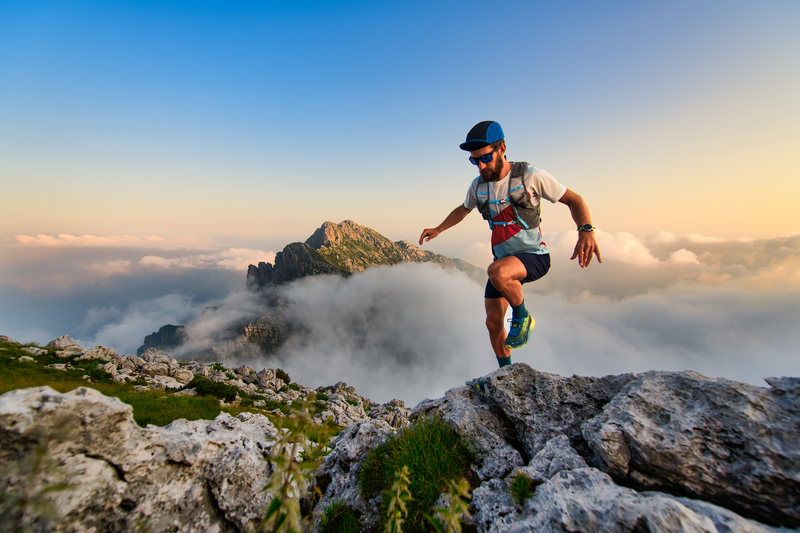
95% of researchers rate our articles as excellent or good
Learn more about the work of our research integrity team to safeguard the quality of each article we publish.
Find out more
ORIGINAL RESEARCH article
Front. Commun. , 17 September 2024
Sec. Advertising and Marketing Communication
Volume 9 - 2024 | https://doi.org/10.3389/fcomm.2024.1460321
Purpose: The research focuses on the crucial role of online reviews in shaping consumer trust in e-commerce platforms, examining the impact of perceived authentic and fake reviews on purchasing decisions and platform reputation. It assesses how consumers perceive review authenticity and quality and their effects on trust levels in reviews, marketplaces, and reputation systems. It also explores the relationship between trust forms and overall experiences.
Design/methodology: A quantitative approach is employed, utilizing a questionnaire distributed to recent Mercado Libre buyers. To test hypotheses, data from 326 valid responses are analyzed using confirmatory factor analysis and Partial Least Squares Structural Equation Modeling (PLS-SEM).
Findings: Findings reveal that fake review perception negatively affects trust in rating systems, while high-quality reviews positively influence all trust forms. Customer experience is directly impacted by trust in marketplaces and rating systems, indicating a mediation effect of trust in the rating system on the relationship between fake review perception and customer experience.
Research limitations/implications: Limitations include using a convenience sample and focusing on trust in the rating system rather than reviews or the marketplace, suggesting avenues for future research. Practical implications include recommendations to ensure review quality, enhance rating system controls, and promote review usage in the purchase process.
Originality: The study addresses a timely and relevant gap in understanding the impact of reviews on e-commerce trust, particularly within the context of Latin America and Mercado Libre’s dominance in the region’s e-commerce landscape.
Online reviews are a form of digital Word-of-mouth (Senthilkumar and RubanRaja, 2021). After an online purchase, consumers can evaluate the reliability and quality of a seller who offers their products through a Marketplace (Curchod et al., 2020). As a result, online reviews have become an essential part of the consumer purchasing decision process (Schoenmueller et al., 2020). A study by Brightlocal showed that an average consumer could read a maximum of 10 online reviews before making a purchase decision (Alzate et al., 2021). Therefore, it is not surprising that reviews can considerably influence the sale of products (Dai et al., 2020).
Given the positive influence that reviews generate on sales conversion (Senthilkumar and RubanRaja, 2021) and that the use of reviews makes companies that include them look much more trustworthy (Alzate et al., 2021), companies have encouraged their customers to write more reviews. In this way, some platforms even recognize users who do the most product reviews. For example, Yelp has its “Elite” status, Tripadvisor has its “Contributor Level,” Google has its “Badges” program, and Amazon has its Amazon Vine program. Consequently, consumers have increased their participation by giving reviews of the products they buy online (Nguyen et al., 2021).
Its popularity lies in the fact that reviews have become a rich source of information containing consumer preferences, what matters to them, and their feelings about products. That is why reviews work for other consumers who plan to purchase a product (Zhao et al., 2021) because they work as a trust transfer mechanism (Pavlou and Gefen, 2004) since it allows buyers to trust sellers based on the information they receive from other customers. This ultimately builds trust in the community of sellers who trade in a Marketplace. Also, based on the overall reputation of a marketplace, consumers should be able to form their judgments about the nature of the community of sellers that trade on it. In this way, an effective feedback mechanism that, for this work, we will call reviews, acts as an informal certifier that drives the purchase of the products offered on the e-commerce platform (Pavlou and Gefen, 2004).
Consequently, the consumer’s trust in e-commerce platforms is decisive in future purchases and the recommendations a buyer can give to another. For this reason, although reviews can be beneficial when guiding consumers in their purchasing processes, there are fake reviews that can confuse potential buyers and undermine the credibility of e-commerce platforms. In this context, a fraudulent review can significantly undermine the integrity of the entire online sales ecosystem. Initially, it may prompt consumers to make ill-informed purchasing decisions, resulting in the acquisition of substandard products that fail to meet their expectations. However, more importantly, such deceptive practices can erode trust in online reviews (Mayzlin et al., 2014), ultimately precipitating a widespread loss of credibility. Consequently, users may become overly hesitant to rely on the online platform again (Dery et al., 2021), potentially leading to enduring reputational damage.
The adverse effects of creating fake reviews, or the customer perception of fakeness in reviews, have led to an increasing trend in research articles published on this topic in recent years (Sahut et al., 2021). However, most of the different investigations that address the subject in question are from countries with a high penetration of electronic commerce, that is, in stable and mature economies, to which relevant e-commerce platforms such as Amazon and Alibaba belong, which have already been case studies. However, given that Latin America’s macroeconomic, geopolitical, and cultural context is far from the context in which developed countries live, these studies may not entirely fit our reality.
It is precisely for this reason that this research is timely and relevant since the analysis of the reviewed phenomenon and its impact on trust toward e-commerce platforms is still limited for studies applied to companies in Latin America. An example is Mercado Libre, the company with the highest monthly traffic since it receives 667 million visits per month, four times more than Amazon, the second most consulted marketplace, and reaches 169 million visits per month in Latin America. Likewise, it has 25.4% of the Growth Merchandise Volume (GMV) of retail sales through e-commerce in Latin America (Statista, 2023). Therefore, this study aims to identify how the perception of whether a review is false or has high information quality impacts different types of consumer trust in Mercado Libre Colombia and their customer experience.
Recent research have notably focused on innovative technologies such as machine learning and neural networks for detecting fake reviews (Niranjani et al., 2022; Deshai and Rao, 2023), and automating review evaluation processes (Goyal and Deora, 2022; Dhabliya et al., 2023). Other studies have explored the impact of fake reviews on website visibility (Tufail et al., 2022) and companies’ responses to reviews on their platforms, including review manipulation (Jin Ma and Lee, 2014). However, to the best of our knowledge, there is a shortage of research contrasting perceptions of quality and fakeness across the three types of trust under examination in this paper.
Our study provides valuable insights into how perceptions of review quality influence trust dynamics within e-commerce platforms. Specifically, our findings underscore that higher quality information in reviews significantly enhances trust across multiple dimensions This relationship is pivotal as it elucidates how consumers’ trust in e-commerce ecosystems can be bolstered by the quality of information available through reviews. These findings are crucial for e-commerce platforms seeking to enhance consumer confidence and foster more robust interactions between buyers and sellers.
Furthermore, this research contributes significantly to consumer behavior literature and trust theory by elucidating the mechanism through which trust is built and sustained in online marketplaces. It also sheds light on fake reviews, offering valuable insights into their implications. By examining the perceptions of quality and fakeness within the context of trust, this study adds a nuanced understanding to the existing body of literature.
The study begins with a comprehensive literature review on variables selected to explore the customers perceptions of reviews’ fakeness and quality, trust in reviews, reputation system, marketplace credibility, and customer experience (CX). Subsequently, the research methodology is presented in materials and methods, a quantitative cross-sectional investigation is detailed to generating conclusive results that are rigorously analyzed. Finally, the document discusses the results obtained, draws conclusions, and outlines research implications and limitations.
