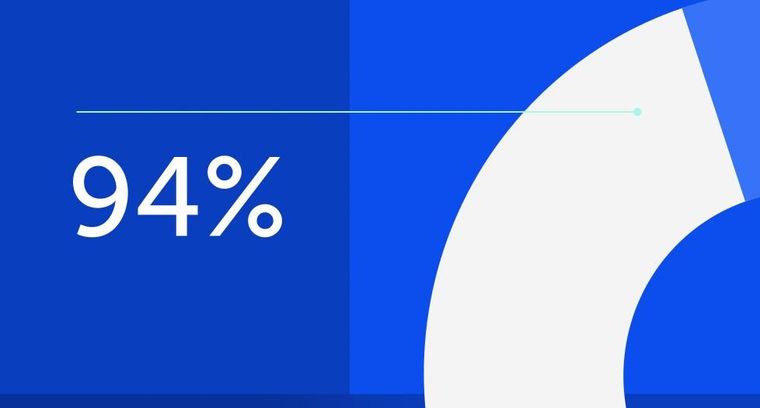
94% of researchers rate our articles as excellent or good
Learn more about the work of our research integrity team to safeguard the quality of each article we publish.
Find out more
ORIGINAL RESEARCH article
Front. Commun., 26 June 2024
Sec. Media Governance and the Public Sphere
Volume 9 - 2024 | https://doi.org/10.3389/fcomm.2024.1410995
This article is part of the Research TopicThe Impact of Artificial Intelligence on Media, Journalists, and AudiencesView all 6 articles
As technological advancements continue to shape our daily lives, and social TV has emerged as an interactive platform that fosters connections between families and friends. This study investigates the selection of social TV content by examining the influence of AI and other contributing factors, with gender proposed as a mediating factor. Involving 300 students from two randomly selected public universities in Irbid, Jordan, data were collected through an online survey with self-reported responses. The study revealed that AI enhances characteristics such as information gathering, social awareness, and knowledge sharing, subsequently affecting user attitudes and content selection on social TV platforms. Both AI and user attitudes significantly contribute to content selection, while gender acts as a mediator, influencing AI integration and attitudinal shifts. Ultimately, AI provides seamless access to preferred content and improves ease of use, enriching content recommendation systems and fostering increased user interest and satisfaction. This positive experience with AI services shapes user attitudes towards technology. This study offers a comprehensive examination of the intersection between AI, gender, attitudes, and social TV content selection within the context of Jordanian young users, marking a pioneering contribution to the field of media studies in the MENA region.
The evolution of AI has led to a deep integration of this technology in various sectors, transforming the way we live and work. From improving medical diagnoses to personalizing educational and entertainment experiences (Goni and Tabassum, 2020), AI is playing a crucial role in advancing society especially in the media and its various means. The impact of AI on media is broad and multifaceted, transforming the way media content is produced, distributed and consumed (Tahat et al., 2023). While it offers numerous opportunities for efficiency and customization (Alhussain et al., 2020). Social TV is not a new phenomenon anymore, as the rise of online networks was the initial step to introducing a newly developed way to provide ease of communication accompanied by accessibility to viewers (Barker, 2018; Kim et al., 2020). Later significant additions also played a role in the evolving social media technology (Harboe, 2010). For example, computers before that were big machines that were hard to fit in a room and had limited operating capabilities. Later, the size of computers decreased, then converted into portable laptops, and now smart portable devices such as mobile watches, laptops, and tablets. These devices decreased in size, became user-friendly, and introduced new platforms for information, communication, education, and entertainment (Odunaiya et al., 2020). In this sense, social TV is a significant addition to the services of these portable devices to their potential users. More specifically, social TV merges all relevant qualities (information, communication, education, and entertainment) in one place, where users feel more comfortable and facilitated in the exposure and selection of content. For example, social TV platforms (Netflix) offer several services to their users several services (Goel and Batra, 2017). Subscription, content search, selection, and even if a user misses a program, the system reminds and redirects to the same page to help the users continue watching from where they left. Other platforms include Hulu, Amazon Prime, Apple TV, and others (Guo, 2019). Choi (2022) considers affordable rates as another important reason behind social TV selection. As noted, despite traditional media being economic friendly and offering several services to users, social TV is improved at affordable rates. Subscribers pay an affordable fee and have access to the content whenever and whatever they want. As a result, social TV users are increasing worldwide, heralding a great transformation in the viewing patterns of conventional television.
Similarly, while discussing the importance of the feature represented by social TV, it is important to discuss the technological aspect that is also accelerating its adoption among users. For Kerres and Buntins (2020), the relevant technological aspect is an intelligent automated system based on AI that is widely designed and deployed for user convenience and satisfaction. Social awareness is another vital purpose, as online media are widely used as knowledge exchange and information gathering venues. However, as a part of tremendous technological growth and development, AI is well-suited to these purposes on social media, particularly social TV platforms. As a result, a positive and optimistic attitude towards social TV adoption is seen (Zawacki-Richter et al., 2019). For example, they stated that Jordanian youth is rapidly adopting social TV to enhance their television watching experiences (Sultana, 2020).
On the other hand, sharing thoughts, opinions, and experiences are also important factors behind the adoption of social networks among the relevant youth. It is noted that the young generation considers being part of an online community an important part of their daily lives. When virtual catchups replace physical meetings, the young generation is more likely to adopt online platforms that require less effort, yet positive and constructive outcomes. However, many consider personal factors, such as demographics, to strongly affect online platforms in general and AI adoption. Gender is one of these demographical factors (Langley, 2019) that accelerates or declines social TV content. Although previous studies have well-witnessed gender as a strong factor influencing social media selection and usage, we can observe a scarcity of literature analyzing the role of gender in social TV content selection.
Therefore, this study focuses on the perceptions of AI in the generation of social TV content. Notably, on social TV platforms, e.g., Netflix, Amazon Prime, Apple TV, etc. that are gradually rooting in Jordan. Users are showing an optimistic intention and adoption process. However, little is known about the reasons for accelerating the relevant adoption process. This study divides this article into several sections to provide a systematic and empirical investigation. The first part of this research involves a brief discussion about the adoption of social media, its popularity among Jordanian audiences, and their perceptions of AI in content selection. The second part involves a review of the supporting literature, which further leads to developing the conceptual model and the hypotheses. The third part discusses and highlights the most suitable methods used. The last section involves data analysis and results. Finally, the fifth part discusses the conclusion and limitations with future research recommendations.
