- 1Department of Biology, Ecology and Biodiversity Research Group, Vrije Universiteit Brussel, Brussels, Belgium
- 2Department of Plant Science and Landscape Architecture, University of Maryland, College Park, MD, United States
- 3Department of Entomology, University of Maryland, College Park, MD, United States
Dispersal plays a crucial role in the connectivity of established mangrove populations and in species range dynamics. As species ranges shift in response to climate change, range expansions can occur from incremental short-distance dispersal events and from stochastic long-distance dispersal events. Most population genetic research dealt with historically accumulated events though evidence of actual propagule dispersal allows to estimate genotypic features and origin of founders. In this study, we aim to disentangle a contemporary dispersal event. Using microsatellite markers, we genotyped 60 Rhizophora racemosa drift propagules obtained on a bare unforested coastal area in southern Cameroon, estimated their relationship to 109 adult trees from most proximate sites (which were 3–85 km away), and assessed their relative difference with 873 trees of major mangrove areas (> 300 km) along the Cameroonian coastline. Proximate mangrove populations were considered as potential source populations in assignment tests. However, drift propagules could not be assigned to any of the Cameroonian mangrove sites and were genetically isolated from Cameroonian populations. Drift propagules showed higher levels of genetic diversity and private alleles giving a higher relatedness to each other than to any putative source population. Chloroplast sequences were used to confirm the identity of drift propagules as R. racemosa. We postulate that a complex interaction of ocean currents, estuarine geomorphology, and tidal patterns explain drift propagule dispersal to an area. Most likely the investigated cohort of propagules originated from more southern mangrove areas of the West African range beyond the Cameroonian border. This study unraveled the allelic, genetic, and genotypic features of stranded propagules following a stochastic long-distance dispersal. Transboundary dispersal of these propagules highlights the need for intergovernmental efforts in the management of biodiversity.
Introduction
The seafaring propagules of mangroves depend on local hydrodynamics and ocean currents for both short- and long-distance dispersal. Evidence of historical long-distance trans-oceanic dispersal are accumulating based on signatures of genetic markers (Takayama et al., 2013, 2021; Lo et al., 2014; Wee et al., 2020). Yet, assessing real-time dispersal events in mangroves is difficult because of the challenges of tracking drifting propagules particularly over large-scale and dynamic seascapes (Van der Stocken et al., 2019b). Additionally, the difficulty of quantifying the number of candidate dispersers, owing to how much fecundity and depredation pressure varies across space and time (Van der Stocken et al., 2019b), further compounds the challenge. Such real-time dispersal events are relevant for understanding patterns and spatial scales of connectivity and range dynamics of species. To overcome the challenges, numerical dispersal models have proven very useful to elucidate the spatial extent and patterns of dispersal in mangroves at regional (Ngeve et al., 2016) and continental or transoceanic scales (Van der Stocken et al., 2019a); but they are not without limitations (Van der Stocken et al., 2019b). At much smaller spatial scales, dispersal experiments are feasible (Van der Stocken et al., 2015; Ngeve et al., 2017b), although labor-intensive. More recently, by employing a citizen science approach, propagules caught in fishing nets over more than 2 years, have also revealed dispersal patterns of mangrove propagules (Van der Stocken et al., 2018). One phenomenon unifies all these methods: a leptokurtic dispersal kernel describing patterns of mangrove propagule dispersal (Van der Stocken et al., 2019b), where the number of short-distance dispersal (SDD) events far exceeds the number of long-distance dispersal (LDD) events which occur rarely (see review by Van der Stocken et al., 2019b). Yet, LDD events are crucial; e.g., ancient LDD and vicariance explain contemporary distribution of mangrove genera (e.g., Triest, 2008; Lo et al., 2014; Takayama et al., 2021; Triest and Van der Stocken, 2021; Triest et al., 2021a,c). However, more frequent short distance dispersal events are notable for maintaining the connectivity of populations at regional to fine spatial scales (Ngeve et al., 2017a,b; Triest et al., 2020, 2021a), and contributing to range expansions (Saintilan et al., 2014; Giri and Long, 2016; Kennedy et al., 2020; Triest et al., 2021b).
Regardless of whether dispersal is long- or short-distanced, the scale over which these terminologies are used is usually ambiguous. Developing predictive dispersal models is difficult because between systems, short- and long-distance labels are used inconsistently. We will use the term long-distance dispersal (LDD) to mean dispersal long enough to stretch beyond an individual's genetic neighborhood and outside of the geographic limit of source stand, i.e., strict-sense long-distance dispersal sensu Jordano (2017). Dispersal within a population's geographical limit but outside its genetic neighborhood, and vice versa could also be termed LDD (see Jordano, 2017), but we will stick with the “strict-sense” definition for this paper. Apart from the terminology, dispersal potential varies among different mangrove species (Van der Stocken et al., 2019b). Such differences in dispersal potential among species have strong implications for patterns of genetic connectivity and the spatial scale over which dispersal occurs (Mori et al., 2015; Do et al., 2019; Wee et al., 2020). For example, higher dispersal potential in Rhizophora mucronata and Bruguiera gymnorrhiza allowed for genetic connectivity over larger geographical scales than Avicennia alba and Sonneratia alba (Wee et al., 2020).
The spatial scale over which pollen dispersal occurs in many mangrove species is usually shorter than seedling (propagule) dispersal (e.g., Wee et al., 2015; Ngeve et al., 2020). As a result, mangrove species mainly depend on propagule dispersal for connectivity among populations, both close by and remote. For such dispersal to be effective, propagules must have access to the dispersal vector (water in the case of mangroves), emigrate from their site of origin while being transported by the vector, immigrate to /establish at a new site, and contribute to the genetic pool of that new site. Hydrological accessibility varies per mangrove assemblage in the zonation patterns along the intertidal, i.e., landward vs. seaward stands do not usually have equal accessibility to water. This difference in hydrological accessibility has consequences on dispersal and leaves imprints on patterns of fine-scale genetic structure (Triest et al., 2020; Triest and Van der Stocken, 2021). Besides mere hydrological accessibility, flood levels also determine whether a propagule overcomes the first barrier in their landscape matrix of their origin (e.g., tree density, dense prop root network, root barrier types because of species composition). Propagules must first escape their forest matrix of origin before embarking on potential LDD (Van der Stocken et al., 2018, 2019b). Meanwhile before this, several propagules suffer depredation and pre-dispersal mortality, thereby reducing the number of candidate propagules for LDD (Van der Stocken et al., 2019b). For viable propagules that make it to the open waters, local hydrodynamic forces and oceanic currents drive dispersal patterns (passive dispersal). Dispersing propagules must remain within their window of biological activity in order to root and establish at suitable habitats (Van der Stocken et al., 2015, 2019b) (effective dispersal entails successful establishment). Yet, upon arrival at a potentially suitable site, propagules must also surpass thresholds of rapid root development and anchorage to withstand dislodgement from high hydrodynamic forces (Balke et al., 2011), and escape post-dispersal depredation (Van der Stocken et al., 2019b). High wave energy has a stronger impact on seedling establishment especially at bare areas where pneumatophores of adult mangrove plants are lacking (Huisman et al., 2009). There also exists the possibility that some propagules regain buoyancy after an initial “settlement” and embark on secondary dispersal (Van der Stocken et al., 2019b). Therefore, many factors can affect effective dispersal patterns and the spatial extent over which it occurs in mangroves.
Since all these factors affect patterns of effective propagule dispersal, only a proportion of all propagules produced by a mangrove population/plant contributes to effective dispersal. Yet, evidence of transboundary dispersal, i.e., dispersal among populations in different countries, are accumulating, based on genetic (e.g., Hodel et al., 2018; Triest et al., 2021c,d) and modeling studies (e.g., Van der Stocken and Menemenlis, 2017; Van der Stocken et al., 2019a). Mangroves occur across large stretches of subtropical and tropical coastlines of over 120 countries (Duke et al., 2007); and several studies assessing dispersal patterns have focused either on specific countries or region (except for modeling studies (e.g., Di Nitto et al., 2013; Van der Stocken and Menemenlis, 2017; Van der Stocken et al., 2019a). Therefore, most evidence of transboundary dispersal are based on an accumulated historically realized gene flow (effective dispersal) more than on tracking of actual propagule dispersal events. Relying only on patterns of effective dispersal from genetic signatures obscures our ability to estimate all actual dispersal events. Yet, it is important to study actual dispersal patterns because they are informative for understanding what drives spatial limits of dispersal and ranges of species. For example, whether limits are driven by species dispersal potential (viability, floatability, size) vs. environmental constraints (ocean current patterns, eddies, habitat suitability).
The small dimensions of most field experiments (e.g., Van der Stocken et al., 2015; Ngeve et al., 2017b) limit the wide applicability of capture-release experiments at larger scales. As such, tracking actual LDD events, although important, is impossible in real-time. Often their realized effects, quantifiable using genomic markers, are only possible after a considerable time lag between actual dispersal events and growth of established individuals.
The large propagules of Rhizophora spp have a higher tendency (compared to propagules of other mangrove species e.g., Avicennia spp.) to be trapped by the dense prop root system of the same species, at their origin (Van der Stocken et al., 2015), favoring SDD. At the same time, Rhizophora propagules are among the best floaters with long viability; a period between 80 and 365 days has been reported, depending on the species (Van der Stocken et al., 2019b), offering LDD potential. Many mangrove propagules can travel over considerable long distances under favorable hydrodynamic conditions; many propagules get lost at sea, or get washed ashore to sites where they are unable to establish (pers. obs., also see De Ryck et al., 2016); while others arrive at suitable sites to either connect existing patches or ultimately expand species range. Previous research on R. racemosa from West Africa indicated that propagule dispersal allowed for a genetic connectivity of two major mangrove estuaries (Rio del Rey and Cameroon Estuary complex) through dispersal corridors that were formed after rising sea levels submerged lowlands of the Cameroon Volcanic Line (Ngeve et al., 2016). Additionally, virtual propagule dispersal modeling in congruence with genetic data from R. racemosa sampled from the entire Cameroonian coastline revealed an oceanic front, formed by two converging currents offshore the CEC, presents a significant contemporary barrier to regional scale genetic connectivity (Ngeve et al., 2016). This ocean current front isolates the more southerly estuaries, Lokoundje (Kribi) and Ntem (Campo) from the other mangrove estuaries along the Cameroonian coastline. Despite many population genetic studies that describe historically accumulated events, any further genetic evidence of actual dispersal events in mangroves are welcomed as truly novel information on the dynamics and extent of propagule dispersal. In this paper, we surveyed two non-mangrove forested sandy beach areas in the Ntem Estuary (Campo, southern Cameroon) for Rhizophora racemosa propagules with the goal of (1) identifying and quantifying drift propagules, (2) assessing potential for their establishment, and (3) determining their populations of origin. We hypothesize that drift propagules come from the closest mangrove forest patches (Campo, then Kribi). Our analyses are based on a dataset on nine microsatellite loci from both drift propagules and potential source populations along the Cameroonian coastline.
Materials and Methods
Study Species and Study Area
Mangroves of the Rhizophora genus have a pantropical distribution (Duke, 1995; Tomlinson, 2016; Takayama et al., 2021), abundant and often predominant in both the Atlantic East Pacific (AEP) and Indo-West Pacific (IWP) Biogeographic regions (sensu Spalding, 2010). Four Rhizophora species can be found in the AEP region, but only two of these occur along the eastern Atlantic coastline (see Takayama et al., 2021)—R. mangle and R. racemosa, and their hybrid R.x harrisonii. The distribution of R. racemosa within the AEP, along estuaries and bays, can be explained by its preference for areas with relatively higher freshwater input as it tends to inhabit more brackish waters than the other congeners (Duke and Allen, 2006).
