- 1Department of Cognitive Science, University of California, San Diego, La Jolla, CA, United States
- 2Global Health Program, Department of Anthropology, University of California, San Diego, La Jolla, CA, United States
- 3Department of Anesthesiology, San Diego School of Medicine, University of California, San Diego, La Jolla, CA, United States
Background: Explanations for why social media users propagate misinformation include failure of classical reasoning (over-reliance on intuitive heuristics), motivated reasoning (conforming to group opinion), and personality traits (e.g., narcissism). However, there is a lack of consensus on which explanation is most predictive of misinformation spread. Previous work is also limited by not distinguishing between passive (i.e., “liking”) and active (i.e., “retweeting”) propagation behaviors.
Methods: To examine this issue, 858 Twitter users were recruited to engage in a Twitter simulation task in which they were shown real tweets on public health topics (e.g., COVID-19 vaccines) and given the option to “like”, “reply”, “retweet”, “quote”, or select “no engagement”. Survey assessments were then given to measure variables corresponding to explanations for: classical reasoning [cognitive reflective thinking (CRT)], motivated reasoning (religiosity, political conservatism, and trust in medical science), and personality traits (openness to new experiences, conscientiousness, empathy, narcissism).
Results: Cognitive reflective thinking, conscientiousness, openness, and emotional concern empathy were all negatively associated with liking misinformation, but not significantly associated with retweeting it. Trust in medical scientists was negatively associated with retweeting misinformation, while grandiose narcissism and religiosity were positively associated. An exploratory analysis on engagement with misinformation corrections shows that conscientiousness, openness, and CRT were negatively associated with liking corrections while political liberalism, trust in medical scientists, religiosity, and grandiose narcissism were positively associated. Grandiose narcissism was the only factor positively associated with retweeting corrections.
Discussion: Findings support an inhibitory role for classical reasoning in the passive spread of misinformation (e.g., “liking”), and a major role for narcissistic tendencies and motivated reasoning in active propagating behaviors (“retweeting”). Results further suggest differences in passive and active propagation, as multiple factors influence liking behavior while retweeting is primarily influenced by two factors. Implications for ecologically valid study designs are also discussed to account for greater nuance in social media behaviors in experimental research.
1 Introduction
Breakthroughs in communication technologies have historically been tied to massive societal change on a global scale. Inventions such as the printing press and TV expand the reach and accessibility of other people's thoughts, knowledge, and experiences, and often radically challenge cultural norms that impact existing political and social systems (Postman, 2005). The emergence and wide-spread adoption of the internet in recent years has led to a new technological era, where the average citizen can now instantly send a message across the planet with a few strokes of a finger. However, the same capabilities that facilitate rapid communication at relatively low costs are unfortunately used to evoke fear and confusion by disseminating rumors, conspiracies, and false information. Efforts to address false information online has been an ongoing concern since the early days of the internet (Eysenbach, 2002, 2009), and has grown into an ubiquitous issue in public discourse due to its prevalence on social media platforms.
The present state of misinformation research is heavily colored by the COVID-19 pandemic: since the emergence of COVID-19, misinformation related to the novel coronavirus proliferated across social media sites such as Twitter (currently “X”) with topics ranging from sensational rumors about its origin (e.g., signals emitted by 5G towers) (Bruns et al., 2020, 2022; Haupt et al., 2023), claims about the efficacy of debunked treatments (Haupt et al., 2021a; Mackey et al., 2021), and false claims about the COVID-19 vaccine (Lee et al., 2022; Zhao et al., 2023). However, misinformation propagation has long been an issue in public health discourse. For example, false claims that the MMR (measles, mumps, rubella) vaccine causes autism (Kata, 2010, 2012) were subsequently associated with measles outbreaks among unvaccinated children in the US (Nelson, 2019) and more widespread outbreaks in Greece and the United Kingdom (Robert et al., 2019). The topic of misinformation was also prominent during the 2016 US Presidential election, when misinformation concerning the election was prevalent on social media platforms (Bovet and Makse, 2019; Budak, 2019). In recent years, it has become increasingly difficult to distinguish between political and health-related misinformation, as public health debates have been used to exacerbate political discord across party lines as reflected in COVID-19 discourse surrounding masks, treatments, and vaccines (Bruine de Bruin et al., 2020; Kerr et al., 2021; Levin et al., 2023; Pennycook et al., 2022).
Despite ongoing research efforts investigating the spread of false information online, there remain multiple explanations in the literature for the large-scale volume of misinformation that continues to propagate on social media (Chen et al., 2023; Ecker et al., 2022; Wang et al., 2019). Within the field of cognitive science, there are two competing theoretical accounts for misinformation spreading behaviors. These include a failure to engage in classical reasoning (Pennycook and Rand, 2019, 2021) and a tendency for motivated reasoning (i.e., conformity to group opinions) (Kahan et al., 2017; Osmundsen et al., 2021). By contrast, psychologists have appealed to personality traits such as narcissism to explain misinformation propagation (Sternisko et al., 2021; Vaal et al., 2022). An ongoing challenge is that this literature is balkanized, with some researchers arguing misinformation propagation is attributable to a single primary cause (Pennycook and Rand, 2019, 2021; Osmundsen et al., 2021) and others failing to consider alternative explanations (Wang et al., 2019). The present study aims to test all three explanations for engagement with misinformation by quantifying and comparing effect sizes within an experimental setting.
1.1 Lack of classical reasoning
One of the more popular explanations for misinformation propagation is the classical reasoning or “inattention” account that claims people spread misinformation unintentionally due to a lack of careful reasoning and relevant knowledge (Pennycook and Rand, 2019, 2021). Because they lack the time and energy to adequately assess the large volume of content on social media, people use heuristics or mental shortcuts that lead to the spread of misinformation (van der Linden, 2022).
The classical reasoning account draws on a dual-process theory of human cognition that posits two distinct reasoning processes: System 1 is predominantly automatic, associative, and intuitive, while System 2 is more analytical, reflective, and deliberate (Evans, 2003). According to this account, misinformation spread is due to an overreliance on System 1 processing and can be mitigated by interventions that activate System 2-type reasoning (Pennycook and Rand, 2021). The most common metric used to operationalize System 2-type reasoning is the Cognitive Reflection Test (CRT), a set of word problems in which the answer that comes “first to mind” is wrong, while the correct answer requires one to pause and carefully reflect (Frederick, 2005). CRT is widely used in the misinformation literature to measure individuals' propensity to engage in analytic thinking. Accordingly, CRT scores have been shown to be positively correlated with truth discernment of headlines (Pennycook and Rand, 2019), and negatively associated with sharing low credibility news sources on Twitter (Mosleh et al., 2021). Based on the literature, we hypothesize that:
H1: CRT is negatively associated with liking/retweeting misinformation
Despite the large body of evidence supporting the classical reasoning account, this explanation is limited in that it does not consider the influence of social factors such as group identity (e.g., political affiliation) or how users relate to others socially (e.g., being narcissistic or highly empathetic). Since sharing information to one's network is a social behavior, exclusively focusing on the recognition of misinformation may not fully account for all factors associated with online propagation behaviors. It is also worth noting that while CRT is often used in the literature to operationalize the classical reasoning account, it is a proxy measure that does not directly reflect one's capacity to engage in System 2-type thinking, but rather one's tendency to do so. Rather than a direct measure of classical reasoning ability such as IQ, CRT indexes individuals' tendency to engage in reflective thinking. Given that those adept at System 2-type thinking may nonetheless fail to exert the energy required to deliberate on the content of a potentially false post, the tendency to engage in reflective thinking before deciding to share a post is far more relevant for misinformation propagation.
1.2 Motivated reasoning
An alternative explanation for misinformation propagation that is also based on dual-process theory is the motivated reasoning account, which states that individuals tend to assess information based on pre-determined goals, and to selectively endorse and share content that coincides with those goals (Kahan, 2015; van der Linden, 2022). These goals could involve: maintaining a positive self-image Dunning, 2003, avoiding anxiety from unwelcome news (Dawson et al., 2002), and rationalizing self-serving behavior (Hsee, 1996). In politically motivated reasoning, the goal is presumed to be identity protection, as people form beliefs that maintain their connection to a social group with shared values (Kahan, 2015). Consequently, social media users may be motivated to share factually incorrect posts that align with their beliefs and group identities, and to ignore posts that challenge those beliefs. Indeed, research suggests participants' political identities influence their recognition of misinformation on controversial issues (Kahan et al., 2017), as well as their willingness to share political fake news (Osmundsen et al., 2021).
Relative to classical reasoning, motivated reasoning adopts a somewhat different perspective on rationality and its role in social behavior. Like classical reasoning, motivated reasoning suggests misinformation susceptibility results from an overreliance on System 1 processing (i.e., intuitive response). However, the failure to engage System 2 (i.e., deliberation) results not from a lack of effort or ability, but from the desire to maintain a political identity. Although motivated reasoners may have different beliefs, they all utilize an optimal procedure for updating those beliefs when considering new information. The Bayesian inference framework involves a “prior”, that is, an existing estimate of the probability of some factual hypothesis (e.g., getting vaccinated for COVID-19 will prevent the spread of the virus), novel information or evidence (e.g., a new study showing evidence of vaccine efficacy), a “likelihood ratio” that reflects how much more consistent the new information is with the relevant hypothesis than some rival one, and a revised estimate reflecting the weight given to the new information (Kahan, 2015). Individuals display politically motivated reasoning when they utilize a likelihood ratio that weighs priors aligned with their political identity higher than factual evidence.
Within the context of COVID-related misinformation, those higher in conservatism have been shown to be more likely to resist taking COVID-19 precautions (Conway et al., 2021; Havey, 2020), more susceptible to health-related misinformation (Calvillo et al., 2020, 2021; Kaufman et al., 2022), and more likely to share low credibility news sources (DeVerna et al., 2022; Guess et al., 2019). While the literature mostly focuses on politically motivated reasoning, there are other relevant bias factors concerning COVID-related misinformation that are often overlooked. Previous studies showing that lower trust in science is associated with greater likelihood to believe in COVID-19 misinformation narratives indicate that motivated reasoning may also be driven by distrust toward medical scientists (Agley and Xiao, 2021; Pickles et al., 2021; Roozenbeek et al., 2020). Despite there being no religious scriptures that directly address suspicion to scientific institutions, higher religious belief within the US (more specifically Christianity) has been associated with science skepticism (Azevedo and Jost, 2021) and susceptibility to conspiracy theories and false news (Bronstein et al., 2019; Frenken et al., 2023). Therefore, those higher in religiosity may be more likely to evaluate evidence as factual if it is in opposition to scientific institutions. Based on the existing literature of factors that may evoke motivated reasoning for COVID-related misinformation, we tested the following hypotheses:
H2: Political conservatism is positively associated with liking/retweeting misinformation
H3: Religiosity is positively associated with liking/retweeting misinformation
H4: Trust in medical scientists is negatively associated with liking/retweeting misinformation
1.3 Personality traits
An explanation for misinformation spread that does not draw upon dual-process theory attributes misinformation propagation to personality traits. Using measures such as narcissism and other “dark triad” traits of psychopathy, researchers in this tradition argue that engagement with conspiracy theories results from interpersonal and affective deficits, unusual patterns of cognition, and manipulative social promotion strategies (Barron et al., 2018; Bruder et al., 2013; Cichocka et al., 2016; Douglas and Sutton, 2011; Hughes and Machan, 2021; March and Springer, 2019; P.Y.K.L. et al., 2022; Vaal et al., 2022). For example, susceptibility and dissemination of conspiracy theories related to COVID-19 has been associated with collective narcissism (Hughes and Machan, 2021; Sternisko et al., 2021). Also referred to as “national narcissism,” collective narcissism is tied to the belief that one's ingroup is exceptional, deserves special treatment, and that others do not sufficiently recognize it (de Zavala et al., 2009, 2019). Because they have a national image of invulnerability and self-sufficiency, collective narcissists may be attracted to COVID-related conspiracies that deny the disease's existence.
