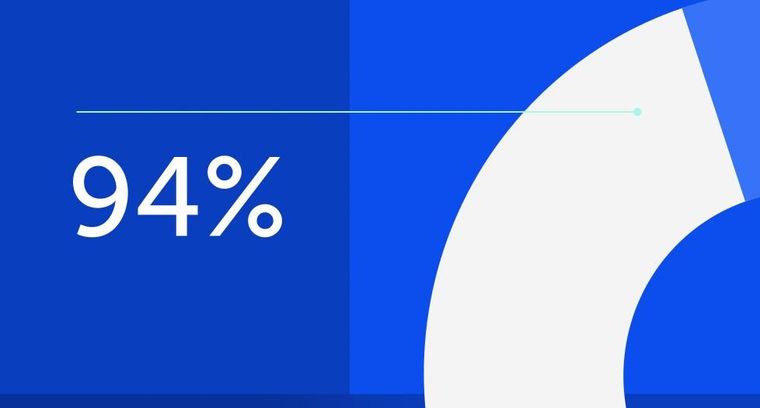
94% of researchers rate our articles as excellent or good
Learn more about the work of our research integrity team to safeguard the quality of each article we publish.
Find out more
ORIGINAL RESEARCH article
Front. Commun., 21 August 2024
Sec. Visual Communication
Volume 9 - 2024 | https://doi.org/10.3389/fcomm.2024.1440384
This article is part of the Research TopicCommunicating with Non-Humans: A New Visual LanguageView all 9 articles
As artificial intelligence (AI) continues to pervade our daily lives, expanding the boundaries of participatory design to include aspects of visual communication beyond humans is imperative. This research explores the intriguing convergence of participatory design and AI-enabled visual-based design as powerful methodologies for shaping the creation of new ideas (e.g., products/services). The specific research objectives are 3-fold: to unravel the nuanced dynamics in terms of perception inherent in the increasingly intertwined visual communication between humans and AI (e.g., sketch AI); to investigate how AI-enabled visual communication supports the participatory process with stakeholders; and to explore their experiences in AI-enabled visual-based design for non-human subjects (e.g., animals and plants). A laboratory study of 18 experimental groups found that AI-enabled visual-based design significantly enhances creative ideation performance when (novice) designers engage AI as a partner rather than merely a tool or collaboratively interact with stakeholders. Notably, the engagement level of internal stakeholders is much higher than that of external stakeholders.
Since the Bauhaus laid the foundation for modern design, every modern design is “if not explicitly participatory, at least programmatically collaborative” (Bannon and Ehn, 2012). Participatory design makes participation and skill development explicit and crucial in creative design practices (Schuler and Namioka, 1993). Extant participatory design practices have embraced diverse technologies, tools, environments, and businesses (Simonsen and Robertson, 2013). One central issue has been how human agents can drive the collaborative design process. With the increasing adoption or incorporation of artificial intelligence (AI) technology and applications by human agents, various new forms of intervention in the collaborative design space are apparent. Key benefits of involving AI include enhanced efficiency, improved prediction (e.g., of individuals' behaviors), and reduced time and human error, offering significant value to stakeholders' communications (e.g., Eisingerich et al., 2021). Recent examples of large language models (LLM) like Open AI's ChatGPT-4 can facilitate both verbal and text-based interactions, enabling dynamic communication in the design practice. Some particular AI agents, like AutoDraw, can assist with visual-based design by providing quick and intuitive sketching capabilities to suggest creative designs, thus coined “sketch AI” in this study. Sketching as a central design skill has drawn strong academic interest in creating novel ideas within groups in modern design (Van der Lugt, 2005; Paay et al., 2023). Often as a visual form, sketching has been proven to support human agents in problem identification, idea exploration, and solution development in an effective and depictive way, thereby helping communicate their ideas to other stakeholders in design meetings by using papers or digital media to date (Fish and Scrivener, 1990; Van der Lugt, 2005; Olofsson and Sjölen, 2006; Paay et al., 2023). However, there has been a lack of research on the participatory process of stakeholders engaging with a visual-based (sketch) AI agent in creative design.
Nevertheless, the increasing demands for integrating technology and social, economic, and environmental values further press the need to include both humans and non-humans in creative design practices. This expanded focus on design underlies more inclusivity for creating more adaptable solutions. By incorporating the perspectives and impacts of non-humans, such as animals, plants, and even AI agents, whereby verbal communications can be restricted, the inclusivity in a visual-based design fosters a deeper understanding of diverse contexts in which complex interactions between people, technology, and the world occur.