Since this study focuses on the impact of customer perception of reviews on different types of trust and its influence on the consumer experience in a marketplace, it is essential to first understand the broader context of online shopping behavior. Several studies have shown that consumers’ attitudes toward online shopping are shaped by a combination of factors (Wang et al., 2013; Lim et al., 2016; Peña-García et al., 2020). Key drivers include ease of use, usefulness (Davis, 1989), enjoyment (Cai and Xu, 2006), commitment, perceived risk and trust (Gefen et al., 2003), among others, which are often influenced by the design and functionality of the platform itself, as well as personal traits.
However, these intrinsic factors are complemented by exogenous variables such as product features, previous online shopping experience, and trust. Research by Gefen et al. (2003) demonstrates that trust, particularly in unfamiliar environments, plays a critical role in shaping consumer behavior. Similarly, Pavlou and Gefen (2004) found that consumers’ trust in an e-commerce platform significantly influences their willingness to engage in online transactions. Trust acts as a mediating factor, reducing perceived risk and increasing the likelihood of purchase.
Given this context, our study explicitly focuses on trust as a foundational element among several factors influencing online shopping behavior. This is particularly relevant in emerging markets such as Colombia, where online shopping is rapidly developing and expanding. Colombian consumers, while generally lacking extensive online shopping experience, represent a significant market due to their openness to adoption. This is driven by the perception that online shopping simplifies and enhances their lives, a sentiment that aligns with the indulgent cultural tendencies prevalent in the region (Peña-García et al., 2020).
The literature review begins by exploring the types of trust selected because it is the cornerstone of consumer decision-making in online environments. Establishing a clear understanding of the different types of trust is crucial, as it sets the stage for examining how perceptions of review fakeness and information quality influence this trust. This approach follows the recommendations of Bryanov and Vziatysheva (2021), who emphasize the importance of expanding studies on susceptibility to information across various digital platforms. After establishing this foundation, the review then addresses perceived fakeness and information quality, and finally, it discusses how these factors interact to shape the customer experience (CX).
Considering the literature, it has been established that the advisor’s level of trust is vital in reducing consumer uncertainty, especially in online environments (Sniezek and Van Swol, 2001; Alzate et al., 2021). Trust is the strength of a person’s belief that a statement, opinion, or decision is the best possible (Alzate et al., 2021). According to the Judge-Advisor System paradigm (Sniezek and Van Swol, 2001), high levels of trust in the advisor can signify experience, influencing the judge to accept the advice. This study will focus on three types of trust: trust in reviews, the marketplace, and the rating systems. To guide this investigation, the study will adopt the institutional-based trust model, which posits that consumers trust the assurances and endorsements provided by third parties (Zucker, 1986; Shapiro, 1987; Pavlou and Gefen, 2004).
Trust is critical in consumer decision-making and loyalty, influencing how customers form strong relationships with brands (Cardoso et al., 2022). In the context of online reviews, trust represents consumers’ confidence in the reliability and integrity of the reviews posted by other customers. This trust in reviews is essential because it helps mitigate the risk of purchasing products or services online without physical inspection.
According to Pavlou and Dimoka (2006), significant information asymmetry exists between consumers and online platform vendors. This asymmetry arises from the inability of consumers to physically inspect a product or service before purchase, which compels them to rely on electronic reporting mechanisms to mitigate the associated risks. Moreover, the information accessible to consumers is often limited to what the brand is willing to share, so independent reviews not sponsored or influenced by the brand are essential for informed decision-making. External opinions provide an unbiased perspective that is vital for consumers. Additionally, feedback mechanisms, such as positive or negative ratings, have played a crucial role in inducing trust in online sellers by mitigating information asymmetry (Zhang et al., 2014).
Marketplaces have gained interest from academy and enterprises. Considerable research has been conducted on electronic marketplaces over the past years. This topic has been a prominent feature of the information systems research landscape during this period, reflecting the rapid growth and subsequent consolidation of electronic marketplace (e-marketplace) numbers (Shankar et al., 2022). Today, e-marketplaces play a significant role in business and continue to be a vibrant research topic. According to Cano et al. (2022), a marketplace is a physical or digital platform where vendors and customers engage in buying and selling activities, often serving as social and economic hubs.
E-Marketplaces have contributed significantly to developing countries’ economies by connecting sellers with customers who otherwise might not have been able to find each other (Viswanathan et al., 2021). Marketplaces provide sellers with a powerful and secure platform to offer their products, allowing customers to find, compare easily, and purchase products from their computers (Standing et al., 2010). This accessibility and efficiency have fostered economic growth by expanding market reach and facilitating smoother transactions, benefiting businesses and consumers.
From the perspective of consumer trust, studies such as those conducted by Pavlou and Gefen (2004) have determined that marketplaces play an essential role in mitigating transaction risks, fostering a trustworthy environment, and encouraging online transactions. According to these authors, this phenomenon is explained by the theory of institutional-based trust (Zucker, 1986; Shapiro, 1987), which is particularly relevant in online markets where buyers often engage in transactions with new and unfamiliar sellers, relying on third-party protections that establish an institutional framework.
Hence, this research posits trust in the marketplace as a critical variable for investigation, designating it as a dependent variable, given its foundational role in electronic commerce (Keen et al., 1999). Consequently, it is pertinent for this study to ascertain whether consumers of Mercado Libre perceive the platform’s review mechanisms as reliable and beneficial.
Rating systems, such as star ratings, thumbs up, or user reviews, serve as proxies for product reliability and customer satisfaction (Zhang et al., 2014). Following Beck et al. (2023), consumer trust in the rating system refers to the belief that the platform will reliably fulfill its commitments, offer precise information, and act in ways that cater to the consumer’s needs and long-term benefits.
Several factors influence trust in rating systems. First, the perceived credibility of the rating source is crucial. Studies such as Filieri (2015) have shown that verified purchasers’ ratings are more trusted than anonymous or unverified sources. Second, the volume and diversity of ratings contribute to perceived reliability. Products with a higher number of ratings and a balanced distribution across different rating levels are typically viewed as more trustworthy (Chevalier and Mayzlin, 2006). Third, the system design, including the algorithms used to aggregate and display ratings, is vital. Transparency in how ratings are calculated and presented can significantly affect user confidence. Luca and Zervas (2016) highlighted that user trust increases when platforms disclose their rating algorithms and moderation policies. Ensuring that fraudulent activities or biased algorithms do not influence ratings is essential for maintaining credibility.
Research by Nguyen et al. (2021) investigates how rating scale mechanisms should be implemented on online platforms, considering factors such as the maturity of online shopping habits among buyers, and whether they are experts or novices. This highlights the importance of understanding how different consumer experiences and expertise levels affect the use and perception of rating systems in online reviews. Furthermore, studies have explored the veracity of reviews, identifying inconsistencies between a person’s feelings towards an online purchase and the rating they assign when evaluating the product (Hazim et al., 2018). One significant finding is that rating deviations can indicate potential falseness in reviews (Hajek et al., 2020).
In e-commerce, rating systems classify and present products under a rating scheme, giving them a particular position following business rules. Ratings are easily visible and understandable, often designed with color changes or numerical scales (Anderson and Magruder, 2012). These systems reduce the cognitive effort required from consumers, allowing them to quickly assess whether a product or service is good or bad (Nakayama and Wan, 2017).
Rating systems are essential because they are tied to the trust they generate in online products. Fogel and Zachariah (2017) found that trust in products increases with positive ratings, and consumers are more likely to recommend these products. From a psychological perspective, ratings grant a reputation, essentially serving as a sign of social validation and improving trust (Liu and Park, 2015).
This variable directly impacts purchasing decisions. Products with positive ratings are more likely to be purchased, while those with negative ratings discourage consumers from buying them. Negative ratings significantly influence purchase decisions, limiting product acquisition (Chen et al., 2019). Reviews with higher ratings demonstrate a correlation between positive ratings and higher sales conversion, although this can also lead to fake reviews (Curchod et al., 2020).
Online reviews are the product of commercial exchanges where consumers share their experiences with products or services on digital platforms. These reviews play a crucial role in shaping consumer perceptions and decision-making processes. To provide a comprehensive understanding of online reviews, it is essential, to begin with the definition provided by Cho et al. (2022), who describe ratings as a form of quantitative review where an “ex-post quality” rating is given based on the quality experienced after the use or consumption of a product. In other words, online reviews are based on the consumer’s direct experience with the product.
Literature has demonstrated that online reviews can also be fake, either by individuals who have not used the product or by users sponsored by the brand to provide positive reviews. Additionally, some users act on behalf of competitors and post negative comments to damage the product’s reputation. This phenomenon raises significant concerns about the perception of fakeness and the quality of information provided in online reviews.
