- School of Media and Communication, Shanghai Jiao Tong University, Shanghai, China
The widespread use of the Internet has transformed communication, including listening to music and watching movies. With the development of new technologies, consumers now have more options for viewing new media across many platforms and devices. Consumers now have more options for watching new material across many platforms and devices via OTT (Over-the-Top) streaming platforms. Future trends in consumer behavior will significantly increase competition for the digital media business, particularly in the digital video and music sectors. This article aims to understand better Thai streaming service users, where the country’s digital infrastructure has been developing. By examining their heavy viewing addiction, intention to continue using the expectation confirmation model, personality traits, and implementation of the 7Ps in marketing. An online questionnaire-based survey of Thai respondents was used to evaluate the proposed models empirically. The study used path analysis and a Structural Equation Model (SEM) to investigate how continuance intention toward streaming services is affected by other endogenous variables in Thailand. The empirical results found that the user’s satisfaction and addiction of users to heavy viewing were found to be key factors influencing the intention to continue using streaming services in the Thai environment. Likewise, the study confirms the importance of the Big Five personality traits theory in impacting the behavior of streaming service consumers. Introverted persons may perceive streaming services as more useful and enjoyable. The findings deepened our understanding of Thai streaming consumer behavior and offered more insight into market trends for streaming services.
1 Introduction
The advance of technology prevalence has altered creators’ behavior and strategies in every industry, even in the streaming industry. Consumers can now access video content via a variety of devices, including PCs, smartphones, tablets, gaming consoles, television sets, and other Internet-connected equipment, anytime and anywhere, thanks to the quick spread of broadband technologies and ongoing innovations in the media and information and communication technologies (ICT) industries (Federal Communication Commission, 2012). The most recent and disruptive innovation in the media sector, Over-The-Top (OTT), is an example of this trend, with internet-based video services growing proliferating in many countries. With the growth of digital transformation, OTT is seen as the next generation of media that will bring efficiency and innovation. Digital transformation is a continuous process by which businesses use their digital competencies to develop new business models, products, and services in response to market and customer disruptions (International Data Corporation, 2015; Lee and Cho, 2021).
The growing prevalence of OTT has resulted in an increasing number of users, including in Thailand, where the infrastructure was historically weak. The National Broadcasting and Telecommunications Commission (The National Broadcasting and Telecommunications Commission, 2022) examined the increasing number of subscriptions to streaming services in Thailand, especially subscription-based services, such as subscription video-on-demand (SVoD). The results found that there is exponential growth. It grew by more than 230.52% during 2015–2016, increasing from 0.24 million to 0.80 million accounts. Users’ subscriptions increased to 8.99 million accounts in 2022. The subscriber is expected to reach 10.92 million accounts at the end of 2023. Over the past several years, more than 26 million Thai users, or nearly one in three, have watched content through the streaming platform OTT (Over-the-Top) or video on demand (VoD), where viewers can access content such as movies and television programs through the Internet network. Watching this content online takes about one to three hours daily, and 92% of Thai users use more than one OTT platform. There are more than 20 video streaming platforms in Thailand, for example, Netflix, Iflix, Prime Video, Apple TV, HBO GO, iQiyi, Viu, and Line TV (Electronic Transactions Development Agency, 2022).
With the growth of new technology, users have an alternative way of consuming new media on different devices and platforms. In the future, the digital media market will face an entirely new number of competitors, and customer behavior will change, especially in the digital video and music markets. Maintaining customers and creating a business strategy for different regions is challenging for the digital media market. To ensure those who are relevant in the media industry survive long-term, marketers and producers must engage in strategies aimed at attracting new users, maintaining the loyalty of current users, and promoting the continued use of their services.
From past studies, there has been a shift in the study of continuance intention to use new technology in the various fields and pointed out a crucial marketing area related to new technology, for instance, information systems (e.g., Franque et al., 2020), smartphone banking services (e.g., Susanto et al., 2016), information technology (e.g., Lin and Bhattacherjee, 2008), social mobile apps (e.g., Akdim et al., 2022), and e-learning (e.g., Roca et al., 2006). Similarly, there are previous studies concerning the intention to continue streaming services in many countries, for example, the United States (Yousaf et al., 2021), Spain (Martínez-Sánchez et al., 2021), Indonesia (Kusumawati et al., 2021), India (Nagaraj et al., 2021), South Korea (Shin and Park, 2021), Portugal (Barata and Coelho, 2021), Malaysia (Suki, 2011), Vietnam (Tran et al., 2019) and Thailand (Pal and Triyason, 2018).
However, this previous research has contributions and applications that apply mainly in its specific country’s context. Many researchers suggested that future research should extend more ideas and more extensive models backed up by theories to explore a broader perspective regarding marketing and economic analysis with robustness techniques such as structural equation modeling (SEM) in different countries areas (Nagaraj et al., 2021; Martínez-Sánchez et al., 2021; Pereira and Tam, 2021).
Based on the research problems and gaps identified above, this study makes several significant contributions to the literature on the streaming services market. First, this study expands on existing adoption models by incorporating the Expectation Confirmation Model (ECM) to develop a new research model that better explains the continuance intention of users to engage with streaming services. In this study, we adopt the Expectation-Confirmation Theory (ECT), which posits that when users’ expectations are met or exceeded, their satisfaction increases, leading to a higher likelihood of continuance intention. This approach goes beyond initial adoption to consider the factors influencing long-term engagement. It is crucial to understand how users form habits around media consumption and what drives their sustained usage is crucial. Second, the study examines why users continue using streaming services and explores strategies that streaming platform providers can implement to enhance user experience and increase subscriber retention. This analysis is vital for service providers seeking to differentiate themselves in a competitive market and retain loyal customers through improved user satisfaction and engagement tactics. Third, this research introduces the Big Five personality traits into the context of technology adoption and media consumption, a relatively underexplored area. By investigating how personality traits such as openness, conscientiousness, extraversion, agreeableness, and neuroticism influence continuance intention, the study offers a more nuanced understanding of individual differences in technology use behavior. Fourth, the study addresses the impact of addiction to heavy viewing and the marketing mix on user retention. It highlights how these factors can be leveraged to enhance user engagement and maintain subscription levels, particularly in a rapidly evolving digital landscape. Finally, this study provides a culturally specific analysis by focusing on the Thai context, identifying positive and negative personality traits related to the continuance of streaming services. This cultural perspective adds depth to our understanding of consumer behavior in diverse markets and offers actionable insights for international streaming service providers looking to expand in similar contexts.
2 Literature review
2.1 Overview of OTT
Over-the-top, often known as OTT, is a service that allows transmitting audio, video, and audiovisual content over the Internet. This type of service does not need service providers to invest in or operate the signal networks themselves. Due to the expansion of the Internet infrastructure and access in Thailand, OTT services were introduced to the nation in 2014 and were utilized for the first time that year. In 2017, the National Broadcasting and Telecommunications Commission (NBTC), Thailand’s media regulator, regulated OTT as a subscription television service. This regulation compelled all OTT platforms to register with the authorities to continue functioning legally in Thailand (Ananthos, 2018).
It is common for OTT operators in Thailand to generate money in various ways, depending on their business strategies and areas of specialization. In addition to these, Subscription Video on Demand (SVoD) is the first option. SVoD is comparable to regular television packages, enabling consumers to access unlimited material for a fixed monthly cost. Users are not bound to a long-term contract when they subscribe to SVoD, which means they have much flexibility when opting out. HOOQ, Hollywood HDTV, Primetime, Monomax, Doonee, and iFlix were among the biggest OTT providers in Thailand that provided SVoD (Ananthos, 2018).
Regarding the distribution of demand in Thailand, according to the most recent information obtained from YouGov Profiles, the most popular video streaming service in Thailand currently is Netflix, located in the United States. More than one in three customers (35%) are members of Netflix. Over 21% of users are enrolled in TrueID, making it the second most popular OTT service. TrueID is the most subscribed local OTT provider. More than one in ten users (13%) have indicated they are members of Disney+ Hotstar, making it the third most popular video streaming platform. The other places it ahead of Chinese streaming providers Viu (11%), WeTV (11%), iQIYI (10%), and AIS Play (7%), which is the second most popular local streaming platform. Under the same circumstances, less than 20% of Thai customers are enrolled in Apple TV+ (3%) or Amazon Prime Video (4%). Netflix (88%) and WeTV (81%) have achieved the most significant levels of customer satisfaction. More than four out of five users have identified themselves as pleased consumers. There are at least three out of every four members who are satisfied with iQIYI (78%), Amazon Prime Video (78%), Disney+ Hotstar (76%), and Viu (75%). These platforms have the following most significant satisfaction percentages after iQIYI. Last but not least, a comparatively smaller percentage of subscribers who have Apple TV+ (74%), TrueID (71%), and AIS play (69%) indicate that they are pleased consumers. This percentage is nevertheless extremely acceptable (YouGov, 2023).
2.2 Expectation confirmation model (ECM)
This study focuses on the continuance intention of Thai users regarding streaming service platforms. To better understand post-acceptance behavior, the Expectation Confirmation Model (ECM) has been widely employed in various contexts, including digital services and information systems (Albashrawi and Motiwalla, 2017; Bhattacherjee, 2001; Jumaan et al., 2020; Lee and Cho, 2021; Lee, 2010; Lestari and Soesanto, 2020; Lin et al., 2005). The ECM is derived from the Expectation Confirmation Theory (ECT), which is traditionally used in marketing to measure customer satisfaction and post-purchase behavior (Halilovic and Cicic, 2013). ECT posits that customers’ repurchase intentions are influenced by their satisfaction, which is determined by the extent to which their initial expectations are confirmed.
According to the ECM, an individual’s intention to continue using information technology (IT) is determined by three key factors: the user’s satisfaction with the IT, the degree to which the user’s expectations are confirmed, and perceived usefulness (Lee, 2010) as shown in Figure 1. In streaming services, confirmation refers to users’ experiences with the service exceeding their initial expectations. At the same time, satisfaction involves users feeling they made the correct decision in choosing the service and being generally content with its performance.