Instead of a single theory or technique, participatory design not only represents a collection of diverse perspectives and experiences but also a predominant value (Schuler and Namioka, 1993). It prioritizes a more humane and effective relationship between stakeholders involved in the design process to meet end-users' needs and preferences (Schuler and Namioka, 1993). The participatory design-related design traditions have been within the realm of human-centered approaches, emphasizing the direct involvement of human representatives as the stakeholders in creative practices (Wilkinson and De Angeli, 2014). The conceptualization of the process reflects the possible collaborative efforts that could foster a sense of ownership and empowerment among the involved stakeholders as a group and ultimately lead to more innovative and user-friendly designs. One classic model to study the participatory design group emphasizes the placement of the group through the entire design process (Wilkinson and De Angeli, 2014). In this research, we adapted this model to conceptualize the involvement of an AI agent for creative ideation beyond just humans (see Figure 1).
The adapted model maintains the group's original central role while distinctively incorporating an AI agent into the group, highlighting its possible contributions to the participatory design process in front-end design. The other adaptation informs the front-end design process in the model, particularly regarding creative ideation. The cycle of the four main designerly activities concerning creative ideation is specified from problem to solution. It is worth mentioning that the activity starts with problem identification, which is not limited to human needs but also extends to non-human related needs or subjects. Then, a series of activities related to creative ideation follows within the solution space. In light of Sanders (2002) notion of participatory design, each agent from the participatory group is believed to offer inputs at every stage of the design process when having the media to express themselves in terms of generating new thinking and ideas. Sketching is acknowledged as an appropriate medium to capture current thoughts, inputs, and feedback from everyone involved (e.g., designers and stakeholders), ideally at every stage, with input from everyone involved. This study, then, attempts to approach the AI-enabled visual design through sketching and involves the human agents in a front-end design process, seeking an advanced understanding of the intertwined dynamics within the participatory design group.
This study is set to contribute to what is underexplored in the theory and practice of participatory design, focusing on its new relevance in a visual AI-enabled scene of front-end design. For instance, how does the group interact to communicate and internalize the problem and then create solutions with specific visual AI applications? We link the participatory design theory with the process of examining AI involvement, stakeholder engagement, and creative design interfaces for non-human subjects. Past cases by Verganti et al. (2020) demonstrated that AI reshapes design practices focusing on solving problems. Nevertheless, few have investigated the dynamics and characteristics of AI usage on different stakeholder engagement and the different perceived roles of AI agents in the front-end design space where creativity and imagination issues matter the most (Wetzels, 2021). Dated back to the 1980s, stakeholder theory (Donaldson and Preston, 1995), which defined stakeholder engagement as “a stakeholder's [cognitive, emotional, and behavioral] resource endowment in his/her role-related interactions, activities, and/or relationships” (Hollebeek et al., 2022, p. 328), presents a key issue to boost stakeholder communication/collaboration in digital environments (e.g., Viglia et al., 2018). Since stakeholders differ in their needs and roles as internal stakeholders (e.g., focal designer or team member) or external stakeholders (e.g., customer or users) in the participatory design group (Freeman, 2010), studies on stakeholders' engagement in different participatory contexts with specific AI-enabled (visual) applications have been scarce. In response, the renewed interdisciplinary methodological focus in this study holds the original contributions to enhancing the front-end design process through the scientific exploration of the perceived roles of a specific AI (visual) agent, the stakeholders' engagement or communications in different participatory contexts, and their impact on ideation performance for non-human subjects. It also has practical implications, such as how to engage with visual AI together with participatory design for creative outcomes.
This study explores multiple real-world cases in our laboratory settings, where the conceptual model (i.e., Figure 1) has been instrumental in studying interaction modes between humans and visual AI. In this study, we conduct experiments within the controlled environment with novice designers, sketch AI, and potential stakeholders. The experimental data help unveil which perceived roles of the AI agent matter and how AI-enabled visual communication facilitates stakeholder collaborations in the participatory process of ideating for non-human subjects. Ultimately, this study acknowledges the research limitations and encourages further research and practical implementation of these methodologies to shape the future of visual-based communication in creative design.