Fake reviews are used on online platforms to confuse users’ decisions in their purchase process (Hazim et al., 2018). Its importance is due to the relevance that reviews have been gaining in the purchase decision process and their high impact on sales conversion. This power of influence leads to 84% of buyers believing the reviews they read in online publications (Senthilkumar and RubanRaja, 2021). It has generated a rapid and unethical push in ill-intentioned groups to change the ranking of products (Liu et al., 2016) through fake reviews.
Its impact falls on negative aspects for internet sales since there is a loss of reputation, which can be associated with a loss of income since users may be too cautious before trusting the platform again (Dery et al., 2021). Recent studies have found that out of every three reviews on TripAdvisor, one is false. For this reason, misleading reviews have become one of the biggest concerns in the industry (Hajek et al., 2020). Therefore, this research proposes to study consumers’ perceptions of the veracity of reviews as a critical variable in building trust in reputation systems and marketplaces. Its study is critical because detecting fake reviews and understanding their antecedents and consequences would help penalize their perpetrators and restrict the prevalence of this type of review (Wu et al., 2020).
For this research, fakeness perception is understood as clients’ perception of inauthentic reviews used on online platforms, written by consumers or any other type of player, such as customers, online sellers, and review platforms (Wu et al., 2020; Andrade Cruz et al., 2021). In this way, fake reviews are inconsistent with genuine evaluations of products or services. Therefore they represent deceptive practices on the Internet that are used to give the impression to potential consumers that other ordinary users and customers are recommending a particular product when in fact, the recommendation is being made by the seller or by someone on the seller’s behalf (Malbon, 2013), or made by individuals motivated by some reward derived from three sources: annoying consumers, self-proclaimed brand managers and social status (Anderson and Simester, 2014; Thakur, 2018; Wu et al., 2020). Thus, the perception of false reviews will be understood as those that consumers recognize as shameless or malicious.
Fakeness perception negatively impacts the three types of trust being studied. The perception of fakeness directly compromises trust in reviews. If consumers believe that reviews are inauthentic or manipulated, they will doubt the validity of the information provided. This skepticism can also extend to genuine reviews, making it difficult for consumers to distinguish between honest and deceptive feedback. The overall credibility of the review platform is thus undermined (Filieri, 2015).
On the other hand, when consumers detect fake reviews, their trust in the vendor diminishes. They may perceive the marketplace as dishonest and manipulative, leading to a loss of confidence in the brand’s reliability and integrity. This erosion of trust can result in decreased customer loyalty and negative word-of-mouth, ultimately harming the marketplace’s reputation (Cardoso et al., 2022). Finally, Perceived fakeness in reviews also affects trust in the rating systems that aggregate these reviews. Consumers rely on these systems to provide an accurate and unbiased product quality and customer satisfaction summary. When fake reviews infiltrate these systems, ratings’ reliability is questioned. This can lead to a general mistrust of the rating mechanism, reducing its effectiveness as a decision-making tool (Zhang et al., 2014).
Thus, we present the first hypothesis of this research:
H1a: Fakeness perception about reviews negatively and directly impacts trust in reviews.
H1b: Fakeness perception about reviews negatively and directly impacts trust in the marketplace.
H1c: Fakeness perception about reviews negatively and directly impacts trust in the rating system.
The quality of the information is a subjective construct and difficult to define. High-quality information can be defined as accurate, reliable, current, concise, easy to understand, and organized (Alkhattabi et al., 2011; Arazy and Kopak, 2011; Yaari et al., 2011). Since the general purpose is to inform, the information quality goal is defined as “appropriately informative” (Huang et al., 2015). That being said, information quality refers to how valuable, relevant, precise, helpful, credible, understandable, and timely the information provided to the consumer is (Berry and Parasuraman, 1997).
This variable has been present in different investigations as a determining variable when positively persuading or convincing consumers in their purchase intention (Zhang et al., 2014). Aligned with this position, Chen et al. (2019) and Aditya and Alversia (2019) found that the quality of the review positively influenced consumer decision-making (Aditya and Alversia, 2019).
Through the Information Adoption Model, it was verified that the information quality variable works as a useful predictor in different contexts, such as the adoption of social media (Chuang et al., 2015), eWOM (Filieri, 2015; Hussain et al., 2017) and even in online reviews (Filieri et al., 2018). It has also been determined that the stronger the relationship between consumers and electronic platforms, the higher the credibility and quality perception of reviews (He and Zhu, 2020). Furthermore, the quality of the information depends on the utility it generates for consumers (Yeap et al., 2014). Therefore, this variable plays a key role and helps them evaluate the quality of a product or service (Lata and Rana, 2021).
Among the factors determining the quality of the information in reviews, Cheung et al. (2008) identified four dimensions: relevance, accuracy, timeliness, and comprehensiveness. Furthermore, some studies have shown that when reviews are of quality, they are linguistically constructed differently than when they are true or false. Furthermore, in terms of quality, they are expected to be written understandably. Finally, it is said that the reviews with more quality in the specificity of what is said tend to be truthful (Banerjee et al., 2017).
With the increasing adoption of online shopping, believing in the information provided plays a significant role due to the uncertainty that online shopping can have. Information quality helps buyers to resolve these information asymmetries (Anderson and Magruder, 2012).
Online platforms are prone to abuse and manipulation by certain actors related to the purchase process. For example, websites are manipulated in their reviews by posting sentiments in fake reviews. The consequences of such manipulations are the deterioration of the quality of the information and the loss of consumer welfare (Lee et al., 2018). However, the quality of the information is essential to understanding the implications of reviews on consumers’ purchasing behavior, mainly because online shoppers tend to believe more and pay more attention to negative reviews (Short, 2012).
In considering the quality of information, two fundamental perspectives emerged: truth and trust. Truth refers to the belief that online content has to be authentic, while trust is the condition of being considered honest. These two definitions are crucial in the context of this variable because they are closely linked to the consumer’s purchasing choice (Gössling et al., 2018). Likewise, quality is determined by a series of dimensions, such as the degree of elaboration (duration) and the preference of the review judged by the number of readers (number of “Like” votes that the review receives) (Nguyen et al., 2021).
In exploring the intricacies of online reviews, it becomes evident that trust, as a facet of consumer decision-making, can also be understood as a reflection of perceived truth. This concept is supported by empirical findings in the literature, which highlight the intertwined nature of trust and truth in consumer behavior (Mayer et al., 1995; Doney and Cannon, 1997). Trust, akin to truth, represents the conviction in the authenticity and reliability of information presented. Scholars have emphasized the significance of trust in reducing consumer uncertainty, particularly within the context of online environments (Cui et al., 2020; Alam et al., 2021; Goyal and Deora, 2022). Hence, the alignment between trust and truth underscores their pivotal roles in shaping consumers’ perceptions and choices.
According to the characteristics of high-quality perception given by Alkhattabi et al. (2011), Arazy and Kopak (2011), and Yaari et al. (2011), the concept is directly related to enhancing the three types of trust under study. Firstly, regarding trust in reviews, high-quality information ensures that consumer reviews are perceived as genuine, unbiased, and helpful. When reviews provide accurate and valuable insights, consumers are more likely to trust in reviews (Hussain et al., 2017). Secondly, high-quality information enhances trust in marketplaces by ensuring that consumers’ information about a brand’s product or services is accurate, reliable, and credible (Berry and Parasuraman, 1997). Lastly, high-quality information also supports trust in rating systems by contributing to aggregated ratings’ perceived fairness and reliability. Transparent and accurate rating mechanisms based on high-quality information reduce skepticism about the rating system’s credibility and encourage trust overall in the marketplace’s products or services (Zhang et al., 2014).
In light of the literature, we propose the second research hypothesis.
H2a: High-quality perception about reviews positively and directly impacts trust in reviews.
H2b: A high-quality perception of reviews positively and directly impacts trust in the marketplace.
H2c: A high-quality perception of reviews positively and directly impacts trust in the rating system.
Customer experience (CX) is a dynamic process involving continuous evaluation of consumer perceptions across interactions before, during, and after purchases (Siqueira et al., 2019). It encompasses a consumer’s journey with a brand or product, including product information, payment methods, delivery experiences, service quality, perceived risk, privacy concerns, security, and overall satisfaction (Ariyono et al., 2022).
In academic and business contexts, CX is increasingly recognized as a critical determinant of consumer behavior and organizational success (Becker and Jaakkola, 2020). Positive CX fosters customer loyalty, enhances brand reputation, and increases the likelihood of repeat purchases and positive word-of-mouth referrals (Barari et al., 2020). Research underscores the multidimensional nature of CX, emphasizing its role in shaping consumer perceptions and behaviors beyond individual transactions (Senthilkumar and RubanRaja, 2021). Effective management of CX involves understanding and meeting consumer expectations across various touchpoints, thereby influencing overall satisfaction and loyalty (Berry et al., 2002).