There are also more personal variants of narcissism that are underexamined in the misinformation propagation literature. Grandiose narcissism is a dispositional trait characterized by an unrealistically positive self-view, a strong self-focus, and a lack of regard for others (Miller et al., 2011), and is associated both with greater activity on social media platforms (Gnambs and Appel, 2018; McCain et al., 2016; McCain and Campbell, 2018) and higher incidence of belief in conspiracy theories (Cichocka et al., 2016). Covert or “vulnerable” narcissism in contrast, reflects an insecure sense of grandiosity that obscures feelings of inadequacy, incompetence, and negative affect (Miller et al., 2011), and tends to involve social behavior that is characterized by a lack of empathy, higher social sensitivity, and increased frequency of social media use (Dickinson and Pincus, 2003; Fegan and Bland, 2021). Individuals high in narcissistic traits were less likely to comply with COVID-related guidelines (e.g., wearing a mask) due to an unwillingness to make personal sacrifices for the benefit of others, a desire to stand out from consensus behavior, a tendency to engage in paranoid thinking, and a need to maintain a sense of control in response to government-imposed regulations (Hatemi and Fazekas, 2022; Sternisko et al., 2021; Vaal et al., 2022). Based on previous work examining the effects of narcissism on social media engagement and COVID-19 compliance, we hypothesize:
H5: Narcissism (Grandiose and Covert) is positively associated with liking/retweeting misinformation
As opposed to narcissistic individuals, those higher in empathy may be more concerned about the wellbeing of others, and thus less likely to share health-related misinformation. This is suggested in previous work showing that empathetic messaging interventions can correct erroneous beliefs (Moore-Berg et al., 2022), improve misinformation discernment (Lo, 2021), and reduce the incidence of online hate speech (Hangartner et al., 2021). However, there is currently no work examining how trait empathy influences COVID-related misinformation propagation on social media. Based on findings from related work on empathy and communication behaviors, we hypothesize:
H6: Empathy is negatively associated with liking/retweeting misinformation
Other relevant personality traits to misinformation propagation, as highlighted in a recent review (Sindermann et al., 2020), are conscientiousness and openness from the Big Five Inventory (BFI). Conscientiousness, which measures the tendency to be organized, exhibit self-control, and to think before acting (Jackson et al., 2010), has been shown to be negatively correlated with disseminating misinformation (Lawson and Kakkar, 2022). Openness to experience, which assesses one's intellectual curiosity and propensity to try new things, has been shown to be negatively associated with belief in myths (Swami et al., 2016) and positively associated with tendencies to scrutinize information (Fleischhauer et al., 2010). Both BFI traits are also positively associated with better news discernment (Calvillo et al., 2021). While previous research examines these traits separately, there is currently no work that tests these traits against other relevant factors for misinformation propagation. Based on the existing literature, we hypothesize:
H7: BFI Conscientiousness and Openness are negatively associated with liking/retweeting misinformation
1.4 Present study
If we hope to design effective interventions to minimize the propagation of misinformation on social media platforms, it is important to understand how and why it occurs. Unfortunately, there is almost no contact between misinformation researchers in the “cognitive” traditions (classical vs. motivated reasoning) and those in the more “social” traditions that focus on personality traits (Chen et al., 2023). The existence of these parallel tracks of inquiry presents a need to compare the influence of the variety of factors that contribute to misinformation spreading behaviors. Although some researchers have advocated a unitary explanation of online misinformation spread (Pennycook and Rand, 2019, 2021), others have argued there are likely multiple factors at play (Batailler et al., 2022; Chen et al., 2023; Ecker et al., 2022). Here we test all three major accounts and quantify their relative importance for online misinformation propagation. In addition to testing the previously mentioned hypotheses for predicting effects of individual variables, we also propose open-ended research questions to further frame our analysis:
RQ1: Which individual traits of users are the strongest predictors of passive propagation (i.e., liking) of misinformation posts?
RQ2: Which individual traits of users are the strongest predictors of active propagation (i.e., retweeting) of misinformation posts?
One limitation of previous research is the use of outcome measures that do not correspond to social media behaviors, such as the headline evaluation task in which participants rate the truthfulness of news headlines (Bago et al., 2020; Pennycook and Rand, 2019; Ross et al., 2021; Vegetti and Mancosu, 2020). Consequently, some have questioned the external validity of headline evaluation tasks, calling for more realistic experimental settings that resemble social media environments (Sindermann et al., 2020). Relatedly, it is important for researchers to distinguish between passive social media use (e.g., scrolling through the newsfeed without engaging with posts) and more active behaviors (e.g., sharing a post on your profile) (Burke et al., 2011; Verduyn et al., 2015, 2017; Yu, 2016). Clearly, deciding whether to retweet a post, which will broadcast it to other users on the site, is not the same as privately rating a news headline on its truthfulness.
To address these shortcomings, the present study employs a Twitter simulation task in which participants are shown real tweets from COVID-19 discourse and asked to engage with them (e.g., “retweet”) as they would on the platform. A similar task has been used in previous research examining misinformation susceptibility for platforms such as Facebook, Twitter, and Reddit (Bode and Vraga, 2018; Mourali and Drake, 2022; Porter and Wood, 2022; Tully et al., 2020), and for eliciting responses from participants on issues related to early COVID-19 quarantine guidelines (Coulson and Haupt, 2021). Rather than allowing participants to engage directly with misinformation stimuli, these prior studies have used simulated newsfeeds to expose participants to misinformation and then asked them in a separate section to rate their belief in the information or intention to share. The present study differs from past work in that our main outcome measure is direct engagement with posts within the simulated newsfeed. Analysis will focus on “liking” to observe more passive forms of social media propagation, and “retweeting” (i.e., sharing) COVID-related misinformation since retweeting is a more active form of propagation in the real world. See Table 1 and Figure 1 in Methods for examples of tweet stimuli and simulation task.
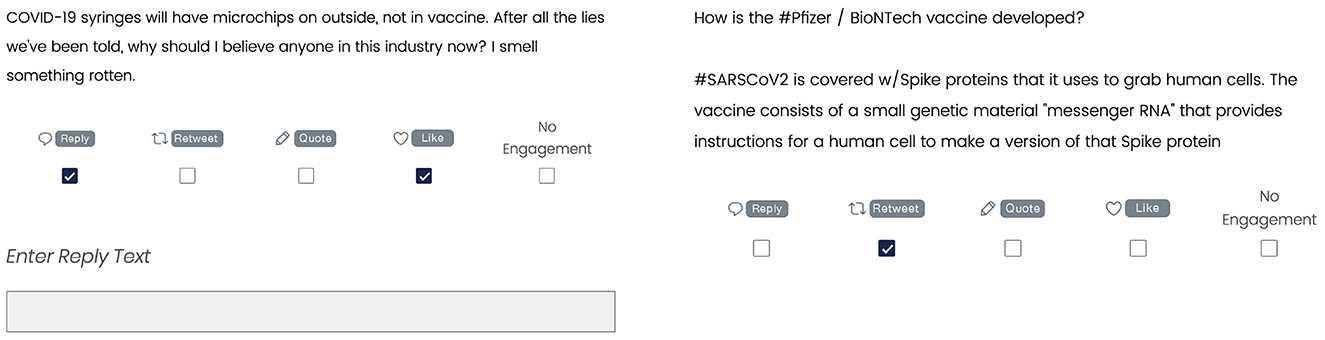
Figure 1. Example tweets from Twitter simulation task, (left) vaccine misinformation tweet, (right) vaccine misinformation correction tweet.
While researchers have identified multiple types of misinformation which ranges from propaganda, misleading advertising, news parody and satire, manipulated news, and news that has been completely fabricated (Baptista and Gradim, 2022; Tandoc et al., 2018; Waszak et al., 2018), within the current study misinformation was defined based on whether it made a declarative statement about a false claim related to each health-related topic according to scientific consensus at the time of data collection. For instance, the tweet “Btw hydroxychloroquine cures covid” is considered misinformation since scientific consensus during the study period (September 2021) was that the anti-malaria drug hydroxychloroquine was not an effective treatment for COVID-19. We further distinguish our definition of misinformation from disinformation, which is false information sent with the intention to deceive or manipulate others, and “fake news,” which is disinformation that has the appearance and format of journalistic reports from news outlets (Baptista and Gradim, 2022). As our study focuses on factors influencing propagating behaviors of message receivers, intentions and journalistic affiliations of the post senders are not considered.
Despite there being multiple studies that investigate how individual traits influence misinformation spreading behaviors, effects related to whether one shares misinformation corrections are understudied, as reflected in a recent call for research (Vraga and Bode, 2020). Since the propagation of misinformation corrections is underexamined, here we conduct an exploratory analysis to inform future work. A misinformation correction was defined as a tweet that directly counters false rumors or provides factual information concerning a topic. See Supplementary Table S6 for specific hypotheses on how we expect the tested factors in the current study to influence corrections engagement. We also propose more general research questions similar to those previously outlined for misinformation engagement:
RQ3: Which individual traits of users are the strongest predictors of passive propagation (i.e., liking) of correction posts?
RQ4: Which individual traits of users are the strongest predictors of active propagation (i.e., retweeting) of correction posts?
Our approach is to assess the adequacy of these theoretical accounts of misinformation propagation on social media by quantifying relevant factors using psychometrically validated scales. The relative importance of these variables on misinformation propagating behavior will then be modeled using multivariate regression. See Table 2 for a full list of tested variables and hypotheses, and Methods for description of tested variables. If CRT is the only variable that is negatively associated with liking and retweeting misinformation tweets, then those results would indicate that the classical reasoning account is the primary explanation for propagation. Evidence supporting the motivated reasoning account would show positive associations between higher conservatism, higher religiosity, and lower trust in medical scientists with liking and retweeting COVID-related misinformation. If misinformation engagement is positively associated with narcissism traits and negatively associated with empathy and BFI traits, results would support the personality traits account.
The regression analysis adopted here allows us to recognize the impact of multiple factors and to quantify their relative importance for the propagation of misinformation. In the case where variables from multiple accounts show similar effect sizes that are statistically significant, then the results would suggest there is no singular explanation for online misinformation spread, but rather multiple factors that influence different aspects of propagating behaviors. Differences in observed effects for passive vs. active propagation (liking and retweeting) would also suggest a more nuanced view of factors influencing engagement behaviors on social media. However, it is difficult to form predictions based on the literature, as previous work examining the influence of dispositional traits on engagement behaviors often do not delineate effects attributed to passive and active propagation. Since liking is a less public behavior than retweeting, we expect that traits that are more socially oriented (i.e., narcissism, empathy) and reflect group identity (i.e., political orientation, religiosity) would have larger effect sizes when predicting active propagation.