This study employed an exploratory, experimental approach. According to Schön (1984), an exploratory experiment is “when an action is undertaken only to see what follows, without accompanying predictions or expectations” (p. 144). This experiment was set to openly explore the creative design actions of the participatory design group, which was ideating for non-human subjects. Given the evolving phenomena and nascent theoretical underpinnings of our research inquiries, we embraced the design logic in research (Stompff et al., 2022). As such, research inquiries are also practice-led design inquiries that account for the development of induced theory, aspiring to be “provisional, aspirational, and contingent” (Gaver, 2014). The experiment was designed to reach contextual evidence and derive insights based on the conceptual model of activities (Figure 1), which assembles the core quality of research through design (Prochner and Godin, 2021). Drawing from Dewey's pragmatism logic to “purposefully introduce changes which will alter the direction of the course of events” (Dewey, 1929, p. 81), the inquiries regarding participatory design groups with both human and AI agents were addressed in the form of designed interventions in their formation (Halse and Boffi, 2016). The purpose of this experimental design not only corresponded to key research inquiries in terms of perception inherent to the visual AI and stakeholder engagement dynamics during the participatory process but also to create new knowledge. The perceptions of the visual AI and different types of stakeholders could be viewed as behavioral choices by a human agent and, thus, possibly modified and intervened (March, 1978). The new knowledge could be raised in the dynamic interplay of a human agent's actions and responses under the intervened contexts by adhering to the process: “we undergo a situation, act upon it, and reflect on outcomes” (Stompff et al., 2022, p. 3). In other words, the experiment was designed for observable evolving situations through interventions and to study the pathways of actual actions and their impact. It is plausible for “an experiment of finding out what possible lines of action are really like (..) in a dramatic rehearsal (in imagination) of various competing lines of action” (Dewey, 1922, p. 190). The corresponding analysis across the contexts can reflect on outcomes and help learn how to act for more creative ideation performance.
The experimental setting was undertaken as a controlled environment, which minimized external influences on the study's outcomes. The setting reflected the intended experimental design to assess the interplay between humans and AI agents within the participatory design group. It configured the key environmental conditions regarding the group formation, the contexts in which the group is acting, the tasks to perform, and the population of participants. Through designed interventions, the controlled environment was set for its validity, replicability, and reliability with respect to small-scale design experimentation (Cash et al., 2012; Stompff et al., 2022).
In the participatory design group setting, the visual-based AI agent is the sketch AI (i.e., autodraw.com, Alphabet Inc.) designed for collaborating with human agents. This is because, once an input of any sort of sketch is provided by a participant, simple or complex, AutoDraw can predict and generate what is believed to be relevant computed shapes and sketches simultaneously as options for the participants to use. The setting involved the target participants due to its accessibility, importance to the homogeneity, and relevance to the research inquiries. The participants were recruited among graduate students aged 25–35 years from a top European university. The inclusion criteria also included the ownership of an animal or a plant or having a history of taking care of either one. The initial candidates were further filtered to include only novice designers, participants who have never professionally worked in design and have never used AutoDraw before. Qualified participants were randomly allocated to one of the six contexts shown in Table 1. In Contexts 1 and 2, participants were given a single intervention to perceive the AI agent as either a tool or a creative partner, respectively. In Contexts 3 and 4, participants were given two interventions: one for the perceived role of the AI agent and the other for the stakeholder role of other human agents. Herein, the participants were randomly assigned roles as either a teammate to the focal designer (as the representative of internal stakeholders) or a customer (as the representative of external stakeholders) when joining the participatory design group.
The task setting for the participatory design group is aligned with the four designer activities in the conceptual model (Figure 1), which aims to create good ideas for non-human subjects. According to the conceptual model, the designerly activities, including problem identification, initial ideation, (best) idea selection, and idea recombination/improvement, are indexed as Stages 1–4. The descriptions of the tasks were specified. The tasks for each context were the same and included four timed stages, as shown in Table 2.
The experiment was conducted over 2 months in early 2024. With the pre-approval of the Norwegian Social Science Data Services (NSD), informed consent was obtained from all participants. Data collection procedures were conducted in accordance with the ethical standards of the NSD. To ensure confidentiality and privacy, all data were anonymized and stored securely. Participants were informed of their rights to withdraw from the study at any point without any consequences.