From a theoretical standpoint, CX integrates psychology, sociology, and marketing insights to explain how consumers perceive and evaluate their interactions with brands (Berry et al., 2002). It is influenced by factors such as service quality, emotional engagement, ease of interaction, and the perceived value derived from the overall experience (Ariyono et al., 2022). In practical terms, organizations prioritize CX by improving service delivery, personalizing interactions, and leveraging digital technologies to enhance consumer engagement and satisfaction (Becker and Jaakkola, 2020).
Trusting reviews, marketplaces, and rating systems significantly alleviate pressure across the customer journey. CX becomes pivotal as it vividly showcases the specific attributes of products and services purchased online, often articulated through detailed reviews (Lata and Rana, 2021). As online reviews continue to gain prominence, businesses increasingly prioritize CX enhancements to meet consumer expectations effectively.
Studies highlight that a substantial majority, around 80%, of consumers trust recommendations from fellow buyers with prior product experience (Hajek et al., 2020). This trust influences purchasing decisions and diminishes uncertainty for new buyers by providing valuable insights into product performance and quality (Liu and Park, 2015). Furthermore, peer experiences shared through reviews often hold more sway than traditional advertising efforts by sellers (Short, 2012). Consumers perceive reviews from fellow users as more authentic and relatable, enhancing the trustworthiness and credibility of the information conveyed.
In sectors like hospitality, online ratings prove to be more reliable predictors of a positive CX compared to conventional satisfaction metrics (Kim and Park, 2017). This reliability stems from the collective wisdom embedded in numerous consumer reviews, offering a more precise depiction of what to expect and easing purchasing decisions’ uncertainties. When consumers trust reviews, marketplaces, and rating systems, they approach the customer journey with reduced apprehension.
Therefore, this study posits consumer experience as a dependent variable and aims to elucidate its relationship with trust in reviews, marketplaces, and reputation systems. Thus, it introduces the final hypotheses.
H3a: Trust in reviews has a positive and direct impact on CX.
H3b: Trust in the marketplace has a positive and direct impact on CX.
H3c: Trust in the reputation system has a positive and direct impact on CX.
Based on the literature review, the research model that summarizes all the proposed hypotheses is proposed, as detailed in the following figure:
The sample comprises online buyers who have purchased on the Mercado Libre website or app in the last 6 months. Data was collected through an online self-administered survey using a structured questionnaire. A pre-test was conducted with 20 Mercado Libre customers to assess the items’ clarity and understanding. Based on the feedback, adjustments were made to improve the readability of the survey questions. Additionally, we included product categories that had not been initially considered for descriptive study. No substantial changes were made to the measurement instrument.
The survey link was distributed via emails, social networks, and WhatsApp groups to a convenience sample. Participants were also encouraged to share the survey with family and friends to broaden the reach and allow for a more varied sample, which would better reflect consumer behavior. As a result, 1,173 questionnaires were delivered during November 2022, of which 528 were answered. After purifying the database, 326 complete and valid questionnaires were obtained.
The sample is mainly composed of men (65.44%). Most of the participants are employed (67.28%), are married or in a free union (58.72%) and are between the ages of 25 and 34 (42.51%). Regarding the graphic web features, 98.78% of people have been using the Internet for over 6 years, and most spend more than 15 h a week browsing it (43.73%). Finally, the majority of those surveyed bought through Mercado Libre since before 2019 (65.75%), they have a purchase frequency of once every 3 months (42.51%), with an average ticket between 70,000 and 140,000 pesos (55.96%) and the most demanded category through this platform corresponds to electronic products (22.63%). Table 1 presents categories of products bought in Mercado Libre, seniority, purchase frequency, and average ticket.
Scales previously validated in the literature were used to construct the information collection instrument. Thus, it is proposed to measure the perception of the falsity of the reviews from the work of Wu et al. (2019). The research by Oliveira et al. (2020) was used to measure the high-quality perception variable. The scale to measure trust in reviews was adapted from the study by Zhang et al. (2014), while the scales to measure trust in the Marketplaces and rating systems were based on the study by Pavlou and Gefen (2004). Finally, the studies by Kim and Choi (2013) and Siqueira et al. (2019) were adapted to measure CX.
The adapted scales are presented in the Table 2.
A confirmatory factor analysis CFA was performed in the SmartPLS 3.0 software through a PLS algorithm analysis to analyze the reliability and validity of the measurement instrument (Table 3).
To improve the goodness of fit, the items FKRV1, FKRV2, FKRV3, and TRSREV2, whose beta was <0.708, were eliminated, following Hair et al. (2019). The information obtained by the PLS algorithm indicates that the information collection instrument is reliable and valid since Cronbach’s alpha of all its factors is above 0.5 and below 0.9. Furthermore, composite reliability obtained values above 0.6, and the AVE of all factors was above 0.5, following the recommended values.
The discriminant validity is determined by the Fornell-Larcker criterion and the Heterotrait-Monotrait Ratio (HTMT). The Fornell-Larcker criterion compares the shared variance between pairs of constructs with the variance extracted for each construct. At the same time, the HTMT assesses the correlation between constructs to ensure they are sufficiently distinct from one another (Ab Hamid et al., 2017) (Table 4).
Discriminant validity is confirmed, as the correlation within the factors is less than the square root of the AVE for each factor (as per the Fornell-Larcker criterion), and all HTMT values are below the commonly accepted threshold, further supporting the distinctiveness of the constructs (Risher and Hair, 2017).
To assess potential multicollinearity issues, we calculated the Variance Inflation Factor (VIF) for each construct coefficient’s increase due to collinearity. According to Hair (2011), VIF values below 5 indicate that multicollinearity is not a significant concern, ensuring the independence of the constructs in the model.
As shown in Table 5, all VIF values are below the threshold of 5, ranging from 1.463 to 2.937. These results indicate that multicollinearity is not a concern in our model, confirming that the constructs are sufficiently independent.
To contrast the hypotheses proposed by this investigation, a PLS-SEM analysis was carried out through a Bootstrapping analysis in Smart PLS 3.0, where the model was tested (Table 6).
According to the PLS-SEM analysis, hypotheses H1a and H3a are rejected, while the other hypotheses are significantly contrasted with p < 0.005, indicating a high relationship between the variables. The results are discussed in the next section.
The analysis suggests that while a trend indicates that higher perceptions of fakeness in reviews might reduce trust in those reviews, this effect is not statistically significant (𝛽 = −0.081, 𝑡 = 1.729, 𝑝 = 0.084). This finding aligns with some literature, which suggests that consumers may not significantly alter their trust based solely on the perceived authenticity of individual reviews. For instance, studies have shown that even when reviews are perceived as exaggerated or potentially fake, this does not always lead to a substantial decrease in trust. Banerjee (2022) notes that exaggeration in fake reviews is not always distinguishable from authentic reviews, particularly in specific contexts, such as luxury vs. budget hotels, which could explain the minimal impact on trust. Similarly, research by Sebastianelli and Tamimi (2018) supports the idea that other factors, such as the volume of reviews and the overall star rating, may play a more significant role in shaping trust, overshadowing concerns about the authenticity of individual reviews. These findings suggest that while consumers may be somewhat skeptical about the authenticity of individual reviews, they rely on additional cues, such as the number of reviews or verified purchases, to maintain their overall trust in the review content.
Hypotheses H1b and H1c results indicate that the perception of fakeness in reviews significantly undermines trust in the marketplace and the rating system. This suggests that when consumers perceive a high level of fakeness in reviews, their trust in the entire marketplace and its rating system is compromised. This could be because consumers view the marketplace as responsible for ensuring the integrity of the reviews. If they believe the marketplace allows fake reviews to persist, it erodes their confidence in the platform and the systems that aggregate these reviews. This underscores the importance of marketplaces actively managing and ensuring the authenticity of reviews to maintain trust across all levels.
Within the analysis of H2a, H2b, and H2c, we tried to verify the positive influence that the quality of the information has on the trust in the reviews, the marketplace, and the reputation systems. We found that, for every point in the quality of information, there was a 0.586-point increase in trust in reviews, 0.385 in trust in marketplaces, and 0.679 in trust in reputation systems. It was also found that the quality of the information explains the increase in trust in reviews by 0.353, trust in the marketplace by 0.174, and trust in reputation systems by 0.461. This shows that trust in reviews, trust in Marketplaces, and trust in reputation systems increases to the extent that the quality of the information in the reviews also increases.