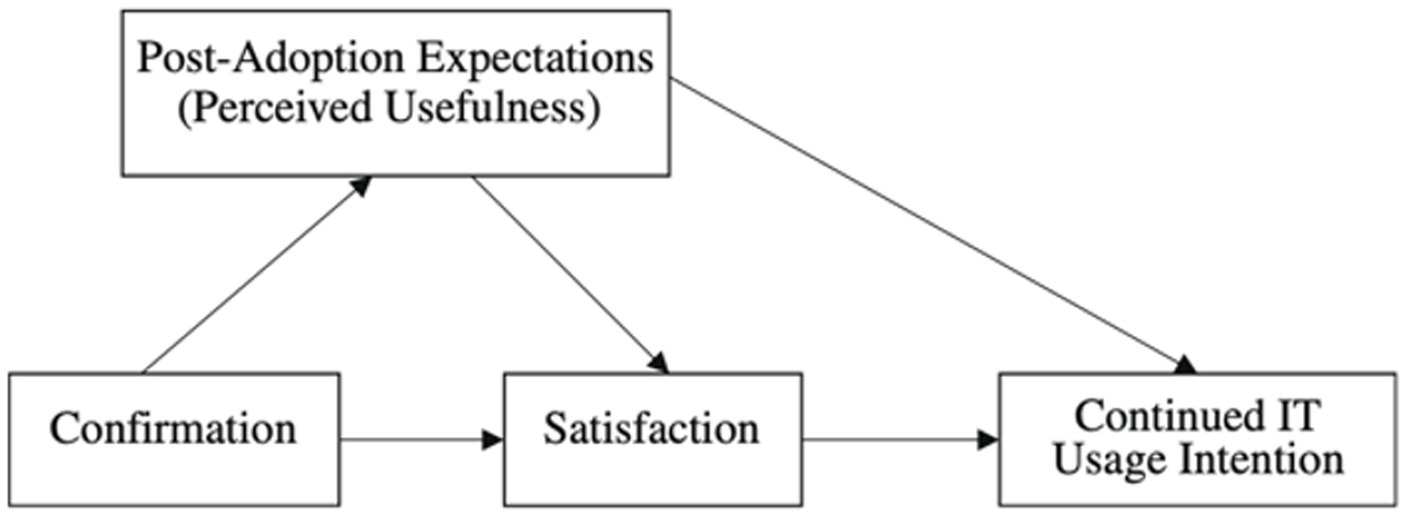
Figure 1. The Expectation confirmation (ECM) by Lee (2010).
Previous studies have demonstrated that confirmation positively influences perceived usefulness, as users are more likely to perceive a service as applicable when their expectations are met or exceeded (Davis, 1989; Zhou, 2017; Alraimi et al., 2015; Bhattacherjee, 2001; Ogbanufe and Gerhart, 2017). For example, Venkatesh et al. (2011) found that confirmation of user expectations in online learning platforms led to higher perceived usefulness, increased user satisfaction, and continued usage intentions. This relationship is particularly pertinent in streaming services, where users have varied expectations for content quality, usability, and value. Therefore, we hypothesize:
H1: Confirmation of expectation (CF) positively affects perceived usefulness (PU) associated with the use of streaming services.
Perceived enjoyment, or the intrinsic pleasure derived from using a service, has also been shown to be a crucial determinant of continued usage intentions in digital contexts (Oghuma et al., 2015). Studies have indicated that when users’ expectations are confirmed, they are more likely to find the service enjoyable, which enhances their overall satisfaction (Oliver, 1980; Churchill and Surprenant, 1982; Oghuma et al., 2015). For instance, in the context of mobile internet services, Kim et al. (2016) found that confirmation significantly influenced perceived enjoyment, a strong predictor of continued use. Applying this to streaming services, where enjoyment is a primary motivator for use, we propose:
H2: Confirmation of expectation (CF) positively affects perceived enjoyment (PE) associated with the use of streaming services
The direct relationship between confirmation and satisfaction is well-documented in the literature (Bhattacherjee, 2001; Oghuma et al., 2015). Satisfaction is enhanced when the service meets or exceeds user expectations, which leads to positive evaluations of the decision to continue using the service. Zhou (2017) found that confirmation significantly predicted user satisfaction in social networking sites, which further influenced continued use intentions. This relationship is expected to hold in streaming services, where user satisfaction is critical for retention. Hence, we hypothesize:
H3: Confirmation of expectation (CF) positively affects the user’s satisfaction (SAT) associated with the use of streaming services
Perceived usefulness, the extent to which a service is considered beneficial, has been identified as a critical antecedent of user satisfaction in various digital contexts (Lee, 2010; Lestari and Soesanto, 2020). Users who perceive a service as applicable are more likely to be satisfied with their experience and continue using it. Studies in online banking (Lin et al., 2005) and mobile services (Kim et al., 2016) have shown that perceived usefulness significantly impacts user satisfaction and continued usage intentions. For streaming services, where the value provided by the service (e.g., quality content, ease of use) directly impacts user satisfaction, we propose:
H4: Perceived usefulness (PU) positively affects the user’s satisfaction (SAT) associated with the use of streaming services
Perceived enjoyment is defined as the extent to which the activity of using a particular service is perceived to be enjoyable, independent of any performance outcomes that may be anticipated (Davis et al., 1992). It is a form of intrinsic motivation that refers to the pleasure or emotional value derived from using a technology or service, such as a streaming platform. In streaming services, perceived enjoyment encompasses the fun, pleasure, and excitement users experience when consuming content. This concept is crucial for understanding user engagement because it directly impacts user satisfaction and continuance intention (Venkatesh and Davis, 2000; Alalwan et al., 2018).
The theoretical foundation for including perceived enjoyment in this study is grounded in the Expectation Confirmation Model (ECM) and related technology adoption and continuance theories. The ECM suggests that users’ continuance intention is influenced by their level of satisfaction, which in turn is affected by the perceived usefulness and enjoyment of the service (Bhattacherjee, 2001). Enjoyment is often considered a critical determinant of user satisfaction in digital and interactive media contexts, as it provides intrinsic value that complements utilitarian benefits (Van der Heijden, 2004).
Research has shown that perceived enjoyment is a significant predictor of user satisfaction and continuance intention across various digital contexts, including social networking sites (Lin and Lu, 2011), online learning platforms (Roca et al., 2006), and mobile internet services (Kim et al., 2016). For instance, Praveena and Thomas (2014) demonstrated that perceived enjoyment was a primary determinant of perceived value and behavioral intentions among users in the United States. Their findings suggest that the more users enjoy an activity, the more likely they will continue engaging. Similarly, in a study by Alalwan et al. (2018), perceived enjoyment significantly influenced user satisfaction, affecting the continuance intention to use information technology (IT) services.
Perceived enjoyment is particularly relevant in streaming services because these platforms are primarily used for entertainment, where enjoyment is a crucial driver of user engagement and satisfaction (Hsu and Lin, 2016). When users find the content engaging and pleasurable, it enhances their overall experience, leading to higher satisfaction and a stronger intention to continue using the service. Moreover, Dickinger et al. (2008) found that perceived enjoyment and social norms significantly foster continued usage intentions in IT and IS services.
Given the importance of perceived enjoyment in digital media consumption, its inclusion in this study is justified. It allows for a more comprehensive understanding of the factors influencing user satisfaction and continuance intention in streaming services. The hypothesis is formulated as follows:
H5: Perceived enjoyment (PE) positively affects the user’s satisfaction (SAT) associated with the use of streaming services.
2.3 Continued intention to use
In consumer behavior and technology adoption, continuance intention is a key factor determining a service’s long-term success. Drawing from the Expectation Confirmation Model (ECM) and previous research, this study explores how perceived usefulness (PU), perceived enjoyment (PE), and user satisfaction (SAT) influence users’ continuance intention to use streaming services.
Several studies have demonstrated the importance of perceived usefulness in shaping continuance intentions in various digital contexts. For example, in e-learning and mobile services, users who perceive a service as applicable are likelier to continue using it (Venkatesh and Bala, 2008). In the context of streaming services, usefulness might relate to the platform’s ability to provide diverse content that meets users’ entertainment needs. Studies like Nagaraj et al. (2021) and Shin and Park (2021) have shown similar trends in streaming services, suggesting that users’ perceptions of a service’s utility strongly influence their decision to remain subscribed. Based on these findings, we hypothesize:
H7: Perceived usefulness (PU) positively affects the continuance intention (CI) associated with the use of streaming services
Similarly, perceived enjoyment has been highlighted as a crucial factor influencing continuance intention, especially in entertainment and digital content contexts. Previous research indicates that enjoyment derived from using a service not only enhances user satisfaction but also increases the likelihood of continued use (Praveena and Thomas, 2014; Pereira and Tam, 2021). Within streaming platforms, the enjoyment of content (e.g., movies, music) is a primary driver of user engagement and retention. The enjoyment factor significantly predicts continued use in studies examining digital media consumption behaviors (Dickinger et al., 2008; Lin & Lu, 2011). Extending these findings to our study’s context, we propose:
H8: Perceived enjoyment (PE) positively affects the continuance intention (CI) associated with the use of streaming services
User satisfaction remains a pivotal determinant of continuance intention across many service industries, including digital and streaming services. Satisfied users are likelier to have a favorable attitude toward service, resulting in higher loyalty and continued usage (Bhattacherjee, 2001; Oliver, 1980). Previous studies, such as Oghuma et al. (2015) and Shin and Park (2021), have confirmed that satisfaction derived from a positive user experience directly impacts the intention to continue using a service. In the context of this study, we hypothesize:
H9: The user’s satisfaction (SAT) positively affects the continuance intention (CI) associated with the use of streaming services
2.4 Addiction to heavy viewing (ADD)
Addiction, in the context of streaming services, can be described as problematic usage where an individual cannot control the amount of time spent engaging in online activities, particularly viewing, which are not directly related to their occupational duties. This inability to regulate viewing behavior is characterized by psychological and behavioral changes, withdrawal symptoms, and avoidance of social and familial responsibilities, potentially leading to long-term negative effects (American Psychiatric Association, 2000).
Research has identified several symptoms associated with this type of addiction, leading to both positive motivations for users (e.g., entertainment, escapism) and negative consequences. These adverse effects include brain disorders (Li and Chung, 2006), compulsive behaviors (Young and Abreu, 2011), social and family withdrawal (Kuss and Lopez-Fernandez, 2016), and excessive or “heavy” viewing habits (Kesici and Tunc, 2018). For instance, individuals who engage in heavy viewing—defined as watching four or more hours per day—may exhibit addiction-like behaviors as this level of engagement can interfere with real-life relationships and other daily activities (Engberg et al., 2019; Horvath, 2004; Young and Abreu, 2011).
The relevance of addiction to heavy viewing is particularly significant in the context of online streaming services. These platforms often provide highly engaging content designed to capture user attention and encourage prolonged usage, which can lead to heavy viewing habits. Several studies have found that streaming services can prolong viewing behavior regardless of potential negative outcomes due to the captivating nature of their content (Li and Chung, 2006; Young and Abreu, 2011). This is particularly evident when the content is mood-stimulating, such as in the case of highly engaging genres like pornographic films, violent videos with sexual content, fantasy genres, and gaming videos. Such content can intensify viewing habits, leading to a substantial risk of addiction and affecting users’ inclination to continue using such services (Kesici and Tunc, 2018).
For this study, addiction to heavy viewing is defined as the tendency to use streaming services for longer than initially intended, prioritizing viewing over other activities, spending an excessive amount of time on streaming content, having streaming services consume almost all leisure time, and experiencing irritation when viewing is interrupted (Horvath, 2004). These dimensions capture the extent of addictive behaviors and are crucial for understanding the continuance intention in the context of streaming platforms.
Given the significant impact of addictive behaviors on user engagement and continuance intention, we hypothesize the following:
H10: The addiction to heavy viewing (ADD) positively affects the continuance intention (CI) associated with the use of streaming services
2.5 Marketing mix (MM)
The Marketing Mix, originally conceptualized by McCarthy (1964) as the 4 Ps (Product, Price, Place, Promotion), has been widely recognized as a foundational framework for developing marketing strategies. This model was later expanded to include three additional elements—People, Process, and Physical Evidence—forming the 7 Ps of the marketing mix, which provides a more comprehensive approach to managing and strategizing in service-oriented businesses (Booms and Bitner, 1981). The marketing mix is widely utilized in traditional business settings and digital and online environments, where companies must adapt their strategies to accommodate unique challenges and opportunities (Lim et al., 2021; Farid et al., 2023).