In Cameroon, Rhizophora racemosa makes up 90% of mangrove extent, accompanied by Avicennia germinans (5%), and six other sparsely distributed species (Corcoran et al., 2007). R. racemosa is most preferred by local people for fuel and construction wood; as a result, its coverage along the Cameroonian coastline has declined enormously (Nfotabong-Atheull et al., 2013). Amidst challenges from excessive logging, sea-level rise, and other direct and indirect anthropogenic effects, understanding how this species extent is shifting, its potential for colonization of new sites or recovery through replenishing depauperate populations is critical.
The Cameroonian coastline (~400 km) has third largest (~2,000 km2) mangrove cover in West Africa, after Nigeria (~7,300 km2) and Guinea Bissau (~3,000 km2) (Corcoran et al., 2007). Mangroves in Cameroon occur in 4 main estuaries. The northernmost Rio Del Rey Estuary (RDR) (close to the Nigerian border) boasts the most extensive and more pristine mangrove cover. The more centrally located Cameroon Estuary complex (CEC) is also large, but is the most disturbed mangrove area because of urban development and increasing human population from rural exodus, as well as other natural and anthropogenic factors (Nfotabong-Atheull et al., 2013; Din et al., 2017). The southerly estuaries, Lokoundje (Kribi) and Ntem (Campo), which are separated by about 85 km, are naturally smaller mangrove areas.
The Ntem River acts as a natural boundary between Cameroon and mainland Equatorial Guinea (Figure 1), characterized by semidiurnal tidal system of an average 1.8 m tidal amplitude (Ondoa et al., 2018). Mangroves near the Ntem Estuary are the smallest, in terms of spatial extent, in Cameroon; occurring around the mouth of the Campo River (which empties into the Ntem River) and at Ipono, in the Campo Ma'an National Park. These small mangrove forest patches are surrounded landward by extensive forests, and by bare long beach stretches (with no mangroves) (Figure 1). Some of these areas are not suitable for mangrove establishment, because of the sandy soil.
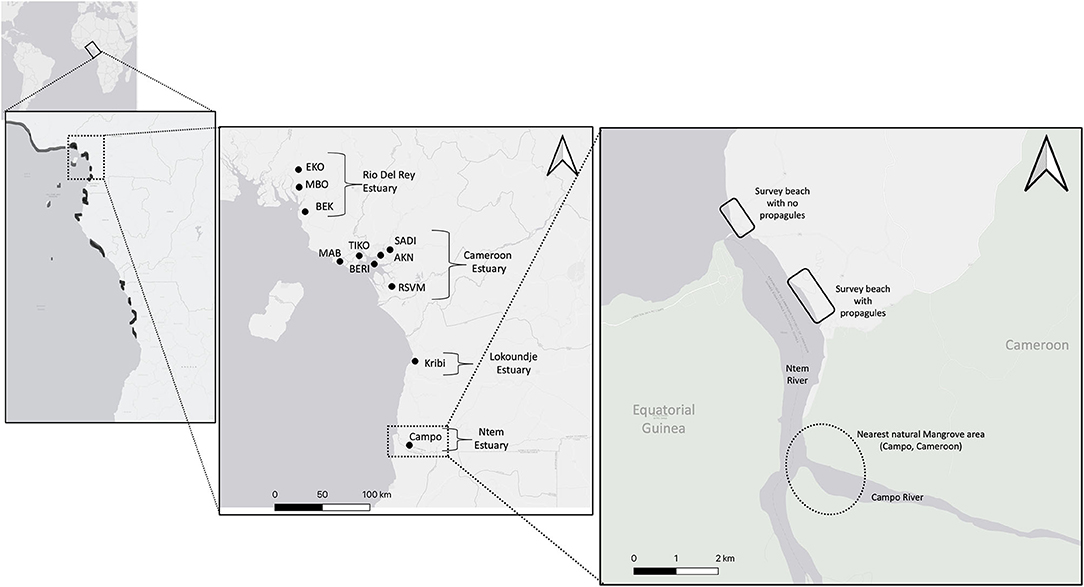
Figure 1. Map of the Cameroonian Coastline, showing our extensive sampling from North to south at all 4 mangrove estuaries (from north to south: Rio Del Rey, Cameroon Estuary, Lokoundje, and Ntem). On the left the black markings along the coastline show the mangrove estuaries. Zoomed at the Ntem Estuaries to show the survey beaches, which were ~2 km apart and about a square kilometer area each. Base map generated from ESRI Gray (light), CRS:3857 in QGIS version 3.10.0-A Coruña.
For comparative purposes, we included materials and microsatellite genotypes from Ngeve et al. (2016, 2017a,b); Ngeve et al. (2020) (details below) and Figure 1 shows in detail all sites within all estuaries from which we had samples: from Ekondo Titi (EKO), Mbongo (MBO), Bekumu (BEK) in the Rio del Rey Estuary in the north; Mabeta (MAB), Tiko (TIK), Douala-Bonamoussadi (SADI), Douala—Akwa-Nord (AKN), Douala—Bonaberi (BERI), Mouanko at the Douala-Edea National Park (RSVM) in the central Cameroon Estuary complex; Kribi in the Lokoundje Estuary; and Campo in the Ntem Estuary. Hereafter, the Rio Del Rey samples are all referred to as RDR, the Cameroon Estuary complex samples as CEC, the Lokoundje Estuary as Kribi, and the Ntem Estuary as Campo, while the cohort of drift propagules are referred to as Drift.
Beach Surveys Around the Ntem River Estuary and Collection of Propagules
During a field expedition in January of 2015, we surveyed two beach areas that each stretched ~2 km where we could (1) identify the different mangrove propagules dominating this stretch of coastline; (2) assess whether mangroves could expand into this area by checking for vagrant establishing mangrove juveniles; (3) assess the state (interpreted as viability) of propagules found here, whether structurally damaged or intact; and (4) to sample drift propagules for further genotyping, to determine genetic make-up and geographic origin. We photographed all types of propagules observed and collected a total of 77 Rhizophora racemosa propagules for genetic analyses in the laboratory. Samples were washed, sun dried, and transported to the Vrije Universiteit Brussel, Belgium.
During the same field effort, we also sampled plant material for genetic analyses in 52 R. racemosa adult trees at Ntem River Estuary, which is the closest (~ 3–6 km) mangrove area to the bare beach areas (Campo). Also, about 85 km north from this area, we sampled 57 trees from the Lokoundje Estuary (Kribi). This way, sample sizes were nearly equal for the “Drift” population and of the adults from populations in close vicinity. The plant material from these adult trees, along with plant material previously described (Ngeve et al., 2016, 2017a,b), for another 873 trees from the Cameroonian coastline, made up of sites from the CEC and the RDR estuaries, further northwards > 300 km along the Cameroonian coastline, were partially reconsidered in this study only to assess relative differences with drift propagules and in assignment tests.
DNA Extraction and Genotyping
Genomic DNA was extracted from the outer layer of the hypocotyl of all 77 propagules. The outer layer was the tissue of choice to obtain material for DNA extraction since it would be the part with the highest yield of the seedling's DNA; yet for several extractions, DNA yield was very low due to interfering effects of secondary metabolites (e.g., Triest, 2008). Extraction was done using the E.Z.N.A SP PLANT DNA kit. The protocol used for PCR amplification is the same used in our earlier studies using samples from adult trees (Ngeve et al., 2016, 2017b), only this time, using a multiplex of nine microsatellite primers (Rrace 1, Rrace 3, Rrace 6, Rrace 5, Rrace 7, Rrace 12, Rrace 18, Rrace 20, Rrace 24). These primers had been initially developed for this species (Ngeve et al., 2017b). Amplified fragments were genotyped by Macrogen (Seoul, S. Korea). GeneMarker (SoftGenetics LLC, State College, USA) was used to score peaks of the fragments. Of the 77 propagules, we used only 60 hypocotyl samples that could be genotyped confidently in subsequent analyses. Due to low yield of DNA in some samples, fragment amplification resulted in weak peaks, thus preventing unambiguous calling alleles.
Data Analyses
Measures of Genetic Diversity and Structure
To assess whether the drift propagules were made of a genetically diverse pool of propagules, we summarized and compared measures of genetic diversity between drift propagules and the closest mangrove populations (Campo and Kribi) by assuming the drift propagule pool as a single population (Drift). Average number of alleles (At), number of effective alleles (Ae), observed (Ho) and expected (He) heterozygosity, and percentage polymorphic loci (%P) were calculated in GenAlEx (Peakall and Smouse, 2012). Inbreeding coefficient (FIS), Allelic richness (Ar) rarified to the smallest population size (k 52) was done in FSTAT (Goudet, 1995).
To visualize whether the drift propagules bring any new alleles to this area or have alleles identical to those of close by populations, we estimated and plotted the allele frequency of all alleles across all loci using GenAlex; and highlighted all private alleles in the three “populations” (Kribi, Campo, Drift propagules). A list of private alleles and allele frequencies was summarized in GenAlex.
Relatedness and Population Structure
To investigate whether the drift propagules were products of an outcrossing or inbreeding, we calculated multilocus outcrossing rates (t), using, Wright's (1984) within population inbreeding coefficient, based on the equation: t = (1 – FIS)/(1 + FIS) (also see Yan et al., 2016). We estimated the selfing rate and kinship coefficient (FIJ; Loiselle et al., 1995), of individuals within each of three populations as well as kinship coefficient of individuals between sites using SPAGeDi (Hardy and Vekemans, 2002), to assess whether drift propagules originated from a single genetic population (propagule pool) or from a group of diverse pool populations (migrant pool). Selfing rates were estimated based on standardized identity disequilibrium which assumes a mixed mating model, and computed standard error estimates by jackknifing over loci (as described in Hardy, 2016). Assuming mixed mating system is appropriate based on previous studies in R. racemosa (Ngeve et al., 2020). For all SPAGeDi analyses, we estimated significance based on 10,000 permutations, and reference allele frequencies used for computing pairwise statistics were from all three sites—Kribi, Campo, and Drift. Additionally, we estimated mean pairwise within-population relatedness in GenAlEx, based on an individual level genetic distance matrix, with 9,999 permutations and a bootstrap value of 10,000.
Based on the prevailing ocean current patterns and genetic signatures (Ngeve et al., 2016), we hypothesized that high dispersal among sites within the same ocean currents, but not among sites between ocean currents. Implying the central (CEC) and northern (RDR) estuaries are unlikely to be the source of drift propagules obtained at Campo. However, Kribi and Campo sites could be potential sources of these propagules or belong to the same gene pool/genetic neighborhood. Moreover, to evaluate the relative differences between these drift propagules and adults from all areas along the Cameroonian coastline, we considered these and previously published data set in multivariate analyses [discriminant analysis of principal components (DAPC)], as described below. To investigate the level of differentiation between these drift propagules and nearby forest areas, as well as the other forest areas along the Cameroonian coastline, we calculated pairwise F′ST (Meirmans and Hedrick, 2011) and DEST (Jost, 2008) in GenAlEx.
We performed a multivariate clustering analyses of samples using DAPC (Jombart et al., 2010) to visualize genetic structure of the drift propagules with respect to the closest mangrove areas to their sampled location, as well as with respect to other adult stands along the Cameroonian coastline. These analyses were done with original site data, using Adegenet (Jombart, 2008) in R version 4.0.4 (R Core Team, 2019). For DAPC, we chose to set the number of retained principal components to 12 (Kribi, Campo, and Drift only) and 23 (Drift and all sites), and the number of clusters kept were 2 and 4, respectively. These settings were confirmed following an alpha-score assessment, and all analyses were done based on the samples. In addition to the DAPC, we estimated the probability of assignment for every individual (from Kribi, Campo and Drift) using the compoplot function of Adegenet.
