and the rise in human hyper-density. Sci. Rep. 7, 43869. doi: 10.1038/srep43869
Callaghan C., Poore A., Major R., Cornwell W., Wilshire J., Lyons M. (2021). How to build a biodiverse city: environmental determinants of bird diversity within and among 1581 cities. Biodiver Conserv. 30, 217–234. doi: 10.1007/s10531-020-02088-1
Ceballos G., Ehrlich A. H., Ehrlich P. R. (2015). The annihilation of nature: Human extinction of birds and mammals (Baltimore, MD: JHU Press).
DeLong J. P., Burger O., Hamilton M. J. (2010). Current demographics suggest future energy supplies will be inadequate to slow human population growth. PloS One 5 (10), e13206. doi: 10.1371/journal.pone.0013206
Dijkstra L., Poelman H. (2014). “Regional working paper,” in A harmonised definition of cities and rural areas: the new degree of urbanisation (European Comission Directorate-General for Regional and Urban Policy: Working Paper). Available at: https://ec.europa.eu/regional_policy/sources/docgener/work/2014_01_new_urban.pdf.
Dunn R., Burger J., Carlen E., Koltz A., Light J., Martin R., et al. (2022). A theory of city biogeography and the origin of urban species. Front. Conserv. Sci. 3. doi: 10.3389/fcosc.2022.761449
Fattorini S., Lin G., Mantoni C. (2018). Avian species–area relationships indicate that towns are not different from natural areas. Environ. Conserv. 45, 419–424. doi: 10.1017/S0376892918000048
Ferenc M., Sedláček O., Mourková J., Exnerová A., Škopek J., Formánek J., et al. (2016). Disentangling the influences of habitat availability, heterogeneity and spatial position on the species richness and rarity of urban bird communities in a central European city. Urban Ecosyst. 19, 1265–1281. doi: 10.1007/s11252-016-0558-9
Finance O., Swerts E. (2020). “Scaling laws in urban geography. linkages with urban theories, challenges and limitations,” in Theories and models of urbanization. lecture notes in morphogenesis. Ed. Pumain D. (Cham: Springer).doi: 10.1007/978-3-030-36656-8_5.
Florczyk A. J., Corbane C., Ehrlich D., Freire S., Kemper T., Maffenini L., et al. (2019) GHSL data package 2019: public release GHS P2019. Available at: https://op.europa.eu/publication/manifestation_identifier/PUB_KJ1A29788ENN.
Fragkias M., Lobo J., Strumsky D., Seto K. C. (2013). Does size matter? scaling of CO2 emissions and U.S. urban areas. PLoS One 8, e64727. doi: 10.1371/journal.pone.0064727
Fuller R. A., Gaston K. J. (2009). The scaling of green space coverage in European cities. Biol. Lett. 5, 352–355. doi: 10.1098/rsbl.2009.0010
Guernier V., Hochberg ME., Guégan JF. (2004). Ecology Drives the Worldwide Distribution of Human Diseases. PLOS Biology 2(6):e141. doi: 10.1371/journal.pbio.0020141
Gudipudi R., Rybski D., Lüdeke M. K., Kropp J. P. (2019). Urban emission scaling — research insights and a way forward. Environ. Plann. B: Urban Analytics City Sci. 46, 1678–1683. doi: 10.1177/2399808319825867
Hidalgo C. A. (2009) The dynamics of economic complexity and the product space over a 42 year period. Available at: https://dash.harvard.edu/handle/1/37366208.
Krausmann F., Fischer-Kowalski M., Schandl H., Eisenmenger N. (2008). The global sociometabolic transition. J. Ind. Ecol. 12, 637–656. doi: 10.1111/j.1530-9290.2008.00065.x
Lobo J., Bettencourt L., Smith M., Ortman S. (2019). Settlement scaling theory: Bridging the study of ancient and contemporary urban systems. SSRN Electronic J. 6, 1–29. doi: 10.2139/ssrn.3427336
Melchiorri M. (2022). The global human settlement layer sets a new standard for global urban data reporting with the urban centre database. Front. Environ. Sci. doi: 10.3389/fenvs.2022.1003862
Meyfroidt P., Noordwijk M., Minang P. A., Dewi S., Lambin E. F. (2011) Drivers and consequences of tropical forest transitions: options to bypass land degradation? Available at: https://cgspace.cgiar.org/handle/10568/42016.