The marketing mix elements are critical for developing a business strategy that aligns with consumer needs and preferences, and numerous studies have highlighted their importance in various contexts, including online retailing and digital services (Constantinides, 2006; Chaffey and Ellis-Chadwick, 2019). Specifically, in the realm of streaming services, each marketing mix component plays a crucial role in shaping user experiences and influencing their decisions to continue using a platform.
2.5.1 Product
In the context of streaming services, ‘product’ refers to the variety and quality of content offered and the platform’s brand reputation among Thai users. Well-known brands like Netflix, Disney+, WeTV, and Viu are recognized for their diverse and high-quality content libraries, which cater to various user preferences.
2.5.2 Price
‘Price’ pertains to the perceived value-for-money streaming services offer, including subscription and membership fees. Offering competitive pricing or good value for money is crucial in retaining customers, particularly in markets where multiple streaming platforms vie for user attention.
2.5.3 Place
This element refers to the distribution channels available for content consumption. Streaming services include the convenience of accessing content through multiple devices, such as smartphones, tablets, and smart TVs. A broad range of viewing options enhances user satisfaction by providing flexibility and convenience.
2.5.4 Promotion
‘Promotion’ involves marketing efforts to attract and retain users, such as discounts, free trials, special promotions, and partnerships with influencers and celebrities. Effective promotional strategies can increase user engagement and subscription rates.
2.5.5 People
In streaming services, ‘people’ refers to customer service quality. Providing fast and effective customer support can significantly enhance user satisfaction and loyalty.
2.5.6 Process
This component includes the ease and convenience of the subscription process, user interface design, and the efficiency of content delivery. A seamless and user-friendly process can enhance user satisfaction by reducing friction points in the user experience.
2.5.7 Physical evidence
This refers to the tangible elements that support the user experience, such as a visually appealing platform design, intuitive navigation, and accessible customer support.
This study employs the marketing mix as a comprehensive framework to understand its impact on user satisfaction and continuance intention in the context of streaming services in Thailand. The rationale for including the marketing mix in this research is that a well-crafted marketing strategy, encompassing all 7 Ps, can significantly influence users’ perceptions and behaviors, leading to higher satisfaction levels and increased likelihood of continued usage.
Several studies have demonstrated the importance of the marketing mix in enhancing customer satisfaction and driving continuance intention. For example, Lin et al. (2005) found that a well-structured marketing mix positively influences customer satisfaction and loyalty in the digital service industry. Similarly, Kusumawati et al. (2021) showed that digital marketing strategies based on the marketing mix significantly impact customer retention and engagement in online platforms.
Based on the comprehensive understanding of the marketing mix and its relevance to streaming services, the following hypotheses are proposed:
H6: The decision to use streaming services based on the 7 Ps Marketing Mix positively affects the user’s satisfaction (SAT) associated with the use of streaming services.
H11: The decision to use streaming services based on the 7 Ps Marketing Mix (MM) positively affects the continuance intention (CI) associated with the use of streaming services.
2.6 The big five personality traits (FFM)
The Five-Factor Model (FFM), also known as the Big Five or OCEAN model, has been extensively employed in psychological and management research to predict various cognitions, attitudes, and behaviors (Goldberg, 1990; Costa and McCrae, 1992). The FFM’s relevance in the context of technology acceptance and use is underscored by its ability to provide a nuanced understanding of how individual differences shape user interactions with information systems (Devaraj et al., 2008; Agarwal and Prasad, 1999).
Understanding personality traits is crucial in the context of streaming services because these traits can significantly influence how users perceive the usefulness and enjoyment of the service, which in turn affects their continuance intention. For example, users high in openness might seek new and diverse content, while those high in neuroticism might use streaming services for emotional regulation (Zmud, 1979; Bhattacherjee, 2001). As such, integrating the FFM into this study provides a robust framework for exploring how intrinsic personality factors affect user satisfaction and continuance behavior.
The FFM posits five broad dimensions of personality—Openness to Experience, Conscientiousness, Extraversion, Agreeableness, and Neuroticism—that have been linked to various behavioral tendencies and preferences (Barrick and Mount, 1991; Feist, 1998). Research has shown that these traits can influence users’ technology adoption and continued usage behavior:
2.6.1 Openness to experience (OP)
Individuals high in openness are characterized by curiosity, creativity, and a preference for novelty and variety. In technology contexts, users high in openness may be more inclined to explore and engage with new features or content offered by streaming services. However, this trait could also lead to dissatisfaction if the service fails to provide the expected diversity or innovation (Devaraj et al., 2008).
2.6.2 Conscientiousness (CO)
Conscientious individuals are typically diligent, disciplined, and goal-oriented. Prior studies suggest that conscientious users might value the practical utility and efficiency of a streaming service (e.g., ease of use, reliable access to desired content) and may perceive high usefulness in platforms that offer structured content or learning-oriented material (Gallivan et al., 2005).
2.6.3 Extraversion (EX)
Extraverts are characterized by sociability, assertiveness, and a preference for interaction. In the context of streaming services, extroverts might prefer content that allows for shared experiences or social interactions (such as watch parties). However, they may not find solitary or passive activities like binge-watching enjoyable, leading to a potentially negative perception of usefulness or enjoyment if the service lacks social features (Huang et al., 2007; DeYoung et al., 2007; Correa et al., 2010).
2.6.4 Agreeableness (AG)
Agreeable individuals are cooperative, friendly, and compassionate. They may be more inclined to engage with content that promotes social harmony or aligns with their values (e.g., family-friendly content). If the streaming service caters to these preferences, this trait might positively influence perceived enjoyment and usefulness (McCrae and Costa, 1997; Huang et al., 2007).
2.6.5 Neuroticism (NE)
Neuroticism is associated with emotional instability, anxiety, and mood swings. In technology usage, neurotic individuals may use streaming services as a means of escapism or emotional comfort. This could result in a higher perceived usefulness and enjoyment if the service effectively meets their emotional needs. Conversely, high neuroticism might also lead to overuse or problematic use patterns (Gallivan et al., 2005; Butt and Phillips, 2008).
Based on the FFM and its relevance to user behavior in streaming services, the following hypotheses have been developed:
H12: Openness’s characteristic (OP) negatively affects perceived usefulness (PU) associated with the use of streaming services
H13: Neuroticism’s characteristic (NE) positively affects perceived usefulness (PU) associated with the use of streaming services
H14: Agreeableness’s characteristic (AG) positively affects perceived usefulness (PU) associated with the use of streaming services
H15: Conscientiousness’s characteristic (CO) positively affects perceived usefulness (PU) associated with the use of streaming services
H16: Extraversion’s characteristic (EX) negatively affects perceived usefulness (PU) associated with the use of streaming services
H17: Openness’s (OP) characteristic negatively affects perceived enjoyment (PE) associated with the use of streaming services
H18: Neuroticism’s characteristic (NE) positively affects perceived enjoyment (PE) associated with the use of streaming services
H19: Agreeableness’s characteristic (AG) positively affects perceived enjoyment (PE) associated with the use of streaming services
H20: Conscientiousness’s characteristic (CO) positively affects perceived enjoyment (PE) associated with the use of streaming services
H21: Extraversion’s characteristic (EX) negatively affects perceived enjoyment (PE) associated with the use of streaming services
3 Materials and methods
This quantitative research focuses on Thai users’ streaming behavior and continued usage of these platforms within the Thai environment. After doing conceptual, theoretical, and model assessments, it is essential to have a methodological framework to understand the methodologies used in each approach and the results they yield. This section details the study’s subject area, the methodology utilized for data collection, the chosen degree of analysis, and the statistical strategy employed for data analysis.
3.1 Sampling procedure
A nonprobability sampling method was employed to choose the sample, increasing the likelihood of obtaining biased conclusions. Participants were recruited using two different sampling methods. The first population was a representative sample of the general public, selected using a purposive sampling technique to get highly accurate information about streaming users and fans. Utilizing a purposive sample allows the researcher to gather information from the group of interest efficiently (Wrench et al., 2008). Purposive sampling allowed us to target individuals who were active users of music streaming services directly. Networking sampling was then used to expand the sample by leveraging participants’ connections to recruit others. This combination helped balance targeted selection with broader representation.
The researcher collected data from various social media networks. Participants were instructed to complete the online questionnaires by scanning the QR code or clicking the HTML link provided. These questionnaires were available on various Facebook groups, such as Disney+ Hotstar Thailand, We TV Thailand, Spotify Thailand, and Netflix Thailand Community, targeting fans of streaming services, movies, and music. The second group was recruited using an immediate network sampling procedure, whereby the researcher’s friends and coworkers now using streaming services were included. In this study, the researcher established a link between the user’s social media networks, including Facebook, Instagram, Line, and WeChat, and their friends and coworkers.
To access the streaming viewers ‘fans, all participants are Thai men and women residing in Thailand. The target audience for this study is older than 15, has some Internet experience, and has used streaming services before. Through purposive and network sampling, only individuals who had previously utilized streaming services for at least 1 month and had an average service use for at least 1 week were recruited. Pre-screening was designed to find active online streamers in Thailand who have been using the service for at least a month. By filtering the questionnaires, the process was expected to provide an average service usage of at least once per week.
In order to determine the sample size, a confidence interval was employed to compute the minimum required sample size. The confidence levels guarantee that the findings may be generalized to the entire population and are not only due to random chance. As a general guideline, it is recommended to use a larger sample size. The confidence level that is most frequently used in research is 95%. This indicates that the sample results should correctly predict the population results with a 95% degree of confidence. Thus, a rule of thumb is used to determine whether the sample size is suitable. Tabachnick and Fidell (1996) proposed that a widely acknowledged recommendation for factor analysis is to have a minimum of 300 cases.
Hence, the minimum number of participants for this study is 400 instances. For an unknown population of significant size, a sample size of approximately 400 to 500 or more would be ideal to limit the potential margin of error (Cochran, 1963; Comrey and Lee, 1992). The study utilized an online questionnaire survey with a sample size of 603 participants. Of these, 557 respondents were considered legitimate and reported using streaming services (n = 557). The other 37 respondents were excluded from the analysis due to their lack of usage of streaming services and their limited experience of using them for less than 1 month. Nine respondents expressed their disagreement with the terms required to complete the questionnaire.
3.2 Questionnaire design
The questionnaire consists of three screening questions, which inquire about the use of music streaming services. Additionally, it includes 10 questions regarding the behavior of using music streaming services, 33 questions related to perceptions toward streaming services, 23 questions about personality traits, and six questions regarding basic demographics. The survey was intended to be free and allow for open-ended responses. The survey consisted of open-ended and close-ended questions to analyze the participants’ reactions to a series of questions. We employed a five-point Likert scale in both section three and section four. The participants are given assertions and instructed to rate their agreement or disagreement using a pre-established scale. This scale spans from Strong Agreement (point 5) to Strong Disagreement (point 1), and is used to evaluate all items on the scale.