The increased pace of data has compelled marketing to adopt new types of exploitation for the rapid processing of these data and to better satisfy consumer expectations. Today, the issue is not a lack of information or processing tools, but rather a lack of time. Internet users do not want to wait anymore; they prefer to have their requests completed promptly and without restrictions (Ye et al., 2020). The appearance of AI has made this task possible. With the immense opportunities, it provides, handling these vast amounts of data has become quite simple, and new marketing avenues have been opened to precisely and immediately contact all Internet users precisely and immediately. However, many of us have an irrational vision of AI, which is influenced by American blockbusters in which a robot of the future destroys or controls the human race. AI has nothing to do with it, but integrates predictive intelligence with the platforms we use to make daily life more intuitive and intelligent (Ye et al., 2020). The relationships between AI and the variables of social awareness, knowledge sharing, and information gathering are bidirectional (Habes et al., 2024). AI not only influences these variables, but is also influenced by them, creating a feedback loop that continually improves the personalization and relevance of content in SVODs. This analysis provides a deeper understanding of how to optimize recommendations and improve user satisfaction through an integrated approach to AI and user behavior (Somandepalli et al., 2021; Ahn et al., 2022).
According to Cant et al. (2017), using AI on these platforms has become standard and commonplace. YouTube, for example, recommends content based on the browsing and search history of a user on the platform. Facebook suggests certain users on its network based on their location (Kelly et al., 2023). AI is beginning to emerge on social media as we are in a moment of transaction. The latter gradually infiltrates our regular social media habits. Understanding how the public views AI is essential for product creation (Nader et al., 2022). This has led to many assessments of general awareness about AI adoption (Saud et al., 2020), using news and social media analysis to gauge public opinion (Zajko, 2022).
Social awareness has an impact on (H1a) AI and (H1b) attitudes.
In addition, the topic of knowledge exchange encompasses a variety of specialties. Psychology, epistemology, and cognitive science all fall under the knowledge-sharing umbrella. Collaboration, knowledge sharing, creation, use, and repurposing are all parts of knowledge sharing (Olan et al., 2022). It is used to improve performance, increase innovation, and expand our knowledge, both from an individual and organizational standpoint. At the heart of AI and information sharing lies the concept of knowledge. Machines can learn using AI that can learn, process, and apply knowledge to accomplish tasks and unlock the information they can share with humans to help them make better decisions (Li, 2013). Knowledge sharing is an extension of AI. AI allows us to extend, use, and generate information in ways we can only dream of currently because of the ability to share and use existing knowledge. Cognitive computing results from the link between knowledge sharing and AI (Fikri and Zaibon, 2004). Human thoughts processes are simulated using computer models. In the future, applications incorporating AI and knowledge sharing will be driven by cognitive computing (Sanzogni et al., 2017).
The new Ipsos survey from the World Economic Forum indicated that 60% of the world’s population expects AI-powered products and services to significantly impact their daily lives over the next 3 to 5 years. However, only half of those polled believe that AI products and services will make their lives easier (Sanzogni et al., 2017). Only half of those polled said they have the same trust in AI firms as in other companies. According to Nakamura (2014), to trust AI, people must know and comprehend exactly what AI is, what it does, and its impact. As this technology is implemented, leaders and organizations must prioritize transparency and trustworthiness in AI (Jallow et al., 2020).
Knowledge sharing has an impact on (H2a) AI and (H2b) attitudes.
Despite its widespread unfamiliarity, AI represents a technology that revolutionizes all aspects of life. It serves as a versatile tool, reshaping how information is integrated, data is analyzed, and resulting insights are utilized to enhance decision-making processes. Through this comprehensive review, our aim is to demystify AI for a diverse audience including policymakers, opinion leaders, and interested observers, while also highlighting its current impact on the world and the critical challenges it poses for society, the economy, and governance (Horowitz et al., 2018). Although algorithms and artificial intelligence are closely related, the main difference lies in AI’s ability to learn and adapt (Alhussain et al., 2020). According to Bdoor and Habes (2024) Traditional algorithms execute tasks with fixed rules, while AI uses advanced algorithms to learn from data and continually improve. AI are designed to make judgments, often using real-time data, setting them apart from passive machines with predefined responses (Fan and Gauch, 2019). Using sensors, digital data, and remote input, these algorithms aggregate information from various sources, quickly assess the data, and take actions based on the insights derived. With advances in storage systems, computing speeds, and analytical techniques, AI can conduct sophisticated analyses and making informed decisions. Therefore, as argued by Stothart et al. (2020), AI is not a futuristic concept but a present reality, already integrated and utilized across diverse industries and smart cities. Numerous examples illustrate how AI is significantly impacting the world and improving human capabilities.
Information gathering has an impact on (H3a) AI and (H3b) attitudes.
Because of movies like The Terminator, Avengers: Age of Ultron, and I, AI has become part of popular culture. However, what if the term AI could be used to describe the process of making a film? The artistic mind of AI has also been witnessed in the world of art, where it has already proven its potential in the music sector by making wonderful compositions (Horowitz and Kahn, 2021). The debut of AI in entertainment is changing the way people watch movies, television shows, sports and video games. According to research, the entertainment industry’s specialists may see AI as the next industrial revolution. According to media experts, AI will increase power by automating various monotonous tasks and providing a counterbalance to human dominance (Horowitz et al., 2018; Anantrasirichai and Bull, 2022). Using AI automation, entertainers can focus more of their time on developing their skills and delivering engaging content. By analyzing important data, AI will help industrial businesses make better judgments regarding advertising and marketing (Vasiljeva et al., 2021). For example, influencing streaming music and video services like Netflix and Spotify have succeeded by catering to different demographics, diversity tastes and preferences (Goel and Batra, 2017). They use AI algorithms to analyze individual user performance and demographics to encourage users’ interest in seeing or listening to close and keep them engaged (Chatterjee and Bhattacharjee, 2020). As a result, these AI platforms provide consumers with material tailored to their specific interests, enhancing their overall experience. Furthermore, Netflix has introduced the idea of an AI-powered application that would deliver more accurate video suggestions (Belanche et al., 2019). Additionally, there is a platform that focuses on establishing user-to-digital content synergy ‘We call it IRIS for short’. This is exactly what the television does. Based on the user’s choices or choices they may have made, the computer adjusts the content to their preferences, as mentioned by Sindermann et al. (2021).