The most significant finding of our study is that the relationship between information quality and trust in reviews, marketplaces, and ratings is linear and positive. Previous studies have shown that the quality of the information is an essential predictor of the intention to book a hotel online; high-quality reviews make the consumers trust and, therefore, see them as a helpful resource, which helps them plan their trips (El-Said, 2020). Information quality is crucial in online reviews as it builds trust (Arazy and Kopak, 2011; Yaari et al., 2011; Huang et al., 2015).
Likewise, it complements the study by Pavlou and Gefen (2004), which shows that the perceived effectiveness of review mechanisms increases buyers’ confidence in the marketplace. One dimension that makes a review effective is its quality (Nguyen et al., 2021), which, as demonstrated in this study, also positively and directly affects trust in the marketplace. These studies, however, could explain, in a limited way, why this is the case (the relationship between these variables) in Latin American countries, so the present study manages to complement the existing literature on this subject, replicating previous research but applying it to a case of a Colombian Marketplace.
Hypothesis H3a shows a non-significant negative relationship between trust in reviews and CX. This is an unexpected finding, particularly given the established role of trust in shaping consumer behavior. One possible explanation is that while trust in reviews is essential, it may not directly impact the overall CX. Instead, other factors, such as product quality or platform usability, might play a more prominent role in determining CX. This aligns with findings from Filieri (2015), who suggests that while trust in online reviews can influence purchase intentions, it does not always directly translate into overall satisfaction or a positive customer experience. Additionally, McKnight et al. (2002) argue that in highly trusted platforms, consumers may take the credibility of reviews for granted, thus diminishing the direct influence of review trust on their overall experience. These considerations suggest that in a particular context, the impact of trust in reviews on CX may be mediated or overshadowed by other elements of the consumer journey.
Finally, the fact that the H3c has been supported differs from the results found in the studies conducted by Munzel (2015) in his research. They did not confirm that the rating system influenced the buyers’ confidence. However, this study had age and gender as moderating variables. Additionally, it was proposed within the findings that these results should be looked at considering the product categories. In the case of H3b and H3c, it was possible to show the positive influence of trust in the marketplace and trust in reputation systems on the consumer experience. We observed that each point in trust in the marketplace and the reputation systems improved the consumer experience by 0.480 and 0.337, respectively. Likewise, 51.5% of the variability of the consumer experience is explained by trust in the marketplace and trust in reputation systems. The consumer experience improves as trust in the marketplace and reputation systems grow.
This finding is similar to previous postulates in which it has been determined that a positive CX is influenced by customer trust. Likewise, this is a central element in forming long-term relationships (Muharam et al., 2021). Similarly, customer trust can increase significantly when the marketplace is perceived to have a good reputation (Kim and Ahn, 2007) and is aligned with the studies by Pavlou and Gefen (2004) that are based on the theory based on institutions (Zucker, 1986; Shapiro, 1987) to demonstrate the importance of trust towards the marketplace seen as a community since it acts as a guarantor of positive experiences and influences the perceived effectiveness of the transactional behavior of buyers.
In turn, this study could support the hypothesis that confidence in ratings positively influences the experience of the Mercado Libre Colombia buyer (H3c). This reinforces previous findings showing that buyers use ratings as the basis for their trust in a Marketplace. It means that buyers give trust by judging their reputation. Therefore, the buyer’s experience is enhanced by these ratings because the future behavior of a seller can be predicted in a certain way (Kim and Ahn, 2007).
To further understand the relationship between online review perceptions and customer experience (CX), we examined the mediating role of trust. Using SmartPLS, we conducted a mediation analysis that included both direct and indirect paths from the perceptions of fakeness and high quality on reviews to CX, with trust as the mediator. Results are presented in Table 7.
The mediation analysis revealed several interesting findings regarding the role of trust in the relationship between online review perceptions and customer experience (CX). Notably, the mediation effect of trust in the rating system was significant in the relationship between the perception of fake reviews and CX and between perceived review quality and CX. This suggests that when consumers perceive reviews as fake, their trust in the rating system diminishes, negatively impacting their overall experience on the platform. Conversely, high-quality reviews enhance trust in the rating system, leading to a more positive customer experience. These results are consistent with the findings of Filieri (2015), who noted that trust in the system that aggregates reviews plays a critical role in shaping customer satisfaction and subsequent behaviors.
Additionally, trust in the marketplace significantly mediated the relationship between the perception of fake reviews and CX and perceived review quality and CX. This implies that consumer trust in the overall platform, influenced by their perceptions of review authenticity and quality, is crucial for enhancing or undermining their experience. The significant mediation effect in these cases aligns with the argument by McKnight et al. (2002) that trust in the platform is a crucial determinant of user satisfaction and loyalty.
Interestingly, trust in reviews did not significantly mediate the relationship between the perception of fake reviews and CX or between perceived review quality and CX. These non-significant results suggest that while trust in individual reviews is essential, it may not be as influential in directly shaping the overall customer experience as trust in the broader systems of the marketplace or the rating system. This could indicate that consumers are more concerned with the integrity of the platform and its review aggregation systems rather than individual reviews themselves when considering their overall experience.
These findings highlight trust’s complex role in mediating the effects of online review perceptions on customer experience. For e-commerce platforms, this underscores the importance of ensuring the authenticity and quality of reviews and maintaining strong trust in the overall marketplace and rating systems to foster a positive customer experience (Shaheen et al., 2019).
Given the growing trend of the exponential increase in online purchases, the research team of this work set itself the objective of studying the confidence that the Mercado Libre buyer has about the reviews and about the marketplace in Colombia and its rating systems, as well as how this affected in the consumer experience. Therefore, the first step was to understand if it was a tool used and a relevant factor in their CX. For this study, the trust variable was used because the literature indicates that high levels of trust in the assessor can act as a sign of experience and influence the judge to accept the advice as an ideal variable (Alzate et al., 2021).
Empirical research shows that the hypotheses where we tried to relate the variable of false reviews with trust in the reviews and the marketplace were rejected because their significance level was higher than 5%, so there was no statistical evidence to support that it was statistically verifiable. For the same reason, we had to reject the hypothesis that related consumer experience with trust in reviews. Likewise, it was possible to show a high and positive relationship between trust in the reviews, the marketplace, and the reputation systems vs. the quality of the information in the reviews. Therefore, it can be concluded that the quality of information obtained in the reviews is relevant to the trust generated in the consumer.
Lastly, the CX increases to the extent that trust in the marketplaces and their reputation systems also increases. This result shows that the quality of the information in the reviews is a determining factor in using these. Additionally, to promote the consumer experience, it is a priority that marketplaces strengthen the measures taken on their rating systems.
Suppose marketplaces want to increase the experience of buyers and increase their sales. In that case, they must work on improving trust since, according to what was demonstrated in this study, individuals use reviews as a reference during their purchase process and establish a direct relationship with the trust generated in their platforms and their rating systems. Therefore, marketplace managers must emphasize strengthening their reputation systems and creating measures that foster consumer confidence before purchasing. Based on the entire investigative process of this text, we suggest the following three recommendations:
1. Ensure that the information other buyers provide through reviews is high-quality. This means that they must be reliable and valuable.
2. Ensure rating systems have control systems that minimize information asymmetry between the product and reality.
3. Encourage using reviews within the purchase process of articles published on the online sales platform.
This recommendation is also supported by the authors Kim and Ahn (2007), who, finding similar results to ours, consider it essential that marketplaces manage customer perceptions about rating systems, the usefulness, and security of the website, over time through clever advertisements and advertising. They further suggest that marketplaces constantly develop, maintain, and update their website to make it a safe and reliable transaction place.
This work supports previous research on the use and trust in online product reviews. More specifically, it strengthens the literature at the regional level since very few academic papers have previously studied the phenomenon of online reviews in the Latin American context.
The research previously consulted in this paper responds to economies with advanced development and greater adoption of online sales. For this reason, this work contributes to understanding consumer behavior in emerging economies. Additionally, the research team, when carrying out the information gathering with Mercado Libre, showed that there were no robust mechanisms for detecting false reviews and no programs that ensured the quality of the information.
This study used a convenience sample of buyers from Mercado Libre Colombia. While this provided valuable insights into the behaviors of Colombian consumers, a more extensive and more diverse sample across different Latin American countries could offer a broader understanding of the regional market. Future research should consider scaling this study to include countries like Mexico, Chile, Brazil, and Argentina, where e-commerce has grown significantly in recent years. Such expansion would allow for a more comprehensive analysis of the Latin American market and could uncover regional differences in consumer behavior and trust dynamics.