The measurement items used in this study are based on constructs derived from previous research (see 1Supplementary Appendix A). The questionnaire was structured into five main sections: screening questions, usage and opinion behavior, perspective on streaming services, personality attributes, and demographics. A total of 50 respondents consented to take part in the pilot study. Feedback from the pilot participants was used to refine and improve the questionnaire before administering it to the entire sample. To assess the reliability of questions within each concept, we initially verified the consistency and dependability of the collected data. In order to conduct a reliability study, we employed a Cronbach’s Alpha reliability test, which social scientists widely utilize (Cronbach, 1951).
The target audience of this study comprises Thai citizens who are proficient in the Thai language. Consequently, the questionnaire was translated from English to Thai and back-translated to English to assess its validity and reliability. In this study, we employed the back-translation method to improve the content. The process involved first having an expert translator translate the English text into Thai and then having another expert translator translate the Thai data back into English. The Thai-translated questionnaire was compared with the English version to confirm face validity, ensuring the meaning remained unchanged. Finally, the researcher sought the expertise of three Thai Ph.D. Professors employed at Thai government universities are fluent in both Thai and English. Their task was to thoroughly examine and validate the final copies of the translated questionnaire in Thai. Nevertheless, specific inquiries in the Thai language were revised to ensure accuracy, and the final Thai version was produced for implementation in the online survey as the next stage.
3.3 Data collection and data analysis
The questionnaires were distributed to the target group via Google Forms. Targeted networking techniques were sent out the questionnaire via several online platforms, including the Facebook streaming community, email, and chat messaging applications such as Facebook Messenger, Instagram, WeChat, and Line. The typical time needed for each target respondent to finish the questionnaire is 15–20 min. The users were required to respond to a total of 100 questions. Data was collected over approximately 30 days, specifically from October 1, 2023, to October 30, 2023. The collected replies were inputted into a Google spreadsheet and uploaded to a statistical tool for further analysis.
This study employed two data analysis software programs: SPSS 26.0 for data entry and Smart PLS 4.0 for analyzing the variables within the model (Ringle et al., 2022). Consequently, this study has three different parts of analysis. Initially, we utilized SPSS 26.0 to analyze the demographic and behavioral patterns related to streaming service consumption. The data was analyzed using descriptive analysis, which included measures such as Mean (M), Frequency, Standard Deviation (SD), and Percentage. Secondly, the PLS-SEM Algorithm was utilized to construct a measurement model for evaluating Cronbach’s Alpha, composite reliability (CR), convergent validity (AVE), and discriminant validity.
Additionally, factor loading was examined to assess the model’s fit. We picked the PLS-SEM Algorithm because one of the endogenous factors in our study is a formative variable. Specifically, the 7P’s marketing mix includes a component that has an eight-item formative measurement model.
Finally, the PLS-SEM bootstrapping method assessed the differences between the structural models and hypotheses. Specifically, 5,000 random subsamples or bootstrap samples were generated for testing. The statistical measures included in this case were factor loading, standard error, p-values, and t-values. The study included structural equation modeling (SEM) to investigate hypothesis testing and structural interpretation to assess route analysis and determine significance levels. This test included testing for direct, indirect, and total effects.
4 Results
In this part, we outlined five inquiries for the online survey to obtain respondents’ personal information. The variables included in the study were gender, age, education, occupation, and monthly income, as shown in Table 1. A total of 603 individuals completed an online survey. After excluding cases that did not meet the pre-screening criteria and excluding responses with conflicting answers, 557 participants were identified as current streaming users throughout the specified time frame. They had over a month of experience utilizing streaming services and used them at least once a week.
4.1 Demographic characteristics
The demographic characteristics of 557 respondents who used streaming services comprised 66.8% females, 32.7% males, and 0.5% others. Similarly, the average age of respondents from the total sample is around 15–64 years old; the age group of 15–24 was the most frequent users, 39.5%, and the second group of streaming users was aged between 25 and 34, 33.0%.
Most respondents held bachelor’s degrees, the highest education level at 50.8%. Streaming users’ second level of education was a diploma of 19.4%, while 15.8% had a high school degree. The majority of respondents were students, 35.0%. The second group consisted of freelancers or employees, roughly 18.1%. The third group was company employees; approximately 14.9 and 10.8% were business owners. Regarding monthly income, 38.4% reported their monthly personal income between 10,001 THB and 30,000 THB (280–838 USD); the second majority of 30.5% said their monthly personal income was under 10,000 THB (280 USD). The third group, 17.8%, reported their monthly personal income between 30,0001THB and 50,000 THB (838–1,395 USD).
4.2 Behavior usage of streaming users
The researcher asked the respondents about their streaming services usage behavior using seven close-ended questions, as shown in Table 2; example questions were how long they had been using streaming services, the frequency of listening and watching streaming platforms, and other behavioral consumption. Meanwhile, three multiple-choice questions were asked about the reason for subscription and not subscription to the VIP accounts and which platforms they recently used.
We pointed out the close-ends question to ask users to self-rate their experience of how long they had been using streaming services. In both groups of users, roughly 20.5% were using streaming services for more than one year to two years and more than one to three months. This point shows that the beginner group and those with more streaming experience for a while were the most samples in this research to represent the streaming users in Thailand.
Subsequently, 16.5% had experience using streaming services for more than three months to one year, 16.2% had used streaming services for more than three years to four years, followed by loyalty users of 16.0% had used streaming services for more than five years.
When it comes to watching or listening to media content via streaming service platforms per week, most users use streaming platforms: About 38.8% use them at least once every week, followed by 30.7% consuming them at least once weekly. Then, close to 30.5% use them at least once every two to five days.
By examination of the frequency of watching or listening to media content via streaming service platforms per day, medium users 33% were using streaming services for around one to two hours per day, followed by 31.6% using streaming services for roughly two to four hours per day.
There were three heavy-user groups, 15.4% consuming streaming content four to six hours a day. 7.4% consuming six to eight hours a day, and 2.7% consuming more than eight hours a day. However, light users, consuming streaming services for less than an hour daily, made up only 9.9% of the sample.
Then, we asked the participants whether they had a subscription plan or a VIP account to consume streaming services. Most 67% had a subscription or a VIP account. In contrast, the remaining 33% did not subscribe or did not have a VIP account but used a free or freemium service instead of paid services.
To review the users’ opinions about how likely they would pay for streaming content instead of using a free service, the majority of users, approximately 78.1%, feel likely to pay for streaming content instead of using a free service. Considering the details, roughly 42.9% were willing to pay for a long-term period, and 35.2% were willing to pay for a short-term period. However, light users, consuming streaming services for less than an hour daily, made up only 9.9% of the sample.
The average amount users were likely to spend on streaming services is around 100 THB-300 THB (2.79–8.87 USD) per month, as reported by 32.3%. Surprisingly, more respondents said they still do not intend to pay, yielding 24.4%. Nevertheless, the minority comprises 36 respondents (6.5%) likely to pay an average between 501 THB-701 THB (13.97–19.52 USD). Only 12.4% were likely to pay less than 100 THB (less than 2.79 USD) monthly. Overall, all respondents, 75.6%, are likely to pay to consume streaming content every month.
We ask double questions to ensure that users still want to use streaming services or not by asking whether they intend to continue to subscribe to streaming services. Most of the respondents, 82.4%, confirmed that they still want to subscribe to the streaming services, divided into two categories: 44.2% intended to subscribe for the short term, and 38.2% intended to subscribe for the long term. Nonetheless, about 17.6%% had no intent to subscribe to a streaming premium or VIP account.
Regarding consumer behavior, we surveyed which platforms they recently used in the past 3 months by asking the respondents to select multiple-choice. We found that YouTube was the most used streaming app at 78%, and Netflix was the second most used at 58.5%. The Global market streaming applications were popular in Thailand, consisting of 31.6% Apple TV and Disney+ Hotstar, 18.7%. Interestingly, the music streaming app Spotify was also popular among Thai users at 43.8%, while JOOX music had nearly 40.8%, followed by 31.6% for Apple music. The Asian streaming applications such as Viu, WeTV, and iQiyi, and Asian series such as Chinese, Korean, and Japanese were a hot trend for Thai viewers at 35.7, 33.6, and 25.5%, respectively. Thai streaming applications, such as Line TV, True ID, and AIS Play, have become trendy among Thai viewers and were favored entertainment applications at 33.9, 19.9, and 13.8%, respectively.
By investigating the respondents’ favorite content they watched or listened to, the top three most popular content types among Thai users were music shows at 72.9%, followed by series and movies, at 67.5 and 64.5%, respectively. Other content types, including special one-time events, sports events, video games, and business workshops, were moderately popular, approximately 30–34%.
4.3 Reliability and validity
Three tests were conducted on the measurement model: reliability, convergent validity, and discriminant validity. Table 3 presents a Cronbach’s alpha value, construct reliability (CR), and average variance extracted (AVE). These values indicated a satisfactory level of internal consistency in the measure (Hair et al., 2010).
All eleven of the study’s variables had Cronbach’s alpha values greater than 0.7, indicating that the respective variables had internal consistency (Bagozzi and Yi, 1988). There is evidence of satisfactory reliability and convergent validity for each construct because its composite reliability (CR) is greater than the recommended cutoff value of 0.70 (Bollen and Lennox, 1991), and its average variance extracted (AVE) is greater than 0.5 (Fornell and Larcker, 1981).
Furthermore, discriminant validity is shown when one variable’s square root of the AVE is greater than its correlation coefficient with the other variable. Each value on the diagonal is greater than all of the values to its left and below, as demonstrated by the data in Table 4, indicating the discriminant validity of all the variables in this study. In addition, the discriminant and convergent validity among 11 latent variables were subjected to model fit. Ten items across the variables were deleted to enhance factor loading.
An exploratory factor analysis (EFA) was performed. All 56 measurement items representing 12 variables in models were included in the study. The chosen approaches for the analysis were axis factor extraction and varimax rotation. Specific criteria were employed to guarantee a valid factor solution. Subsequently, the measurement items with a factor loading of 0.5 or higher were retained in a particular factor, while no significant loadings on other factors were seen. Each item with substantial cross-loadings was systematically eliminated, and the exploratory factor analysis (EFA) was recalculated after each removal. Supplementary Appendix B contains the primary loadings and cross-loadings of each item within the domain in SmartPLS, as well as the conclusive outcomes of the EFA.
The current literature states that the items were connected with each component. Addiction accounted for 52.59% of the variability and had the highest eigenvalue at 6.311. Conversely, the satisfaction factor accounted for only 1.594% of the variance, with an eigenvalue of 0.19, the lowest among all the components under consideration, including that they satisfied the necessary conditions for factor interpretation and may be subjected to additional analysis appropriately. As a formative assessment, several of the marketing item’s scores fall short of the cutoff for the marketing mix constructions. Because this was a formative variable, these low-loading items could not be deleted. Eliminating a formative measure is akin to removing a crucial element of the construct, as proposed by Bollen and Lennox (1991). As a result, all eight marketing mix components remained in place for further analysis.