2 Methods
2.1 Data collection
One thousand Twitter users were recruited from Amazon Mechanical Turk (MTurk) during September 2021. Participants were considered Twitter users if they reported having a Twitter account. After filtering for data quality, a final sample of 858 participants was used for analysis. Data quality was based on failing an attention check question (“In order to make sure you're paying attention please select option five”) and having a survey completion time in the top 10th percentile (<12 min, median survey completion time = 26.85 minutes). Of the total sample, 60% identified as male with a mean age of 37.26 (SD = 10.22). Further, 76% were White, 14% Black, 4% Asian, and 2% Hispanic. Median income was between $50,000 to $74,999 and participants reported spending an average of 3.18 hours per day on social media (SD = 2.2). Ethics approval for this study was granted by University of California, San Diego. Informed consent was obtained from participants before taking the survey and the research was conducted in accordance with guidelines for posting a survey on the platform. Participants were compensated based on standard survey-taking rates on Amazon Mechanical Turk and no personal identifying information is reported in this study. The data and materials necessary to reproduce the findings reported in this manuscript are available on the Open Science Framework (OSF) repository (osf.io/nv28f).
2.2 Twitter simulation task
Participants engaged in a Twitter simulation task where they were asked to “like”, “reply”, “retweet”, “quote”, or select “no engagement” for tweets related to three public health topics (vaccines, hydroxychloroquine, masks). Similar to actual Twitter use, participants were able to select multiple reactions to a tweet (or to simply select “no engagement”). If “reply” or “quote” was selected, a text box would appear under the tweet for participants to generate a written response. Tweets were presented in random order. See Figure 1 for examples of the Twitter simulation task. 36 tweets were tested in the simulation task with 12 tweets for each public health topic. For each topic, four of the tweets contained misinformation and two misinformation corrections, resulting in a total of 12 misinformation and six correction tweets tested in the simulated newsfeed. A higher number of misinformation posts were tested than corrections to better reflect newsfeed dynamics, where previous studies show that misinformation is more prevalent than factual information on Twitter (Haupt et al., 2021b; Shin et al., 2018; Vosoughi et al., 2018; Zarocostas, 2020). Six additional tweets on each topic were also tested; these tweets neither contained misinformation nor were they corrections but expressed varying sentiment toward the topic (two positive, two negative, two neutral). See (Kaufman et al., 2022) for further description of tweets not focused on in the present study, and Table 1 for examples of tweets used in the current analysis. As shown in Kaufman et al. (2022), these tweet stimuli were also used in a separate study where 9.32 of the 12 misinformation tweets on average were correctly classified as containing misinformation by a sample of 132 undergraduate students.
2.3 Classical reasoning
2.3.1 Cognitive reflective thinking (CRT)
Three questions from the Cognitive Reflection Test were used to measure CRT (α = 0.79). Questions from this test initially have an answer that appears “intuitive”. However, producing the correct answer requires careful reflection. For example, Question 1 asks “A bat and a ball cost $1.10 in total. The bat costs $1.00 more than the ball. How much does the ball cost?” The intuitive answer is 10 cents, while the correct answer is 5 cents (since $1.05 is $1 more than 5 cents and together they total $1.10). Number of correct answers corresponds to higher CRT score.
2.4 Motivated reasoning
To examine how motivated reasoning influences engagement with misinformation posts, this study measures political orientation and two other factors relevant to bias in politicized health-related misinformation: trust in medical scientists and religiosity.
2.4.1 Political orientation
A question asking participants about their political beliefs, with 1 = Very Conservative to 6 = Very Liberal.
2.4.2 Trust in medical scientists
One 4-item scale adapted from the 2019 Pew Research Center's American Trends Panel survey (Funk et al., 2019) asking participants “How much confidence, if any, do you have in each of the following to act in the best interests of the public?” Participants were given response options ranging from 1 = (“No confidence at all”) to 4 = (“A great deal”) to rate their confidence in the following institutions: elected officials, news media, medical scientists, and religious leaders. Trust in medical scientists was the only item examined in the present analysis.
2.4.3 Religiosity
One 7-item scale (α = 0.87) from the Centrality of Religiosity Scale (Huber and Huber, 2012), which measures the intensity, salience, and importance or centrality of religious meanings for an individual. The interreligious version was used for the current study.
2.5 Personality traits
2.5.1 Narcissism
Grandiose narcissism was measured using the 16-item version (α = 0.81) of the Narcissism Personality Inventory (NPI) (Ames et al., 2006). Each item in the NPI-16 asks participants to select which of a pair of statements describes them most closely. Grandiose narcissism scores are calculated based on the number of statements selected that are consistent with narcissism. Covert narcissism was measured using the 10-item Hypersensitive Narcissism Scale (Hendin and Cheek, 1997) with each item rated on a 5-point Likert scale ranging from 1 (“very uncharacteristic or untrue, strongly disagree”) to 5 (“very characteristic or true, strongly agree”) (α = 0.89).
2.5.2 Empathy
The current study tests two types of empathy: perspective-taking and emotional-concern. Perspective-taking (PT) empathy refers to the capacity to make inferences about and represent others' intentions, goals, and motives (Frith and Frith, 2005; Stietz et al., 2019). Emotional-concern (EC) refers to other-oriented emotions elicited by the perceived welfare of someone in need (Batson, 2009). Empathy was assessed by having participants respond to Perspective Taking (PT) (α=0.60) and Emotional Concern (EC) (α = 0.71) subscales taken directly from the Interpersonal Reactivity Index (Davis, 1983), with each item rated on a 5-point Likert scale ranging from 1 (“does not describe me well”) to 5 (“describes me well”).
2.5.3 BFI conscientiousness and openness
Two 8-item subscales from the Big Five Inventory (BFI) (John et al., 2008) were used to evaluate participants across the personality dimensions conscientiousness (α = 0.72) and openness (α = 0.65). Each item was rated on a 5-point Likert scale ranging from 1 (“Disagree strongly”) to 5 (“Agree strongly”).
2.6 Regression analysis
Multiple regression analysis was conducted to assess the relationship between tested traits and engagement with misinformation tweets. A Shapley value regression was also implemented that allows us to determine the proportion of variance attributed to each independent variable when controlled for multicollinearity (Budescu, 1993). Originally used in economics (Israeli, 2007; Lipovetsky and Conklin, 2001), this technique has been used in data science both for interpreting machine learning models (Covert and Lee, 2021; Okhrati and Lipani, 2021; Smith and Alvarez, 2021) and for evaluating how dispositional traits of crowd workers influence accuracy of detecting misinformation in Twitter posts (Kaufman et al., 2022).
3 Results
3.1 Distribution of engagement behaviors
Overall, passive engagement behavior occurred more often than active behavior with participants being more likely to “Like” than to “Retweet,” “Quote,” or “Reply” to posts containing both misinformation and corrections. Participants on average liked half of the total misinformation tweets (median = 6). By contrast, on average only 1.5 of the 12 misinformation tweets were retweeted. Correction tweets received more engagement on average with two-thirds of correction tweets being liked and 1 out of 6 retweeted. See Supplementary Table S1 for full descriptive statistics and Figures 2, 3 for distribution of behaviors from simulation task.
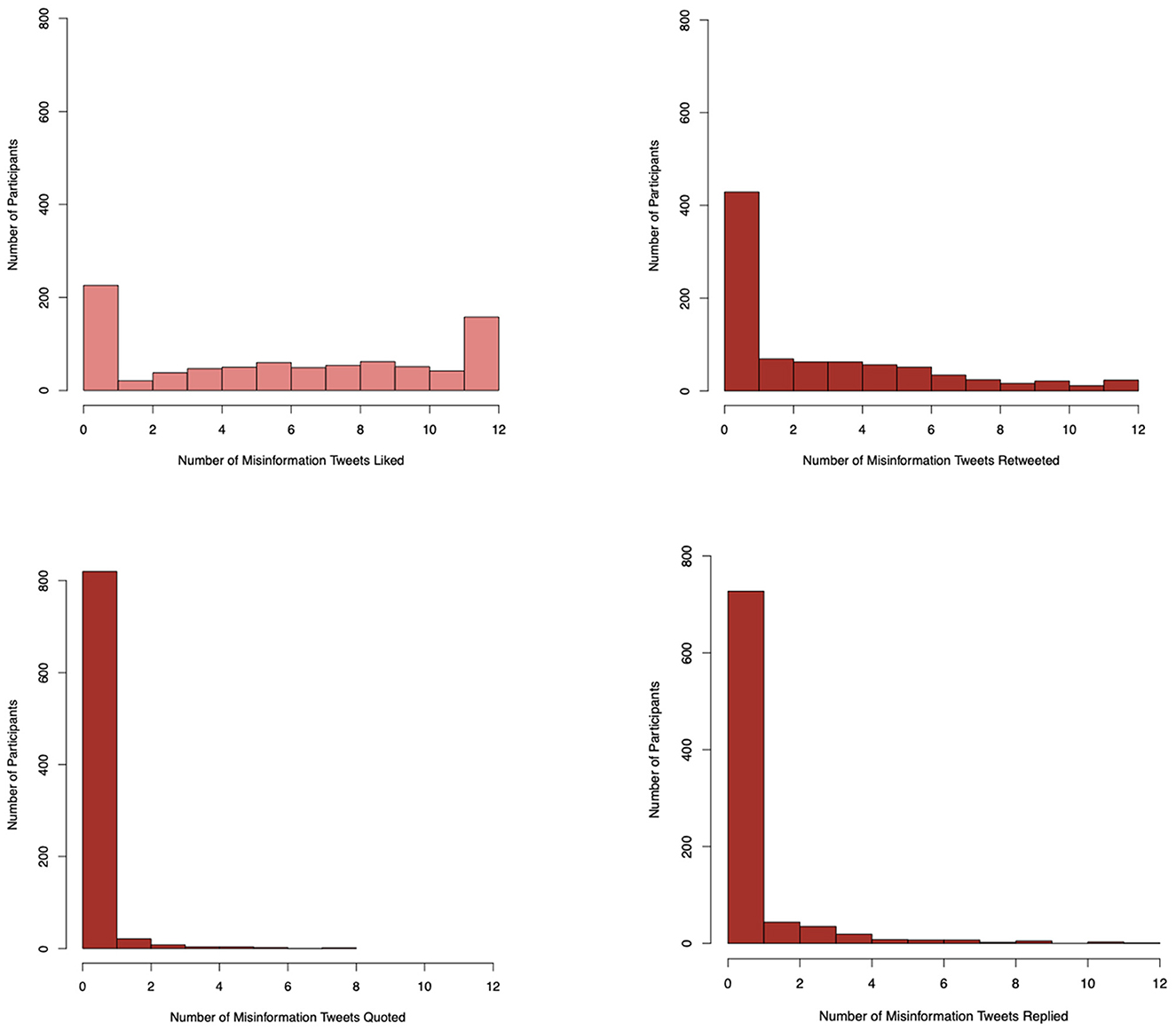
Figure 2. Distribution of engagement (Like, Retweet, Quote, Reply) with misinformation tweets. This figure shows the distribution for the different engagement behaviors when shown misinformation tweets. Passive behavior (i.e., Like) is depicted in a lighter color than the more active behaviors (e.g., Retweet). Liking misinformation tweets shows a bimodal distribution, where many participants either did not like any of the misinformation tweets (n = 181) or liked all 12 of them (n = 158). However, most of the sample fell between these two extremes (see upper left panel). For retweeting behavior, a large portion of participants did not retweet any misinformation posts (n = 363), and the vast majority of participants retweeted 6 or fewer misinformation tweets (n = 729). Participants were also less likely to Reply or Quote a post than Like and Retweet. Since replying and quoting also require users to generate a written response to the tweet, participants may be less likely to initiate these behaviors due to the extra costs in effort and time.