Bayesian clustering analyses in Structure (Pritchard et al., 2000) was also performed to determine to which genetic cluster(s) individuals from Kribi, Campo, and the drift propagules (Drift) were assignable. For Structure analyses, we started with an initial burn in of 100,000 before 1,000,000 Markov Chain Monte Carlo repeats. We tested 5 different K's with 10 iterations for each K. The online program Structure Harvester (Earl and vonHoldt, 2012) was used to estimate the best K value based on the Evanno et al. (2005) method of highest change in K value. After which we plotted the genetic clusters of all individuals using the online program Clumpak—Cluster Markov Packager Across K (Kopelman et al., 2015).
Assignment Tests and Species Identification
Before performing actual assignment tests, we first explored the resolution of our markers for this purpose by using progeny arrays originally genotyped for the same loci (Ngeve et al., 2020), to assess the power of the markers to validate known origin of progenies, similar to Kennedy et al. (2020). Secondly, we used the population assignment option in GenAlex to explore our actual data with drift propagules, to see where they plot with samples from closest mangrove areas (Kribi and Campo) as well as others in RDR and CEC. For these analyses, we used default allele frequency threshold of 0.01, and used the leave one out option which corrects for any bias in allele frequency estimations. The plots from this population assignments tests in GenAlex are usually informative for data exploration purposes (Blyton and Flanagan, 2012).
Two sets of population assignment tests were done to determine the origin of these drift propagules. Firstly, we used just the hypothesized source populations, which were the closest mangrove areas, Kribi and Campo. Secondly, to confirm the relative difference between these propagules and the other two northerly estuaries of the Cameroonian coastline (RDR and CEC), we did another assignment test that included samples from these areas, although the chances of these propagules originating from these estuaries was low because of prevailing ocean currents (see Ngeve et al., 2016). We calculated the probability that each drift propagule originated from any of the sampled sites along the Cameroonian coastline using GeneClass2 (Piry et al., 2004). These assignment tests were based on the allele frequencies of each putative source population, following 10,000 resampling with the Monte Carlo simulation of Paetkau et al. (2004), with Type I alpha of 0.05, and using all nine loci. Assignment threshold scores was set at 0.05.
In addition, we determined whether any of the drift propagules were first-generation migrants from the nearby proximate mangrove sites—Kribi and Campo. For this analysis, we used the allele frequency method of assignment by Paetkau et al. (2004) to calculate the log-likelihood of the origin of drift propagules; and still using all nine loci, using GeneClass2. This test is supposed to identify individuals with a significantly high probability of originating from the Kribi and Campo sites. We used the L_home statistic (log likelihood of originating from the propagule pool vs. any of the putative source sites) with 10,000 Monte Carlo permutations (Paetkau et al., 2004) to test significance probability at p = 0.05. The L_home is an appropriate statistic when all reference populations have not been sampled. All tests in GeneClass2 were based on the default frequency for missing alleles (0.01).
To rule out a different species identity of the drift propagules, one sample of each Cameroonian population, in addition to a pool sample of six “drift” propagules, was used for species identification and a comparative analysis of chloroplast sequences. After passing quality inspection (DNA concentration between 5 and 15 ng/ul), the constructed library (TruSeq Nano DNA Kit) was sequenced by 300 bp × 2 paired-end sequencing in an Illumina MiSeq platform (Macrogen, Seoul, South Korea). Raw data were used for de novo chloroplast assembles of several genes/introns from a nearly complete genome. All assemblies used MK070169 (R. stylosa) as a reference genome (similar as done in Triest et al., 2021b) and the mapping of reads of Cameroonian samples were performed in Geneious Prime v 2019.2.1 (© 2005–2019 Biomatters Ltd.) (https://www.geneious.com). The assemblages averaged 42,445 to 80,347 reads with a mean depth of reads ranging from 77 to 146 coverage.
The de novo targeted chloroplast assemblies were processed against the species R. racemosa as a reference for the rbcL gene (Genbank accession number AB668365.1; 1371 bp), the rpl16 gene (AB668349.1; 895 bp), the trnS-trnG intron (AB668333.1; 481 bp) and the atpB-rbcL intergenic spacer (AB668317.1; 710bp) representing haplotype H from Ankobra in Ghana, West Africa as defined by Takayama et al. (2013); against the species R. mangle as a reference for the rbcL gene (AB668356.1; 1371 bp), the rpl16 gene (AB668340.1; 972 bp), the trnS-trnG intron (AB668324.1; 479 bp) and the atpB-rbcL intergenic spacer (AB668308.1; 705bp) representing haplotype A from Galeta in Panama, West Atlantic Coast (Takayama et al., 2013); against the species R. mucronata as a reference for the rbcL gene (AB668370.1; 1371 bp), the rpl16 gene (AB668354.1; 923 bp), the trnS-trnG intron (AB668338.1; 458 bp) and the atpB-rbcL intergenic spacer (AB668322.1; 699bp) representing haplotype K from Maheburg in Mauritius, Western Indian Ocean (Takayama et al., 2013). The consensus sequences of each gene/intron were aligned with ClustalW (Thompson et al., 1994).
A part of the nuclear ribosomal cistron (18S, ITS1, 5.8S, ITS2 and 26S) was assembled from a Rhizophora mucronata [KJ194220.1 (Lo et al., 2014)] 639 bp sequence containing an internal transcribed spacer 1 (partial sequence), 5.8S ribosomal RNA gene (complete sequence) and internal transcribed spacer 2 (partial sequence) and was subsequently used as a seed in Geneious Prime v 2019.2.1 to obtain the ITS1+ITS2 region of the same Cameroon samples as used for cpDNA sequencing. A 627bp long sequence, averaging 2,469–7,732 reads, was obtained with a mean depth of reads ranging from 388 to 962 coverage. The overlapping reads were consistent and did not show any sign of heterozygosity or hybridization. The generated consensus sequences of 628 bp length for a total of 6 samples (three Cameroonian samples from Campo, Kribi and the stranded propagules and three reference sequences from Genbank) were aligned for comparative description of mutated positions using MAFFT v7.388 (Katoh et al., 2002; Katoh and Standley, 2013). Genbank accessions of ITS1 and ITS2 were obtained for R. racemosa originating from Panama, West Atlantic (KJ194274); R. mangle from Panama, West Atlantic (HQ337954); and R. mucronata from India (KX231338) (Surya and Hari, 2017). Unfortunately, no Genbank accession was available from the East Atlantic, to consider as a reference for our samples.
A Neighbor-Joining tree was built with Tamura-Nei as genetic distance and 100 bootstrap resampling method without outgroup, using Geneious Tree Builder for the concatenated cpDNA sequences of the rbcL gene, rpl16 gene, trnS-trnG intron and atpB-rbcL intergenic spacer (Cameroonian vs. Haplotypes A, H, and K) and for the nuclear internal transcribed spacer regions ITS1 and ITS2 (Campo, Kribi, stranded propagules, R. racemosa, R. mangle and R. mucronata).
Ocean Current Patterns Around Our Sampling Period
To verify the role of ocean current patterns in the dispersal of these drift propagules based on their genetic signatures, we use a global ocean current visualization platform by Beccario (2021) (http://earth.nullschool.net) to visualize the ocean currents around the Ntem Estuary for 3 weeks prior to our field surveys. On this ocean current visualization platform, we chose the ocean mode and animated ocean currents. Beccario's (2021) visualizes data of the Ocean Surface Current Analysis Real-time (OSCAR) model of the Earth & Space Research (ESR) (ESR, 2009). This model is based on satellite data of sea surface height, wind, and temperature to calculate ocean currents. Ocean current calculations used quasi-steady geostrophic model, with an eddy viscosity based wind-driven ageostrophic component, and wind temperature adjustment (ESR accessed 05/14/2021).
Results
On-Site Surveys
We monitored drift propagules on a beach area ~3 km from the closest adult mangrove stands around Campo River. These propagules were predominantly from two Rhizophora racemosa, although a few Avicennia germinans propagules were also observed (Figure 2)—representative of the most common mangrove species in this region. Of the 77 R. racemosa propagules collected, the majority (91%) appeared “viable”—based on the absence of any damage on their external presentation, with healthy roots or root buds on many. About 7% of all the propagules had signs of abrasion and other structural damage of the hypocotyl but could potentially still grow and establish (Figure 2), while the remaining 2% were severely damaged—broken in two and were incomplete from top to bottom (Figure 2).
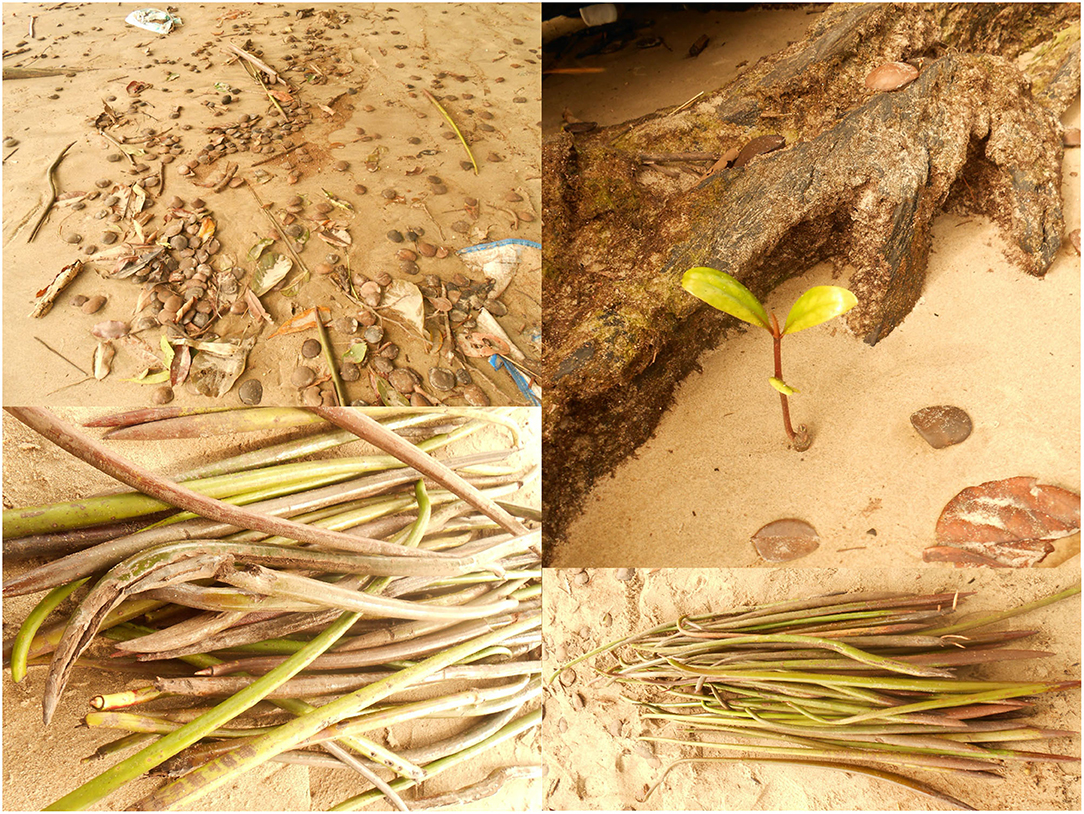
Figure 2. Photos from field surveys and propagules, showing a clearly rooted plant with leaves at the landward edge of the beach. Majority of the propagules were viable, and a few others had structural damages.
We observed a single individual seedling which had successfully rooted and had 3 leaves. We did not find any other vagrant trees or seedlings along another 2 km stretch of the coastline we surveyed (~ 1 km from the first area).