H4: AI has an impact on attitudes.
Although AI and its related technologies are poised to disrupt media markets (e.g., social TV), the role of automated content generation is expected to remain relatively limited for the foreseeable future (Goel and Batra, 2017; Jain et al., 2022). However, AI will continue to have a vital impact on the demand side of media, particularly in terms of matching content to consumer needs. Future advances in AI have the potential to revolutionize this process, resulting in both positive and negative outcomes (Verganti et al., 2020). One of the most apparent benefits of AI will be its ability to facilitate easier access to desired STV content for consumers. Its search challenge encompasses not only traditional search technologies, but also recommendations, reviews, and other tools that help consumers in navigating content (Jain et al., 2022). On the surface, search appears to be an ideal application for AI, particularly in domains with clearly defined decision issues, measurable objectives, and ample prior case data (Anantrasirichai and Bull, 2022). The process of selecting content to meet consumer immediate needs is inherently satisfying, while metrics such as clicks and viewing time provide tangible measures of success. Additionally, the vast amount of data generated by online interactions provides ample fodder for AI algorithms (Horowitz and Kahn, 2021).
Artificial intelligence (AI) has significantly transformed the way subscription video on demand (social TV) services select and configure their content (Castellón, 2021). According to Habes et al. (2021), this process focuses on personalizing the user experience, optimizing the content catalog and predicting future trends. The ability to predict what content will be popular or what a user will like is another critical aspect of AI in social TV. Predictive analytics uses advanced techniques to predict viewing trends and future behaviors based on real-time and historical data. The study “A recommendation engine for predicting movie ratings using a big data approach” by Awan et al. (2021) explores how predictive analytics and matrix analysis can predict ratings and recommend movies based on user reviews Similarly recurrent neural networks (RNNs) are particularly effective at capturing temporal patterns in visualization data. The work “Recurrent Neural Networks for Collaborative Filtering” by Bernhardsson (2014). Discusses how RNNs can be used to improve recommendations based on sequences of user interactions with content. Netflix, for example, uses AI to personalize not only recommendations, but also the thumbnails and descriptions shown to users, optimizing the presentation of content to maximize engagement (Ahn et al., 2022). As well as social TV platforms use AI algorithms to personalize the notifications and emails they send to users, based on their viewing habits and preferences. Thus The IA brings a crucial papal in the selection and configuration of the content and the social TV services (Somandepalli et al., 2021). These recommendations and predictive analyzes have the optimization of the catalog and the personalization of the user experience, the IA permits the social TV platforms to provide a more intuitive experience, attractive and efficient (Goni and Tabassum, 2020).
The strides made by AI in addressing search and recommendation challenges have been remarkable. Machine learning techniques, as demonstrated in initiatives such as the Netflix Challenge, have shown promise in predicting future user preferences based on past behavior. Major tech players such as Google, Amazon, and Facebook heavily rely on AI technologies to power their search engines (Goel and Batra, 2017; Zajko, 2022), product recommendations, and news feeds, showcasing the widespread adoption and effectiveness of AI in this domain.
H5: AI has an impact on the selection of social media content.
AI is not a futuristic concept; rather, it is a reality that is currently being incorporated and used across various industries. This includes media and communication, users’ online consumption. There are countless instances in which AI is already significantly influencing the world and augmenting human capabilities (Loureiro et al., 2021). Unlike sectors (e.g., manufacturing and transportation), where a large number of jobs involve repetitive tasks that can be easily automated with existing technologies, the primary value in the media industry and consumption lies in creating intricate content that relies heavily on skills such as judgment, interpretation, creativity, and communication. These are areas where humans excel and are expected to outperform algorithms for the foreseeable future (Blum and Langley, 2018). An example of people’s positive attitudes toward AI is the social media applications WhatsApp and Telegram, as they allow users to express their views on a wide range of issues freely. Analyzing conversations between persons of various ages, geographic locations, and sociodemographic groups could reveal interesting things about the people involved (Stothart et al., 2020) There are also many interests that constitute the public’s social telephony, including attention economic The fusion of the attention economy with social television offers significant opportunities to increase engagement and monetization of media content (Bhargava and Velasquez, 2021). By leveraging social interactions and real-time feedback, content creators can not only capture but also retain viewers’ attention more effectively. However, it is crucial to address challenges related to content saturation, privacy, and authenticity of engagement to maximize the benefits of this convergence (Dawson, 2014). A case study further affirmed students’ attitudes towards accepting and employing AI in media content selection. They found positive attitudes from students towards accepting and using AI for media content selection. Additionally, respondents indicated their trust in the role of AI to facilitate them with less effort and greater results (Alhammad et al., 2021).
H6: Attitudes have an impact on the selection of social TV content.
A literature assessment of existing studies finds that while more individuals support AI development than oppose it, it is mostly men with a high degree of education and income who hold these views. According to self-awareness for both genders, women are less knowledgeable about AI than men. Moreover, most AI research and development is conducted by men (Project, 2021; Mansoori et al., 2023). In Germany, only 16 percent of those employed in the AI sector are women. In the U.S., that number is just under 25%. Thus, not only are outdated stereotypes the basis for AI development decisions, but they are also embedded into AI’s data foundation. Female voices are less reliable for voice and speech recognition systems, as are female faces for face recognition systems. In addition, preliminary research indicates that elements such as comprehension and trust significantly influence the successful use of AI, but gender disparities remain unknown (Reilly, 2019; Lütz, 2022). The use of AI in technology adoption and use, in general, is on the rise, particularly in the distribution and presentation of visual content. For example, social media firms face difficulties in creating large amounts of high-quality material, so they turn to AI to help them (Reilly, 2019; Haleem et al., 2022).
In addition, studies have indicated that there are gender differences in the use of social media by young students. Males use social media mainly for pleasure, whereas women use it for conversation and information (Mansoori et al., 2023). There is a significant gap between the social media usage of men and women. Men are solely responsible for establishing new relationships, whereas women are more likely to use social networks for intellectual or informative purposes (Reyes et al., 2022). Similarly, the use of social networks was found to have exploded with the development of technology. Furthermore, the amount of time spent on social networking sites has increased by 62.5% since 2012 and continues to rise. During the previous year, adolescents spent an estimated 2.6 h daily on social media (Anantrasirichai and Bull, 2022).
Gender mediates the relationship between (H7a) AI and (H7b) attitudes towards selecting social TV content (see Figure 1).