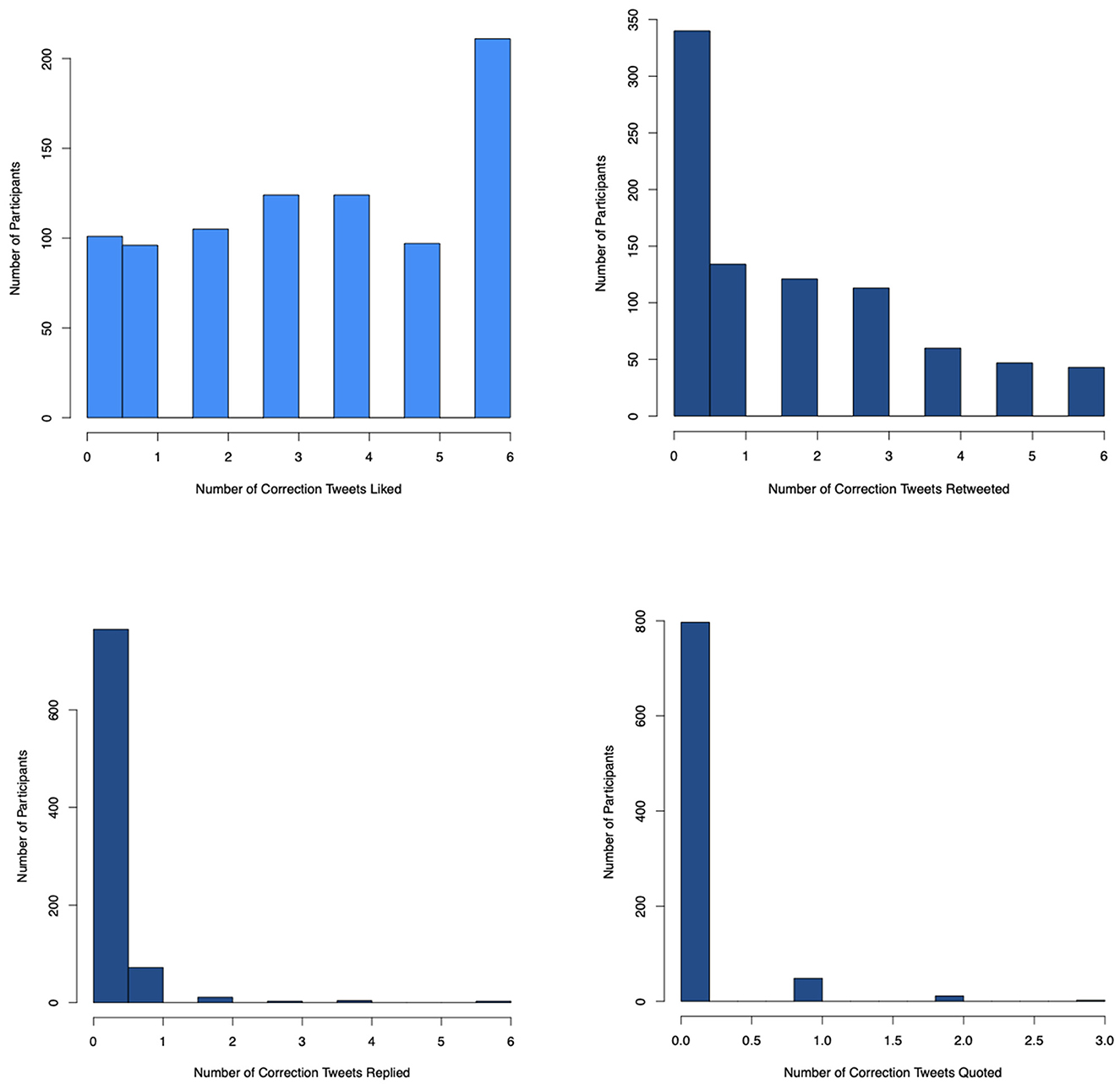
Figure 3. Distribution of engagement (Like, Retweet, Quote, Reply) with misinformation correction tweets. This figure shows the distribution for the different engagement behaviors when shown tweets that correct misinformation. Passive behavior (i.e., Like) is depicted in a lighter color than the more active behaviors (e.g., Retweet). Liking correction posts resembles more of a normal distribution compared to liking misinformation (see Figure 2). However, there is also a sizable proportion of participants who liked all 6 correction tweets (n = 211), which skews the distribution toward the right. The distribution for retweeting correction posts is similar to that for retweeting misinformation with a large portion of participants not retweeting any at all (n = 340) and the majority of participants retweeting half or less of the available correction tweets. Similarly to misinformation tweets, participants were also less likely to Reply or Quote a correction post than Like and Retweet.
3.2 Logistic mixed effects regression model
To control for individual variation in participants and stimuli, mixed effects models were run to assess the likelihood of liking and retweeting posts, as shown in Table 3. The fixed effects were the information classification (e.g., misinformation) while the random effects were variation from individual respondents and tweet stimuli. For information classifications, the reference level is neutral sentiment tweets. The dependent variables are whether the participant liked or retweeted each tweet. Before modeling, the data was restructured from the participant level to the tweet level, resulting in 30,888 observations (858 participants × 36 tweets).
Models of both liking and retweeting posts showed that variance from individual participants was greater than the variance from tweet stimuli. Compared to neutral sentiment, participants were less likely to like tweets classified as misinformation (−0.47 logits, p <0.05) and problematic sentiment (−0.52 logits, p < 0.05), and more likely to like nonproblematic sentiment (0.45 logits, <0.05). For the retweet model, participants were less likely to retweet misinformation (-0.37 logits, <0.001) and problematic sentiment tweets (−0.32 logits, p < 0.01) compared to neutral sentiment. Within both models, correction tweets showed no statistically significant differences in engagement compared to neutral posts. Since engagement with corrections is indistinguishable from neutral sentiment engagement, the remainder of the analysis will focus on examining misinformation propagating behaviors. See Supplementary Discussion S1 for further interpretation on engagement with correction tweets.
3.3 Regression analysis—Engagement with misinformation
Multivariate regression was conducted with measures outlined above as independent variables, and the number of misinformation tweets that were liked and retweeted as dependent variables. To determine which variable contributes most to tweet engagement, we used a Shapley value regression, which assesses the relative importance of the independent variables by computing all possible combinations of variables within the model and recording how much the R2 changes with the addition or subtraction of each variable [(see Groemping, 2007) for further description using an example dataset with a higher degree of multicollinearity than the current analysis, and Supplementary Table S2 and Supplementary Discussion S2 for correlation matrix of tested variables]. Shapley weights were standardized in Table 4A to show the proportion of the model's total R2 that is attributed to each tested variable.
As shown in Tables 4A, B, personality traits and religiosity were among the most influential for predicting passive engagement with misinformation tweets. When controlled for the other variables in the model, CRT was negatively associated with liking misinformation tweets (p < 0.001). Based on the results from the Shapley value regression, CRT ranks 5th in explaining variance for liking misinformation. However, CRT shows no significant effect in the retweet model, indicating classical reasoning shows an inhibitory effect on passive but not active forms of misinformation propagation.
Among traits relevant for the motivated reasoning account, religiosity was positively associated with both liking (β = 0.85, p < 0.001) and retweeting misinformation (β = 0.52, p < 0.001), while trust in medical scientists was negatively associated with these behaviors (liking: β = −0.31, p < 0.05; retweeting: β = −0.34, p < 0.01). Based on the Shapley results, religiosity explained the second highest amount of variance for both liking and retweeting misinformation. Political orientation had no statistically significant effects and ranked lowest in predictor strength for both liking and retweeting misinformation when controlled for all tested variables.
Among variables testing the personality trait account, grandiose narcissism was positively associated both with liking (β = 2.49) and retweeting (β = 3.27) misinformation (p < 0.001). Grandiose narcissism ranked as the top predictor in the retweet model and 4th for liking misinformation. Covert narcissism showed no statistically significant effects for liking or retweeting misinformation when controlled for all tested variables. EC empathy was the only empathy trait to have a statistically significant effect and was negatively associated with liking misinformation (β = −0.63, p < 0.05). When controlled for the other variables in the model, BFI Conscientiousness and Openness were both negatively associated with liking misinformation tweets (p < 0.001). Based on the results from the Shapley value regression, Openness ranks as the variable that explains the most variance in liking misinformation and Conscientiousness ranks 3rd among the tested variables. Neither BFI traits show significant associations with retweeting misinformation.
Additionally, the adjusted r-squared of the models suggest that the selected predictor variables performed better when predicting the more passive engagement behavior of liking (R2 = 0.52) than for the more active engagement behavior of retweeting (R2 = 0.15).
4 Discussion
The results from the current study suggest there is no singular explanation for online misinformation spread, but rather there are multiple factors influencing different types of propagating behavior. CRT, which corresponded to the classical reasoning account, was negatively associated with liking misinformation and explained a moderate amount of variance in this behavior, suggesting that tendencies to engage in deliberative processes have an inhibitory effect on passive propagation. However, CRT was unrelated to retweeting. In accordance with predictions of motivated reasoning, religiosity was positively associated with sharing COVID-19 misinformation (both like and retweet), while trust in medical science was negatively associated. Contrary to what is indicated in the literature, the motivated reasoning account was driven primarily by religious-based motivations rather than political ones. In fact, after controlling for all other variables, political orientation had no significant association with any form of engagement. Grandiose narcissism was associated with both liking and retweeting misinformation, in keeping with accounts that suggest personality traits lead to misinformation propagation. Among the tested factors, narcissistic tendencies and religiosity showed the strongest association with active misinformation spreading behaviors. See Table 2 that outlines support shown for each tested hypothesis related to misinformation engagement and Supplementary Table S6 for corrections engagement.
As revealed in the present study, the variance attributed to grandiose narcissism dwarfs effect sizes associated with CRT and political orientation for retweeting misinformation. When considering the proposed research questions, multiple variables corresponding to all 3 accounts are predictive of passive propagation and are similar in effect size (RQ1) while active propagation was primarily associated with one variable corresponding to personality (grandiose narcissism) and to a lesser extent motivated reasoning (religiosity) (RQ2). Similar findings are shown for corrections engagement, where 7 of the 10 tested variables showed a significant effect for liking (RQ3) (CRT: β = −0.29, p < 0.001; Conscientiousness: β = −0.49, p < 0.001; Openness: β = −0.46, p < 0.01; Religiosity: β =0.20, p < 0.001; Grandiose Narcissism: β =0.91, p < 0.01; Trust in Med: β =0.20, p < 0.01; Political: β =0.10, p < 0.05) but only grandiose narcissism was associated with retweeting (β = 1.45, p < 0.001)(RQ4) (see Supplementary Table S5 for full corrections model). This indicates that passive propagation is influenced by a greater number of factors while the variance of active propagation is more concentrated within one to two socially related traits.
Results suggest that there are multiple causes for liking misinformation on social media, arguing against a unitary cognitive account of misinformation spread. Notably, one contributing factor—driven by people with low CRT scores and low BFI conscientiousness scores—is the failure to perceive it as misinformation. Our results also suggest motivated reasoning contributes to passive misinformation spread since these predictors (religiosity and trust in medical science) accounted for substantial variance even when controlling for CRT. Finally, our study suggested that personality factors (grandiose narcissism and EC empathy) also contribute to passive misinformation spread. The multiple effects observed here for passive propagation is in line with previous research investigating social meanings of online behaviors, which shows that the decision to like a post can reflect a variety of factors including motivations for gift giving and impression management, and whether users perceive post content as humorous, newsworthy, useful, and authentic (Hong et al., 2017; Syrdal and Briggs, 2018).