Out of all the indices available, the SRMR was chosen as the main fit index for reporting because it focuses on the standardized difference between observed and predicted correlations. Henseler et al. (2014) propose using the Standardized Root Mean Square Residual (SRMR) as a measure of goodness of fit for PLS-SEM, which helps prevent model misspecification. In this study, SmartPLS offers bootstrap-based inference statistics for the SRMR criterion. To understand the implications of SRMR bootstrap confidence interval results, refer to the bootstrap-based test that assesses the exact overall model fit. For the SRMR value, the saturated model value is 0.064, while the estimated model value is 0.079. Both values meet the needed standards (Hu and Bentler, 1998).
Given the complexity of our model and the nature of our data, SRMR is especially well-suited for it as it is less susceptible to sample size and model complexity than other indices like TLI and CFI (Hair et al., 2010). In addition, the Exploratory Factor Analysis (EFA) conducted before the Structural Equation Modeling (SEM) showed positive results, suggesting that the data’s underlying factor structure is consistent with theoretical expectations. Based on the results of the EFA, it is clear that the measured variables have strong construct validity. Each factor loaded significantly on its respective construct and demonstrated good internal consistency. Based on the EFA results, it is evident that the measurement model was robust and aligned with the theory. This reinforces our choice to prioritize SRMR for assessing model fit.
The SRMR suggests a strong overall model fit, and the satisfactory EFA results validate the construct validity of our measurement model. Thus, we have full confidence that the observed connections between user addiction, continuance intention, and personality traits are authentic patterns in the data rather than errors in the model’s design.
The present study initially assessed the value for each analyzed construct to determine how the model explained the variation (Falk and Miller, 1992). Additionally, predictive Relevancevalue is a criterion of predictive accuracy and an indicator of the model’s predictive relevance, also known as Stone-Geisser’s by using the blindfolding procedure (Geisser, 1974; Stone, 1974). Hence, the result’s values of , adjusted, and are illustrated in Table 5.
4.4 Test the hypotheses
For the hypotheses testing, we analyze the structural model to test the hypotheses shown in Table 6 and report direct, indirect, and total effect testing in Table 7. The results found that, out of a total of twenty-one hypotheses, fifteen were significant, and only six were insignificant. The model explains 61.5% of the variance in continuance intention toward streaming services. Figure 2 shows the estimates of the structural model.
The findings of the measuring model indicate that confirmation has a significant positive effect on perceived usefulness and perceived enjoyment (H1, H2). It was found that confirmation significantly predicted perceived usefulness ß = 0.203, t = 3.823, p < 0.001, and confirmation significantly predicted perceived enjoyment ß = 0.276, t = 5.474, p < 0.001. Thus, the hypotheses are accepted, implying that when users expectations are confirmed, that will increase the perceived usefulness of streaming services.
In addition, the outcomes of the hypothesized model reveal that four factors, confirmation, perceived usefulness, perceived enjoyment, and marketing mix, have a significant positive effect on satisfaction (H3-H6). It was found that confirmation significantly predicted satisfaction ß = 0.226, t = 5.080, p < 0.001, perceived usefulness significantly predicted satisfaction ß = 0.178, t = 4.552, p < 0.001, perceived enjoyment significantly predicted satisfaction ß = 0.359, t = 8.065, p < 0.001, and marketing mix significantly predicted satisfaction ß = 0.225, t = 4.591, p < 0.001. Thus, these hypotheses are accepted, implying that when users have higher confirmation, perceived usefulness, and perceived enjoyment toward using streaming services, and the marketing mix of streaming service providers makes users confident in their decision to continue using the streaming service. The more users will increase the satisfaction level to use streaming services.
Subsequently, the results of the measuring model reveal that five factors, perceived usefulness, perceived enjoyment, satisfaction, addiction to heavy viewing, and marketing mix, have a significant positive effect on continuance intention (H7-H11). It was found that perceived enjoyment significantly predicted continuance intention ß = 0.205, t = 3.864, p < 0.001, satisfaction significantly predicted continuance intention ß = 0.354, t = 6.264, p < 0.001, addiction to heavy viewing significantly predicted continuance intention ß = 0.205, t = 5.638, p < 0.001, and marketing mix significantly predicted continuance intention ß = 0.131, t = 2.919, p < 0.01. Thus, these hypotheses are accepted, implying that the higher a user’s perceived enjoyment, satisfaction level, addiction to heavy viewing, and appreciation of streaming services’ marketing, the more they intend to continue using streaming services. However, perceived usefulness insignificantly predicted continuance intention ß = 0.014, t = 0.303, p = 0.762.
Furthermore, this research investigated the mediation effect of satisfaction on the relationship between perceived usefulness and continuance intention. The dependent variable used for the study was continuance intention. The predictor variable in the analysis was the perceived usefulness. The mediator variable for the analysis was satisfaction. Zhao et al. (2010) proposed a two-step approach in which several regression analyses are conducted, and the significance of the coefficients is examined at each step. In step 1, determining the significance of the indirect effect on path PU - > SAT - > CI (IE = 0.063, t = 4.005, p < 0.001), the finding found that the indirect effect was significant in both paths. Step 2: Determine the type of effect and mediation. The finding found a positive significance of the direct effect in the path PU - > CI (DE = 0.014, p = N.S. [not significant]). Thus, it was found that satisfaction had an indirect-only mediation effect on the relationship between perceived usefulness and continuance intention.
Similarly, this research investigated the mediation effect of satisfaction on the relationship between perceived enjoyment and continuance intention. The dependent variable used for the study was continuance intention. The predictor variable in the analysis was the perceived enjoyment. The mediator variable for the analysis was satisfaction. Zhao et al. (2010) proposed a two-step approach in which several regression analyses are conducted, and the significance of the coefficients is examined at each step. In step 1, determining the significance of the indirect effect on path PE - > SAT - > CI (IE = 0.127, t = 5.218, p < 0.001), the finding found that the indirect effect was significant in both paths. Step 2: Determine the type of effect and mediation. The finding found a positive significance of the direct effect in the path PE - > CI (DE = 0.205, p < 0.001). Thus, it was found that satisfaction had a complementary mediation effect on the relationship between perceived enjoyment and continuance intention.
In this study, we added the Big-Five personality traits as external variables to find the relationship between all five personality characteristics with perceived usefulness and perceived enjoyment toward using streaming services in the expectation confirmation model. The findings of the measuring model found that three characteristics, namely neuroticism, agreeableness, and conscientiousness, have a significant positive effect on perceived usefulness (H13, H14, H15). It was found that neuroticism significantly predicted perceived usefulness ß = 0.071, t = 2.267, p < 0.05, agreeableness significantly predicted perceived usefulness ß = 0.269, t = 5.636, p < 0.001, and conscientiousness significantly predicted perceived usefulness ß = 0.284, t = 5.208, p < 0.001.
Thus, these hypotheses are accepted, implying that the more users measure themselves as having neuroticism, agreeableness, and conscientiousness, the more they feel like streaming services are useful and valuable. However, openness’ s characteristics (H12) negatively but insignificantly predicted perceived usefulness ß = −0.009, t = 0.176, p = 0.860, and extraversion’s characteristics (H16) negatively but insignificantly predicted perceived usefulness ß = −0.064, t = 1.558, p = 0.119.
Finally, this research investigated the relationship between the Big five personality characteristics and perceived enjoyment of streaming services. The findings of the hypothesized model found that two characteristics, namely agreeableness and conscientiousness, have a significant positive effect on perceived enjoyment (H19, H20). It was found that agreeableness significantly predicted perceived enjoyment ß = 0.332, t = 7.877, p < 0.001, conscientiousness significantly predicted perceived enjoyment ß = 0.251, t = 5.371, p < 0.001. Thus, the hypothesis is accepted, implying that the more users measure themselves as having agreeableness characteristics such as being kind, generous, trusting, getting along with people, and being a helpful person, and having conscientiousness such as a high level of thoughtful, good impulse control, and goal-directed behavior, the more they perceived increase enjoyment during using streaming services. However, openness’ s characteristic (H17) insignificantly predicted perceived enjoyment ß = 0.057, t = 1.266, p = 0.205, neuroticism’s characteristic (H18) negatively but insignificantly predicted perceived enjoyment ß = −0.016, t = 0.514, p = 0.607, and extraversion’s characteristics (H21) negatively but insignificantly predicted perceived enjoyment ß = −0.058, t = 1.822, p = 0.069.
5 Discussion and conclusions
5.1 Discussion
This research integrated the Expectation Confirmation Model (ECM) with the Five-Factor Model (FFM) of personality traits to examine factors influencing users’ continuance intention in streaming services. The combined theoretical framework provided a robust model for analyzing empirical data using structural equation modeling (SEM), with 15 hypotheses found significant and six found insignificant. These results highlight the model’s robustness and predictive power in understanding intentions to use streaming services.
The findings support the hypotheses related to the ECM, demonstrating that confirmation positively influences perceived usefulness, perceived enjoyment, and satisfaction in streaming services usage. These findings align with previous research on new technology adoption (Praveena and Thomas, 2014; Dickinger et al., 2008). According to the ECM, confirmation positively impacts users’ perceptions of the system’s utility and overall satisfaction (Zhou, 2017). Several studies have similarly noted that customer satisfaction with a product or service correlates with the level of affirmation of its value (Alraimi et al., 2015; Bhattacherjee, 2001; Oliver, 1980; Venkatesh et al., 2011). Satisfaction is achieved when users perceive that the service meets or exceeds their expectations, resulting in a positive usage experience (Oghuma et al., 2015).
The study also confirms that perceived enjoyment is positively related to service utilization satisfaction, which positively influences the intention to continue using the service (Dickinger et al., 2008). Increased satisfaction has been shown to enhance loyalty to streaming services, consistent with findings in other research contexts (Mangini et al., 2023; Periaiya and Nandukrishna, 2023; Katz et al., 1973; Bae, 2018).
The study identified a positive relationship between continuance intention and factors such as perceived usefulness, perceived enjoyment, satisfaction, addiction, and marketing mix. Consistent with the expectation confirmation model in information systems (ECM-IS), the findings emphasize that user satisfaction is the most critical determinant of continuance intention (Alalwan et al., 2018). Additionally, the marketing mix significantly influences customers’ purchasing decisions by catering to their needs and enhancing satisfaction (Yoyada and Kodrat, 2017).
This study explores the one-way relationship between addiction and continuance intention. Previous studies have found a direct link between addiction and continued usage (Kesici and Tunc, 2018), while others suggest that frequent usage may lead to habitual behaviors and potential addiction, particularly in social media contexts (Maqableh et al., 2021; Turel et al., 2010; Ronis et al., 1989). This finding highlights the need for future research to explore these factors further.
The study also examines the role of personality traits in streaming service usage. The findings affirm that the Big Five personality traits, particularly agreeableness, conscientiousness, and neuroticism, significantly influence perceived usefulness and enjoyment (Leephaijaroen, 2016; Kline et al., 1981). These traits align with the cultural context of Thailand, where most individuals exhibit high levels of agreeableness and conscientiousness.
The insignificant relationships found in this study may be unique to the Thai population. Moreover, the insignificant relationships may not fully imply that perceived usefulness does not influence continuance intention at all. Other factors may be more critical in the decision to continue using streaming services. This is where future research could come into play.