This research involves enrolled students from several universities, using two randomly selected public universities in Irbid, Jordan (Yarmouk University and University of Science and Technology). From active users who have an active subscription to one of the major subscription video on demand (SVOD) services, such as Netflix, Amazon Prime Video, Disney, or Hulu, were included. Furthermore, a sample of 340 participants from the Mass Communication Departments participated in this research. However, the shortlist of the questionnaires indicated a total of n = 294 questionnaires, since n = 6 were missing or incorrectly filled, indicating a response rate of 88%. For the questionnaire, which was based on a five-point Likert scale directly administered by one of the authors. Data were collected from May to June 2022 and further manipulated and encoded for data analysis using structural equation modelling (SEM) for the analysis using IBM Amos.
Respondents were informed to withdraw from this study anytime they wanted without further obligations. Furthermore, informed consent was used before respondents took place (Roache, 2014), we provided all information and details about the study objectives, problem, and potential outcomes to the respondents.
To analyze the data, the researchers followed a two-phase process; consisting of measurement model analysis and structural model analysis. Initially, they conducted a convergent validity analysis to assess the internal consistency among the study participants. This involved evaluating internal consistency through a convergent validity analysis of the measurement model. Table 1 presents the calculated factor loading, the extracted average variance, the Cronbach alpha, and the composite reliability values. Analysis indicated that most factor loading values1 exceeded the threshold of 0.5, and that all values extracted from the mean variance surpassed the designated threshold of 0.5 (Alarcón and Sánchez, 2015). Additionally, Cronbach’s alpha values ranged from 0.730 to 0.836, and composite reliability values ranged from 0.728 to 0.836, all exceeding the designated threshold of 70%. Therefore, it is inferred that the convergent validity of the measurement model is confirmed.
Once the convergent validity was confirmed, the study proceeded to assess the discriminant validity based on two criteria proposed by Tadesse et al. (2018) (Table 2). First, the results of the Fornel-Larcker criterion showed that the squared values of all average variance extracted exceeded the correlation values. Additionally, for the Heterotrait-Monotrait ratio, the study manually computed the HTMT correlation formula. The findings indicated an overall HTMT calculation of 0.537, significantly below the recommended threshold of 0.9, ensuring the fulfillment of discriminant validity requirements for the measurements (Zaiţ and Bertea, 2011).
The goodness-of-fit was run to measure our model (Hair et al., 2019). It helps to determine the extent to which the obtained values fit the gathered data (Rönkkö and Cho, 2020). Therefore, the analysis revealed the chi-square value x2 = 0.322 (18), p = 0.000. Additionally, the not-fit index value remained at 0.436 and the standardized root mean square value (RMSEA) at 0.26, which is smaller than the threshold value of 0.85. Therefore, it is well observed, seeing that the measurement model is suitable for the evaluation of the structural model (Figure 2).
After assessing the internal validity of the measurement model, the study proceeded with SEM analysis, in order to evaluate the strength of the relationships between the study variables. Initially, it conducted an analysis of the coefficients of determination R2, as suggested by Zumbo (2005). As presented in Table 3, the R2 values (0.538–0.732) indicate a robust predictive power of the exogenous variables in the study.
As outlined by Suhr (2016), path analysis plays a crucial role in exploring relationships among observed variables, allowing simultaneous examination of both direct and indirect relationships. Consequently, this study used path analysis to investigate the structural relationships between latent variables (Table 4 and Figure 3). Specifically, the first hypotheses (H1a, H1b) assumed the influence of social awareness on both AI and user attitudes. The analysis revealed that these proposed impacts remained highly significant (β = 0.473 and 3.97, p > 0.000, respectively). Similarly, the second hypotheses (H2a, H2b) posited the impact of knowledge sharing on AI and attitudes. Once again, the results demonstrated that these proposed relationships remained statistically significant (β = 3.93 and 3.94, p > 0.000, respectively).
Hypotheses H3a and H3b proposed that information gathering would have an impact on AI and attitudes. These relationships also retained significance, (β = 3.87 and 3.85, p > 0.000) respectively. Furthermore, H4 suggested that AI would influence the process of selecting social TV content. The results indicated a notable impact of AI on the selection of social TV content selection (β = 3.95, p > 0.000). Furthermore, the impact of AI on user attitudes also remained significant, with a coefficient of β = 3.94, p > 0.000. H6 proposed that attitudes would affect the selection of social TV content. The findings showed that this proposed impact remained significant (β = 3.95, p > 0.000).
Regarding H7a and H7b, which investigated mediation analysis suggesting a significant indirect influence of gender on AI, attitudes, and selection of social TV content, the study explored the mediating effect of gender on AI leading to the selection of relevant social TV content. In particular, mediation analysis was conducted using the Sobel test, as discussed by Gu et al. (2019). The findings indicated that the mediating effect remained significant (β = 3.91, p > 0.000). Similarly, the mediating effect of gender on attitudes leading to the selection of social TV content remained significant, (β = 3.93, p > 0.000). The indirect effects values in both hypotheses were 0.859 and 0.696, respectively.
Social television, as a union of different features such as communication, information, education and entertainment, provides the ability to connect and share experiences even with other users on virtual social networks (Song et al., 2019; TC Lin, 2019). Another major facility is the availability of smartphones and devices that enable users to connect easily even if users and audiences have geographical and cultural boundaries (Loureiro et al., 2021). As noted by Ritsma (2006) earlier, we were unfamiliar with many cultures, languages, and traditions. However, users recognize, like and acknowledge them, indicating the value of overall technology, especially social TV. In addition, incorporating AI also improved the technology and its services, but equipping it with characteristics such as content personalization and content discovery. In addition, media professionals, technology providers, and owners of social TV-based platforms also recognized its importance as a part of their audience-centered approach.
Structural equation models (SEM) indicated that exposure to socially conscious content significantly increased social awareness among users (p < 0.05). Add to Users’ social awareness influenced their viewing behavior, with more socially aware users consuming more content of this type, which fed back to the AI algorithms to improve future recommendations. The AI successfully recommended educational content that promoted knowledge sharing (p < 0.01).as well as SEM analyzes showed an increase in knowledge sharing activity after exposure to educational recommendations.