In the current analysis, the perception of fake reviews impacted trust primarily in the rating system, with no significant effects on trust in individual reviews or the marketplace. This finding suggests that future research should investigate the factors influencing trust in these other dimensions. Specifically, investigating whether there is manipulation of reviews by sellers on platforms like Mercado Libre could be a valuable area of study. Understanding whether such practices are used strategically to sway purchase intentions could provide insights into consumer trust and decision-making mechanisms.
The paper touches on the challenges associated with detecting fake reviews and suggests room for further exploration in this area. Future research could benefit from developing and proposing methods for identifying and filtering out fake comments. These methods could then be validated in real-world scenarios to enhance the practical relevance of the findings. Advancing these detection techniques would be important for improving the reliability of online reviews and, consequently, consumer trust.
Lastly, while this study touched on the importance of information quality, it did not delve into the specific dimensions of this construct. Future research should define and measure the various dimensions of information quality—such as relevance, accuracy, timeliness, and comprehensiveness—in detail. By clarifying how each dimension affects trust and consumer behavior, researchers can provide more targeted recommendations for improving the quality of information presented on e-commerce platforms. This, in turn, could lead to more effective strategies for enhancing customer experience and satisfaction. Finally, this study used cross-sectional data, analyzed by regression, which means these results could be based on spurious correlations. For example, the flow of causality in Figure 1 may in fact be in the reverse direction (a positive customer experience bolsters trust and perceptions of online reviews).
The original contributions presented in the study are publicly available. This data can be found here: Mendeley Data, doi: 10.17632/kxwsk6k9wf.1.
The studies involving humans were approved by the CESA–Colegio de Estudios Superiores de Administración Ethics Committee approval. The studies were conducted in accordance with the local legislation and institutional requirements. The participants provided their written informed consent to participate in this study.
NP-G: Conceptualization, Formal analysis, Methodology, Project administration, Supervision, Writing – original draft. ML-O: Conceptualization, Supervision, Writing – original draft. DA: Data curation, Investigation, Resources, Writing – original draft. MC: Data curation, Investigation, Resources, Writing – original draft.
The author(s) declare that no financial support was received for the research, authorship, and/or publication of this article.
The authors declare that the research was conducted in the absence of any commercial or financial relationships that could be construed as a potential conflict of interest.
All claims expressed in this article are solely those of the authors and do not necessarily represent those of their affiliated organizations, or those of the publisher, the editors and the reviewers. Any product that may be evaluated in this article, or claim that may be made by its manufacturer, is not guaranteed or endorsed by the publisher.
Ab Hamid, M. R., Sami, W., and Sidek, M. M. (2017). Discriminant validity assessment: use of Fornell & Larcker criterion versus HTMT criterion. J. Phys. Conf. Series 890:012163. doi: 10.1088/1742-6596/890/1/012163/meta
Aditya, A. R., and Alversia, Y. (2019). The influence of online review on consumers’ purchase intention. J. Mgt. Mkt. Rev. 4, 194–201. doi: 10.35609/jmmr.2019.4.3(4)
Alam, M. M. D., Karim, R. A., and Habiba, W. (2021). The relationship between CRM and customer loyalty: the moderating role of customer trust. Int. J. Bank Mark. 39, 1248–1272. doi: 10.1108/IJBM-12-2020-0607
Alkhattabi, M., Neagu, D., and Cullen, A. (2011). Assessing information quality of e-learning systems: a web mining approach. Comput. Hum. Behav. 27, 862–873. doi: 10.1016/j.chb.2010.11.011
Alzate, M., Arce-Urriza, M., and Cebollada, J. (2021). Online reviews and product sales: the role of review visibility. J. Theor. Appl. Electron. Commer. Res. 16, 638–669. doi: 10.3390/jtaer16040038
Anderson, M., and Magruder, J. (2012). Learning from the crowd: regression discontinuity estimates of the effects of an online review database. Econ. J. 122, 957–989. doi: 10.1111/j.1468-0297.2012.02512.x
Anderson, E. T., and Simester, D. I. (2014). Reviews without a purchase: low ratings, loyal customers, and deception. J. Mark. Res. 51, 249–269. doi: 10.1509/jmr.13.0209
Andrade Cruz, B. D. P., Silva, S. C., and Dutt Ross, S. (2021). The social TV phenomenon and fake online restaurant reviews. Tour. Hosp. Manage. 27, 25–42. doi: 10.20867/thm.27.1.2
Arazy, O., and Kopak, R. (2011). On the measurability of information quality. J. Am. Soc. Inf. Sci. 62, 89–99. doi: 10.1002/asi.21447
Ariyono, K. Y., Irdiana, S., and Darmawan, K. (2022). Effect of online buying experience on customer loyalty. Int. J. Soc. Sci. 1, 549–552. doi: 10.53625/ijss.v1i5.1293
Banerjee, S. (2022). Exaggeration in fake vs. authentic online reviews for luxury and budget hotels. Int. J. Inf. Manag. 62:102416. doi: 10.1016/j.ijinfomgt.2021.102416
Banerjee, S., Chua, A. Y. K., and Kim, J.-J. (2017). Don’t be deceived: using linguistic analysis to learn how to discern online review authenticity. J. Assoc. Inf. Sci. Technol. 68, 1525–1538. doi: 10.1002/asi.23784
Barari, M., Ross, M., and Surachartkumtonkun, J. (2020). Negative and positive customer shopping experience in an online context. J. Retail. Consum. Serv. 53:101985. doi: 10.1016/j.jretconser.2019.101985
Beck, B. B., Wuyts, S., and Jap, S. (2023). Guardians of trust: how review platforms can fight fakery and build consumer trust. J. Mark. Res. 61, 682–699. doi: 10.1177/00222437231195576
Becker, L., and Jaakkola, E. (2020). Customer experience: fundamental premises and implications for research. J. Acad. Mark. Sci. 48, 630–648. doi: 10.1007/s11747-019-00718-x
Berry, L. L., Carbone, L. P., and Haeckel, S. H. (2002). Managing the total customer experience. MIT Sloan Manag. Rev. 43, 85–89.
Berry, L. L., and Parasuraman, A. (1997). Listening to the customer–the concept of a service-quality information system. MIT Sloan Manag. Rev. 38:65.
Bryanov, K., and Vziatysheva, V. (2021). Determinants of individuals’ belief in fake news: A scoping review determinants of belief in fake news. PLoS One 16:e0253717. doi: 10.1371/journal.pone.0253717
Cai, S., and Xu, Y. (2006). Effects of outcome, process and shopping enjoyment on online consumer behaviour. Electron. Commer. Res. Appl. 5, 272–281. doi: 10.1016/j.elerap.2006.04.004
Cano, J. A., Londoño-Pineda, A., Castro, M. F., Paz, H. B., Rodas, C., and Arias, T. (2022). A bibliometric analysis and systematic review on E-marketplaces, open innovation, and sustainability. Sustain. For. 14:5456. doi: 10.3390/su14095456
Cardoso, A., Gabriel, M., Figueiredo, J., Oliveira, I., Rêgo, R., Silva, R., et al. (2022). Trust and loyalty in building the brand relationship with the customer: empirical analysis in a retail chain in northern Brazil. J. Open Innov.: Technol. Mark. Complex. 8:109. doi: 10.3390/joitmc8030109
Chen, L., Li, W., Chen, H., and Geng, S. (2019). Detection of fake reviews: analysis of sellers’ manipulation behavior. Sustain. For. 11:4802. doi: 10.3390/su11174802
Chevalier, J. A., and Mayzlin, D. (2006). The effect of word of mouth on sales: online book reviews. J. Mark. Res. 43, 345–354. doi: 10.1509/jmkr.43.3.345
Cheung, C. M., Lee, M. E., and Rabjohn, N. (2008). The impact of electronic word‐of‐mouth: The adoption of online opinions in online customer communities. Internet Research, 18, 229–247.