Allelic and Genetic Diversity
Of the 77 R. racemosa propagules collected, successful DNA genotyping was done for 60 propagules. The drift propagules were genetically more diverse than the proximate mangrove areas from which we sampled adult trees. The pool of 60 drift propagules genotyped had higher mean number of different alleles (At), allelic richness (Ar), unbiased expected heterozygosity (uHe), percentage polymorphic loci, and higher inbreeding coefficient (FIS) than all two proximate mangrove populations (Table 1). Outcrossing rate and observed heterozygosity were highest for adult trees at Campo and lowest in the drift propagules (Table 1). Private alleles could be found in the drift propagule pool (21) and in Campo (3) but not in Kribi (Supplementary Table S1).
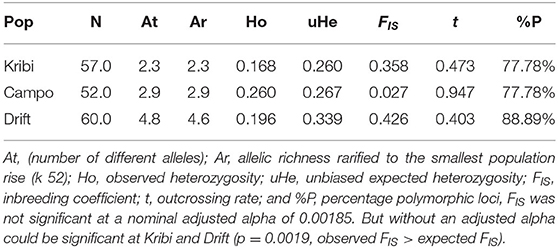
Table 1. Genetic diversity measures show higher genetic diversity in the drift propagule pool (Drift) compared to nearest mangrove forest areas.
Relatedness and Genetic Structure
Within-site selfing rate was ~ zero at Kribi, Campo; the Drift propagule pool also did not originate from within-site selfing. However, kinship coefficient (Supplementary Table S3) of individuals within each site was largest at the Drift propagule population (FIJ = 0.287, p = 0.0002) than at Kribi (FIJ = 0.125, p = 0.012), and Campo (FIJ = 0.088, p = 0.0023) sites. Kinship coefficients of individuals between site pairs, relative to whole sample (3 populations—Kribi, Campo, and Drift pool) was low among all possible pairs. Although generally low, kinship coefficient was highest between Kribi and Campo (FIJ = +0.063) and negative, hence no kinship at all, between each of these two sites and the drift propagule pool (Drift and Kribi FIJ = −0.176, Drift and Campo FIJ = −0.137; Supplementary Table S4). Similarly, mean within-population relatedness was lowest in Campo (r = 5.2), followed by Kribi (r = 6.9) and it was highest in the drift propagule pool (r = 9.3). Based on pairwise F′ST was highest between the drift propagules and Campo (F′ST = 0.380) and drift propagules and Kribi (F′ST = 0.424); and very low between Kribi and Campo (F′ST = 0.071). DEST was different from 0 (p = 0.0001) between these two sites—Kribi and Campo—and the propagule pool (Drift); higher between Kribi and Drift (DEST = 0.192), than between Campo and Drift (DEST = 0.165). Kribi and Campo also had significant but lower level of DEST(0021). A DAPC of Kribi, Campo and Drift “populations” (Figures 3A,C), and that of Drift and all other Cameroonian sites (Figures 3B,D) both show a very clear separation of the drift propagules from the Cameroonian sites along the first axis. Zooming in to the DAPC assignment for only the southern estuaries and the drift propagules, Kribi and Campo samples were highly admixed with their two site clusters, while the third cluster was made of the drift propagules only (Figure 4B). Based on the Evanno method, the highest delta K was 2 (Supplementary Figure S1); indicating all individual drift propagules and the samples from Kribi and Campo were assigned to two genetic clusters following Bayesian clustering analyses in Structure. Structure grouped all samples (from Kribi, Campo) into a single genetic cluster, separated from a second cluster containing the drift propagules only (Figure 4A).
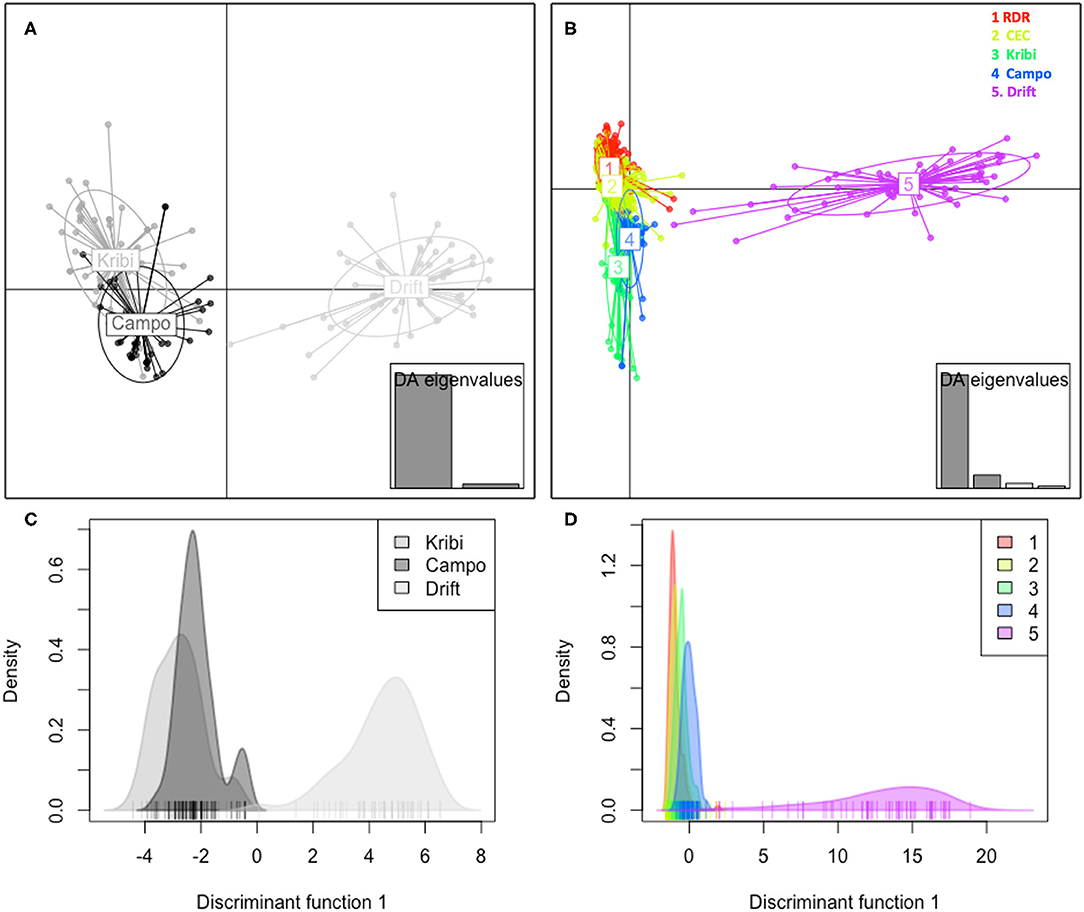
Figure 3. Discriminate analysis of principal components (DAPC) clustering of drift propagules with the closest mangrove estuaries (Kribi and Campo) (A) and with the other central and northern estuaries (B) along the Cameroonian coastline. DAPC was performed with original populations and colors represent the different clusters at K = 2 for the analyses using Kribi, Campo, and Drift propagules (A), and K = 4 for the analysis including all other sites of the Cameroonian range (B) [i.e., RDR, Rio Del Rey Estuary; CEC, Cameroon Estuary complex; Kribi, Lokoundje Estuary, Campo (Ntem Estuary), see Figure 1]. Additionally, discriminant analyses of each corresponding data set are shown under each respective DAPC result (C,D); the first discriminant function (x-axis) vs. the smoothed density of observations (y-axis).
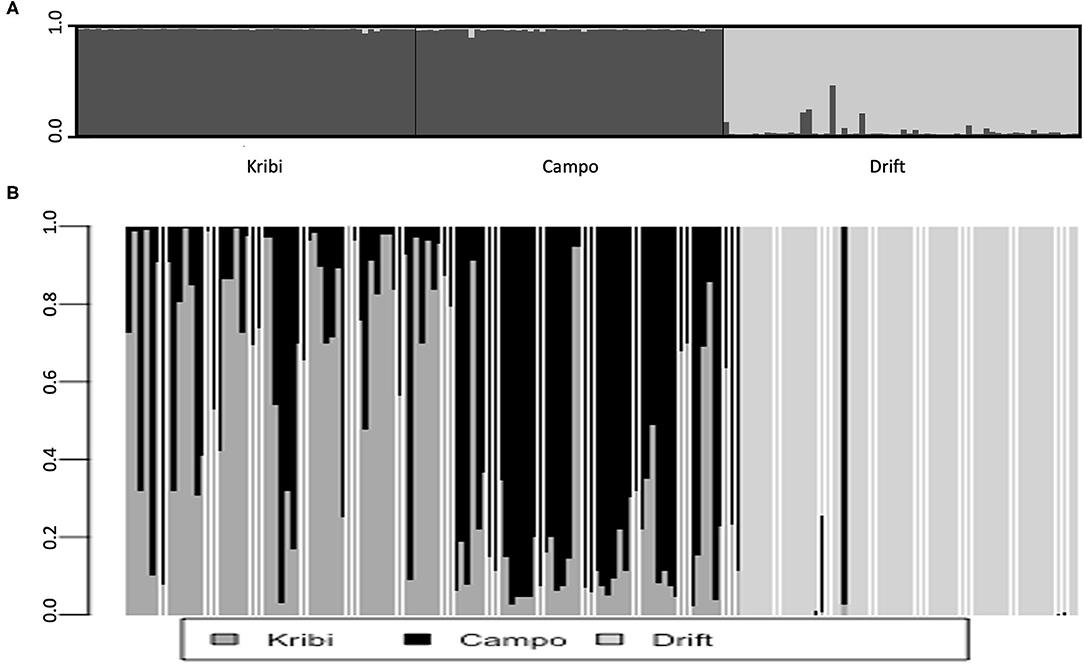
Figure 4. Bayesian clustering analyses (A) and Compoplot of all samples assignment based on DAPC (B) both show the Drift propagules form a single cluster which is isolated from Kribi and campo cluster. Compolot show more admixture in individuals from the Kribi and Campo but not the drift propagules.
Assignment Tests and Identification
Considering these same two southerly populations, Kribi and Campo, population assignment tests in GenAlex (for data exploration) show that only a single drift propagule out of 60 could potentially be assigned to any other population (Supplementary Table S2). The plot of these explorative population assignments, first with only Kribi and Campo included as potential source populations (Supplementary Figure S2A) and secondly with all other mangrove sites included as source populations (Supplementary Figure S2B; Supplementary Table S2), show the drift propagules are most likely from a different genetic neighborhood from all other sites along the Cameroonian range, based on our extensive samples from Cameroon mangrove areas. To confirm this hypothesis of a different origin, assignment tests (GeneClass2) based on allele frequency showed that the probability of any of the drift propagules being assigned to any of the sites along the Cameroonian coastline was very low (<0.05 threshold). Only 1 out of the 60 drift propagules had a probability of 0.075 of originating from Campo. That same propagule had a 0.023 chance of originating from RDR Estuary (northernmost estuary), and a 0.014 chance of originating from CEC (central mangrove area). Four other propagules had lower than the threshold probability, while all others had a probability of zero, of originating from any of the Cameroonian sites. These low probabilities show that none of these drift propagules were from the Cameroonian range, and as such—their origin could not be determined. For detection of first-generation (F0) migrants, we focused on just the drift propagules and the closest mangrove areas Campo and Kribi. Four individuals from Kribi could be first generation migrants from Campo; and two individuals from Campo could be assigned to Kribi (p < 0.05). However, individual samples of the drift propagule pool were not assigned as first-generation migrants to any of these two southerly forested areas. Because of the numerous private alleles and a suite of analyses supporting a separate gene pool of the drift propagules, their species identity warranted confirmation. Chloroplast sequences of samples of Cameroon resulted in exactly similar sequences of the rbcL gene (1371 bp) and the rpl16 gene (895 bp); a single substitution in the trnS-trnG intron (481 bp with G instead of A in position 242); and a mononucleotide repeat in the atpB-rbcL intergenic spacer (710bp with A[11] instead of A[10] in position 486–496) in comparison to the reference haplotype H of R. racemosa from Ghana (Genbank accessions provided in methods section). These identical sequences or minor mutations refer to a maternal identity of R. racemosa of the stranded propagules as well as of all considered Cameroonian populations throughout the studied area. These were strongly different (100 bootstrap) from R. mangle (Haplotype A) or R. mucronata (Haplotype K) in a NJ tree (Supplementary Figure S3A) and thus refer to a R. racemosa chloroplast genome.