Similarly, this research examined the selection of social TV content from the perspective and opinion of potential Jordanian young users. In particular, users are the innovators (Ismail, 2006; Zhang et al., 2015; Dearing and Cox, 2018) and tend to accept and adopt a technology comparatively fast, indicating similar attitudinal patterns towards social TV acceptance among the youth. The study involved a self-reported conceptual model linking different features to AI leading to social TV content selection. The proposed model also indicated the role of gender in mediating AI, attitudes, and social TV content selection. The study suggested the impact of social awareness on AI and the attitudes of the respondents. The proposed relationship was in-line with the arguments (Olan et al., 2022), stating that AI is accompanied by Social Awareness capability leading to positive attitudes. In other words, AI is capable of informing the users about social phenomena, including trending issues and topics in the entertainment industry. Findings remained consistent with the assumption made by Olan et al. (2022). Additionally, the impact of knowledge sharing using AI was found on the attitudes of the respondents. This assumption was supported by Jallow et al. (2020), proposing that knowledge and experience sharing are linked with AI, leading to positive attitudes towards the acceptance process. The findings also remained validated, indicating that Jordanian youth understand the use of AI in content creation.
Likewise, AI has a vital impact on the social media content selection process. This assumption was supported by Sindermann et al. (2021), who proposed AI as a factor in the content recommendation and selection process. The proposed relationship between AI and attitudes also remained significant, as it remained consistent with early studies (Jayanthiladevi et al., 2020; Malik, 2020), assuming AI-enabled entertainment and communication positively affect user attitudes in multiple ways. Furthermore, user attitudes towards social TV content selection were also proposed as witnessed by the existing literature (Blum and Langley, 2018; Stothart et al., 2020). The results affirmed the relevant impact, providing and adding more evidence to the existing literature. Finally, the study proposed a possible gender mediation in two distinct ways. First, it is important to understand that gender has a great influence on acceptance and usage in general (Reilly, 2019). This mediation can also be understood in the context of AI and users’ attitudes (Lütz, 2022). Therefore, the study found that gender mediation in AI adoption for the selection of social TV content. The mediating role of gender in attitudes leading to the selection of social TV content also remained significant. According to the respondents, the overall role of additional factors in the use of AI also affects users’ attitudes towards the selection of social TV content. Gender, as one of the prominent demographical factors, also mediates this content selection, as witnessed by Project (2021).
The fusion of various features of social TV, including communication, information, education, and entertainment, offers a platform for connecting and sharing experiences between users across geographical and cultural boundaries. With the widespread availability of smartphones and devices, users can easily engage with Social TV content, fostering recognition, appreciation, and acceptance of diverse cultures and traditions. In addition, the integration of AI enhances social TV technology, particularly through features such as content personalization and discovery. Media professionals, technology providers, and owners of social TV platforms recognize the importance of AI in catering to audience preferences and improving user experiences. This research delved into the selection of social TV content from the perspective of potential Jordanian young users, revealing their desire to adopt technology and their role as innovators. The proposed conceptual model linking AI to social TV content selection highlighted the mediating role of gender, with AI influencing social awareness and knowledge sharing, thus shaping user attitudes towards content selection. The study affirmed the significant impact of AI on the social TV content selection process, consistent with the existing literature on the role of AI in content recommendation and the attitudes of users towards AI-enabled entertainment and communication. Furthermore, the mediating influence of gender on technology acceptance and user attitudes underscores the importance of considering demographic factors in understanding user engagement with social TV content. Overall, the findings contribute to our understanding of the complex interaction between AI, gender, attitudes, and social TV content selection, providing valuable insights for stakeholders in the media industry and inform future research in this domain. This study makes a significant contribution by providing an in-depth and contextualized analysis of how AI and algorithms are transforming the media consumption experience in Jordan. The findings not only offer practical benefits for social TV platforms and content developers, but also enrich academic understanding of the interplay between technology, culture and consumer behavior in the Jordanian context. In doing so, the study sets a precedent for future research and technological applications in the region, and potentially, in similar contexts in other parts of the world.
The practical implications stemming from this conclusion are manifold. Firstly, the fusion of social TV features presents a unique opportunity for fostering cross-cultural connections and sharing experiences among users globally. This highlights the potential for social TV platforms to serve as vehicles for promoting cultural appreciation and understanding.
Furthermore, the integration of AI technology holds immense potential for enhancing social TV experiences through features such as content personalization and discovery. Recognizing the pivotal role of AI in catering to audience preferences, media professionals, technology providers, and platform owners must prioritize the integration of AI-driven solutions to improve user experiences and engagement.
Regarding the social implications, the facilitation of cross-cultural connections and sharing of experiences through social TV platforms can contribute to the promotion of global harmony and mutual understanding. By fostering interactions between individuals from diverse backgrounds, social TV has the potential to bridge cultural divides and promote empathy and tolerance.
Moreover, the accessibility of social TV content via smartphones and devices democratize access to information and entertainment, potentially narrowing the digital divide and empowering marginalized communities. This can lead to increased social inclusion and participation in cultural discourse, fostering a more equitable and interconnected society.
The integration of AI technology in social TV not only enhances user experiences but also raises important ethical considerations regarding data privacy, algorithmic bias, and the automation of content curation. It is imperative for stakeholders to address these concerns transparently and ethically to ensure that AI-driven social TV platforms contribute positively to societal well-being.
Several limitations should be acknowledged in this research. First, the study focused solely on young Jordanian users, limiting the generalizability of the findings to other demographic groups or cultural contexts. Future research could explore a more diverse sample to ensure a broader representation and understanding of social TV usage patterns. Second, the research methodology should be considered. Longitudinal studies could offer a deeper understanding of how attitudes towards AI and social TV content selection evolve over time, allowing the exploration of causal relationships and trends. Third, self-reported data was collected, which can be subject to bias, suggesting a more comprehensive understanding of the behavior and attitudes of users towards social TV. Although the study identified gender as a mediating factor in AI adoption and attitudes towards social TV content selection, the mechanisms underlying this relationship remain underexplored. Future research could consider gender-specific drivers and barriers influencing technology acceptance and usage patterns. Scholars can advance our understanding of the complex interplay between AI, social television, and user engagement, ultimately forming the development of more personalized, inclusive, and ethically sound media technologies Although the sample is limited to two universities, these institutions can host a diverse student population in terms of geographical, socioeconomic and cultural origin, which can make their students representative of the general young Jordanian population. The selected universities and their partial representativeness of Jordan’s youth population partially justify the results, but future research should include a wider variety of contexts and demographic groups to ensure the general applicability of the conclusions.
The raw data supporting the conclusions of this article will be made available by the authors, without undue reservation.