Moses M. E., Brown J. H. (2003). Allometry of human fertility and energy use. Ecol. Lett. 6, 295–300. doi: 10.1046/j.1461-0248.2003.00446.x
Murthy A. C., Fristoe T. S., Burger J. R. (2016). Homogenizing effects of cities on north American winter bird diversity. Ecosphere 7, e01216. doi: 10.1002/ecs2.1216
Oliveira E. A., Andrade J. S., Makse H. A. (2014). Large Cities are less green. Sci. Rep. 4, 4235. doi: 10.1038/srep04235
Ortman S. G., Lobo J. (2020). Smithian gowth in a non-industrial society. Sci. Advances 6 (25), eaba5694. doi: 10.1126/sciadv.aba5694
Ortman S. G., Lobo J., Smith M. E. (2020). Cities: Complexity, theory and history. PLoS One 15, e0243621. doi: 10.1371/journal.pone.0243621
Pesaresi M., Florczyk A., Schiavina M., Melchiorri M., Maffenini L. (2019). GHS-SMOD R2019A - GHS settlement layers, updated and refined REGIO model 2014 in application to GHS-BUILT R2018A and GHS-POP R2019A, multitemporal (1975-1990-2000-2015). doi: 10.2905/42E8BE89-54FF-464E-BE7B-BF9E64DA5218
Rees W. E., Wackernagel. M. (1996). “Urban ecological footprints: Why cities cannot be sustainable–and why they are a key to sustainability,” in Our ecological footprint, reducing human impact on the earth. Eds. Wackernagel M., Rees W. E. (Gabriola Island: New Society Publishers), 223–248.
Ribeiro H. V., Sunahara A. S., Sutton J., Perc M., Hanley Q. S. (2020). City size and the spreading of COVID-19 in Brazil. PLoS One 15 (9), e0239699. doi: 10.1371/journal.pone.0239699
Schramski J. R., Gattie D. K., Brown J. H. (2015). Human domination of the biosphere: Rapid discharge of the earth-space battery foretells the future of humankind. PNAS 112, 9511–9517. doi: 10.1073/pnas.1508353112
Schramski J. R., Woodson C. B., Brown J. H. (2020). Energy use and the sustainability of intensifying food production. Nat. Sustain 3, 257–259. doi: 10.1038/s41893-020-0503-z
Schramski J. R., Woodson C. B., Steck G., Munn D., Brown J. H. (2019). Declining country-level food self-sufficiency suggests future food insecurities. Biophys. Econ Resour Qual 4, 12. doi: 10.1007/s41247-019-0060-0
Steffen W., Rockström J., Richardson K., Lenton T. M., Folke C., Liverman D., et al. (2018). Trajectories of the earth system in the anthropocene. PNAS 115, 8252–8259. doi: 10.1073/pnas.1810141115
Stier A., Berman M. G., Bettencourt L. (2020). COVID-19 attack rate increases with city size (Mansueto Institute for Urban Innovation Research Paper No. 19). Available at: https://ssrn.com/abstract=3564464.
Uchida K., Blakey R. V., Burger J. R., Cooper D. S., Niesner C. A., Blumstein D. T. (2021). Urban biodiversity and the importance of scale. Trends Ecol. Evol. 36, 123–131. doi: 10.1016/j.tree.2020.10.011
Keywords: allometry, cities, complex systems, urban policy, macroecology, macroeconomics, urban ecology, urban sustainability
Citation: Burger JR, Okie JG, Hatton IA, Weinberger VP, Shrestha M, Liedtke KJ, Be T, Cruz AR, Feng X, Hinojo-Hinojo C, Kibria ASMG, Ernst KC and Enquist BJ (2022) Global city densities: Re-examining urban scaling theory. Front. Conserv. Sci. 3:879934. doi: 10.3389/fcosc.2022.879934
Received: 20 February 2022; Accepted: 11 November 2022;
Published: 05 December 2022.
Edited by:
Rachel Vera Blakey, University of California, Los Angeles, United StatesReviewed by:
Haroldo V. Ribeiro, State University of Maringá, BrazilDave Kendal, University of Tasmania, Australia
Copyright © 2022 Burger, Okie, Hatton, Weinberger, Shrestha, Liedtke, Be, Cruz, Feng, Hinojo-Hinojo, Kibria, Ernst and Enquist. This is an open-access article distributed under the terms of the Creative Commons Attribution License (CC BY). The use, distribution or reproduction in other forums is permitted, provided the original author(s) and the copyright owner(s) are credited and that the original publication in this journal is cited, in accordance with accepted academic practice. No use, distribution or reproduction is permitted which does not comply with these terms.
*Correspondence: Joseph R. Burger, robbieburger@uky.edu
†ORCID: Joseph R. Burger, orcid.org/0000-0002-7361-3858