Moreover, the factors associated with more active propagation mechanisms differed substantively from those for passive propagation—being limited to Grandiose Narcissism, Religiosity, and Trust in Medical Science. This highlights the importance of social signaling as a key motivator for the active spread of misinformation and implies that interventions for tagging particular tweets as misinformation may not be effective unless the source of the tag is tied to a cultural identity respected by users. Indeed, because the motivation of narcissistic individuals may be to attract attention from other users, explicit “misinformation” labels may make active engagement behaviors more attractive rather than less. The prominence of the effect of grandiose narcissism on retweeting when controlled for other factors related to classical and motivated reasoning indicate that personality traits are highly relevant for active propagating behaviors. Accordingly, these personality traits should be considered when designing interventions to attenuate misinformation spread.
4.1 Implications for personality trait account
The positive association between grandiose narcissism and retweeting misinformation may be driven in part by beliefs of individuals high in grandiose narcissism, as they were less likely to comply with COVID-related guidelines (Hatemi and Fazekas, 2022; Vaal et al., 2022) and are more likely to engage in conspiratorial thinking (Cichocka et al., 2016). However, it is also possible that the observed effects are attributed to the greater tendency of these individuals to be active on social media (Gnambs and Appel, 2018; McCain et al., 2016; McCain and Campbell, 2018). This is suggested when considering the supplementary findings (Supplementary Table S4), where a regression model examining retweet engagement on all 36 tested tweets in the simulation exercise (with 24 not containing misinformation) shows that grandiose narcissism is the strongest predictor of retweeting all tweet types, and is highly significant (p < 0.001). Supplementary Table S3 also shows no statistically significant difference in retweeting misinformation between narcissistic conservatives and narcissistic liberals, further suggesting that political beliefs may not drive engagement. See Supplementary Discussion S3 for more interpretation of these findings, and Supplementary Discussion S2 for discussion of correlations between tested variables. For corrections engagement, grandiose narcissism was the only tested variable significantly associated with retweeting correction posts (Supplementary Table S5). While this effect may be attributed to higher engagement tendencies as well, there are other potential explanations suggested by the literature: those higher in grandiose narcissism could be more likely to retweet corrections due to receiving positive attention from other users, as grandiose narcissists can act as “strategic helpers” by engaging in prosocial behaviors in order to increase their esteem through attention or praise (Konrath et al., 2016), which includes helping behaviors during the COVID-19 pandemic (Freis and Brunell, 2022). For further discussion of the other tested personality traits, see Supplementary Discussion S4 for additional interpretation of effects associated with covert narcissism, and BFI conscientiousness and openness.
4.2 Implications for motivated reasoning account
Religiosity was the second most influential factor for retweeting misinformation, which also provides support for the motivated reasoning account. Since the majority of the sample identified as Christian (78.2%), higher religiosity refers primarily to those with stronger Christian beliefs. Despite political conservatism showing a significant correlation with misinformation engagement when tested alone using bivariate correlations (Supplementary Table S7), its influence disappears when controlled for religiosity and the other tested factors. Although religiosity and political orientation are generally correlated with each other (Jost et al., 2014), the findings from this study indicate religiosity is a more influential factor for the propagation of COVID-related misinformation. Researchers examining misinformation propagation in future work should consider measuring and controlling for effects from religiosity when investigating politically-based motivated reasoning.
The present results are only partly consistent with previous studies (Azevedo and Jost, 2021; Frenken et al., 2023) that show religiosity and conservatism are both associated with scientific trust and conspiracy theory endorsement, even when controlled for each other. This inconsistency may be due to differences in outcome measures and tested covariates from previous work (Agley and Xiao, 2021). Lastly, trust in medical scientists was negatively associated with both liking and retweeting misinformation, in keeping with results reported in other studies investigating misinformation susceptibility (Agley and Xiao, 2021; Pickles et al., 2021; Roozenbeek et al., 2020). However, the effect size from medical scientist trust is relatively small compared to those for grandiose narcissism and religiosity, suggesting it should be a lower priority for targeting when designing interventions.
4.3 Implications for classical reasoning account
Findings from the present study reveal a noteworthy limitation for the classical reasoning account. CRT only showed a negative effect on liking misinformation tweets, which indicates that those who have a higher tendency to engage in deliberative processes are less likely to interact with posts containing false information. This is consistent with previous work showing that CRT is associated with better news discernment (Pennycook and Rand, 2019; Mosleh et al., 2021). However, when observing the most direct form of propagation on social media (i.e., retweeting), CRT showed no statistically significant effects. Other personality traits (i.e., conscientiousness, openness, and empathy) when controlled for CRT show a similar inhibitory effect on passive misinformation propagation but no association with retweeting. In general, the lack of any significant effects for CRT, conscientiousness, openness, and empathy with active spreading behavior suggest they are less important variables in misinformation propagation. Although liking is relevant to propagation dynamics since it influences the content that gets promoted by newsfeed algorithms, identifying factors that influence more direct forms of propagation are more integral to understanding large-scale misinformation spread.
4.4 Integrating explanations for misinformation propagation
While we detected distinct effects from personality traits and cognitive processes, the three accounts tested in this study could be integrated. As suggested by the present findings, narcissistic tendencies may be the underlying driver of misinformation propagation while classical and motivated reasoning processes could be the cognitive mechanisms that individuals engage in when sharing online content. For example, the insecure nature of those higher in narcissistic tendencies may make them more likely to engage in motivated reasoning to maintain their identity and self-esteem. This is further suggested when considering that the conceptual distinctions between narcissistic tendencies and motivated reasoning are blurred in the case of collective narcissism, that is, the belief that one's ingroup is exceptional and deserves special treatment (Nowak et al., 2020). In collective narcissism, over adherence to an identity leads to preferential and biased behaviors that prioritize gains to the in-group over the wellbeing of society (Sternisko et al., 2021). This is exactly the proposed mechanism for why people engage in politically (or other group-based) motivated reasoning (see e.g., Kahan, 2015). Since grandiose narcissism shows distinct variance from motivated reasoning factors in the current study, effective interventions for addressing misinformation spread stemming from nationalistic variants of narcissism should consider accounting for motivated reasoning processes that might be recruited to protect group-related identities held by users.
It may also be possible that those higher in trait narcissism are more likely to employ classical reasoning during social interactions to further their goals. This is echoed in a related theory for misinformation propagation described as the motivated numeracy account, which claims those who are more capable of engaging in deliberative processes are in fact more likely to show biased thinking due to being better equipped at selecting information that aligns with pre-existing beliefs (Kahan et al., 2012, 2017; van der Linden, 2022). In this view, trait narcissism could be the main driver of misinformation propagation while classical and motivated reasoning correspond to cognitive processes underlying engagement behavior. From this perspective, trait narcissism could be conceptualized as a tendency to engage in self-focused cognitions, where classical and motivated reasoning processes are then recruited to maintain one's identity. However, this integrated framework needs to be further tested by conceptually clarifying differences between traits and cognitive tendencies and accounting for the lack of effects from covert narcissism. Since covert narcissism is also defined by a high degree of self-centeredness but showed no significant effects on engagement, this suggests that grandiose narcissism accounts for variance beyond self-focused cognitions. Overall, grandiose narcissism may be a stronger factor for predicting active engagement because it measures interpersonal relational styles with others, making it more relevant for predicting communication behaviors than measures only accounting for cognitive tendencies.
4.5 Future directions
One relatively novel contribution of the present study was the inclusion of dependent measures both for passive (viz. like responses) and more active (viz. retweet) propagation behaviors. Results demonstrate that these more active measures that are far more consequential for real-world misinformation propagation were also less likely to elicit responses from our participants. These findings from the simulation exercise correspond to differences in passive and active behaviors observed in real-world Twitter activity since the majority of social media users spend most of their time browsing and not publicly engaging with content (Benevenuto et al., 2009; Lerman and Ghosh, 2010; Sun et al., 2014; Van Mieghem et al., 2011). In sum, using a social media simulation task allowed us to detect more nuanced effects than headline evaluation tasks or rating scales measuring engagement frequency.
Researchers interested in adapting simulated newsfeed exercises would benefit from considering the effects of platforms features on information sharing behaviors and the social significance attributed to online actions by users. In fields such as user experience (UX) research, interactions between users and technology features are referred to as affordances, which are defined as multifaceted relational structures between a technology and a user that enable or constrain potential behavioral outcomes within a particular context (Evans et al., 2017; Haupt et al., 2022; Hutchby, 2001). Adopting an affordance lens allows researchers to recognize the mutual influence between users and environments (Gibson, 2014), and can add further nuance in the interpretations of analyzed behaviors in experiments simulating online environments (Wang and Sundar, 2022). For instance, if an analysis focuses on an affordance for message propagation, then this framework would identify retweeting as the most relevant outcome measure. If the research question focuses on understanding factors influencing affordances for dialogue between users, then replying to a tweet would be identified as the most relevant behavior since replies facilitate direct conversation between users on a post. Overall, researchers investigating propagation behaviors should adapt newsfeed simulation tasks to better reflect behaviors occurring within online environments and consider affordances to account for the meaning of behaviors on social media platforms. For those interested in adapting simulated newsfeeds, see the following open-source platform created to address the lack of ecologically valid social media testing paradigms (Butler et al., 2023).
Discrepancies between studies in the misinformation literature may be attributed to the lack of distinction made between passive and active propagation behaviors. As observed in the present findings, CRT was negatively associated with liking misinformation. Since liking is a more private behavior compared to sharing, it is possible that deciding to like a post reflects a personal evaluation of the content. If assumed that liking corresponds to truth evaluation, then these findings would be consistent with misinformation work using truthfulness ratings of news headlines as an outcome variable (Pennycook and Rand, 2019). The lack of observed effects for CRT on retweeting when controlled for personality and motivated reasoning factors also corresponds to findings in previous work using measures of active, socially-oriented behaviors such as sharing links (Osmundsen et al., 2021). Evidence from a prior study using the same tweet stimuli from the current analysis further suggests that passive engagement behaviors reflect cognitive processes that correspond to truth evaluation tasks. In this previous study, where the main outcome variable measured misinformation detection accuracy on a task more reminiscent of headline evaluation, CRT was a much stronger predictor for accurately classifying misinformation for the same tested tweets (Kaufman et al., 2022). These findings from the misinformation detection task aligns more with the results for liking misinformation observed in the current study. In order to shed further light on the psychological significance of liking misinformation on social media, future work is needed to examine the correspondence of truth evaluation processes with passive propagation behaviors.
The distinction between influential and non-influential users can also be important for disentangling effects related to each theoretical explanation. Previous work shows that the position of users within a network can be a relevant factor in misinformation propagation as online misinformation discourse is typically driven by a handful of influential accounts (Grinberg et al., 2019; Haupt et al., 2021a,b). Discrepancies in the literature could be caused by not considering network positions of users, where those who are hosts of political cable tv news shows may post a low credible news article due to narcissistic tendencies while their less influential followers may reshare the post due to motivated reasoning.