The nuclear ribosomal internal transcribed regions ITS1 and ITS2 were similar for all Cameroonian populations, including the stranded propagules and differed strongly from the other species R. mangle, R. mucronata as well as from R. racemosa of the West Atlantic in a NJ tree (Supplementary Figure S3B). Thus, both chloroplast sequences and nuclear rRNA intergenic spacers indicated that the Cameroon populations belong to an East Atlantic taxon, considered as R. racemosa and showed 100% identity with the stranded propagules.
Patterns in Ocean Currents Around Sampling Period
Patterns in ocean currents around the Cameroonian coastline are complex. Although the predominant Guinea Current flows southwards, there is a northward flowing surface current also. For the studied region, ocean current patterns around the Ntem Estuary around December 2014 to January 2015 (moment of our sample collection) were south to north in directionality (Figure 5). Such complex ocean current patterns could facilitate rare drift propagule dispersal into the survey site, from more southern West African mangrove stands. Such southern mangrove areas include mainland Equatorial Guinea (5 ~ 100 km from this propagule survey site), Gabon (~ 265 km), and Angola (> 1,110 km) mangrove range (see Figure 1 showing mangrove areas in the region, black markings along the coastline on the left of the figure). As well as other Atlantic islands—Bioko, São Tomé and Príncipe, islands (~ 165–440 km).
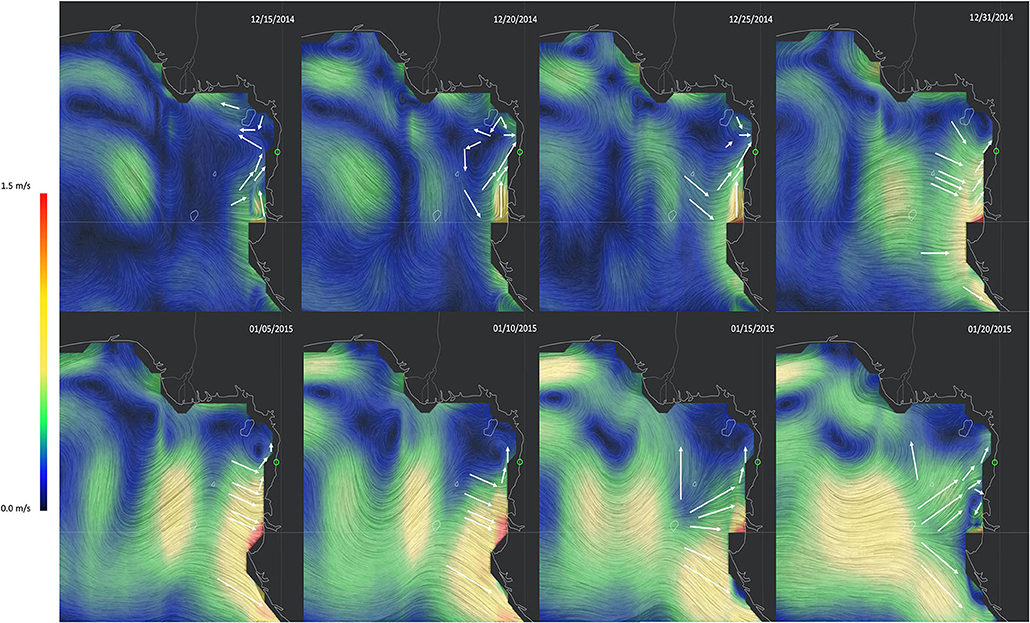
Figure 5. Ocean current patterns in the months of December 2014 and January 2015 (when we collected samples) show a predominant south to north direction of movement. These surface ocean current patterns are based on data of Earth and Space Research's (ESR) Ocean Surface Current Analysis Real-time (OSCAR) model (https://www.esr.org/research/oscar/oscar-surface-currents/); and the visualization of this OSCAR data is done by Cameron Beccario (http://earth.nullschool.net).
Discussion
We observed a cohort of drift propagules at a non-mangrove beach area at the Ntem Estuary in Cameroon and used that as a cue to assess dispersal dynamics and possibilities of the red mangrove R. racemosa along the Cameroonian coastline. Observations of one vagrant juvenile plant and over 92% of sampled R. racemosa propagules seemed “viable” (i.e., intact and/or rooting), indicating potential for establishment at this site. By genotyping drift propagules and assessing their relatedness, we found out that, contrary to our hypothesis, these drift propagules did not originate from the nearest populations or any of the more distant populations of the Cameroonian range. Stranded propagules were higher in genetic diversity and more related to each other than samples from proximate sites. Although microsatellite alleles were substantially different, the drift propagules were identified as the East Atlantic R. racemosa on basis of four chloroplast genes and nuclear rRNA intergenic spacers without any indication of introgression with R. mangle.
The Origin and Indications of the Drift Propagules (Locally vs. Unknown (Remote) Sources)
The presence of drift propagules on bare, non-mangrove-forested beaches (e.g., De Ryck et al., 2016) provide evidence that actual dispersal events exceed realized gene flow and more seldom contemporary ranges of species. Drift propagules beyond present day mangrove forested areas following major storm events (Kennedy et al., 2020) highlight the impact that stochastic environmental events could have on range expansions by bolstering dispersal into sites beyond present limits of mangrove distribution (e.g., Kennedy et al., 2020). LDD events contribute in the colonization of remote areas, the connectivity of populations especially in a fragmented landscapes, the spread of advantageous alleles (Attard et al., 2018), and drive the mechanism of species adaptation to changing environmental conditions (Blanquart et al., 2012). Despite the importance of LDD, the scientific community has a limited understanding of the extent, frequency, and consequences of LDD because of limited observations of actual LDD events and also inaccurate predictions of the behaviors of the tails of dispersal kernels and the property of LDD (Jordano, 2017).
From the two two-kilometer beach areas surveyed, we observed viable mangrove propagules in one and none in the other. These propagules were observed at an area close (~3 km) to established mangrove site around the Campo River estuary. Several of the Rhizophora propagules were rooting, and one Rhizophora seedling was observed already rooted and growing at the upper edge of the beach line. These are all indications of a potential mangrove expansion site. Yet where these propagules are from is important for understanding dispersal dynamics along this coastline and how that drives the distribution of genetic variation of mangroves here. Compared to other sites along the Cameroonian coastline, Campo and Kribi populations were isolated both geographically (i.e., no mangroves between) and genetically from all other mangrove areas along the Cameroonian coastline; due to complex surface ocean current front around the Cameroonian coastline (see Ngeve et al., 2016). Historically, mangroves might have expanded from these southerly sites to the northern sites (Ngeve et al., 2017a). So, the isolation of Campo and Kribi populations from all others along the Cameroonian coastline reflects more contemporary oceanic patterns on the dispersal of propagules. Nevertheless, based on occurrence of both Kribi and Campo within the same ocean current, it was sensible to assume that they contributed to the dispersal of these propagules to this unforested beach, in addition to the spatial proximity to these bare sites; first Campo (~ 3–6 km) and then Kribi (~85 km) (compared to others along the Cameroonian coastline, > 300 km). Yet our assignment tests showed that none but 1 of the drift propagules had a greater-than-threshold probability (0.05) of being assigned to either Campo or Kribi populations and none were even first-generation migrants from these sites. In fact, graphs of population assignment tests and both Bayesian (Structure) and multivariate (DAPC) clustering of samples isolated the drift propagules apart from the two closest mangrove areas as well as from all other sites along the Cameroonian coastline. The Kribi and Campo populations are relatively small, well-delineated and restricted such that we may assume that our sampling design was sufficiently random to capture most of the allele diversity and certainly the most common ones. Drift propagules were not released from the closest mangrove sites and did not originate from northerly estuaries along the Cameroonian coastline, suggesting remote (beyond the Cameroonian range) dispersal into this area by the prevailing local (hydrodynamic) conditions. Visualizations of total cloud water (TCW) content (Supplementary Figure S4) (by Beccario, 2021) is based on data from the Global Forecast System (GFS) of the National Centers for Environmental Prediction Environmental Modeling Center (EMC), which is an arm of the National Oceanic and Atmospheric Administration (NOAA). The TCW for this region around our sampling period suggest high levels (~ 1 kg/m2) on several days around our sampling period (Supplementary Figure S4). Such high total cloud water content levels might indicate scattered thunderstorms prior to our sampling. Extreme weather events have been associated with stochastic LDD of propagules (see Nathan et al., 2008; Van der Stocken et al., 2019b). Any storms prior to this period could have forced propagule dispersal beyond normal dispersal limits into remote locations such as this unforested beach area, under favorable local geomorphologic and oceanographic conditions.
Complex Interaction of Ocean Currents and Tidal Fluxes and Estuarine Geomorphology in Drift Propagule Dispersal
The Cameroonian coastline is characterized by two main surface ocean current patterns. The southwards flowing Guinea Current and the northwards flowing surface current also known as littoral drift. Both the Guinea Current and the Littoral drift converge offshore around the CEC, forming a soft barrier between populations to the south (Kribi and Campo), and those to the north of this oceanic front (Ngeve et al., 2016). Being within the same ocean current, it was very interesting to find that neither Kribi nor Campo were the sources of any of the drift propagules we sampled. In fact, on assessing the relative difference between these propagules and mangrove samples from the Cameroonian coastline, these propagules were very different from our samples from Cameroon. To understand these patterns, we zoomed in to the Ntem Estuary area, for the months of December 2014 to January 2015, and visualized ocean currents based on OSCAR ocean model data (by Beccario, 2021). Ocean current patterns around this period showed a predominant south to north movement around this estuary. This south to north pattern matches the expected littoral drift patterns, also observed in Ngeve et al. (2016); and suggests that these propagules originated from the more southern mangrove areas of the West African range (Equatorial Guinea, Congo, Gabon, Angola range) and/or Atlantic islands in the Bight of Bonny. The Ntem River is a natural border between Cameroon and mainland Equatorial Guinea; and such an open system might allow for propagule dispersal between mangrove sites across the river. River channel structure and proximity to water (landward vs. seaward) has been shown to determine patterns of fine scale genetic structure in mangroves (e.g. see Triest et al., 2020; Triest and Van der Stocken, 2021). High local hydrological accessibility within Campo mangrove stands may explain why they lacked fine-scale genetic structure (Ngeve et al., 2017a), while at the same time, being regionally isolated from other Cameroonian sites (Ngeve et al., 2016).