All participants received a context description regarding AI and/or other human participants and the tasks. Each group was given a tablet with AutoDraw to sketch and capture their solutions, with a brief orientation to its usage beforehand. The orientation was implemented to mitigate the likely biases regarding different levels of exposure to the increasing wave of AI among the participants. The implemented strategies to mitigate potential limitations in data collection also included the strict experiment setting, the random allocation of the participants, questions of background information, self-reporting of experience with AI, and stakeholder communications.
Furthermore, the key metrics from the experiments were recorded for data collection and analysis. Each experimental context was run three times with different participants. In total, there were 18 groups for the six contexts, involving 30 participants. The participants comprised 14 female and 16 male students with bachelor's or postgraduate degrees in various domains. Data were collected during the entire experiment via video recording, and sketches were saved on the tablet. Each participant completed the survey at the end of the group's sketching activities. Video, survey, and sketch data were all analyzed. The primary outcome measures included self-reporting of their experience in the surveys. The video data were coded to identify behaviors among the interactions between the agents. The sketches were used to validate survey responses. The cross-context analysis was employed to inform the primary experimental results. With a small sample size of three groups per context, the cross-context analysis was important to limit performance variability across the three groups. This qualitative analysis enabled an in-depth understanding of the experimentation data by identifying and discussing the cross-context differences in actual actions and impact in detail. The overarching themes aligned with the designed interventions have led us to structure the analysis to answer the following research inquiries.
Based on the observational data, most of the participatory design group chose a problem close to their life experience about animals or plants, and only a few chose fictional characters or aliens. The design sketches the group generated were commonly treated as a proxy for capturing the externalizations of ideas in a tentative form toward a solution (Paay et al., 2023). The total number of generated ideas by the groups in each context is summarized in Table 3.
The above data in Contexts 1 and 2 reveal that the perception of the AI agent, either as a tool or a creative partner in the controlled environment, is most likely to impact the ideation outputs. The video and sketch data from the video also disclose that individual designers are more engaged in working with AI in Context 2 (AI as a creative partner) and have more creative ideas in comparison to those in Context 1 (AI as a tool). However, when the potential stakeholders joined the participation design group, the scenes of the front-end design collaboration became more complex. While engaging the visual AI as a tool, ideation outputs by the groups with internal stakeholders (Context 3a, the other focal designer as a teammate) outperformed those by individual designers (Context 1) but not the groups with external stakeholders (Context 3b, the customer). While engaging the visual AI as a partner, neither groups with internal nor external stakeholders (in Contexts 4a and 4b) generated more ideas than individual designers (Context 2).
Moreover, the groups' performance in the process of engaging AI as a tool (Context 3a or 3b) is seemingly indifferent from those that engaged AI as a creative partner (Context 4a or 4b). It is questionable to what extent the groups in Context 4a and 4b managed to engage AI as a creative partner instead of a tool, as several groups reminded themselves in the later stage about this setting in the video footage. Regardless of the perceptions of AI, among those groups with stakeholders, we found that the involvement of an internal stakeholder (a teammate) created more ideas than the ones of an external stakeholder (a customer). This result could pertain to stakeholder engagement and their interactions with the visual AI, which are further explored in the following analysis.
Based on the recorded videos, the groups' behavior interactions focusing on AI usage are coded for each task (Stages 1–4, see Table 2) in the experiment. Table 4 summarizes the interaction between the focal (novice) designer and the AI agent in Contexts 1 and 2. The observed interactions were regarding whether and how many novice designers used the visual AI to sketch their thoughts and ideas and/or used the sketch components that AI suggested in their front-end design activities, respectively. As a result, it was found that the degree of interaction between the novice designer and the visual AI agent was much higher in Context 2, where AI is perceived as a creative partner instead of a tool in Context 1. In the design process, novice designers commonly use visual AI as a sketching and viewing interface. The high degree of interaction also appeared in the later stage of idea recombination or improvements in both Contexts 1 and 2. In Context 2, most novice designers used AI suggestions throughout, except during idea selection.
Across the two high-level Contexts 3 and 4 (either a tool or a partner), we did not find a clear difference in AI usage by the different participatory design groups. However, we identified clear variations in interactions between the focal designers and different types of stakeholders. Despite Table 5 showing nearly the same level of AI usage for sketching in problem identification (Stage 1) across Contexts 3 and 4, the adoption of AI suggestions varied across different groups within Stage 1. Similarly, all focal designers and stakeholders tended to work together with the AI agent in the later stages, but to varied extents. All of the groups used AI for sketching in the initial ideation (Stage 2), but the idea selection stage (Stage 3) was mostly dominated by discussions between the focal designer and stakeholders, likely due to the lack of chat functionality in AutoDraw. The successful adoption of AI suggestions was most likely to happen in the later stage of idea combination and improvement (Stage 4).