4.6 Limitations
The present study addresses limitations from previous work by simulating a social media environment instead of using less generalizable tasks such as headline evaluations. However, the tweet simulation exercise still does not fully capture how users may act in real-world environments. For example, the fact that participants knew that selecting “retweet” would not actually share the tweet to their follower's timelines limits the extent to which these results reflect actual propagation behavior. Despite this limitation, participant engagement in the simulation is consistent with reported differences in passive vs active behaviors on actual social media platforms (Benevenuto et al., 2009; Lerman and Ghosh, 2010; Sun et al., 2014; Van Mieghem et al., 2011), suggesting that participants adhered to the exercise prompt instead of randomly selecting engagement options. Another limitation is that we did not account for factors associated with characteristics of post senders, such as their political and professional affiliations, level of fame and influence, and profile pictures. Characteristics of the post itself, such as number of likes and retweets it receives (which are subsequently displayed to others), can also influence engagement behaviors. In the present work, we specifically focused on propagation of messages by only displaying text. Follow-up work interested in accounting for sender and post characteristics can adapt the recently released open-source platform to develop more advanced newsfeed simulations (Butler et al., 2023).
4.7 Concluding remarks
While there is a tendency for scientific researchers to frame questions implying there is only one “correct” answer, online misinformation spread is likely too complex for a single explanation. The present study provides partial support for all the tested accounts in that reasoning ability was negatively associated with passive misinformation engagement, grandiose narcissism was positively associated with active engagement, and factors related to group identity exhibited effects in the predicted directions for the COVID-related misinformation content. Since narcissistic tendencies and religious-based motivated reasoning showed the strongest association with the most direct form of misinformation propagation (i.e., retweeting), interventions should focus particularly on users high in these traits.
Ultimately, the decision to share a post is a social behavior, whether the intent is to genuinely inform others, signal a social identity, or evoke emotional reactions. While much prior research has treated online misinformation spread as mainly related to how people assess what is true, the fact that these interactions occur on social media platforms embed these actions within contexts where users typically consider how their actions are perceived by others. To fully investigate online propagation behaviors, the influential role of socially oriented traits, group identity, and the social contexts of online environments need to be taken into account.
Data availability statement
The datasets presented in this study can be found in online repositories. The names of the repository/repositories and accession number(s) can be found in the article/Supplementary material.
Ethics statement
The studies involving humans were approved by University of California, San Diego IRB. The studies were conducted in accordance with the local legislation and institutional requirements. The participants provided their written informed consent to participate in this study. The social media data was accessed and analyzed in accordance with the platform's terms of use and all relevant institutional/national regulations.
Author contributions
MH: Conceptualization, Data curation, Formal analysis, Funding acquisition, Investigation, Methodology, Writing – original draft, Writing – review & editing. RC: Writing – review & editing. TM: Funding acquisition, Writing – review & editing. SC: Funding acquisition, Methodology, Supervision, Writing – review & editing.
Funding
The author(s) declare financial support was received for the research, authorship, and/or publication of this article. This research was supported by the seed grant program at the Sanford Institute for Empathy and Compassion (No. 2007392).
Conflict of interest
The authors declare that the research was conducted in the absence of any commercial or financial relationships that could be construed as a potential conflict of interest.
Publisher's note
All claims expressed in this article are solely those of the authors and do not necessarily represent those of their affiliated organizations, or those of the publisher, the editors and the reviewers. Any product that may be evaluated in this article, or claim that may be made by its manufacturer, is not guaranteed or endorsed by the publisher.
Supplementary material
The Supplementary Material for this article can be found online at: https://www.frontiersin.org/articles/10.3389/fcomm.2024.1472631/full#supplementary-material
References
Agley, J., and Xiao, Y. (2021). Misinformation about COVID-19: evidence for differential latent profiles and a strong association with trust in science. BMC Public Health 21:89. doi: 10.1186/s12889-020-10103-x
Ames, D. R., Rose, P., and Anderson, C. P. (2006). The NPI-16 as a short measure of narcissism. J. Res. Pers. 40, 440–450. doi: 10.1016/j.jrp.2005.03.002
Azevedo, F., and Jost, J. T. (2021). The ideological basis of antiscientific attitudes: Effects of authoritarianism, conservatism, religiosity, social dominance, and system justification. Group Proc. Intergroup Relat. 24, 518–549. doi: 10.1177/1368430221990104
Bago, B., Rand, D. G., and Pennycook, G. (2020). Fake news, fast and slow: deliberation reduces belief in false (but not true) news headlines. J. Exp. Psychol.: General 149, 1608–1613. doi: 10.1037/xge0000729
Baptista, J. P., and Gradim, A. (2022). A working definition of fake news. Encyclopedia 2, 632–645. doi: 10.3390/encyclopedia2010043
Barron, D., Furnham, A., Weis, L., Morgan, K. D., Towell, T., and Swami, V. (2018). The relationship between schizotypal facets and conspiracist beliefs via cognitive processes. Psychiatry Res. 259, 15–20. doi: 10.1016/j.psychres.2017.10.001
Batailler, C., Brannon, S. M., Teas, P. E., and Gawronski, B. (2022). A signal detection approach to understanding the identification of fake news. Persp. Psychol. Sci. 17, 78–98. doi: 10.1177/1745691620986135
Batson, C. D. (2009). “Two forms of perspective taking: Imagining how another feels and imagining how you would feel,” in Handbook of Imagination and Mental Simulation (New York, NY: Psychology Press), 267–279.
Benevenuto, F., Rodrigues, T., Cha, M., et al. (2009). “Characterizing user behavior in online social networks,” in Proceedings of the 9th ACM SIGCOMM conference on Internet measurement (New York, NY: Association for Computing Machinery), 49–62.
Bode, L., and Vraga, E. K. (2018). See something, say something: correction of global health misinformation on social media. Health Commun. 33, 1131–1140. doi: 10.1080/10410236.2017.1331312
Bovet, A., and Makse, H. A. (2019). Influence of fake news in Twitter during the 2016 US presidential election. Nat. Commun. 10:7. doi: 10.1038/s41467-018-07761-2
Bronstein, M. V., Pennycook, G., Bear, A., Rand, D. G., and Cannon, T. D. (2019). Belief in fake news is associated with delusionality, dogmatism, religious fundamentalism, and reduced analytic thinking. J. Appl. Res. Mem. Cogn. 8, 108–117. doi: 10.1016/j.jarmac.2018.09.005
Bruder, M., Haffke, P., Neave, N., Nouripanah, N., and Imhoff, R. (2013). Measuring individual differences in generic beliefs in conspiracy theories across cultures: conspiracy mentality questionnaire. Front. Psychol. 4:225. doi: 10.3389/fpsyg.2013.00225
Bruine de Bruin, W., Saw, H.-W., and Goldman, D. P. (2020). Political polarization in US residents' COVID-19 risk perceptions, policy preferences, and protective behaviors. J. Risk Uncertain. 61, 177–194. doi: 10.1007/s11166-020-09336-3
Bruns, A., Harrington, S., and Hurcombe, E. (2020). ‘Corona? 5G? or both?': the dynamics of COVID-19/5G conspiracy theories on Facebook. Media Int. Aust. 177, 12–29. doi: 10.1177/1329878X20946113
Bruns, A., Hurcombe, E., and Harrington, S. (2022). Covering conspiracy: approaches to reporting the COVID/5G conspiracy theory. Digital J. 10, 930–951. doi: 10.1080/21670811.2021.1968921
Budak, C. (2019). “What happened? The spread of fake news publisher content during the 2016 U.S. Presidential Election,” in The World Wide Web Conference, WWW '19 (New York, NY: Association for Computing Machinery), 139–150. doi: 10.1145/3308558.3313721
Budescu, D. V. (1993). Dominance analysis: a new approach to the problem of relative importance of predictors in multiple regression. Psychol. Bullet. 114, 542–551. doi: 10.1037//0033-2909.114.3.542
Burke, M., Kraut, R., and Marlow, C. (2011). “Social capital on facebook: differentiating uses and users,” in Proceedings of the SIGCHI Conference on Human Factors in Computing Systems (New York, NY: Association for Computing Machinery), 571–580.
Butler, L. H., Lamont, P., Wan, D. L. Y., Prike, T., Nasim, M., Walker, B., et al. (2023). The (Mis)Information game: a social media simulator. Behav. Res. Methods. 11:2023. doi: 10.3758/s13428-023-02153-x
Calvillo, D. P., Garcia, R. J. B., Bertrand, K., and Mayers, T. (2021). Personality factors and self-reported political news consumption predict susceptibility to political fake news. Pers. Individ. Dif. 174:110666. doi: 10.1016/j.paid.2021.110666
Calvillo, D. P., Ross, B. J., Garcia, R. J. B., Smelter, T. J., and Rutchick, A. M. (2020). Political ideology predicts perceptions of the threat of covid-19 (and susceptibility to fake news about it. Social Psychol. Personal. Sci. 11, 1119–1128. doi: 10.1177/1948550620940539
Chen, S., Xiao, L., and Kumar, A. (2023). Spread of misinformation on social media: what contributes to it and how to combat it. Comput. Human Behav. 141:107643. doi: 10.1016/j.chb.2022.107643
Cichocka, A., Marchlewska, M., and de Zavala, A. G. (2016). Does self-love or self-hate predict conspiracy beliefs? Narcissism, self-esteem, and the endorsement of conspiracy theories. Social Psychol. Personal. Sci. 7, 157–166. doi: 10.1177/1948550615616170
Conway, L. G., Woodard, S. R., Zubrod, A., and Chan, L. (2021). Why are conservatives less concerned about the coronavirus (COVID-19) than liberals? Comparing political, experiential, and partisan messaging explanations. Pers. Individ. Dif. 183:111124. doi: 10.1016/j.paid.2021.111124
Coulson, S., and Haupt, M. R. (2021). “Frame semantics variation,” in Frame Semantics Variation (Berlin: De Gruyter Mouton), 81–94. doi: 10.1515/9783110733945-007
Covert, I., and Lee, S.-I. (2021). “Improving KernelSHAP: practical shapley value estimation using linear regression,” in Proceedings of The 24th International Conference on Artificial Intelligence and Statistics (New York: PMLR), 3457–3465. Available at: https://proceedings.mlr.press/v130/covert21a.html (accessed July 12, 2023).
Davis, M. H. (1983). Measuring individual differences in empathy: Evidence for a multidimensional approach. J. Personal. Soc. Psychol. 44, 113–126. doi: 10.1037//0022-3514.44.1.113
Dawson, E., Gilovich, T., and Regan, D. T. (2002). Motivated reasoning and performance on the was on selection task. Pers. Soc. Psychol. Bull. 28, 1379–1387. doi: 10.1177/014616702236869
de Zavala, A., Dyduch-Hazar, K., and Lantos, D. (2019). Collective narcissism: political consequences of investing self-worth in the ingroup's image. Polit. Psychol. 40, 37–74. doi: 10.1111/pops.12569
de Zavala, A. G., Cichocka, A., Eidelson, R., et al. (2009). Collective narcissism and its social consequences. J. Persona. Soc. Psychol. 97, 1074–1096. doi: 10.1037/a0016904
DeVerna, M. R., Guess, A. M., Berinsky, A. J., Tucker, J. A., and Jost, J. T. (2022). “Rumors in retweet: ideological asymmetry in the failure to correct misinformation,” in Personality and Social Psychology Bulletin (Thousand Oaks, CA: SAGE Publications Inc).
Dickinson, K. A., and Pincus, A. L. (2003). Interpersonal analysis of grandiose and vulnerable narcissism. J. Personal. Disord. 17, 188–207. doi: 10.1521/pedi.17.3.188.22146
Douglas, K. M., and Sutton, R. M. (2011). Does it take one to know one? Endorsement of conspiracy theories is influenced by personal willingness to conspire. Br. J. Soc. Psychol. 50, 544–552. doi: 10.1111/j.2044-8309.2010.02018.x
Dunning, D. (2003). “The zealous self-affirmer: how and why the self lurks so pervasively behind social judgment,” in Motivated Social Perception: The Ontario Symposium, Vol. 9. Ontario Symposium on Personality and Social Psychology (Mahwah, NJ: Lawrence Erlbaum Associates Publishers), 45–72.