The studies involving humans were approved by Ethics Committee Research, Yarmouk University. The studies were conducted in accordance with the local legislation and institutional requirements. The participants provided their written informed consent to participate in this study.
MH: Formal analysis, Investigation, Methodology, Software, Supervision, Validation, Writing – original draft, Writing – review & editing. AA: Investigation, Resources, Software, Writing – original draft. ME: Conceptualization, Validation, Writing – original draft, Writing – review & editing. RA: Conceptualization, Resources, Software, Validation, Writing – original draft.
The author(s) declare that financial support was received for the research, authorship, and/or publication of this article. This work was supported by Princess Nourah Bint Abdulrahman University Researchers Supporting Project number (PNURSP2024R 343), Princess Nourah Bint Abdulrahman University, Riyadh, Saudi Arabia.
The authors declare that the research was conducted in the absence of any commercial or financial relationships that could be construed as a potential conflict of interest.
All claims expressed in this article are solely those of the authors and do not necessarily represent those of their affiliated organizations, or those of the publisher, the editors and the reviewers. Any product that may be evaluated in this article, or claim that may be made by its manufacturer, is not guaranteed or endorsed by the publisher.
1. ^factor loading values: Factor loading values are fundamental in factor analysis, providing key information about the relationships between observed variables and latent factors.Square value: The term “square value” simply refer to the squared value of a number, a perfect square in the context of integers, or variance in the context of descriptive statistics. The exact interpretation will depend on the specific context in which the term is used.
Ahn, J., Kim, J., and Sung, Y. (2022). The effect of gender stereotypes on artificial intelligence recommendations. J. Bus. Res. 141, 50–59. doi: 10.1016/j.jbusres.2021.12.007
Alarcón, D., and Sánchez, J. A. (2015). “Assessing convergent and discriminant validity in the ADHD-R IV rating scale: user-written commands for average variance extracted (AVE), composite reliability (CR), and Heterotrait-Monotrait ratio of correlations (HTMT)” in Spanish STATA Meeting, 1–39.
Alhammad, K. L., Habes, M., Al Olaimat, F., and Haddad, I. (2021). Attitudes of students of the Faculty of Mass Communication at Yarmouk University towards using video platforms in distance education. Rev. Int. Geograph.l Educ. Online 11, 1041–1052. doi: 10.48047/rigeo.11.05.99
Alhussain, T., Al-Rahmi, W. M., and Othman, M. S. (2020). Students’ perceptions of social networks platforms use in higher education: a qualitative research. Int. J. Adv. Trends Comput. Sci. Eng 9, 2589–2603. doi: 10.30534/ijatcse/2020/16932020
Anantrasirichai, N., and Bull, D. (2022). Artificial intelligence in the creative industries: a review, vol. 55. Netherlands: Springer, 589–656.
Awan, M. J., Khan, R. A., Nobanee, H., Yasin, A., Anwar, S. M., Naseem, U., et al. (2021). A recommendation engine for predicting movie ratings using a big data approach. Electronics 10:1215. doi: 10.3390/electronics10101215
Barker, C. (2018). Facebook, Twitter, and the pivot to original content: from social TV to TV on social. Transformative works and cultures. ed. M. McNutt . 26. doi: 10.3983/twc.2018.1291
Bdoor, S. Y., and Habes, M. (2024). “Use chat GPT in media content production digital newsrooms perspective” in Artificial intelligence in education: The power and dangers of ChatGPT in the classroom. eds. A. Al-Marzouqi, S. A. Salloum, M. Al-Saidat, A. Aburayya, and B. Gupta, Studies in Big Data, (Springer: Cham), 144, 545–561.
Belanche, D., Casaló, L. V., and Flavián, C. (2019). Artificial intelligence in FinTech: understanding robo-advisors adoption among customers. Ind. Manag. Data Syst. 119, 1411–1430. doi: 10.1108/IMDS-08-2018-0368
Bernhardsson, E. (2014) Recurrent neural networks for collaborative filtering Available at: https://erikbern.com/2014/06/28/recurrent-neural-networks-for-collaborative-filtering.html
Bhargava, V. R., and Velasquez, M. (2021). Ethics of the attention economy: the problem of social media addiction. Bus. Ethics Q. 31, 321–359. doi: 10.1017/beq.2020.32
Blum, A. L., and Langley, P. (2018). Artificial intelligence selection of relevant features and examples in machine. Artif. Intell. 97, 245–271. doi: 10.1016/S0004-3702(97)00063-5
Cant, M. C., Nel, P., Wiid, J. A., and Hebblethwaite, D. (2017). Empirical results of the awareness of social media and its application by academics in teaching in New Zealand. J. Bus. Retail Manag. Res. 12, 1–12. doi: 10.24052/JBRMR/V12IS01/EROTAOSMAIABAITINZ
Castellón, L. B. (2021). Audiovisual verification in the evolution of television newsrooms: Al Jazeera and the transition from satellite to the cloud. Analisi 64, 85–102. doi: 10.5565/rev/analisi.3414
Chatterjee, S., and Bhattacharjee, K. K. (2020). Adoption of artificial intelligence in higher education: a quantitative analysis using structural equation modelling. Educ. Inform. Technol. 25, 3443–3463. doi: 10.1007/s10639-020-10159-7
Choi, E. Y. (2022). The mediating role of interaction between watching motivation and flow of sports broadcasting in Multi-Channel network. SAGE Open 12:215824402110685. doi: 10.1177/21582440211068513
Dawson, M. (2014). Rationalizing television in the USA: neoliberalism, the attention economy and the digital video recorder. Screen 55, 221–237. doi: 10.1093/screen/hju011
Dearing, J. W., and Cox, J. G. (2018). Diffusion of innovations theory, principles, and practice. Health Aff. 37, 183–190. doi: 10.1377/hlthaff.2017.1104
Fan, Y, and Gauch, S (2019) Adaptive agents for information gathering from multiple, distributed information sources. 1999 AAAI Symposium on Intelligent Agents in Cyberspace: Stanford University. 21–59.
Fikri, S, and Zaibon, S (2004) Artificial intelligence support for knowledge management in construction.. Knowledge Management International Conference and Exhibition 2004 (KMICE 2004). Univeriti Utara Malaysia.
Goel, D., and Batra, D. (2017). Predicting user preference for movies using NetFlix database. Carniege Mellon University: Department of Electrical and Computer Engineering. 156–163.