Table 5. The high-level interactions between the conventional participatory design group (including focal designer and stakeholder) and the visual AI agent.
Based on the video-recording data, the common interactions between a focal designer and an internal stakeholder (teammate) were identified. They started by discussing and agreeing on which non-human subjects or problems to focus on and then generally acted on sketching the problem. The data revealed that most of the focal designers and internal stakeholders built on each other's ideas, and most of them took turns using AI to sketch and add AI components, with one exception, that was, both teammates would like to sketch simultaneously. The interactive sketching pattern while discussing in this group yielded the highest number of ideas than others in the same context (Context 4a, AI as a partner). Generally, the feedback from the internal stakeholders to the focal designer occurred interchangeably with the sketching activity at every stage.
In Contexts 3b and 4b, which involved the external stakeholder (customer), the customer mostly suggested the design problem in Stage 1. Nevertheless, the focal designers took the lead in the later stages while the customers provided feedback alongside. Whether to sketch and sometimes who to sketch were apparent discussion topics between the focal designer and customer, starting from Stage 1 and spreading out in later stages. In most cases, we found the focal designer to sketch, and the customer only added suggestions by engaging in discussions afterwards. In many cases, while the focal designers were sketching, the customers looked away or even used their cellular phones for unrelated activities. As such, the interactions and communications with external stakeholders were limited compared to those with internal stakeholders in the AI-enabled visual design. This reduced engagement with external stakeholders is more consistent with a known design meeting for feedback instead of active co-creation.
Furthermore, we examined the survey data for self-reported experiences by the participants, including both focal designers and stakeholders. With a focus on interactions with the visual AI agent and stakeholder engagement and communication, the key self-reported experiences are summarized in Table 6.
The survey data confirms that the formulated perceptions of the AI agent's role altered the focal designers' experience while interacting with inputs from AI. Under the premise that AI is a tool (Context 1), the focal designers reported no positive experience with the generative functions of the AI agent. However, in the context of AI as a partner (Context 2), the focal designers reported mostly positive experiences with AI usage. For the contexts where internal stakeholders (i.e., another designer/teammate) were involved in the participatory process, more positive experiences appeared when AI was viewed as a partner than when AI was viewed as a tool. However, in involving the external stakeholders (customers), we found mostly positive experiences in both circumstances of AI as a partner and as a tool.
Moreover, it is consistently shown that the internal stakeholders had a higher degree of engagement than the external stakeholders. One participant indicated that working with the other novice designer as a teammate was beneficial for such creative tasks. Another participant also mentioned good (verbal) communication while collaborating with the internal stakeholder but did not use the AI agent during the communication. It is possible that the limited timeframe in the experiment affected the trade-off between communication with stakeholders and AI usage. This study's cross-context evidence shows that communication with stakeholders pressed the usage of visual AI. In the context where external stakeholders were involved, the focal designer's most common response was to meet the customers' needs, expectations, and feedback. One participant indicated that better sketching skills would help benefit more from AI usage and meet the customers' high expectations. Regardless of AI usage, the determining performance of creative ideation by the participatory design group is mostly likely associated with how the focal designer and the stakeholder interact and communicate. Looping back to the number of generated ideas (shown in Table 3), the groups with internal stakeholders (Context 3a or Context 4a) outperformed the ones with external stakeholders (Context 3b or Context 4b). Therefore, in the visual-based AI design, the higher performance of front-end design benefits from the higher-degree engagement and more collaborative behaviors within the participatory design group instead of responsive behaviors from one human agent to the other.
This study contributes to the growing international community of scholars and practitioners engaged in participatory design practices beyond humans in the AI age. In particular, it advances the conceptual development of AI–stakeholder engagement and creative design interfaces through the lens of participatory design. It illustrates a research inquiry through pragmatism-based experiments in real-world situations (Dewey, 1922). The core of the experiment involves interventions designed around the involvement of a visual AI agent and stakeholders, which is based on literature-supported reasoning and the participatory design research model (Dewey, 1929; Wilkinson and De Angeli, 2014). New knowledge emerged from the cases in the experiment, which were self-reported, observed, and reflected upon in terms of participants' actual actions and experiences (Dewey, 1938; Stompff et al., 2022). This study thus offers a more holistic perspective that challenges participatory design-related traditions solely centered around users and designers.