Cho, H. S., Sosa, M. E., and Hasija, S. (2022). Reading between the stars: understanding the effects of online customer reviews on product demand. M&SOM 24, 1977–1996. doi: 10.1287/msom.2021.1048
Chuang, S.-C., Lin, F.-M., and Tsai, C.-C. (2015). An exploration of the relationship between internet self-efficacy and sources of internet self-efficacy among Taiwanese university students. Comput. Hum. Behav. 48, 147–155. doi: 10.1016/j.chb.2015.01.044
Cui, Y., Mou, J., Cohen, J., Liu, Y., and Kurcz, K. (2020). Understanding consumer intentions toward cross-border m-commerce usage: A psychological distance and commitment-trust perspective. Electron. Commer. Res. Appl. 39:100920. doi: 10.1016/j.elerap.2019.100920
Curchod, C., Patriotta, G., Cohen, L., and Neysen, N. (2020). Working for an algorithm: power asymmetries and agency in online work settings. Adm. Sci. Q. 65, 644–676. doi: 10.1177/0001839219867024
Dai, H., Chan, C., and Mogilner, C. (2020). People rely less on consumer reviews for experiential than material purchases. J. Consum. Res. 46, 1052–1075. doi: 10.1093/jcr/ucz042
Davis, F. D. (1989). Perceived usefulness, perceived ease of use, and user acceptance of information technology. MIS Q. 13, 319–340. doi: 10.2307/249008
Dery, L., Hermel, D., and Jelnov, A. (2021). Cheating in ranking systems. Rev. Ind. Organ. 58, 303–320. doi: 10.1007/s11151-020-09754-2
Deshai, N., and Rao, B. B. (2023). Transparency in healthcare and e-commerce: detecting online fake reviews using a dense neural network model with relevance mapping. Soft. Comput. 27, 9861–9875. doi: 10.1007/s00500-023-08437-w
Dhabliya, D., Ghule, G., Khubalkar, D., Moje, R. K., Kshirsagar, P. S., and Bendale, S. P. (2023). Robotic process automation in cyber security operations: optimizing workflows with AI-driven automation. J. Elect. Syst. 19, 96–105. doi: 10.52783/jes.654
Doney, P. M., and Cannon, J. P. (1997). An examination of the nature of trust in buyer–seller relationships. J. Mark. 61, 35–51. doi: 10.1177/002224299706100203
El-Said, O. A. (2020). Impact of online reviews on hotel booking intention: the moderating role of brand image, star category, and price. Tour. Manag. Perspect. 33:100604. doi: 10.1016/j.tmp.2019.100604
Filieri, R. (2015). What makes online reviews helpful? A diagnosticity-adoption framework to explain informational and normative influences in e-WOM. J. Bus. Res. 68, 1261–1270. doi: 10.1016/j.jbusres.2014.11.006
Filieri, R., McLeay, F., Tsui, B., and Lin, Z. (2018). Consumer perceptions of information helpfulness and determinants of purchase intention in online consumer reviews of services. Inf. Manag. 55, 956–970. doi: 10.1016/j.im.2018.04.010
Fogel, J., and Zachariah, S. (2017). Intentions to use the yelp review website and purchase behavior after reading reviews. J. Theor. Appl. Electron. Commer. Res. 12, 53–67. doi: 10.4067/S0718-18762017000100005
Gefen, D., Karahanna, E., and Straub, D. W. (2003). Trust and TAM in online shopping: an integrated model. MIS Q. 27, 51–90. doi: 10.2307/30036519
Gössling, S., Hall, C. M., and Andersson, A.-C. (2018). The manager’s dilemma: a conceptualization of online review manipulation strategies. Curr. Issue Tour. 21, 484–503. doi: 10.1080/13683500.2015.1127337
Goyal, P., and Deora, S. S. (2022). Trust management techniques and their challenges in cloud computing: a review. Int. J. Comput. Netw. Appl. 9, 761–774. doi: 10.22247/ijcna/2022/217708
Hair, J. F. (2011). Multivariate data analysis: an overview. Int. Encycl. Stat. Sci. 15, 904–907. doi: 10.1007/978-3-642-04898-2_395
Hair, J. F., Risher, J. J., Sarstedt, M., and Ringle, C. M. (2019). When to use and how to report the results of PLS-SEM. Eur. Bus. Rev. 31, 2–24. doi: 10.1108/EBR-11-2018-0203
Hajek, P., Barushka, A., and Munk, M. (2020). Fake consumer review detection using deep neural networks integrating word embeddings and emotion mining. Neural Comput. Applic. 32, 17259–17274. doi: 10.1007/s00521-020-04757-2
Hazim, M., Anuar, N. B., Ab Razak, M. F., and Abdullah, N. A. (2018). Detecting opinion spams through supervised boosting approach. PLoS One 13:e0198884. doi: 10.1371/journal.pone.0198884
He, H., and Zhu, L. (2020). Online shopping green product quality supervision strategy with consumer feedback and collusion behavior. PLoS One 15:e0229471. doi: 10.1371/journal.pone.0229471
Huang, A. H., Chen, K., Yen, D. C., and Tran, T. P. (2015). A study of factors that contribute to online review helpfulness. Comput. Hum. Behav. 48, 17–27. doi: 10.1016/j.chb.2015.01.010
Hussain, S., Ahmed, W., Jafar, R. M. S., Rabnawaz, A., and Jianzhou, Y. (2017). eWOM source credibility, perceived risk and food product customer’s information adoption. Comput. Hum. Behav. 66, 96–102. doi: 10.1016/j.chb.2016.09.034
Jin Ma, Y., and Lee, H.-H. (2014). Consumer responses toward online review manipulation. J. Res. Interact. Mark. 8, 224–244. doi: 10.1108/JRIM-04-2013-0022
Keen, P., Ballance, G., Chan, S., and Schrump, S. (1999). Electronic Commerce Relationships: Trust by Design. Prentice Hall PTR. Available at: https://dl.acm.org/doi/abs/10.5555/323559 (accessed October 5, 2023).
Kim, M.-S., and Ahn, J.-H. (2007). Management of trust in the E-marketplace: the role of the Buyer’s experience in building trust. J. Inf. Technol. 22, 119–132. doi: 10.1057/palgrave.jit.2000095
Kim, H., and Choi, B. (2013). The influence of customer experience quality on customers’ behavioral intentions. Serv. Mark. Q. 34, 322–338. doi: 10.1080/15332969.2013.827068
Kim, W. G., and Park, S. A. (2017). Social media review rating versus traditional customer satisfaction: which one has more incremental predictive power in explaining hotel performance? Int. J. Contemp. Hosp. Manag. 29, 784–802. doi: 10.1108/IJCHM-11-2015-0627
Lata, S., and Rana, K. (2021). What are the determinants of consumers’ online reviews adoption for Hotel bookings: a structural equation modelling approach. Enlight. Tour. Pathmaking J. 11, 171–193. doi: 10.33776/et.v11i1.5354
Lee, S., Qiu, L., and Whinston, A. (2018). Sentiment manipulation in online platforms: an analysis of movie tweets. Prod. Oper. Manag. 27, 393–416. doi: 10.1111/poms.12805
Lim, Y. J., Osman, A., Salahuddin, S. N., Romle, A. R., and Abdullah, S. (2016). Factors influencing online shopping behavior: the mediating role of purchase intention. Procedia Econ. Financ. 35, 401–410. doi: 10.1016/S2212-5671(16)00050-2
Liu, Z., and Park, S. (2015). What makes a useful online review? Implication for travel product websites. Tour. Manag. 47, 140–151. doi: 10.1016/j.tourman.2014.09.020
Liu, L., Zhao, X., Wang, H., Song, W., and Du, C. (2016). Research on identification method of anonymous fake reviews in e-commerce. TELKOMNIKA (Telecommunication Computing Electronics and Control), 14, 1510–1520.
Luca, M., and Zervas, G. (2016). Fake it till you make it: reputation, competition, and yelp review fraud. Manag. Sci. 62, 3412–3427. doi: 10.1287/mnsc.2015.2304
Malbon, J. (2013). Taking fake online consumer reviews seriously. J. Consum. Policy 36, 139–157. doi: 10.1007/s10603-012-9216-7
Mayer, R. C., Davis, J. H., and Schoorman, F. D. (1995). An integrative model of organizational trust. Acad. Manag. Rev. 20:709. doi: 10.2307/258792
Mayzlin, D., Dover, Y., and Chevalier, J. (2014). Promotional reviews: an empirical investigation of online review manipulation. Am. Econ. Rev. 104, 2421–2455. doi: 10.1257/aer.104.8.2421
McKnight, D. H., Choudhury, V., and Kacmar, C. (2002). Developing and validating trust measures for e-commerce: an integrative typology. Inf. Syst. Res. 13, 334–359. doi: 10.1287/isre.13.3.334.81
Muharam, H., Chaniago, H., Endraria, E., and Harun, A. B. (2021). E-service quality, customer trust and satisfaction: market place consumer loyalty analysis. J. Minds Manajemen Ide Inspirasi 8, 237–254. doi: 10.24252/minds.v8i2.23224
Munzel, A. (2015). Malicious practice of fake reviews: experimental insight into the potential of contextual indicators in assisting consumers to detect deceptive opinion spam. Recherche Appl. Market. 30, 24–50. doi: 10.1177/2051570715604155
Nakayama, M., and Wan, Y. (2017). Exploratory study on anchoring: fake vote counts in consumer reviews affect judgments of information quality. J. Theor. Appl. Electron. Commer. Res. 12, 1–20. doi: 10.4067/S0718-18762017000100002
Nguyen, P., Wang, X., Li, X., and Cotte, J. (2021). Reviewing experts’ restraint from extremes and its impact on service providers. J. Consum. Res. 47, 654–674. doi: 10.1093/jcr/ucaa037
Niranjani, V., Agalya, Y., Charunandhini, K., Gayathri, K., and Gayathri, R. (2022). Spam detection for social media networks using machine learning. In 2022 8TH International conference on Advanced computing and communication systems 2082–2088. IEEE: Piscataway, NJ.