5.2 Theoretical contributions
This study extends the theoretical framework of the Expectation Confirmation Model (ECM), addiction theory, continuance intention, marketing mix, and personality traits to the context of Thai streaming consumers. The primary contribution is the investigation of users’ intentions to adopt streaming services as a new technology and their continuance intention to subscribe. This study advances theoretical understanding by examining streaming users’ satisfaction and continuance intention (Bhattacherjee, 2001) through the ECM and Five-Factor Model (FFM) frameworks.
The research developed a comprehensive model to quantify relationships among various factors influencing video streaming services and consumer behavior. It uniquely integrates variables like addiction to heavy viewing and marketing mix to assess ongoing usage intentions. While these variables have been discussed in previous technology adoption studies, their impact within the specific cultural context of Thai streaming services still need to be explored, making this study distinctive.
The ECM model, traditionally used to predict satisfaction with new technology within organizations, has been effectively applied to understand consumer behavior in online contexts (Flavián et al., 2006; George, 2002; Guo and Barnes, 2009). The theory’s application in marketing communications further illustrates its utility in explaining consumer behavior regarding purchase intentions and online usage patterns. Previous studies combined satisfaction theory as a mediator between antecedents (e.g., perceived usefulness, perceived enjoyment) and continuance intention (Chiu et al., 2019; Kwok et al., 2011; Tan and Kim, 2015; Mouakket, 2018). This study expands upon these findings by incorporating personality traits and marketing mix elements.
The study’s analysis of personality traits such as extraversion, openness, agreeableness, conscientiousness, and neuroticism provide novel insights into their relevance to OTT streaming services. The findings emphasize the importance of these traits in influencing consumer behavior, particularly in the Thai context. These insights call for further research to explore the broader implications of personality traits on streaming service usage and potential addiction to heavy viewing.
Finally, the relationship between the marketing mix, satisfaction, and continuance intention demonstrates the adaptability of the technology adoption model in investigating consumer behavior. The research contributes to the theory by applying the marketing mix within the technology adoption model to investigate how consumers perceive and engage with streaming services. By merging these two frameworks, the study shows how marketing mix elements like pricing strategies, product features, and distribution channels can be optimized to support technology adoption. This theoretical advancement offers a new perspective on how marketing interventions influence both the early stages of technology adoption and the continued use of such technologies. This study provides additional evidence of the impact of these variables on users’ propensity to adopt new services, contributing to a more nuanced understanding of user behavior in the streaming industry.
5.3 Practical implications
The results of this study offer several practical implications for streaming service providers seeking to enhance user engagement and retention.
The analysis indicates that higher satisfaction levels significantly increase the likelihood of continued subscription to streaming services. As such, customer satisfaction is critical for maintaining subscription intentions, particularly within the Thai market. Providers should prioritize developing unique marketing mix strategies influencing customer satisfaction and purchasing behavior.
According to the hypotheses testing, the significance of this research is that the higher the satisfaction level experienced by the user, the more likely the subscribers will continue to use the streaming services. Ensuring user expectations and satisfaction are met is essential for maintaining ongoing usage. Providers must consistently seek user feedback and closely monitor satisfaction levels to pinpoint areas that can be enhanced. Users can directly contribute their content and service quality input by incorporating adaptive users feedback loops. This valuable feedback allows for the dynamic adjustment of service offerings to better align with user expectations.
Furthermore, customer satisfaction was the most potent factor driving the continuance intention toward streaming services in Thailand. In other words, the user’s satisfaction is crucial in keeping the user’s intention to subscribe to streaming services in the Thai context. Furthermore, unique marketing mix strategies are essential in influencing clients to receive satisfaction and buy a product or service a specific business offers.
Moreover, this study also found that the agreeableness and conscientiousness of Thai users’ personalities affected the perceived usefulness and enjoyment of streaming services. The niche market of these personality groups (introverts) is the most important for launching unique campaigns to maintain this group of customers and persuade them to subscribe to use streaming services. Regarding personality traits, introverted people need more social reactions and online communication; the marketer should be concerned about keeping and building online communities to let these users target a group presence in the online world, such as community group chats and activities on social media. Each marketer will endeavor to collect data regarding the needs and preferences of every prospective customer. In contrast, customers will seek information about the product or service they want to adopt. In addition, considering the impact of personality traits on continuance intention, streaming services would benefit from creating personalized content recommendation algorithms that cater to various personality profiles. Introverted users might like introspective, instructional, and low-stimulation content. Providers can better personalize suggestions by analyzing user activity patterns using machine learning techniques.
Furthermore, the streaming content also leads to the user’s emotion and enjoyment. Marketers should seek unique content that is attractive to Thai users, especially content that relates to their personalities, as basically, most Thai people are kind and empathize with others. Therefore, content was the first element of the marketing mix of streaming services; if the company launches the content to the right target groups, they will become more addicted to watching. Moreover, the user’s emotion and enjoyment are the most essential elements of the content; if they enjoy the content that streaming platforms provide, they will become more addicted. When users have emotions and feelings about the streaming content, in addition to the decision to use and subscribe, these give an advantage to other commercial players in different industries. Trends from the artists and actors cause the mainstream to support the users in buying other products or services or educating the Thai users in different industries. Customizing marketing strategies to resonate with various user segments is crucial, considering their unique personality traits and viewing habits. As marketing analysts, providers could create targeted campaigns focusing on content that resonates with different personality types. Additionally, one could develop promotions to increase engagement among users who have shown low-interest levels.
Marketers should discover the streaming content that Thai target groups love to consume and the popular content trends. The wide range of streaming content could increase the user’s addiction to heavy viewing. The previous studies confirm that the issue of excessive viewing addiction is significant for online streaming platforms as they provide captivating content that leads to a persistent pattern of heavy viewing, regardless of the negative repercussions (Li and Chung, 2006; Young and Abreu, 2011). Based on the findings, addiction viewing habits can strongly indicate a person’s likelihood of continuing to engage in that behavior. Streaming services can enhance user engagement by introducing interactive content, binge-watching incentives, and community-building tools such as user forums and social sharing options. These features can enhance user engagement with the platform and promote extended viewing sessions.
By following these suggestions, streaming service providers can improve their ability to cater to their users’ various needs and preferences. This will result in higher user satisfaction, lower customer turnover, and the development of extended loyalty. Implementing these strategies will ensure the retention of current users and also attract new users, leading to the long-term growth and sustainability of the streaming platform.
5.4 Limitations and suggestions for future research
While it is crucial to acknowledge the limitations, the research findings still offer valuable insights to researchers in OTT streaming services.
First, this study is limited by the generalizability constraint due to non-probabilistic sampling for data collection. The study’s findings may need more generalizability due to potential biases toward particular demographics, such as age groups, geographical places, or socio-economic backgrounds. Therefore, future studies could use probabilistic sampling to increase external validity. Second, the data used for this research is cross-sectional, which does not evaluate the user’s long-term behavior. A longitudinal approach would better understand the direction of the change in users’ behavior, such as addiction to heavy viewing and personality traits. Third, according to the respondents’ demographic characteristics, future research could work on a multigroup analysis. It may also add more insight into the findings. The moderating effect of demographics should be included. Fourth, adopting technology is constantly changing, and the fast development of OTT streaming services may make the current findings less applicable in the future. The study’s findings may be constrained by the timeframe during which data was gathered. Fifth, contextual factors like cultural differences, regulatory mandates, and limitations on infrastructure can influence OTT streaming service adoption.
In the aspect of the business, future studies could explore further the design of the streaming service and its interaction with users. For instance, the impact of advertising on users’ decision-making is seen in the availability of a free service tier that includes advertisements. In contrast, the subscription tier offers an ad-free experience. Exploring why people like to watch short movie clips more is interesting. Both may relate to marketing and psychology theories that can investigated.
Moreover, this type of business may continue to be famous in the future. Or can it replace streaming? Like the tradition, watch movies in the cinema or go to a live concert. When changing to watching online, can it be replaced? This phenomenon can change Thailand’s economy. Subsequently, in terms of psychology, future research could be carried out to investigate online consumer behaviors. The findings found that streaming content was the most critical factor, as were the viewers’ feelings, emotions, and communication, especially social values and subjective norms. Parasocial may affect the continuation of streaming services and be a prominent commercial factor in other industries. Finally, regarding personality traits, the users’ personalities in Asian countries and Western countries may be different.
Data availability statement
The original contributions presented in the study are included in the article/Supplementary material, further inquiries can be directed to the corresponding author/s.
Ethics statement
Ethical review and approval was not required for the study on human participants in accordance with the local legislation and institutional requirements. The studies were conducted in accordance with the local legislation and institutional requirements. Written informed consent forparticipation in this study was provided by the participants’ legal guardians/next of kin.
Author contributions
KS-t: Writing – original draft, Writing – review & editing, Data curation, Formal analysis, Investigation, Project administration, Software. JL: Supervision, Writing – review & editing. QW: Conceptualization, Methodology, Supervision, Writing – review & editing.
Funding
The author(s) declare that no financial support was received for the research, authorship, and/or publication of this article.
Acknowledgments
We are grateful to our colleagues who have given great support for the study, even though they do not agree with the definitions offered by this paper, names, and surnames.
Conflict of interest
The authors declare that the research was conducted in the absence of any commercial or financial relationships that could be construed as a potential conflict of interest.
Publisher’s note
All claims expressed in this article are solely those of the authors and do not necessarily represent those of their affiliated organizations, or those of the publisher, the editors and the reviewers. Any product that may be evaluated in this article, or claim that may be made by its manufacturer, is not guaranteed or endorsed by the publisher.
Supplementary material
The Supplementary material for this article can be found online at: https://www.frontiersin.org/articles/10.3389/fcomm.2024.1410975/full#supplementary-material
References
Agarwal, R., and Prasad, J. (1999). Are individual differences germane to the acceptance of new information technologies? Decis. Sci. 30, 361–391. doi: 10.1111/j.1540-5915.1999.tb01614.x
Akdim, K., Casaló, L. V., and Flavián, C. (2022). The role of utilitarian and hedonic aspects in the continuance intention to use social mobile apps. J. Retail. Consum. Serv. 66:102888. doi: 10.1016/j.jretconser.2021.102888
Alalwan, A. A., Dwivedi, Y. K., Rana, N. P., and Algharabat, R. (2018). Examining factors influencing Jordanian customers’ intentions and adoption of internet banking: extending UTAUT2 with risk. J. Retail. Consum. Serv. 40, 125–138. doi: 10.1016/j.jretconser.2017.08.026
Albashrawi, M., and Motiwalla, L. (2017). Privacy and personalization in continued usage intention of Mobile banking: an integrative perspective. Inf. Syst. Front., 19, 1329–1344. doi: 10.1007/s10796-017-9814-7
Alraimi, K. M., Zo, H., and Ciganek, A. P. (2015). Understanding the MOOCs continuance: the role of openness and reputation. Comput. Educ. 80, 28–38. doi: 10.1016/j.compedu.2014.08.006
American Psychiatric Association. (2000). Diagnostic and statistical manual of mental disorders, 4th Edition, American Psychiatric Association: Text Revision (DSM-IV-TR).
Ananthos, S. (2018). OTT in Thailand: an analysis of the opportunities and constraints. Int. J. Manag. Appl. Sci. 4, 43–48.