Ecker, U. K. H., Lewandowsky, S., Cook, J., Schmid, P., Fazio, L., Brashier, N., et al. (2022). The psychological drivers of misinformation belief and its resistance to correction. Nat. Revi. Psychol. 1, 13–29. doi: 10.1038/s44159-021-00006-y
Evans, J. St. B. T. (2003). In two minds: dual-process accounts of reasoning. Trends Cogn. Sci. 7, 454–459. doi: 10.1016/j.tics.2003.08.012
Evans, S. K., Pearce, K. E., Vitak, J., and Treem, J. (2017). Explicating affordances: a conceptual framework for understanding affordances in communication research. J. Comp.-Med. Commun. 22, 35–52. doi: 10.1111/jcc4.12180
Eysenbach, G. (2002). Infodemiology: the epidemiology of (mis)information. Am. J. Med. 113, 763–765. doi: 10.1016/S0002-9343(02)01473-0
Eysenbach, G. (2009). Infodemiology and infoveillance: framework for an emerging set of public health informatics methods to analyze search, communication and publication behavior on the internet. J. Med. Internet Res. 11:e11. doi: 10.2196/jmir.1157
Fegan, R. B., and Bland, A. R. (2021). Social media use and vulnerable narcissism: the differential roles of oversensitivity and egocentricity. Int. J. Environ. Res. Public Health 18:9172. doi: 10.3390/ijerph18179172
Fleischhauer, M., Enge, S., Brocke, B., Strobel, A., and Strobel, A. (2010). Same or different? clarifying the relationship of need for cognition to personality and intelligence. Personal. Soc. Psychol. Bullet. 36, 82–96. doi: 10.1177/0146167209351886
Frederick, S. (2005). Cognitive reflection and decision making. J. Econ. Perspect. 19:25–42. doi: 10.1257/089533005775196732
Freis, S. D., and Brunell, A. B. (2022). Narcissistic motivations to help during the COVID-19 quarantine. Pers. Individ. Dif. 194:111623. doi: 10.1016/j.paid.2022.111623
Frenken, M., Bilewicz, M., and Imhoff, R. (2023). On the relation between religiosity and the endorsement of conspiracy theories: the role of political orientation. Polit. Psychol. 44, 139–156. doi: 10.1111/pops.12822
Frith, C., and Frith, U. (2005). Theory of mind. Curr. Biol. 15, 644–R645. doi: 10.1016/j.cub.2005.08.041
Funk, C., Hefferon, M., Kennedy, B., and Johnson, C. (2019). “Trust and mistrust in Americans' views of scientific experts,” in Pew Research Center Science & Society. Available at: https://www.pewresearch.org/science/2019/08/02/trust-and-mistrust-in-americans-views-of-scientific-experts/ (accessed September 18, 2023).
Gibson, J. J. (2014). The Ecological Approach to Visual Perception: Classic Edition. New York: Psychology Press.
Gnambs, T., and Appel, M. (2018). Narcissism and social networking behavior: a meta-analysis. J. Pers. 86, 200–212. doi: 10.1111/jopy.12305
Grinberg, N., Joseph, K., Friedland, L., Swire-Thompson, B., and Lazer, D. (2019). Fake news on Twitter during the 2016 U.S. presidential election. Science 363, 374–378. doi: 10.1126/science.aau2706
Groemping, U. (2007). Relative Importance for Linear Regression in R: The Package relaimpo. J. Stat. Softw. 17, 1–27. doi: 10.18637/jss.v017.i01
Guess, A., Nagler, J., and Tucker, J. (2019). Less than you think: prevalence and predictors of fake news dissemination on Facebook. Sci. Adv. 5:eaau4586. doi: 10.1126/sciadv.aau4586
Hangartner, D., Gennaro, G., Alasiri, S., Bahrich, N., Bornhoft, A., Boucher, J., et al. (2021). Empathy-based counterspeech can reduce racist hate speech in a social media field experiment. Proc. Nat. Acad. Sci. 118:e2116310118. doi: 10.1073/pnas.2116310118
Hatemi, P. K., and Fazekas, Z. (2022). The role of grandiose and vulnerable narcissism on mask wearing and vaccination during the COVID-19 pandemic. Curr. Psychol. 14:2022. doi: 10.1007/s12144-022-03080-4
Haupt, M. R., Chiu, M., Chang, J., Li, Z., Cuomo, R., and Mackey, T. K. (2023). Detecting nuance in conspiracy discourse: Advancing methods in infodemiology and communication science with machine learning and qualitative content coding. PLoS ONE 18:e0295414. doi: 10.1371/journal.pone.0295414
Haupt, M. R., Cuomo, R., Li, J., Nali, M., and Mackey, T. (2022). The influence of social media affordances on drug dealer posting behavior across multiple social networking sites (SNS). Comp. Human Behav. Rep. 8:100235. doi: 10.1016/j.chbr.2022.100235
Haupt, M. R., Jinich-Diamant, A., Li, J., Nali, M., and Mackey, T. K. (2021a). Characterizing twitter user topics and communication network dynamics of the “Liberate” movement during COVID-19 using unsupervised machine learning and social network analysis. Online Soc. Netw. Media 21:100114. doi: 10.1016/j.osnem.2020.100114
Haupt, M. R., Li, J., and Mackey, T. K. (2021b). Identifying and characterizing scientific authority-related misinformation discourse about hydroxychloroquine on twitter using unsupervised machine learning. Big Data Soc. 8:20539517211013843. doi: 10.1177/20539517211013843
Havey, N. F. (2020). Partisan public health: how does political ideology influence support for COVID-19 related misinformation? J. Comput. Soc. Sci. 3, 319–342. doi: 10.1007/s42001-020-00089-2
Hendin, H. M., and Cheek, J. M. (1997). Assessing hypersensitive narcissism: a reexamination of Murray's narcism scale. J. Res. Pers. 31, 588–599. doi: 10.1006/jrpe.1997.2204
Hong, C., Chen, Z., and Li, C. (2017). “Liking” and being “liked”: How are personality traits and demographics associated with giving and receiving “likes” on Facebook? Comp. Human Behav. 68, 292–299. doi: 10.1016/j.chb.2016.11.048
Hsee, C. K. (1996). Elastic justification: how unjustifiable factors influence judgments. Org. Behav. Hum. Decis. Process. 66, 122–129. doi: 10.1006/obhd.1996.0043
Huber, S., and Huber, O. W. (2012). The centrality of religiosity scale (CRS). Religions 3, 710–724. doi: 10.3390/rel3030710
Hughes, S., and Machan, L. (2021). It's a conspiracy: Covid-19 conspiracies link to psychopathy, Machiavellianism and collective narcissism. Pers. Individ. Diff. 171:110559. doi: 10.1016/j.paid.2020.110559
Hutchby, I. (2001). Technologies, texts and affordances. Sociology 35, 441–456. doi: 10.1017/S0038038501000219
Israeli, O. (2007). A Shapley-based decomposition of the R-Square of a linear regression. J. Econ. Inequal. 5, 199–212. doi: 10.1007/s10888-006-9036-6
Jackson, J. J., Wood, D., Bogg, T., Walton, K. E., Harms, P. D., and Roberts, B. W. (2010). What do conscientious people do? Development and validation of the Behavioral Indicators of Conscientiousness (BIC). J. Res. Personal. 44, 501–511. doi: 10.1016/j.jrp.2010.06.005
John, O. P., Naumann, L. P., and Soto, C. J. (2008). “Paradigm shift to the integrative Big Five trait taxonomy: History, measurement, and conceptual issues,” in Handbook of Personality: Theory and Research, 3rd Ed (New York, NY: The Guilford Press), 114–158.
Jost, J. T., Hawkins, C. B., Nosek, B. A., et al. (2014). Belief in a just God (and a just society): a system justification perspective on religious ideology. J. Theoret. Philosoph. Psychol. 34, 56–81. doi: 10.1037/a0033220
Kahan, D. M. (2015). The Politically Motivated Reasoning Paradigm. 2703011, SSRN Scholarly Paper. Rochester, NY. Available at: https://papers.ssrn.com/abstract=2703011 (accessed September 14, 2022).
Kahan, D. M., Peters, E., Dawson, E. C., and Slovic, P. (2017). Motivated numeracy and enlightened self-government. Behav. Public Policy 1, 54–86. doi: 10.1017/bpp.2016.2
Kahan, D. M., Peters, E., Wittlin, M., and Slovic, P. (2012). The polarizing impact of science literacy and numeracy on perceived climate change risks. Nat. Climate Change 2, 732–735. doi: 10.1038/nclimate1547
Kata, A. (2010). A postmodern Pandora's box: anti-vaccination misinformation on the Internet. Vaccine 28, 1709–1716. doi: 10.1016/j.vaccine.2009.12.022
Kata, A. (2012). Anti-vaccine activists, Web 2.0, and the postmodern paradigm - An overview of tactics and tropes used online by the anti-vaccination movement. Vaccine 30, 3778–3789. doi: 10.1016/j.vaccine.2011.11.112
Kaufman, R. A., Haupt, M. R., and Dow, S. P. (2022). Who's in the crowd matters: cognitive factors and beliefs predict misinformation assessment accuracy. Proc. ACM Human-Computer Interact. 6, 1–553:18. doi: 10.1145/3555611
Kerr, J., Panagopoulos, C., and van der Linden, S. (2021). Political polarization on COVID-19 pandemic response in the United States. Pers. Individ. Diff. 179:110892. doi: 10.1016/j.paid.2021.110892
Konrath, S., Ho, M.-H., and Zarins, S. (2016). The strategic helper: narcissism and prosocial motives and behaviors. Curr. Psychol. 35, 182–194. doi: 10.1007/s12144-016-9417-3
Lawson, M. A., and Kakkar, H. (2022). Of pandemics, politics, and personality: the role of conscientiousness and political ideology in the sharing of fake news. J. Exp. Psychol.: General 151, 1154–1177. doi: 10.1037/xge0001120
Lee, S. K., Sun, J., Jang, S., and Connelly, S. (2022). Misinformation of COVID-19 vaccines and vaccine hesitancy. Sci. Rep. 12:13681. doi: 10.1038/s41598-022-17430-6
Lerman, K., and Ghosh, R. (2010). Information contagion: an empirical study of the spread of news on digg and twitter social networks. Proc. Int. AAAI Conf. Web Social Media 4, 90–97. doi: 10.1609/icwsm.v4i1.14021
Levin, J. M., Bukowski, L. A., Minson, J. A., and Kahn, J. M. (2023). The political polarization of COVID-19 treatments among physicians and laypeople in the United States. Proc. Natl. Acad. Sci. U.S.A. 120:e2216179120. doi: 10.1073/pnas.2216179120
Lipovetsky, S., and Conklin, M. (2001). Analysis of regression in game theory approach. Appl. Stochastic Models Busi. Indust. 17, 319–330. doi: 10.1002/asmb.446
Lo, S.-Y. (2021). Empathy reduces susceptibility to false memory. Scient. Rep. 11:22903. doi: 10.1038/s41598-021-02281-4
Mackey, T. K., Purushothaman, V., Haupt, M., Nali, M. C., and Li, J. (2021). Application of unsupervised machine learning to identify and characterise hydroxychloroquine misinformation on Twitter. Lancet Digit. Health 3, e72–e75. doi: 10.1016/S2589-7500(20)30318-6
March, E., and Springer, J. (2019). Belief in conspiracy theories: the predictive role of schizotypy, Machiavellianism, and primary psychopathy. PLoS ONE 14:e0225964. doi: 10.1371/journal.pone.0225964
McCain, J. L., Borg, Z. G., Rothenberg, A. H., Sinatra, M., and Servidio, R. (2016). Personality and selfies: narcissism and the Dark Triad. Comput. Human Behav. 64, 126–133. doi: 10.1016/j.chb.2016.06.050
McCain, J. L., and Campbell, W. K. (2018). Narcissism and social media use: a meta-analytic review. Psychol. Popular Media Cult. 7, 308–327. doi: 10.1037/ppm0000137
Miller, J. D., Hoffman, B. J., Gaughan, E. T., Gentile, B., Maples, J., and Keith Campbell, W. (2011). Grandiose and vulnerable narcissism: a nomological network analysis. J. Pers. 79, 1013–1042. doi: 10.1111/j.1467-6494.2010.00711.x
Moore-Berg, S. L., Hameiri, B., and Bruneau, E. G. (2022). Empathy, dehumanization, and misperceptions: a media intervention humanizes migrants and increases empathy for their plight but only if misinformation about migrants is also corrected. Soc. Psychol. Personal. Sci. 13, 645–655. doi: 10.1177/19485506211012793
Mosleh, M., Pennycook, G., Arechar, A. A., and Rand, D. G. (2021). Cognitive reflection correlates with behavior on Twitter. Nat. Commun. 12:921. doi: 10.1038/s41467-020-20043-0
Mourali, M., and Drake, C. (2022). The challenge of debunking health misinformation in dynamic social media conversations: online randomized study of public masking during COVID-19. J. Med. Internet Res. 24:e34831. doi: 10.2196/34831
Nelson, R. (2019). US measles outbreak concentrated among unvaccinated children. Lancet Infect. Dis. 19:248. doi: 10.1016/S1473-3099(19)30074-X
Nowak, B., Brzóska, P., Piotrowski, J., Sedikides, C., Żemojtel-Piotrowska, M., and Jonason, P. K. (2020). Adaptive and maladaptive behavior during the COVID-19 pandemic: the roles of Dark Triad traits, collective narcissism, and health beliefs. Pers. Individ. Dif. 167:110232. doi: 10.1016/j.paid.2020.110232
Okhrati, R., and Lipani, A. (2021). “A multilinear sampling algorithm to estimate shapley values,” in 2020 25th International Conference on Pattern Recognition (ICPR) (New York, NY: IEEE), 7992–7999.