Oliveira, R., Baldam, E. C. G. D. R., Costa, F. R., and Pelissari, A. S. (2020). The effect of perceived usefulness of online reviews on hotel booking intentions. Rev. Bras. Pesquisa Turismo 14, 30–45. doi: 10.7784/rbtur.v14i2.1695
Pavlou, P. A., and Dimoka, A. (2006). The nature and role of feedback text comments in online marketplaces: implications for trust building, price premiums, and seller differentiation. Inf. Syst. Res. 17, 392–414. doi: 10.1287/isre.1060.0106
Pavlou, P. A., and Gefen, D. (2004). Building effective online marketplaces with institution-based trust. Inf. Syst. Res. 15, 37–59. doi: 10.1287/isre.1040.0015
Peña-García, N., Gil-Saura, I., Rodríguez-Orejuela, A., and Siqueira-Junior, J. R. (2020). Purchase intention and purchase behavior online: A cross-cultural approach. Heliyon 6:e04284. doi: 10.1016/j.heliyon.2020.e04284
Risher, J., and Hair, J. F. Jr. (2017). The robustness of PLS across disciplines. Acad. Bus. J. 1, 47–55.
Sahut, J. M., Laroche, M., and Braune, E. (2021). Antecedents and Consequences of Fake Reviews: A Marketing Approach Short Title (VSI): Fake Reviews. Available at: https://dif2021.sciencesconf.org/data/pages/CFP_Fake_reviews_JoBR_V5.pdf (Accessed October 5, 2023).
Schoenmueller, V., Netzer, O., and Stahl, F. (2020). The polarity of online reviews: prevalence, drivers and implications. J. Mark. Res. 57, 853–877. doi: 10.1177/0022243720941832
Sebastianelli, R., and Tamimi, N. (2018). E-tailer website attributes and trust: understanding the role of online reviews. Online Inf. Rev. 42, 506–519. doi: 10.1108/OIR-02-2016-0039
Senthilkumar, R., and RubanRaja, B. (2021). Brand positioning and segmentation of sneakers through multi-dimensional customer experience analysis. J. Sci. Res. 13:2. doi: 10.3329/jsr.v13i2.47841
Shaheen, M., Zeba, F., Chatterjee, N., and Krishnankutty, R. (2019). Engaging customers through credible and useful reviews: the role of online trust. Young Consum. 21, 137–153. doi: 10.1108/YC-01-2019-0943
Shankar, V., Grewal, D., Sunder, S., Fossen, B., Peters, K., and Agarwal, A. (2022). Digital marketing communication in global marketplaces: a review of extant research, future directions, and potential approaches. Int. J. Res. Mark. 39, 541–565. doi: 10.1016/j.ijresmar.2021.09.005
Shapiro, S. (1987). The social control of impersonal trust. Am. J. Sociol. 93, 623–658. doi: 10.1086/228791
Siqueira, J. R., Peña, N. G., ter Horst, E., and Molina, G. (2019). Spreading the word: how customer experience in a traditional retail setting influences consumer traditional and electronic word-of-mouth intention. Electron. Commer. Res. Appl. 37:100870. doi: 10.1016/j.elerap.2019.100870
Sniezek, J. A., and Van Swol, L. M. (2001). Trust, confidence, and expertise in a judge-advisor system. Organ. Behav. Hum. Decis. Process. 84, 288–307. doi: 10.1006/obhd.2000.2926
Standing, S., Standing, C., and Love, P. E. (2010). A review of research on e-marketplaces 1997–2008. Decis. Support. Syst. 49, 41–51. doi: 10.1016/j.dss.2009.12.008
Statista (2023). Topic: E-Commerce in Latin America. Statista. Available at: https://www.statista.com/topics/2453/e-commerce-in-latin-america/ (Accessed October 5, 2023).
Thakur, R. (2018). Customer engagement and online reviews. J. Retail. Consum. Serv. 41, 48–59. doi: 10.1016/j.jretconser.2017.11.002
Tufail, H., Ashraf, M. U., Alsubhi, K., and Aljahdali, H. M. (2022). The effect of fake reviews on e-commerce during and after Covid-19 pandemic: SKL-based fake reviews detection. IEEE Access 10, 25555–25564. doi: 10.1109/ACCESS.2022.3152806
Viswanathan, M., Umashankar, N., Sreekumar, A., and Goreczny, A. (2021). Marketplace literacy as a pathway to a better world: evidence from field experiments in low-Access subsistence marketplaces. J. Mark. 85, 113–129. doi: 10.1177/0022242921998385
Wang, Y.-S., Yeh, C.-H., and Liao, Y.-W. (2013). What drives purchase intention in the context of online content services? The moderating role of ethical self-efficacy for online piracy. Int. J. Inf. Manag. 33, 199–208. doi: 10.1016/j.ijinfomgt.2012.09.004
Wu, Y., Ngai, E. W., Wu, P., and Wu, C. (2020). Fake online reviews: literature review, synthesis, and directions for future research. Decis. Support. Syst. 132:113280. doi: 10.1016/j.dss.2020.113280
Wu, S., Wingate, N., Wang, Z., and Liu, Q. (2019). The influence of fake reviews on consumer perceptions of risks and purchase intentions. J. Market. Dev. Compet. 13:3.
Yaari, E., Baruchson-Arbib, S., and Bar-Ilan, J. (2011). Information quality assessment of community generated content: a user study of Wikipedia. J. Inf. Sci. 37, 487–498. doi: 10.1177/0165551511416065
Yeap, J. A., Ignatius, J., and Ramayah, T. (2014). Determining consumers’ most preferred eWOM platform for movie reviews: a fuzzy analytic hierarchy process approach. Comput. Hum. Behav. 31, 250–258. doi: 10.1016/j.chb.2013.10.034
Zhang, K. Z., Zhao, S. J., Cheung, C. M., and Lee, M. K. (2014). Examining the influence of online reviews on consumers’ decision-making: a heuristic–systematic model. Decis. Support. Syst. 67, 78–89. doi: 10.1016/j.dss.2014.08.005
Zhao, M., Zhang, C., Hu, Y., Xu, Z., and Liu, H. (2021). Modelling consumer satisfaction based on online reviews using the improved Kano model from the perspective of risk attitude and aspiration. Technol. Econ. Dev. Econ. 27, 550–582. doi: 10.3846/tede.2021.14223
Keywords: trust, fake reviews, customer experience, marketplace, Colombia
Citation: Peña-García N, Losada-Otálora M, Auza DP and Cruz MP (2024) Reviews, trust, and customer experience in online marketplaces: the case of Mercado Libre Colombia. Front. Commun. 9:1460321. doi: 10.3389/fcomm.2024.1460321
Received: 08 July 2024; Accepted: 05 September 2024;
Published: 17 September 2024.
Edited by:
Tereza Semerádová, Technical University of Liberec, CzechiaReviewed by:
Ana Sousa, University of Aveiro, PortugalCopyright © 2024 Peña-García, Losada-Otálora, Auza and Cruz. This is an open-access article distributed under the terms of the Creative Commons Attribution License (CC BY). The use, distribution or reproduction in other forums is permitted, provided the original author(s) and the copyright owner(s) are credited and that the original publication in this journal is cited, in accordance with accepted academic practice. No use, distribution or reproduction is permitted which does not comply with these terms.
*Correspondence: Nathalie Peña-García, bmF0aGFsaWUucGVuYUBjZXNhLmVkdS5jbw==
Disclaimer: All claims expressed in this article are solely those of the authors and do not necessarily represent those of their affiliated organizations, or those of the publisher, the editors and the reviewers. Any product that may be evaluated in this article or claim that may be made by its manufacturer is not guaranteed or endorsed by the publisher.
Research integrity at Frontiers
Learn more about the work of our research integrity team to safeguard the quality of each article we publish.