The geomorphology of the Ntem Estuary has a slight northward orientation. Therefore, based on ocean currents alone, propagules from the southerly areas are likely be washed ashore to the open ocean coastline area between Kribi and the Ntem Estuary rather than the site we sampled them from. Estuarine and coastal geomorphology has been implicated in dispersal limitation and the structure of genetic variation in Panamanian (Cerón-Souza et al., 2012), Farasan Archipelago (Al-Qthanin and Alharbi, 2020), and South African (De Ryck et al., 2016) mangroves. Ntem mangroves are well-separated from other mangrove areas along this coastline; they occur ~85 km south of the Lokoundje mangroves area (Kribi), and ~ 100 km north of the Mbini River mangroves (Equatorial Guinea) (although a few scattered stands could be found midway). Very small mangrove patches are also reported in the Bight of Bonny Islands (~ 165 km: Bioko; 275 km to 440 km: São Tomé and Príncipe). To have bypassed the north-oriented estuarine opening (see Figure 1) and made way into the surveyed area, indicate interaction of ocean current patterns and tidal system of the Ntem River to facilitate such (stochastic) LDD. Experimental studies have shown the role of tides in R. racemosa propagule dispersal (Ngeve et al., 2017b). An alternative hypothesis to a long-distance, beyond-country-border dispersal is that these propagules might have originated from unsampled unique genotypes in the Ntem Estuary. However, this alternative hypothesis is less likely given that (1) we collected a very representative sample of these sites, (2) there are evidences of high within-estuary dispersal in mangroves (e.g., De Ryck et al., 2016; Ngeve et al., 2017b), and (3) prevailing south to north ocean currents dispersed virtual propagules in like manner in the region (see Ngeve et al., 2016). Moreover, these drift propagules were strongly differentiated from samples from Campo and other Cameroonian sites. These propagules do not originate from local sources; and a complex interaction of tides and oceanic currents must have bypassed a potentially prohibitive estuarine geomorphology to bring them to the sampled area. Yet, understanding whether they are from diverse multiple remote sources or a from single source, is relevant to establish the dispersal model employed.
Are Drift Propagules From Genetically Heterogenous or Homogenous Sources—Migrant Vs. Propagule Pool?
The mismatch between historical (Ngeve et al., 2017a) and contemporary (Ngeve et al., 2016) patterns of effective dispersal along the Cameroonian coastline might be explained by several direct (fragmentation, and habitat degradation) and indirect (climate change-induced sea level rise) anthropogenic effects. Historically, effective number of migrants from Campo to Kribi was higher than between any other sites along this coastline (Nem ~ 60; Ngeve et al., 2017a) [based on the coalescent approach to estimate historical migration rates in Migrate-n (Beerli, 2006; Beerli and Palczewski, 2010)]. Such high historical dispersal from Campo to Kribi may explain positive kinship coefficient (i.e., high relatedness) between Kribi and Campo, despite the ~ 85 km distance between the sites. But dispersal over several km is added evidence of the extensive dispersal potential of Rhizophora propagules. Meanwhile, opposite kinship patterns were observed between these two populations and the drift propagule pool. In fact, no kinship at all between the drift propagules and Kribi/Campo sites confirms that these propagules did not originate from these sites. Interestingly, highest kinship was obtained among the cohort of drift propagules and suggests to a single dispersal event of a bunch of propagules kept altogether until stranding. Our results imply that the drift propagules thus are strongly related to each other and potentially from the same source. Our field work coincided with an annual peak season for propagule release/abscission in this region, so it plausible that drift propagules were abscised from the same forest area and dispersed to this area as described above. Parentage analyses could not be performed to identify the parentage of the drift propagules. Yet, their higher kinship coefficient led us to hypothesize that they are from a single or no more than several trees, beyond the Cameroon borders.
Despite being more related than mangrove sites in the south of Cameroon, we observed numerous alleles (higher allelic richness) in the drift propagule pool, than in adult trees of Kribi and Campo sites, suggesting that the propagules were made from outcrossing mating events. Multilocus outcrossing rates <20% is indicative of inbreeding, between 20 and 80% is considered mixed mating system, and > 80% predominantly outcrossing, in self-compatible plants (Yan et al., 2016). Previous work show Rhizophora racemosa employs a mixed mating system, where outcrossing rates exceed geitonogamous biparental inbreeding (Ngeve et al., 2020). Given that Rhizophora species are known to produce self-incompatible flowers, outcrossing is not unexpected, especially when pollinator availability and landscape structure are not restrictive (e.g., Wee et al., 2015). Multilocus outcrossing rates (t) show Campo site is predominantly outcrossing (t = 95%), but the drift propagule pool (t = 40%) indicated a mixed mating system as did Kribi (t = 47%). Being moderately outcrossed and having a high number of alleles (allelic richness), while at the same time originating beyond the range of other Cameroonian populations for which we have samples make it difficult to tell whether these propagules followed Slatkin's migrant pool model vs. propagule pool model of migration. The migrant pool model, where propagules originate from multiple sources to a site, results in a higher level of genetic diversity than if propagules dispersed via the propagule pool model (all from a single source and usually lower in genetic diversity). Moderate levels of multilocus outcrossing rate could also be a computational artifact due to the assumption of drift propagule pool as one population. Although the drift propagules were more related to each other than samples from proximate mangrove forest areas, pooling a few genetically distant propagules into this drift propagules pool as one population could cause a temporal Wahlund's effect and result in an exaggerated estimate of FIS (De Meeûs, 2018). Any exaggerations on FIS estimates would lower outcrossing rates (t), since it is calculated as = (1 – FIS)/(1 + FIS). Higher genetic diversity of these drift propagules suggests a probably higher-than-estimated outcrossing rates. Yet, higher relatedness might indicate some variant intermediate of both propagule pool and migrant pool models of dispersal. Whereby a diverse group of paternal genotypes contributed to the pollen pool of their mother(s), resulting in a diverse group of probable half-siblings which all dispersed as a cohort into this bare site. If such a stochastic dispersal event resulted in established populations, they will impact the evolution of metapopulations (see Goodnight, 2011), and have significant management implications by contributing their unique genotypes to this area and in metapopulation dynamics.
Implications for Conservation and Conclusions
Our results show this cohort of drift propagules originated beyond the Cameroonian borders, as ocean current patterns bring in seedlings probably from the south and/or other Atlantic islands (~ 5 km to over 1,100 km from this site). Were they to establish in this area, the drift propagules highlight the potential of stochastic LDD events in (local) range expansions (e.g., see Davies et al., 2004; Bialozyt et al., 2006; Vanden Broeck et al., 2014). Previous data, which show mangroves of the Ntem Estuary to be strongly differentiated from all others along the Cameroonian coastline (Ngeve et al., 2016), and had no signs of fine-scale genetic structure (Ngeve et al., 2017a). Yet the unique genotypes in the drift propagule cohort, underscores the unique diversity of these southern West African mangrove areas. The high unique number of alleles in drift propagules and previous research findings of no fine scale genetic structure in Campo (Ngeve et al., 2016, 2017a), also allow us to hypothesize that these southerly mangroves (and those of the Bight of Bonny islands: Bioko, São Tomé and Príncipe) may be subject to genetic breaks. Indeed, it is possible that only few or none of these drift propagules really survive or even make it to an adult tree. Such few survivors then are subject to drift in case the founder populations are small in pop size (N = 1 to few) and remote from “native” ones. Repeated LDD events are unlikely to arrive on the same stretch of beach or estuary.
In addition to a unique and high diversity, the small-sized southerly West African mangrove areas can be vulnerable to stochastic losses from drift as well as to anthropogenic pressures; that is the mangroves of Equatorial Guinea, Gabon, and Angola, including Campo in Cameroon (Corcoran et al., 2007). Therefore, these sites need to be monitored for their unique genetic identity and if necessary prioritized for protection.
Given the evidence presented here of dispersal of drift propagules across country borders, such revelation is an important consideration for management of mangroves worldwide. Transboundary connectivity has been observed among several mangrove forest sites (e.g., Triest et al., 2021d). Any dispersal across country borders highlights the need for international and inter-governmental efforts in the conservation of biological diversity. These studies and our current data show that “border problem” is a man-made concept. The movement of (other) species across country boundaries is not out of the ordinary. As such, LDD events, across country borders, could play a significant role in species and ecosystem resilience. Therefore, emphasizing the need for international authorities to cooperate, especially in the face of global change, for the conservation of biological diversity (see Titley et al., 2021). Remarkable is how infrequently such movements (LDD events) are considered for plant conservation action and planning since plants are regarded as sessile and conservation spatially static. Indeed, stochastic LDD events across country borders and accessibility of mangrove areas can contribute distribution of genetic variation among existing populations of mangroves.
Dispersal is a crucial process for persistence of populations and thus is fundamental for ecosystem management and conservation (Edelaar and Bolnick, 2012). Therefore, understanding how, and the spatial scale over which, dispersal occurs is relevant because it will provide useful insights on the drivers and patterns of mangrove range expansion, and extent and/or patterns of connectivity networks of existing populations. Such information is crucial for designing protected areas and implementing effective management strategies in the face of global change. Yet, the spatial extent of effective dispersal of a species is determined both biological traits of the species and by environmental factors. Species-specific dispersal abilities due to propagule traits (size, flotation, viability) interact with environmental factors (eddies, ocean currents, geographic distance) to ensure its effective dispersal extent. In fact, differences in dispersal potential has been associated with differences in genetic signatures of sympatric mangrove species (Cerón-Souza et al., 2012; Wee et al., 2020). Therefore, evidence of LDD observed here for Rhizophora racemosa propagules does not mirror expectations for all mangrove species. Nevertheless, in this region where Rhizophora racemosa is the predominant mangrove, a foundation species and exploited resource, our results therefore show that its dispersal can be much more extensive than current mangrove range and this high dispersal extent could allow for the expansion of its range to suitable areas within this area.
Data Availability Statement
The datasets presented in this study can be found in online repositories. The names of the repository/repositories and accession number(s) can be found in the article/Supplementary Material.
Author Contributions
MN, NK, and LT: originally formulated the idea and reviewed the manuscript. MN: conceived and designed the study, performed the field and laboratory work, collected the samples, analyzed the data, and wrote the paper. MN and LT: contributed reagents, materials, and analysis tools. All authors contributed to the article and approved the submitted version.
Funding
The BAS 42 funding of the VUB supported laboratory analyses of this study; while funding for the field work was partially covered by the Rufford Foundation (small grant: 17335-1).
Conflict of Interest
The authors declare that the research was conducted in the absence of any commercial or financial relationships that could be construed as a potential conflict of interest.
Publisher's Note
All claims expressed in this article are solely those of the authors and do not necessarily represent those of their affiliated organizations, or those of the publisher, the editors and the reviewers. Any product that may be evaluated in this article, or claim that may be made by its manufacturer, is not guaranteed or endorsed by the publisher.
Acknowledgments
We are grateful for the comments received from Prof. Maile Neel (and some of her lab members), and from Prof. Anahí Espíndola, and Dr. Michael Roswell, on the very first draft of this manuscript. We are also thankful for the support MN received in the field from Rev. Atiko Pierre and Mr. Atkin Egbe of Kribi and Mr. Tim Sierens of VUB for lab logistical support.
Supplementary Material
The Supplementary Material for this article can be found online at: https://www.frontiersin.org/articles/10.3389/fcosc.2021.746461/full#supplementary-material
Supplementary Figure S1. Selecting the best K value using the Evanno method of highest delta K.
Supplementary Figure S2. Population assignment test done in GenAlex explore how drift propagules data relate to/standout from (are not assigned to) Kribi and Campo (A) no to any other mangrove area along the Cameroonian coastline (B).
Supplementary Figure S3. Neighbor-Joining tree (NJT) of four concatenated sequences (rbcL gene, rpl16 gene, trnS-trnG intron and the atpB-rbcL intergenic spacer) of haplotypes A, H and K and of Cameroon sample (identical for populations and “drift” propagules) showing large similarity of Cameroon samples with Haplotype H of R. racemosa (A). NJT of nuclear ribosomal internal transcribed regions ITS1 and ITS2 of samples from Cameroon, drift propagules, and other species show similarity between drift propagules and all Cameroonian populations but strong differences of these samples from the other species—R. mangle, R. mucronate—as well as from R. racemosa of the West Atlantic in a NJ tree (B).