The results presented have direct implications for engaging with visual AI and stakeholders, ultimately influencing the potential creative performance of every designed product or service for non-humans. This study is the first to present evidence that the human perception of a visual AI agent has a major impact on creative performance. Specifically, it was found that the human perception of AI's role, whether as a tool or as a creative partner in an experiment, matters. The preferred perception of the AI agent as a creative partner can be fostered in the working environment. Moreover, the identified differences in engagement levels and interactive behaviors provide insights for managing the participatory process when different types of stakeholders are involved. Higher levels of stakeholder engagement and collaborative interaction between stakeholders and focal designers are recommended for more creative outcomes. Potential differences in visual AI-based teamwork experiences were observed in relation to the perceptions of AI agents' roles. However, we did not find critical instances of those different roles that visual AI plays in the development of stakeholder engagement and communication in this study.
It is worth mentioning that this explorative study has a limited scope, such as using a specific visual AI, involving a participant pool of novice designers, and investigating only two types of stakeholders (i.e., teammate and customer) in the participatory process of front-end design. However, the conceptual model and method proposed in this study could serve as a foundation for future studies. For instance, additional studies could be conducted to determine whether similar findings hold in a more longitudinal setting and involve experienced designers. A greater number of characterized stakeholders (e.g., with different business functions in organizations), diverse forms of AI-enabled design applications (e.g., verbal, text, video, etc.), and subsequent design activities after the front-end design phases (e.g., prototyping, testing, etc.) also warrant further exploration. Further understanding of the role of an AI agent in influencing perceived tensions, vulnerabilities, and consequences, whether for different types of stakeholders, as well as in optimizing strategies for designing better AI-based stakeholder engagement and their responses to one another, can be valuable for future development.
The datasets presented in this article are not readily available because the observational videos potentially contain identifiable data, thus not being possible to share. Part of the data (i.e., survey data) is shareable without identifiable data. Requests to access the datasets should be directed to YYZ, eWFuZ3l6QHVpby5ubw==.
The studies involving humans were approved by NSD (Norwegian Center for Research Data). The studies were conducted in accordance with the local legislation and institutional requirements. The participants provided their written informed consent to participate in this study.
YYZ: Conceptualization, Data curation, Formal analysis, Methodology, Project administration, Resources, Visualization, Writing – original draft, Writing – review & editing, Validation.
The author(s) declare that no financial support was received for the research, authorship, and/or publication of this article.
The author declares that the research was conducted in the absence of any commercial or financial relationships that could be construed as a potential conflict of interest.
All claims expressed in this article are solely those of the authors and do not necessarily represent those of their affiliated organizations, or those of the publisher, the editors and the reviewers. Any product that may be evaluated in this article, or claim that may be made by its manufacturer, is not guaranteed or endorsed by the publisher.
Bannon, L. J., and Ehn, P. (2012). Design: “design matters in participatory design,” in Routledge Handbook of Participatory Design, eds. J. Simonsen and T. Robertson (New York, NY: Routledge), 37–63.
Cash, P., Elias, E., Dekoninck, E., and Culley, S. (2012). Methodological insights from a rigorous small scale design experiment. Design Stud. 33, 208–235. doi: 10.1016/j.destud.2011.07.008
Dewey, J. (1922). Human Nature and Conduct: An Introduction to Social Psychology. New York, NY: Henry Holt And Company.
Dewey, J. (1929). The Quest for Certainty: A Study of the Relation of Knowledge and Action. New York, NY: Minton, Balch & Company.
Donaldson, T., and Preston, L. (1995). The stakeholder theory of the corporation: concepts, evidence, and implications. Acad. Manag. Rev. 20, 65–91. doi: 10.2307/258887
Eisingerich, A., Lin, Y., and Doong, H. (2021). Avatar design of virtual salespeople: mitigation of recommendation conflicts. J. Serv. Res. 24, 141–159. doi: 10.1177/1094670520964872
Fish, J., and Scrivener, S. (1990). Amplifying the mind's eye: sketching and visual cognition. Leonardo 23, 117–126. doi: 10.2307/1578475
Freeman, R. E. (2010). Strategic Management: A Stakeholder Approach. Cambridge: Cambridge University Press.