Goni, A., and Tabassum, M. (2020). Artificial intelligence (AI) in journalism: is Bangladesh ready for it? A study on journalism students in Bangladesh. Athens J. Mass Media Commun. 6, 209–228. doi: 10.30958/ajmmc.6-4-1
Gu, D., Guo, J., Liang, C., Lu, W., Zhao, S., Liu, B., et al. (2019). Social media-based health management systems and sustained health engagement: TPB perspective. Int. J. Environ. Res. Public Health 16, 1–15. doi: 10.3390/ijerph16091495
Guo, M. (2019). Social television viewing with second screen platforms: antecedents and consequences. Media Commun. 7, 139–152. doi: 10.17645/mac.v7i1.1745
Habes, M., Alanani, A., Youssef, E., and Sharif, H. (2024). “Why do Jordanian students prefer using ChatGPT a case study of higher education institutions”, Artificial intelligence in education: The power and dangers of ChatGPT in the classroom. eds. A. Al-Marzouqi, S. A. Salloum, M. Al-Saidat, A. Aburayya, and B. Gupta, (Springer: Cham), 127–141.
Habes, M., Ali, S., Elareshi, M., Tahat, K.M., and Ziani, A. (2021). Understanding users’ social TV content using artificial intelligence approach: a survey. International Conference of modern trends in ICT industry: towards the excellence in the ICT Industries, MTICTI 2021. ed. S. Yemen . 1–7. doi: 10.1109/MTICTI53925.2021.9664762Hair
Hair, J. F., Risher, J. J., Sarstedt, M., and Ringle, C. M. (2019). When to use and how to report the results of PLS-SEM. Eur. Bus. Rev. 31, 2–24. doi: 10.1108/EBR-11-2018-0203
Haleem, A., Javaid, M., Asim Qadri, M., Pratap Singh, R., and Suman, R. (2022). Artificial intelligence (AI) applications for marketing: a literature-based study. Int. J. Intell. Netw. 3, 119–132. doi: 10.1016/j.ijin.2022.08.005
Harboe, G. (2010). In search of social television. Social computing: concepts, methodogies, tools, and applicaitons. ed. S. Dasgupta . IGI Global. 719–731. doi: 10.4018/978-1-60566-984-7.ch046Horowitz
Horowitz, M. C., Allen, G. C., Saravalle, E., Cho, A., Frederick, K., and Scharre, P. (2018). Artificial intelligence and international Security, 1–28. doi: 10.1371/journal.pone.0257732
Horowitz, M. C., and Kahn, L. (2021). What influences attitudes about artificial intelligence adoption: evidence from U.S. local officials. PLoS One 16, 1–20.
Ismail, S. (2006). Detailes review of Roger’s diffusion of innovations theory and educational technology. Turk. Online J. Educ. Technol. 5, 14–23.
Jain, M., Mohan, R., Sachi, S., Nigam, A., and Shrivastava, R. (2022). A transformative impact on media markets based on media and artificial intelligence. NeuroQuantology 20, 7570–7576. doi: 10.14704/nq.2022.20.10.NQ55745
Jallow, H., Renukappa, S., and Suresh, S. (2020) Knowledge management and artificial intelligence (AI). Proceedings of the European Conference on Knowledge Management, ECKM 2020-Decem(Eckm): 363–369.
Jayanthiladevi, A., Raj, A. G., Narmadha, R., Chandran, S., Shaju, S., and Krishna Prasad, K. (2020). AI in video analysis, production and streaming delivery. J. Phys. Conf. Ser. 1712:012014. doi: 10.1088/1742-6596/1712/1/012014
Kelly, S., Kaye, S. A., and Oviedo-Trespalacios, O. (2023). What factors contribute to the acceptance of artificial intelligence? A systematic review. Telematics Inform. 77:101925. doi: 10.1016/j.tele.2022.101925
Kerres, M., and Buntins, K. (2020). Recommender in AI-enhanced learning: an assessment from the perspective of instructional design. Open Educ. Stud. 2, 101–111. doi: 10.1515/edu-2020-0119
Kim, J., Merrill, K., and Collins, C. (2020). Touchdown together: social TV viewing and social presence in a physical co-viewing context. Soc. Sci. J. 2020, 1–15. doi: 10.1080/03623319.2020.1833149
Langley, P. (2019) An integrative framework for artificial intelligence education. 33rd AAAI Conference on Artificial Intelligence, AAAI 2019, 31st Innovative Applications of Artificial Intelligence Conference, IAAI 2019 and the 9th AAAI Symposium on Educational Advances in Artificial Intelligence EAAI 33: 9670–9677.
Li, D. (2013). IFIP advances in information and communication technology: preface. IFIP Adv. Inform. Commun. Technol. 392:1. doi: 10.1007/978-3-642-36124-1
TC Lin, T. (2019). Multiscreen social TV system: a mixed method understanding of users’ attitudes and adoption intention. Int. J. Hum. Comput. Interac. 35, 99–108. doi: 10.1080/10447318.2018.1436115
Loureiro, S. M. C., Guerreiro, J., and Tussyadiah, I. (2021). Artificial intelligence in business: state of the art and future research agenda. J. Bus. Res. 129, 911–926. doi: 10.1016/j.jbusres.2020.11.001
Lütz, F. (2022). Gender equality and artificial intelligence in Europe. Addressing direct and indirect impacts of algorithms on gender-based discrimination. ERA Forum 23, 33–52. doi: 10.1007/s12027-022-00709-6
Malik, S. (2020). Artificial intelligence for social media safety and SECURITY. Int. Eng. J. Res. Dev. 5, 1–8.
Mansoori, A., Tahat, K., Tahat, D., Habes, M., Salloum, S. A., Mesbah, H., et al. (2023). Gender as a moderating variable in online misinformation acceptance during COVID-19. Heliyon 9:e19425. doi: 10.1016/j.heliyon.2023.e19425
Nader, K., Toprac, P., Scott, S., and Baker, S. (2022). Public understanding of artificial intelligence through entertainment media. AI Soc., 1–14. doi: 10.1007/s00146-022-01427-w
Odunaiya, O., Agoyi, M., and Osemeahon, O. S. (2020). Social TV engagement for increasing and sustaining social TV viewers. Sustainability 12:4906. doi: 10.3390/su12124906
Olan, F., Arakpogun, E. O., Suklan, J., Nakpodia, F., Damij, N., and Jayawickrama, U. (2022). Artificial intelligence and knowledge sharing: contributing factors to organizational performance. J. Bus. Res. 145, 605–615. doi: 10.1016/j.jbusres.2022.03.008
Project (2021). Sex and gender Bias in artificial intelligence and health: Building a future for equality, 16–19.