Supplementary Figure S4. Total cloud water (TCW) content for some days prior to our sampling period. Bright white areas show higher cloud water content and could indicate scattered thunderstorms in the area around the sampling period. Total Cloud water content visualization is done by Cameron Beccario (http://earth.nullschool.net) is based on data from Global Forecast System (GFS) of the of the National Centers for Environmental Prediction Environmental Modeling Center (EMC) of the National Oceanic and Atmospheric Administration (NOAA).
Supplementary Table S1. Summary of all private (unique) alleles in all populations. There were no private alleles found at KRIBI; 3 were found at CAMPO and 21 unique alleles were seen in the drift propagule pool.
Supplementary Table S2. Summary of Population Assignment Outcomes to “Self” or “Other” Population (With ‘leave one out' Option).
Supplementary Table S3. Within site kinship coefficient (FIJ).
Supplementary Table S4. Pairwise Kinship coefficient (FIJ) with respect to entire sample.
Supplementary Table S5. Chloroplast genes and Nuclear rRNA intergenic spacer region (ITS) regions used in this manuscript. * Rhizophora mangle, **Rhizophora mucronata, all other sequences were generated from Rhizophora racemosa.
References
Al-Qthanin, R. N., and Alharbi, S. A. (2020). Spatial structure and genetic variation of a mangrove species (Avicennia marina (Forssk.) Vierh) in the Farasan Archipelago. Forests 11, 1287. doi: 10.3390/f11121287
Attard, C. R. M., Brauer, C. J., Sandoval-Castillo, J., Faulks, L. K., Unmack, P. J., Gilligan, D. M., et al. (2018). Ecological disturbance influences adaptive divergence despite high gene flow in golden perch (Macquaria ambigua): implications for management and resilience to climate change. Mol. Ecol. 27, 196–215. doi: 10.1111/mec.14438
Balke, T., Bouma, T. J., Horstman, E. M., Webb, E. L., Erftemeijer, P. L. A., and Herman, P. M. J. (2011). Windows of opportunity: thresholds to mangrove seedling establishment on tidal flats. Mar. Ecol. Prog. Ser. 440, 1–9. doi: 10.3354/meps09364
Beccario, C. (2021). earth: A Global Map of Wind, Weather, and Ocean Conditions. Available online at: https://earth.nullschool.net (accessed May 15, 2021).
Beerli, P. (2006). Comparison of Bayesian and maximum-likelihood inference of population genetic parameters. Bioinformatics 22, 341–345. doi: 10.1093/bioinformatics/bti803
Beerli, P., and Palczewski, M. (2010). Unified framework to evaluate panmixia and migration direction among multiple sampling locations. Genetics 185, 313–326. doi: 10.1534/genetics.109.112532
Bialozyt, R., Ziegenhagen, B., and Petit, R. J. (2006). Contrasting effects of long distance seed dispersal on genetic diversity during range expansion. J. Evol. Biol. 19, 12–20. doi: 10.1111/j.1420-9101.2005.00995.x
Blanquart, F., Gandon, S., and Nuismer, S. L. (2012). The effects of migration and drift on local adaptation to a heterogeneous environment. J. Evol. Biol. 25, 1351–1363. doi: 10.1111/j.1420-9101.2012.02524.x
Blyton, M. D. J., and Flanagan, N. S. (2012). A Comprehensive Guide to: Genalex Genetic Analyses in Excel. Available online at: http://biology.anu.edu.au/GenAlEx/ (accessed May 10, 2020).
Cerón-Souza, I., Bermingham, E., McMillan, W. O., and Jones, F. A. (2012). Comparative genetic structure of two mangrove species in Caribbean and Pacific estuaries of Panama. BMC Evol. Biol. 12:205. doi: 10.1186/1471-2148-12-205
Corcoran, E., Ravilious, C., and Skuja, M. (2007). Mangroves of western and Central Africa. Cambridge, United Kingdom: UNEP World Conservation Monitoring Centre: UNEP, Regional Seas Programme.
Davies, S., White, A., and Lowe, A. (2004). An investigation into effects of long-distance seed dispersal on organelle population genetic structure and colonization rate: a model analysis. Heredity 93, 566–576. doi: 10.1038/sj.hdy.6800555
De Meeûs, T. (2018). Revisiting FIS, FST, Wahlund effects, and null alleles. J. Heredity 109, 446–456. doi: 10.1093/jhered/esx106
De Ryck, D. J. R. D., Koedam, N., Stocken, T. V., der Ven, R. M., van der Adams, J., and Triest, L. (2016). Dispersal limitation of the mangrove Avicennia marina at its South African range limit in strong contrast to connectivity in its core East African region. Mar. Ecol. Prog. Ser. 545, 123–134. doi: 10.3354/meps11581
Di Nitto, D., Erftemeijer, P. L. A., van Beek, J. K. L., Dahdouh-Guebas, F., Higazi, L., Quisthoudt, K., et al. (2013). Modelling drivers of mangrove propagule dispersal and restoration of abandoned shrimp farms. Biogeosciences 10, 5095–5113. doi: 10.5194/bg-10-5095-2013
Din, N., Ngo-Massou, V. M., Essomè-Koum, G. L., Ndema-Nsombo, E., Kottè-Mapoko, E., and Nyamsi-Moussian, L. (2017). “Impact of urbanization on the evolution of mangrove ecosystems in the Wouri River Estuary (Douala Cameroon),” in Coastal Wetlands: Alteration and Remediation Coastal Research Library, eds. C. W. Finkl and C. Makowski (Cham: Springer International Publishing), 81–131. doi: 10.1007/978-3-319-56179-0_3
Do, B. T. N., Koedam, N., and Triest, L. (2019). Avicennia marina maintains genetic structure whereas Rhizophora stylosa connects mangroves in a flooded, former inner sea (Vietnam). Estuar. Coast. Shelf Sci. 222, 195–204. doi: 10.1016/j.ecss.2019.04.005
Duke, N. C. (1995). Genetic diversity, distributional barriers and rafting continents—more thoughts on the evolution of mangroves. Hydrobiologia 295, 167–181. doi: 10.1007/BF00029124
Duke, N. C., and Allen, J. A. (2006). Rhizophora mangle, R. samoensis, R. racemosa, R. X harrisonii (Atlantic-East Pacific red mangrove). Species Profiles for Pacific Island Agroforestry 2. Holualoa, Hawaii: Permanent Agriculture Resources (PAR), 1–18.
Duke, N. C., Meynecke, J.-O., Dittmann, S., Ellison, A. M., Anger, K., Berger, U., et al. (2007). A World Without Mangroves? Science 317, 41–42. doi: 10.1126/science.317.5834.41b
Earl, D. A., and vonHoldt, B. M. (2012). Structure harvester: a website and program for visualizing structure output and implementing the Evanno method. Conserv. Genet. Resour. 4, 359–361. doi: 10.1007/s12686-011-9548-7
Edelaar, P., and Bolnick, D. I. (2012). Non-random gene flow: an underappreciated force in evolution and ecology. Trends Ecol. Evol. 27, 659–665. doi: 10.1016/j.tree.2012.07.009
ESR (2009). OSCAR Third Degree Resolution Ocean Surface Currents. ESR OSCAR Surface Currents—Earth and Space Research. Available online at: https://www.esr.org/research/oscar/oscar-surface-currents/ (accessed May 15, 2021).
Evanno, G., Regnaut, S., and Goudet, J. (2005). Detecting the number of clusters of individuals using the software structure: a simulation study. Mol. Ecol. 14, 2611–2620. doi: 10.1111/j.1365-294X.2005.02553.x
Giri, C., and Long, J. (2016). Is the geographic range of mangrove forests in the conterminous united states really expanding? Sensors 16:2010. doi: 10.3390/s16122010
Goodnight, C. J. (2011). Evolution in metacommunities. Philos. Trans. R. Soc. B: Biol. Sci. 366, 1401–1409. doi: 10.1098/rstb.2010.0290
Goudet, J. (1995). FSTAT (Version 1.2): A computer program to calculate F-statistics. J. Hered. 86, 485–486. doi: 10.1093/oxfordjournals.jhered.a111627
Hardy, O. J. (2016). Population genetics of autopolyploids under a mixed mating model and the estimation of selfing rate. Mol. Ecol. Resour. 16, 103–117. doi: 10.1111/1755-0998.12431
Hardy, O. J., and Vekemans, X. (2002). spagedi: a versatile computer program to analyse spatial genetic structure at the individual or population levels. Mol. Ecol. Notes 2, 618–620. doi: 10.1046/j.1471-8286.2002.00305.x
Hodel, R. G. J., Knowles, L. L., McDaniel, S. F., Payton, A. C., Dunaway, J. F., Soltis, P. S., et al. (2018). Terrestrial species adapted to sea dispersal: differences in propagule dispersal of two Caribbean mangroves. Mol. Ecol. 27, 4612–4626. doi: 10.1111/mec.14894
Huisman, T. J., Van Langevelde, F., and De Boer, W. F. (2009). Local positive feedback and the persistence and recovery of fringe Avicennia marina (Forssk.) vierh. mangroves. Wetlands Ecol. Manage. 17, 601–611. doi: 10.1007/s11273-009-9137-7
Jombart, T. (2008). adegenet: a R package for the multivariate analysis of genetic markers. Bioinformatics 24, 1403–1405. doi: 10.1093/bioinformatics/btn129
Jombart, T., Devillard, S., and Balloux, F. (2010). Discriminant analysis of principal components: a new method for the analysis of genetically structured populations. BMC Genetics 11:94. doi: 10.1186/1471-2156-11-94
Jordano, P. (2017). What is long-distance dispersal? And a taxonomy of dispersal events. J. Ecol. 105, 75–84. doi: 10.1111/1365-2745.12690
Jost, L. (2008). GST and its relatives do not measure differentiation. Mol. Ecol. 17, 4015–4026. doi: 10.1111/j.1365-294X.2008.03887.x
Katoh, K., Misawa, K., Kuma, K., and Miyata, T. (2002). MAFFT: a novel method for rapid multiple sequence alignment based on fast Fourier transform. Nucleic Acids Res. 30, 3059–3066. doi: 10.1093/nar/gkf436
Katoh, K., and Standley, D. M. (2013). MAFFT multiple sequence alignment software version 7: improvements in performance and usability. Mol. Biol. Evol. 30, 772–780. doi: 10.1093/molbev/mst010
Kennedy, J. P., Dangremond, E. M., Hayes, M. A., Preziosi, R. F., Rowntree, J. K., and Feller, I. C. (2020). Hurricanes overcome migration lag and shape intraspecific genetic variation beyond a poleward mangrove range limit. Mol. Ecol. 29, 2583–2597. doi: 10.1111/mec.15513
Kopelman, N. M., Mayzel, J., Jakobsson, M., Rosenberg, N. A., and Mayrose, I. (2015). Clumpak: a program for identifying clustering modes and packaging population structure inferences across K. Mol. Ecol. Resour. 15, 1179–1191. doi: 10.1111/1755-0998.12387
Lo, E. Y., Duke, N. C., and Sun, M. (2014). Phylogeographic pattern of Rhizophora (Rhizophoraceae) reveals the importance of both vicariance and long-distance oceanic dispersal to modern mangrove distribution. BMC Evol. Biol. 14:83. doi: 10.1186/1471-2148-14-83