Osmundsen, M., Bor, A., Vahlstrup, P. B., Bechmann, A., and Petersen, P. (2021). Partisan Polarization is the primary psychological motivation behind political fake news sharing on Twitter. Am. Polit. Sci. Rev. 115, 999–1015. doi: 10.1017/S0003055421000290
P.Y.K.L S Das, A., and Pulabaigari, V. (2022). Fake spreader is narcissist; Real spreader is Machiavellian prediction of fake news diffusion using psycho-sociological facets. Expert Syst. Appl. 207:117952. doi: 10.1016/j.eswa.2022.117952
Pennycook, G., McPhetres, J., Bago, B., and Rand, D. G. (2022). Beliefs about COVID-19 in Canada, the United Kingdom, and the United States: a novel test of political polarization and motivated reasoning. Pers. Soc. Psychol. Bull. 48, 750–765. doi: 10.1177/01461672211023652
Pennycook, G., and Rand, D. G. (2019). Lazy, not biased: Susceptibility to partisan fake news is better explained by lack of reasoning than by motivated reasoning. Cognition 188, 39–50. doi: 10.1016/j.cognition.2018.06.011
Pennycook, G., and Rand, D. G. (2021). The psychology of fake news. Trends Cognit. Sci. 25, 388–402. doi: 10.1016/j.tics.2021.02.007
Pickles, K., Cvejic, E., Nickel, B., Copp, T., Bonner, C., Leask, J., et al. (2021). COVID-19 misinformation trends in Australia: prospective longitudinal national survey. J. Med. Internet Res. 23:e23805. doi: 10.2196/23805
Porter, E., and Wood, T. J. (2022). Political misinformation and factual corrections on the facebook news feed: experimental evidence. J. Polit. 84, 1812–1817. doi: 10.1086/719271
Postman, N. (2005). Amusing Ourselves to Death: Public Discourse in the Age of Show Business. London: Penguin.
Robert, A., Funk, S., and Kucharski, A. J. (2019). The measles crisis in Europe-the need for a joined-up approach. Lancet 393:2033. doi: 10.1016/S0140-6736(19)31039-6
Roozenbeek, J., Schneider, C. R., Dryhurst, S., Kerr, J., Freeman, A. L. J., Recchia, G., et al. (2020). Susceptibility to misinformation about COVID-19 around the world. Royal Soc. Open Sci. 7:201199. doi: 10.1098/rsos.201199
Ross, R. M., Rand, D. G., and Pennycook, G. (2021). Beyond “fake news”: analytic thinking and the detection of false and hyperpartisan news headlines. Judgm. Deci. Making 16, 484–504. doi: 10.1017/S1930297500008640
Shin, J., Jian, L., Driscoll, K., and Bar, F. (2018). The diffusion of misinformation on social media: Temporal pattern, message, and source. Comput. Human Behav. 83, 278–287. doi: 10.1016/j.chb.2018.02.008
Sindermann, C., Cooper, A., and Montag, C. (2020). A short review on susceptibility to falling for fake political news. Curr. Opin. Psychol. 36, 44–48. doi: 10.1016/j.copsyc.2020.03.014
Smith, M., and Alvarez, F. (2021). Identifying mortality factors from Machine Learning using Shapley values – a case of COVID19. Expert Syst. Appl. 176:114832. doi: 10.1016/j.eswa.2021.114832
Sternisko, A., Cichocka, A., Cislak, A., and Van Bavel, J. J. (2021). “National narcissism predicts the belief in and the dissemination of conspiracy theories during the COVID-19 pandemic: evidence from 56 countries,” in Personality and Social Psychology Bulletin (Thousand Oaks: SAGE Publications Inc.).
Stietz, J., Jauk, E., Krach, S., and Kanske, P. (2019). Dissociating empathy from perspective-taking: evidence from intra- and inter-individual differences research. Front. Psychiatry 10:126. doi: 10.3389/fpsyt.2019.00126
Sun, N., Rau, P. P.-L., and Ma, L. (2014). Understanding lurkers in online communities: a literature review. Comput. Human Behav. 38, 110–117. doi: 10.1016/j.chb.2014.05.022
Swami, V., Tran, U. S., Stieger, S., Pietschnig, J., Nader, I., and Voracek, M. (2016). Who believes in the giant skeleton myth? An examination of individual difference correlates. SAGE Open 6:2158244015623592. doi: 10.1177/2158244015623592
Syrdal, H. A., and Briggs, E. (2018). Engagement with social media content: a qualitative exploration. J. Market. Theory Pract. 26, 4–22. doi: 10.1080/10696679.2017.1389243
Tandoc, E. C., Lim, Z. W., and Ling, R. (2018). Defining “Fake News”. Digit. Journal. 6, 137–153. doi: 10.1080/21670811.2017.1360143
Tully, M., Bode, L., and Vraga, E. K. (2020). mobilizing users: does exposure to misinformation and its correction affect users' responses to a health misinformation post? Soc. Media + Society 6:2056305120978377. doi: 10.1177/2056305120978377
Vaal, S., Schofield, M. B., Baker, I. S., and Roberts, B. L. H. (2022). Narcissism, national narcissism, COVID-19 conspiracy belief, and social media use as predictors of compliance with COVID-19 public health guidelines. Curr. Psychol. 5:1–8. doi: 10.1007/s12144-022-03715-6
van der Linden, S. (2022). Misinformation: susceptibility, spread, and interventions to immunize the public. Nat. Med. 28, 460–467. doi: 10.1038/s41591-022-01713-6
Van Mieghem, P., Blenn, N., and Doerr, C. (2011). Lognormal distribution in the digg online social network. Eur. Phys. J. B 83:251. doi: 10.1140/epjb/e2011-20124-0
Vegetti, F., and Mancosu, M. (2020). The impact of political sophistication and motivated reasoning on misinformation. Polit. Commun. 37, 678–695. doi: 10.1080/10584609.2020.1744778
Verduyn, P., Lee, D. S., Park, J., Shablack, H., Orvell, A., Bayer, J., et al. (2015). Passive Facebook usage undermines affective well-being: experimental and longitudinal evidence. J.f Exp. Psychol.: General 144:480. doi: 10.1037/xge0000057
Verduyn, P., Ybarra, O., Résibois, M., Jonides, J., and Kross, E. (2017). Do social network sites enhance or undermine subjective well-being? A critical review. Soc. Issues Policy Rev. 11, 274–302. doi: 10.1111/sipr.12033
Vosoughi, S., Roy, D., and Aral, S. (2018). The spread of true and false news online. Science 359, 1146–1151. doi: 10.1126/science.aap9559
Vraga, E. K., and Bode, L. (2020). Correction as a solution for health misinformation on social media. Am. J. Public Health 110, S278–S280. doi: 10.2105/AJPH.2020.305916
Wang, J., and Sundar, S. S. (2022). Liking versus commenting on online news: effects of expression affordances on political attitudes. J. Comp.-Mediat. Commun. 27:zmac018. doi: 10.1093/jcmc/zmac018
Wang, Y., McKee, M., Torbica, A., and Stuckler, D. (2019). Systematic literature review on the spread of health-related misinformation on social media. Soc. Sc. Med. 240:112552. doi: 10.1016/j.socscimed.2019.112552
Waszak, P. M., Kasprzycka-Waszak, W., and Kubanek, A. (2018). The spread of medical fake news in social media – The pilot quantitative study. Health Policy Technol. 7, 115–118. doi: 10.1016/j.hlpt.2018.03.002
Yu, R. P. (2016). The relationship between passive and active non-political social media use and political expression on Facebook and Twitter. Comput. Human Behav. 58, 413–420. doi: 10.1016/j.chb.2016.01.019
Zarocostas, J. (2020). How to fight an infodemic. Lancet 395:676. doi: 10.1016/S0140-6736(20)30461-X
Keywords: misinformation, narcissism, personality traits, motivated reasoning, religiosity, cognitive reflective thinking, social media simulation, correcting misinformation
Citation: Haupt MR, Cuomo R, Mackey TK and Coulson S (2024) The role of narcissism and motivated reasoning on misinformation propagation. Front. Commun. 9:1472631. doi: 10.3389/fcomm.2024.1472631
Received: 29 July 2024; Accepted: 16 September 2024;
Published: 03 October 2024.
Edited by:
Ashok Kumbamu, Mayo Clinic, United StatesReviewed by:
Gabriela Zago, Federal University of Pelotas, BrazilRita Gill Singh, Hong Kong Baptist University, Hong Kong SAR, China
Copyright © 2024 Haupt, Cuomo, Mackey and Coulson. This is an open-access article distributed under the terms of the Creative Commons Attribution License (CC BY). The use, distribution or reproduction in other forums is permitted, provided the original author(s) and the copyright owner(s) are credited and that the original publication in this journal is cited, in accordance with accepted academic practice. No use, distribution or reproduction is permitted which does not comply with these terms.
*Correspondence: Michael Robert Haupt, bWhhdXB0JiN4MDAwNDA7dWNzZC5lZHU=