Gaver, W. (2014). “Science and design: the implications of different forms of accountability,” in Ways of Knowing in HCI, eds. J. Olson, and W. Kellog (New York, NY: Springer), 143–165.
Halse, J., and Boffi, L. (2016). “Design interventions as a form of inquiry,” in Design Anthropological Futures, eds. R. C. Smith, K. T. Vangkilde, T. Otto, M. G. Kjaersgaard, J. Halse, and T. Binder (London: Bloomsbury Publishing), 89–103.
Hollebeek, L., Kumar, V., and Srivastava, R. K. (2022). From customer-, to actor-, to stakeholder engagement: taking stock, conceptualization, and future directions. J. Serv. Res. 25, 328–343. doi: 10.1177/1094670520977680
March, J. G. (1978). Bounded rationality, ambiguity, and the engineering of choice. Bell J. Econ. 9, 587–608. doi: 10.2307/3003600
Paay, J., Kjeldskov, J., Bønnerup, M., and Rasenthiran, T. (2023). Sketching and context: exploring creativity in idea generation groups. Design Stud. 84:101159. doi: 10.1016/j.destud.2022.101159
Prochner, I., and Godin, D. (2021). Quality in research through design projects: recommendations for evaluation and enhancement. Design Stud. 78, 1–34. doi: 10.1016/j.destud.2021.101061
Sanders, E. B. N. (2002). “From user-centered to participatory design approaches,” in Design and the Social Sciences, ed. J. Frascara (London: CRC Press), 18–25.
Schön, D. A. (1984). The Reflective Practitioner: How Professionals Think in Action. New York, NY: Basic Books.
Schuler, D., and Namioka, A. (1993). Participatory Design: Principles and Practices. New Jersey: CRC Press.
Simonsen, J., and Robertson, T. (2013). Routledge International Handbook of Participatory Design. New York, NY: Routledge.
Stompff, G., van Bruinessen, T., and Smulders, F. (2022). The generative dance of design inquiry: exploring Dewey's pragmatism for design research. Design Stud. 83:101136. doi: 10.1016/j.destud.2022.101136
Van der Lugt, R. (2005). How sketching can affect the idea generation process in design group meetings. Design Stud. 26, 101–122. doi: 10.1016/j.destud.2004.08.003
Verganti, R., Vendraminelli, L., and Iansiti, M. (2020). Innovation and design in the age of artificial intelligence. J. Prod. Innovat. Manag. 37, 212–227. doi: 10.1111/jpim.12523
Viglia, G., Pera, R., and Bigné, E. (2018). The determinants of stakeholder engagement in digital platforms. J. Bus. Res. 89, 404–410. doi: 10.1016/j.jbusres.2017.12.029
Wetzels, M. (2021). The road ahead is digital for innovation management and there is no way back. J. Prod. Innovat. Manag. 38, 245–247. doi: 10.1111/jpim.12571
Keywords: participatory design, visual AI, sketching, non-humans, stakeholder communication
Citation: Zhao YY (2024) The creative cosmos beyond humans: a symphony of participatory design and visual artificial intelligence. Front. Commun. 9:1440384. doi: 10.3389/fcomm.2024.1440384
Received: 29 May 2024; Accepted: 30 July 2024;
Published: 21 August 2024.
Edited by:
Melanie Sarantou, Kyushu University, JapanReviewed by:
Satu Miettinen, University of Lapland, FinlandCopyright © 2024 Zhao. This is an open-access article distributed under the terms of the Creative Commons Attribution License (CC BY). The use, distribution or reproduction in other forums is permitted, provided the original author(s) and the copyright owner(s) are credited and that the original publication in this journal is cited, in accordance with accepted academic practice. No use, distribution or reproduction is permitted which does not comply with these terms.
*Correspondence: YangYang Zhao, eWFuZ3l6QHVpby5ubw==
Disclaimer: All claims expressed in this article are solely those of the authors and do not necessarily represent those of their affiliated organizations, or those of the publisher, the editors and the reviewers. Any product that may be evaluated in this article or claim that may be made by its manufacturer is not guaranteed or endorsed by the publisher.
Research integrity at Frontiers
Learn more about the work of our research integrity team to safeguard the quality of each article we publish.