Loiselle, B. A., Sork, V. L., Nason, J., and Graham, C. (1995). Spatial genetic structure of a tropical understory shrub, psychotria officinalis (Rubiaceae). Am. J. Bot. 82, 1420–1425. doi: 10.1002/j.1537-2197.1995.tb12679.x
Meirmans, P. G., and Hedrick, P. W. (2011). Assessing population structure: FST and related measures. Mol. Ecol. Resour. 11, 5–18. doi: 10.1111/j.1755-0998.2010.02927.x
Mori, G. M., Zucchi, M. I., and Souza, A. P. (2015). Multiple-geographic-scale genetic structure of two mangrove tree species: the roles of mating system, hybridization, limited dispersal and extrinsic factors. PLoS ONE 10:e0118710. doi: 10.1371/journal.pone.0118710
Nathan, R., Schurr, F. M., Spiegel, O., Steinitz, O., Trakhtenbrot, A., and Tsoar, A. (2008). Mechanisms of long-distance seed dispersal. Trends Ecol. Evol. 23, 638–647. doi: 10.1016/j.tree.2008.08.003
Nfotabong-Atheull, A., Din, N., and Dahdouh-Guebas, F. (2013). Qualitative and quantitative characterization of mangrove vegetation structure and dynamics in a peri-urban setting of Douala (Cameroon): an approach using air-borne imagery. Estuar. Coasts 36, 1181–1192. doi: 10.1007/s12237-013-9638-8
Ngeve, M. N., Koedam, N., and Triest, L. (2020). Runaway fathers? Limited pollen dispersal and mating system in Rhizophora racemosa populations of a disturbed mangrove estuary. Aquat. Bot. 165:103241. doi: 10.1016/j.aquabot.2020.103241
Ngeve, M. N., Stocken, T. V., der Menemenlis, D., Koedam, N., and Triest, L. (2016). Contrasting effects of historical sea level rise and contemporary ocean currents on regional gene flow of rhizophora racemosa in eastern atlantic mangroves. PLoS ONE 11:e0150950. doi: 10.1371/journal.pone.0150950
Ngeve, M. N., Van der Stocken, T., Menemenlis, D., Koedam, N., and Triest, L. (2017a). Hidden founders? Strong bottlenecks and fine-scale genetic structure in mangrove populations of the Cameroon Estuary complex. Hydrobiologia 803, 189–207. doi: 10.1007/s10750-017-3369-y
Ngeve, M. N., Van der Stocken, T., Sierens, T., Koedam, N., and Triest, L. (2017b). Bidirectional gene flow on a mangrove river landscape and between-catchment dispersal of Rhizophora racemosa (Rhizophoraceae). Hydrobiologia 790, 93–108. doi: 10.1007/s10750-016-3021-2
Ondoa, G. A., Onguén,é, R., Eyango, M. T., Duhaut, T., Mama, C., Angnuureng, D. B., et al. (2018). Assessment of the evolution of cameroon coastline: an overview from 1986 to 2015. J. Coast. Res. 122–129. doi: 10.2112/SI81-016.1
Paetkau, D., Slade, R., Burden, M., and Estoup, A. (2004). Genetic assignment methods for the direct, real-time estimation of migration rate: a simulation-based exploration of accuracy and power. Mol. Ecol. 13, 55–65. doi: 10.1046/j.1365-294X.2004.02008.x
Peakall, R., and Smouse, P. E. (2012). GenAlEx 6.5: genetic analysis in Excel. Population genetic software for teaching and research—an update. Bioinformatics 28, 2537–2539. doi: 10.1093/bioinformatics/bts460
Piry, S., Alapetite, A., Cornuet, J.-M., Paetkau, D., Baudouin, L., and Estoup, A. (2004). GENECLASS2: A software for genetic assignment and first-generation migrant detection. J. Heredity 95, 536–539. doi: 10.1093/jhered/esh074
Pritchard, J. K., Stephens, M., and Donnelly, P. (2000). Inference of population structure using multilocus genotype data. Genetics 155, 945–959. doi: 10.1093/genetics/155.2.945
R Core Team (2019). R: A Language and Environment for Statistical Computing. Vienna, Austria: R Foundation for Statistical Computing. Available online at: https://www.R-project.org/ (accessed April 2, 2020).
Saintilan, N., Wilson, N. C., Rogers, K., Rajkaran, A., and Krauss, K. W. (2014). Mangrove expansion and salt marsh decline at mangrove poleward limits. Glob. Chang. Biol. 20, 147–157. doi: 10.1111/gcb.12341
Surya, S., and Hari, N. (2017). DNA barcoding of mangroves using ribosomal ITS marker in Rhizophoraceae. IJSRST 3, 606–609.
Takayama, K., Tamura, M., Tateishi, Y., Webb, E. L., and Kajita, T. (2013). Strong genetic structure over the American continents and transoceanic dispersal in the mangrove genus Rhizophora (Rhizophoraceae) revealed by broad-scale nuclear and chloroplast DNA analysis. Am. J. Bot. 100, 1191–1201. doi: 10.3732/ajb.1200567
Takayama, K., Tateishi, Y., and Kajita, T. (2021). Global phylogeography of a pantropical mangrove genus Rhizophora. Sci. Rep. 11:7228. doi: 10.1038/s41598-021-85844-9
Thompson, J. D., Higgins, D. G., and Gibson, T. J. (1994). CLUSTAL W: improving the sensitivity of progressive multiple sequence alignment through sequence weighting, position-specific gap penalties and weight matrix choice. Nucleic Acids Res. 22, 4673–4680. doi: 10.1093/nar/22.22.4673
Titley, M. A., Butchart, S. H. M., Jones, V. R., Whittingham, M. J., and Willis, S. G. (2021). Global inequities and political borders challenge nature conservation under climate change. PNAS 118. doi: 10.1073/pnas.2011204118
Tomlinson, P. B. (2016). The Botany of Mangroves. 2nd edn. Cambridge: Cambridge University Press. doi: 10.1017/CBO9781139946575
Triest, L. (2008). Molecular ecology and biogeography of mangrove trees towards conceptual insights on gene flow and barriers: A review. Aquat. Bot. 89, 138–154. doi: 10.1016/j.aquabot.2007.12.013
Triest, L., Del Socorro, A., Gado, V. J., Mazo, A. M., and Sierens, T. (2021a). Avicennia genetic diversity and fine-scaled structure influenced by coastal proximity of mangrove fragments. Front. Mar. Sci. 8:643982. doi: 10.3389/fmars.2021.643982
Triest, L., Sierens, T., and Van der Stocken, T. (2021b). Complete chloroplast genome variants reveal discrete long-distance migration routes of Rhizophora in the Western Indian Ocean. Front. Conserv. Sci. 2:726676. doi: 10.3389/fcosc.2021.726676
Triest, L., Stocken, T. V., der Akinyi, A. A., Sierens, T., Kairo, J., and Koedam, N. (2020). Channel network structure determines genetic connectivity of landward–seaward Avicennia marina populations in a tropical bay. Ecol. Evol. 10, 12059–12075. doi: 10.1002/ece3.6829
Triest, L., and Van der Stocken, T. (2021). Coastal landform constrains dispersal in mangroves. Front. Mar. Sci. 8:723. doi: 10.3389/fmars.2021.617855
Triest, L., Van der Stocken, T., De Ryck, D., Kochzius, M., Lorent, S., Ngeve, M., et al. (2021c). Expansion of the mangrove species Rhizophora mucronata in the Western Indian Ocean launched contrasting genetic patterns. Sci. Rep. 11:4987. doi: 10.1038/s41598-021-84304-8
Triest, L., Van der Stocken, T., Sierens, T., Deus, E. K., Mangora, M. M., and Koedam, N. (2021d). Connectivity of Avicennia marina populations within a proposed marine transboundary conservation area between Kenya and Tanzania. Biol. Conserv. 256:109040. doi: 10.1016/j.biocon.2021.109040
Van der Stocken, T., Carroll, D., Menemenlis, D., Simard, M., and Koedam, N. (2019a). Global-scale dispersal and connectivity in mangroves. PNAS 116, 915–922. doi: 10.1073/pnas.1812470116
Van der Stocken, T., and Menemenlis, D. (2017). Modelling mangrove propagule dispersal trajectories using high-resolution estimates of ocean surface winds and currents. Biotropica 49, 472–481. doi: 10.1111/btp.12440
Van der Stocken, T., Ryck, D. J. R. D., Vanschoenwinkel, B., Deboelpaep, E., Bouma, T. J., Dahdouh-Guebas, F., et al. (2015). Impact of landscape structure on propagule dispersal in mangrove forests. Mar. Ecol. Prog. Ser. 524, 95–106. doi: 10.3354/meps11206
Van der Stocken, T., Vanschoenwinkel, B., Ryck, D. D., and Koedam, N. (2018). Caught in transit: offshore interception of seafaring propagules from seven mangrove species. Ecosphere 9:e02208. doi: 10.1002/ecs2.2208
Van der Stocken, T., Wee, A. K. S., Ryck, D. J. R. D., Vanschoenwinkel, B., Friess, D. A., Dahdouh-Guebas, F., et al. (2019b). A general framework for propagule dispersal in mangroves. Biol. Rev. 94, 1547–1575. doi: 10.1111/brv.12514
Vanden Broeck, A., Van Landuyt, W., Cox, K., De Bruyn, L., Gyselings, R., Oostermeijer, G., et al. (2014). High levels of effective long-distance dispersal may blur ecotypic divergence in a rare terrestrial orchid. BMC Ecol. 14:20. doi: 10.1186/1472-6785-14-20
Wee, A. K. S., Low, S. Y., and Webb, E. L. (2015). Pollen limitation affects reproductive outcome in the bird-pollinated mangrove Bruguiera gymnorrhiza (Lam.) in a highly urbanized environment. Aquat. Bot. 120, 240–243. doi: 10.1016/j.aquabot.2014.09.001
Wee, A. K. S., Noreen, A. M. E., Ono, J., Takayama, K., Kumar, P. P., Tan, H. T. W., et al. (2020). Genetic structures across a biogeographical barrier reflect dispersal potential of four Southeast Asian mangrove plant species. J. Biogeogr. 47, 1258–1271. doi: 10.1111/jbi.13813
Wright, S. (1984). Evolution and the Genetics of Populations, Volume 2: Theory of Gene Frequencies. 1st edn. Chicago, IL: University of Chicago Press Available online at: https://press.uchicago.edu/ucp/books/book/chicago/E/bo5961634.html (accessed June 15, 2021).
Keywords: long distance dispersal (LDD), transboundary dispersal, ocean currents, estuarine geomorphology, seascape ecology, coastal wetland, microsatellite markers, assignment test
Citation: Ngeve MN, Koedam N and Triest L (2021) Genotypes of Rhizophora Propagules From a Non-mangrove Beach Provide Evidence of Recent Long-Distance Dispersal. Front. Conserv. Sci. 2:746461. doi: 10.3389/fcosc.2021.746461
Received: 23 July 2021; Accepted: 02 September 2021;
Published: 08 October 2021.
Edited by:
Yoshiaki Tsuda, University of Tsukuba, JapanReviewed by:
Li Yu, Yunnan University, ChinaKevin Kit Siong Ng, Forest Research Institute Malaysia (FRIM), Malaysia
Copyright © 2021 Ngeve, Koedam and Triest. This is an open-access article distributed under the terms of the Creative Commons Attribution License (CC BY). The use, distribution or reproduction in other forums is permitted, provided the original author(s) and the copyright owner(s) are credited and that the original publication in this journal is cited, in accordance with accepted academic practice. No use, distribution or reproduction is permitted which does not comply with these terms.
*Correspondence: Magdalene N. Ngeve, magngeve@gmail.com