Bae, M. (2018). Understanding the effect of the discrepancy between sought and obtained gratification on social networking site users’ satisfaction and continuance intention. Comput. Hum. Behav. 79, 137–153. doi: 10.1016/j.chb.2017.10.026
Bagozzi, R. P., and Yi, Y. (1988). On the evaluation of structural equation models. J. Acad. Mark. Sci. 16, 74–94. doi: 10.1007/bf02723327
Barata, M. L., and Coelho, P. S. (2021). Music streaming services: understanding the drivers of customer purchase and intention to recommend. Heliyon 7:e07783. doi: 10.1016/j.heliyon.2021.e07783
Barrick, M. R., and Mount, M. K. (1991). The big five personality dimen- sions and job performance: a meta-analysis. Pers. Psychol. 44, 1–26. doi: 10.1111/j.1744-6570.1991.tb00688.x
Bhattacherjee, A. (2001). Understanding information systems continuance: an expectation-confirmation model. MIS Quaterly 25, 351–370. doi: 10.2307/3250921
Bollen, K. A., and Lennox, R. (1991). Conventional wisdom on measurement: a structural equation perspective. Psychol. Bull. 110, 305–314. doi: 10.1037/0033-2909.110.2.305
Booms, H., and Bitner, M. J. (1981). “Marketing strategies and organization: structure for service firms” in Marketing of services, conference proceedings. eds. J. H. Donnelly and W. R. George (Chicago, IL: American Marketing Association).
Butt, M. M., and Phillips, J. G. (2008). Personality and self-reported mobile phone use. Comp. Human Behav. 24, 346–360. doi: 10.1016/j.chb.2007.01.019
Chaffey, D., and Ellis-Chadwick, F. (2019). Digital marketing: Strategy, implementation, and practice. 7th Edn: Pearson.
Chiu, C.-K., Lin, C.-P., Chen, K.-J., Liu, C.-M., and Ma, H.-C. (2019). Modeling continuance intention towards Mobile travel service system (MTSS): a theoretical perspective of motivation and dependency. Rev. Manag. Sci. 13, 749–769. doi: 10.1007/s11846-017-0257-9
Churchill, G. A., and Surprenant, C. (1982). An investigation into the determinants of customer satisfaction. J. Mark. Res. 19, 491–504. doi: 10.1177/002224378201900410
Comrey, A. L., and Lee, H. B. (1992). A first course in factor analysis : Lawrence Erlbaum Associates.
Constantinides, E. (2006). The marketing mix revisited: towards the 21st-century marketing. J. Mark. Manag. 22, 407–438. doi: 10.1362/026725706776861190
Correa, T., Hinsley, A. W., and De Zúñiga, H. G. (2010). The influence of social networks on political participation: the role of offline and online networks. J. Comput.-Mediat. Commun. 15, 1–23.
Costa, P. T., and McCrae, R. R. (1992). The five-factor model of personality and its relevance to personality disorders. J. Personal. Disord. 6, 343–359. doi: 10.1521/pedi.1992.6.4.343
Cronbach, L. J. (1951). Coefficient alpha and the internal structure of tests. Psychometrika 16, 297–334. doi: 10.1007/BF02310555
Davis, F. D. (1989). Perceived usefulness, perceived ease of use, and user acceptance of information a. MIS Q. 13, 319–340. doi: 10.2307/249008
Davis, F. D., Bagozzi, R. P., and Warshaw, P. R. (1992). Extrinsic and intrinsic motivation to use computers in the workplace. J. Appl. Soc. Psychol. 22, 1111–1132. doi: 10.1111/j.1559-1816.1992.tb00945.x
Devaraj, S., Easley, R. F., and Crant, J. M. (2008). How does personality matter? Relating the five-factor model to technology acceptance and use. Inf. Syst. Res. 19, 93–105. doi: 10.1287/isre.1070.0153
DeYoung, C. G., Quilty, L. C., and Peterson, J. B. (2007). Between facets and domains: 5+1=10 facets of the big five. J. Pers. Soc. Psychol. 93, 880–896. doi: 10.1037/0022-3514.93.5.880
Dickinger, A., Arami, M., and Meyer, D. (2008). The role of perceived enjoyment and social norm in the adoption of technology with network externalities. Eur. J. Inf. Syst. 17, 4–11. doi: 10.1057/palgrave.ejis.3000726
Electronic Transactions Development Agency. (2022). Thailand internet user behavior 2022. Available at: https://www.etda.or.th/getattachment/78750426-4a58-4c36-85d3-d1c11c3db1f3/IUB-65-final.Pdf.Aspx
Engberg, E., Figueiredo, R. A., Rounge, T. B., Weiderpass, E., and Viljakainen, H. (2019). Heavy screen users are the heaviest among 10,000 children. Sci. Rep. 9, 1–9. doi: 10.1038/s41598-019-46971-6
Falk, R. F., and Miller, N. B. (1992). A primer for soft modeling. Akron, OH: University of Akron Press.
Farid, M. S., Cavicchi, A., Rahman, M. M., Barua, S., Ethen, D. Z., Happy, F. A., et al. (2023). Assessment of marketing mix associated with consumer’s purchase intention of dairy products in Bangladesh: application of an extended theory of planned behavior. Heliyon 9:e16657. doi: 10.1016/j.heliyon.2023.e16657
Federal Communication Commission. (2012). Annual assessment of the status of competition in the market for the delivery of video programming. Federal Register. Available at: https://www.federalregister.gov/documents/2012/08/08/2012-19107/annual-assessment-of-the-status-of-competition-in-the-market-for-the-delivery-of-video-programming
Feist, G. J. (1998). A meta-analysis of personality in scientific and artistic creativity. Personal. Soci. Psychol. Bullet. 2, 290–309. doi: 10.1207/s15327957pspr0204_5
Flavián, C., Guinalíu, M., and Gurrea, R. (2006). The role played by perceived usability, satisfaction and consumer trust on website loyalty. Inf. Manag. 43, 1–14. doi: 10.1016/j.im.2005.01.002
Fornell, C., and Larcker, D. F. (1981). Evaluating structural equation models with unobservable variables and measurement error. J. Mark. Res. 18, 39–50. doi: 10.1177/002224378101800104
Franque, F. B., Oliveira, T., Tam, C., and Santini, F.De. (2020). A meta-analysis of the quantitative studies in continuance intention to use an information system. Internet Res., 31, 123–158. doi: 10.1108/intr-03-2019-0103
Gallivan, M. J., Spitler, V. K., and Koufaris, M. (2005). Does information tech- nology training really matter? A social information processing analysis of coworkers’ influence on IT usage in the workplace. J. Manag. Inf. Syst. 22, 153–192. doi: 10.1080/07421222.2003.11045830
Geisser, S. (1974). A predictive approach to the random effects model. Biometrika 61, 101–107. doi: 10.1093/biomet/61.1.101
George, J. F. (2002). Influences on the intent to make internet purchases. Internet Res. 12, 165–180. doi: 10.1108/10662240210422521
Goldberg, L. R. (1990). An alternative “description of personality”: the big- five factor structure. J. Pers. Soc. Psychol. 59, 1216–1229. doi: 10.1037/0022-3514.59.6.1216
Goldberg, L. R. (1999). A broad-bandwidth, public-domain, personality inventory measuring the lower-level facets of several five-factor models. In I. Mervielde, I. Deary, F. FruytDe, and F. Ostendorf (Eds.), Personality psychology in Europe (pp. 7–28). Tilburg, The Netherlands: Tilburg University Press.
Goldberg, L. R., Johnson, J. A., Eber, H. W., Hogan, R., Ashton, M. C., Cloninger, C. R., et al. (2006). The international personality item pool and the future of public- domain personality measures. J. Res. Pers. 40, 84–96. doi: 10.1016/j.jrp.2005.08.007
Guo, Y., and Barnes, S. (2009). Virtual item purchase behavior in virtual worlds: an exploratory investigation. Electron. Commer. Res. 9, 77–96. doi: 10.1007/s10660-009-9032-6
Hair, J. F. J., Black, W. C., Babin, B. J., and Anderson, R. E. (2010). Multivariate data analysis. A global perspective. 7th Edn. USA: Prentice Hall.
Halilovic, S., and Cicic, M. (2013). Antecedents of information systems user behavior-extended expectation-confirmation model. Behav. Inf. Technol. 32, 359–370. doi: 10.1080/0144929X.2011.554575
Henseler, J., Dijkstra, T. K., Sarstedt, M., Ringle, C. M., Diamantopoulos, A., Straub, D. W., et al. (2014). Common beliefs and reality about PLS: comments on Rönkkö and Evermann (2013). Organiz. Res. Methods 17, 182–209. doi: 10.1177/1094428114526928
Horvath, C. W. (2004). Measuring television addiction. J. Broadcasting Electronic Media 48, 378–398. doi: 10.1207/s15506878jobem4803_3
Hsu, C.-L., and Lin, J. C.-C. (2016). Effect of perceived value on behavioral intention in using online streaming services: mediating roles of trust and enjoyment. Comput. Hum. Behav. 62, 123–132.
Hu, L.-T., and Bentler, P. M. (1998). Fit indices in covariance structure modeling: sensitivity to underparameterized model misspecification. Psychol. Methods 3, 424–453. doi: 10.1037/1082-989X.3.4.424
Huang, C.-H., Lee, L.-Y., and Chang, M.-L. (2007). The influences of personality and motivation on exercise participation and quality of life. Soc. Behav. Personal. Int. J. 35, 1189–1210. doi: 10.2224/sbp.2007.35.9.1189
International Data Corporation. (2015). Digital transformation (DX): an opportunity and an imperative.
Jumaan, I. A., Hashim, N. H., and Al-Ghazali, B. A. (2020). The role of cognitive absorption in predicting mobile internet users’ continuance intention: an extension of the expectation-confirmation model. Technol. Soc. 63:101355. doi: 10.1016/j.techsoc.2020.101355
Katz, E., Blumler, J. G., and Gurevitch, M. (1973). Uses and gratifications research. Pub. Opinion Quart. 37, 509–523. doi: 10.1086/268109
Kesici, A., and Tunc, N. F. (2018). Investigating the digital addiction level of the university students according to their purposes for using digital tools. Univ. J. Educ. Res. 6, 235–241. doi: 10.13189/ujer.2018.060204
Kim, B., Chan, H. C., and Gupta, S. (2016). Value-based adoption of mobile internet: an empirical investigation. Decis. Support. Syst. 43, 111–126. doi: 10.1016/j.dss.2005.05.009
Kim, M. J., and Lee, C. K. (2009). Marketing mix and consumer perception: an empirical study in the service sector. J. Serv. Market. 23, 517–528.