Reilly, D. H. (2019) Sex differences in cognitive abilities and educational outcomes: examining the contribution of sex-role identification. Gold Coast, Australia: Unpublished Doctoral Thesis, School of Applied Psychology, Griffith University.
Reyes, M. E. S., Morales, B. C. C., Javier, G. E., Ng, R. A. E., and Zsila, Á. (2022). Social networking use across gender: its association with social connectedness and happiness amidst the COVID - 19 pandemic. J. Technol. Behav. Sci. 7, 396–405. doi: 10.1007/s41347-022-00262-6
Roache, R. (2014). Why is informed consent important? J. Med. Ethics 40, 435–436. doi: 10.1136/medethics-2014-102264
Rönkkö, M., and Cho, E. (2020). An updated guideline for assessing discriminant validity. Organ. Res. Methods 25, 6–14. doi: 10.1177/1094428120968614
Sanzogni, L., Guzman, G., and Busch, P. (2017). Artificial intelligence and knowledge management: questioning the tacit dimension. Prometheus 35, 37–56. doi: 10.1080/08109028.2017.1364547
Saud, M., Mashud, M., and Ida, R. (2020). Usage of social media during the pandemic: seeking support and awareness about COVID-19 through social media platforms. J. Public Aff. 20:e2417. doi: 10.1002/pa.2417
Sindermann, C., Sha, P., Zhou, M., Wernicke, J., Schmitt, H. S., Li, M., et al. (2021). Assessing the attitude towards artificial intelligence: introduction of a short measure in German, Chinese, and English language. KI - Kunstliche Intelligenz 35, 109–118. doi: 10.1007/s13218-020-00689-0
Somandepalli, K., Guha, T., Martinez, V. R., Kumar, N., Adam, H., and Narayanan, S. (2021). Computational media intelligence: human-centered machine analysis of media. Proc. IEEE 109, 891–910. doi: 10.1109/JPROC.2020.3047978
Song, H., Kim, J., and Choi, Y. (2019). Social TV viewing: the effect of virtual co-viewers and the role of social presence. J. Digit. Contents Soc. 20, 1543–1552. doi: 10.9728/dcs.2019.20.8.1543
Stothart, C. R., Burland, B. R., Strickland, H. C., Messina, F. D., Couch, D. S., and From, J. D. (2020) Can artificial intelligence systems improve information-gathering efficiency in Army Mission command processes. United States Army Research Institute for the Behavioral and Social Sciences.
Suhr, D. (2016) The basics of structural equation modeling. Irvine, CA: SAS User Group of the Western Region of the United States (WUSS). 1–19.
Tadesse, T., Gillies, R. M., and Campbell, C. (2018). Testing models and measurement invariance of the learning gains scale. Education Sciences 8:192. doi: 10.3390/educsci8040192
Tahat, K., Tahat, D. N., Masoori, A., Habes, M., Alghazo, E., and Ketbi, N. (2023). Role of social Media in Changing the social life patterns of youth at UAE. Artificial intelligence (AI) and finance. eds. B. A. M. Alareeni and I. Elgedawy. (Springer: Cham), 152–163.
Vasiljeva, T., Kreituss, I., and Lulle, I. (2021). Artificial intelligence: the attitude of the public and representatives of various industries. J. Risk Finan. Manag. 14:339. doi: 10.3390/jrfm14080339
Verganti, R., Vendraminelli, L., and Iansiti, M. (2020). Innovation and design in the age of artificial intelligence. Pro. J. Inn Manage. 37, 212–227. doi: 10.1111/jpim.12523
Ye, X., Zhao, B., Nguyen, T. H., and Wang, S. (2020). Social media and social awareness. Manual Digit. Earth 1, 425–440. doi: 10.1007/978-981-32-9915-3_12
Zaiţ, A., and Bertea, P. E. (2011). Methods for testing discriminant validity. Manag. Market. J 9, 217–224.
Zajko, M. (2022). Artificial intelligence, algorithms, and social inequality: sociological contributions to contemporary debates. Sociol. Compass 16, 1–16. doi: 10.1111/soc4.12962
Zawacki-Richter, O., Marín, V. I., Bond, M., and Gouverneur, F. (2019). Systematic review of research on artificial intelligence applications in higher education – where are the educators? Int. J. Educ. Technol. High. Educ. 16, 1–27. doi: 10.1186/s41239-019-0171-0
Zhang, X., Yu, P., Yan, J., and Ton AM Spil, I. (2015). Using diffusion of innovation theory to understand the factors impacting patient acceptance and use of consumer e-health innovations: a case study in a primary care clinic healthcare needs and demand. BMC Health Serv. Res. 15, 1–15. doi: 10.1186/s12913-015-0726-2
Keywords: social TV, AI, Jordan, attitudes, content selection, social media platforms, gender, survey
Citation: Habes M, Alhazmi AH, Elareshi M and Attar RW (2024) Understanding the relationship between AI and gender on social TV content selection. Front. Commun. 9:1410995. doi: 10.3389/fcomm.2024.1410995
Received: 02 April 2024; Accepted: 10 June 2024;
Published: 26 June 2024.
Edited by:
Simón Peña-Fernández, University of the Basque Country, SpainReviewed by:
Javier Odriozola-Chéné, University of A Coruña, SpainCopyright © 2024 Habes, Alhazmi, Elareshi and Attar. This is an open-access article distributed under the terms of the Creative Commons Attribution License (CC BY). The use, distribution or reproduction in other forums is permitted, provided the original author(s) and the copyright owner(s) are credited and that the original publication in this journal is cited, in accordance with accepted academic practice. No use, distribution or reproduction is permitted which does not comply with these terms.
*Correspondence: Mokhtar Elareshi, bWVsYXJlc2hpQHNoYXJqYWguYWMuYWU=
Disclaimer: All claims expressed in this article are solely those of the authors and do not necessarily represent those of their affiliated organizations, or those of the publisher, the editors and the reviewers. Any product that may be evaluated in this article or claim that may be made by its manufacturer is not guaranteed or endorsed by the publisher.
Research integrity at Frontiers
Learn more about the work of our research integrity team to safeguard the quality of each article we publish.