Kline, P., Barrett, P., and Svaste-Xuto, B. (1981). Personality traits of Thai students. J. Soc. Psychol. 114, 23–34. doi: 10.1080/00224545.1981.9922722
Kuss, D. J., and Lopez-Fernandez, O. (2016). Internet addiction and problematic internet use: a systematic review of clinical research. World J. Psychiatry 6, 143–176. doi: 10.5498/wjp.v6.i1.143
Kusumawati, R. D., Oswari, T., Yusnitasari, T., Mittal, S., and Kumar, V. (2021). Impact of marketing-mix, culture and experience as moderator to purchase intention and purchase decision for online music product in Indonesia. Int. J. Bus. Innov. Res. 25:475. doi: 10.1504/ijbir.2021.117089
Kwok, C.-W., Hui, S.-C., Mak, S.-N., Wu, K.-S., Lee, W.-K., and Wong, C.-K. (2011). Can mobile virtual fitness apps replace human fitness trainer?. In Paper Presented at the 5th International Conference on New Trends in Information Science and Service Science. 56–63. Available at: https://www.scopus.com/record/display.uri?eid=2-s2.0-83755164013&origin=recordpage
Lee, M. C. (2010). Explaining and predicting users’ continuance intention toward e-learning: an extension of the expectation–confirmation model. Comput. Educ. 54, 506–516. doi: 10.1016/j.compedu.2009.09.002
Lee, J. S., and Cho, J. H. (2021). Determinants of continuance intention for over-the-top services. Soc. Behav. Personal. Int. J. 49, 1–13. doi: 10.2224/sbp.10566
Leephaijaroen, S. (2016). Effects of the big-five personality traits and organizational commitments on organizational citizenship behavior of support staff at Ubon Ratchathani Rajabhat University, Thailand. Kasetsart J. Soci. Sci. 37, 104–111. doi: 10.1016/j.kjss.2015.03.002
Lestari, E. D., and Soesanto, O. R. C. (2020). Predicting factors that influence attitude to use an its implications on continuance intention to use SVOD: study on Netflix users of Indonesia. DeReMa 15, 184–208.
Li, S. M., and Chung, T. M. (2006). Internet function and internet addictive behavior. Comput. Hum. Behav. 22, 1067–1071. doi: 10.1016/j.chb.2004.03.030
Lim, X.-J., Cheah, J.-H., Ng, S. I., Basha, N. K., and Soutar, G. (2021). The effects of anthropomorphism presence and the marketing mix have on retail app continuance use intention. Technol. Forecast. Soc. Chang. 168:120763. doi: 10.1016/j.techfore.2021.120763
Lin, C.-P., and Bhattacherjee, A. (2008). Elucidating individual intention to use interactive information technologies: the role of network externalities. Int. J. Electron. Commer. 13, 85–108. doi: 10.2753/jec1086-4415130103
Lin, K. Y., and Lu, H. P. (2011). Why people use social networking sites: An empirical study integrating network externalities and motivation theory. Comput. Hum. Behav. 27, 1152–1161. doi: 10.1016/j.chb.2010.12.009
Lin, C. S., Wu, S., and Tsai, R. J. (2005). Integrating perceived playfulness into expectation- confirmation model for web portal context. Inf. Manag. 42, 683–693. doi: 10.1016/j.im.2004.04.003
Mangini, E. R., Silva, R. G., Lopes, L., and Esteves, S. (2023). Assessing the effects of technology adoption model on satisfaction and loyalty in streaming services. Consumer Behav. Rev. 7. doi: 10.51359/2526-7884.2023.254499
Maqableh, M., Obeidat, Z., Obeidat, A., Jaradat, M., Shah, M. H., and Masadeh, R. (2021). Examining the determinants of Facebook continuance intention and addiction: the moderating role of satisfaction and trust. Informatics 8, 62–85. doi: 10.3390/informatics8030062
Martínez-Sánchez, M. E., Nicolas-Sans, R., and Bustos Díaz, J. (2021). Analysis of the social media strategy of audio-visual otts in Spain: the case study of netflix, HBO and Amazon prime during the implementation of disney+. Technol. Forecast. Soc. Chang. 173:121178. doi: 10.1016/j.techfore.2021.121178
McCrae, R. R., and Costa, P. T. (1997). Personality trait structure as a human universal. Am. Psychol. 52, 509–516
Mouakket, S. (2018). The role of personality traits in motivating users’ continuance intention towards Facebook: gender differences. J. High Technol. Manag. Res. 29, 124–140. doi: 10.1016/j.hitech.2016.10.003
Nagaraj, S., Singh, S., and Yasa, V. R. (2021). Factors affecting consumers’ willingness to subscribe to over-the-top (OTT) video streaming services in India. Technol. Soc. 65:101534. doi: 10.1016/j.techsoc.2021.101534
The National Broadcasting and Telecommunications Commission. (2022). OTT services market analysis: Global OTT revenue and subscriber growth. Available at: https://www.scopus.com/record/display.uri?eid=2-s2.0-83755164013&origin=recordpage
Ogbanufe, O., and Gerhart, N. (2017). Watch it! Factors driving continued feature use of the smartwatch. Int. J. Human Comp. Interact. 34, 999–1014. doi: 10.1080/10447318.2017.1404779
Oghuma, A. P., Chang, Y., Libaque-Saenz, C. F., Park, M. C., and Rho, J. J. (2015). Benefit- confirmation model for post-adoption behavior of mobile instant messaging applications: a comparative analysis of KakaoTalk and Joyn in Korea. Telecommun. Policy 39, 658–677. doi: 10.1016/j.telpol.2015.07.009
Oliver, R. L. (1980). A cognitive model of the antecedents and consequences of satisfaction decisions. J. Mark. Res. 17, 460–469. doi: 10.1177/002224378001700405
Pal, D., and Triyason, T. (2018). User intention towards a music streaming service: a Thailand case study. KnE Soci. Sci. 3:1. doi: 10.18502/kss.v3i1.1393
Pereira, R., and Tam, C. (2021). Impact of enjoyment on the usage continuance intention of video-on-demand services. Inform. Manag. 58:103501. doi: 10.1016/j.im.2021.103501
Periaiya, S., and Nandukrishna, A. T. (2023). What drives user stickiness and satisfaction in OTT video streaming platforms? A mixed-method exploration. J. Human Comp. Interact. 40, 2326–2342. doi: 10.1080/10447318.2022.2160224
Praveena, K., and Thomas, S. (2014). Continuance behavioral intention Facebook: a study of perceived enjoyment and TAM. Bonfring Int. J. Industrial Eng. Manag. Sci. 4, 24–29. doi: 10.9756/BIJIEMS.4794
Ringle, C. M., Wende, S., and Becker, J.-M. (2022). SmartPLS 4. Oststeinbek: SmartPLS GmbH http://www.smartpls.com.
Roca, J. C., Chiu, C.-M., and Martínez, F. J. (2006). Understanding e-learning continuance intention: an extension of the technology acceptance model. Int. J. Human Comp. Stud. 64, 683–696. doi: 10.1016/j.ijhcs.2006.01.003
Ronis, D. L., Yates, J. F., and Kirscht, J. P. (1989). Attitudes, decisions, and habits as determinants of repeated behavior. Mahwah, NJ: Lawrence Erlbaum.
Shin, S., and Park, J. (2021). Factors affecting users’ satisfaction and dissatisfaction of OTT services in South Korea. Telecommun. Policy 45:102203. doi: 10.1016/j.telpol.2021.102203
Stone, M. (1974). Cross-Validatory choice and assessment of statistical predictions. J. Royal Stat. Soci. 36, 111–133. doi: 10.1111/j.2517-6161.1974.tb00994.x
Suki, N. M. (2011). Exploring the relationship between perceived usefulness, perceived ease use, perceived enjoyment, attitude, and subscribers’ intention towards using 3G mobile services. Journal of Information Technology Management. 22, 1–7.
Susanto, A., Chang, Y., and Ha, Y. (2016). Determinants of continuance intention to use the smartphone banking services. Indust. Manag. Data Syst. 116, 508–525. doi: 10.1108/imds-05-2015-0195
Tabachnick, B. G., and Fidell, L. S. (1996). Using multivariate statistics. New York, NY: HarperCollins Publishers.
Tan, X., and Kim, Y. (2015). User acceptance of SaaS-based collaboration tools: a case of Google docs. J. Enterp. Inf. Manag. 28, 423–442. doi: 10.1108/JEIM-04-2014-0039
Tran, T.-T.-Q., Tran, Q.-T., and Le, H.-S. (2019). An empirical study on continuance using intention of ott apps with Young generation. Lecture Notes Elect. Eng. 219–229. doi: 10.1007/978-981-32-9244-4_31
Turel, O., Serenko, A., and Bontis, N. (2010). User acceptance of hedonic digital artifacts: a theory of consumption values perspective. Inf. Manag. 47, 53–59. doi: 10.1016/j.im.2009.10.002
Van der Heijden, H. (2004). User acceptance of hedonic information systems. MIS Q. 28, 695–704. doi: 10.2307/25148660
Venkatesh, V., and Bala, H. (2008). Technology acceptance model 3 and a research agenda on interventions. Decis. Sci. 39, 273–315. doi: 10.1111/j.1540-5915.2008.00192.x
Venkatesh, V., and Davis, F. D. (2000). A theoretical extension of the technology acceptance model: four longitudinal field studies. Manag. Sci. 46, 186–204. doi: 10.1287/mnsc.46.2.186.11926
Venkatesh, V., Thong, J. Y. L., Chang, F. K. Y., Hu, P. J. H., and Brown, S. A. (2011). Extending the two-stage information systems continuance model: incorporating UTAUT predictors and the role of context. Inf. Syst. J. 21, 527–555. doi: 10.1111/j.1365-2575.2011.00373.x
Wrench, J. S., Thomas-Maddox, C., Richmond, V. P., and McCroskey, J. C. (2008). Quantitative research methods for communication: A hands-on approach. New York, NY: Oxford University Press, Inc.
YouGov. (2023). OTT video streaming services in Thailand: how do platforms compare in popularity vs satisfaction? Available at: https://business.yougov.com/content/47753-ott-video-streaming-services-platforms-thailand-popularity-vs-satisfaction-netflix-disney-plus-amazon-prime (Accessed November 13, 2023).
Yousaf, A., Mishra, A., Taheri, B., and Kesgin, M. (2021). A cross-country analysis of the determinants of customer recommendation intentions for over-the-top (OTT) platforms. Inform. Manag. 58:103543. doi: 10.1016/j.im.2021.103543
Yoyada, N., and Kodrat, D. S. (2017). Effect of marketing mix (7P) on decision of consumer selection in NY dental clinic of Surabaya City. The Fourth International Conference on Entrepreneurship. 219–230. Available at: https://icoen.org/icoen2017/
Zhao, X., Lynch, J. G., and Chen, Q. (2010). Reconsidering Baron and Kenny: Myth and Truths About Mediation Analysis. Journal of Consumer Research. 37, 197–206. doi: 10.1086/651257
Zhou, J. (2017). Exploring the factors affecting learners’ continuance intention of MOOCs for online collaborative learning: an extended ECM perspective. Australas. J. Educ. Technol. 33, 123–135. doi: 10.14742/ajet.2914
Keywords: streaming platforms, expectation confirmation model (ECM), five-factors model (FFM) of personality, addiction to heavy viewing, marketing mix (7Ps), continuance intention to use, media industries
Citation: Sae-tae K, Ling J and Wang Q (2024) The impact of user addiction on continuance intention to use streaming platforms: incorporating expectation confirmation model and personality traits. Front. Commun. 9:1410975. doi: 10.3389/fcomm.2024.1410975
Edited by:
Tereza Semerádová, Technical University of Liberec, CzechiaReviewed by:
Manuel Sousa Pereira, Polytechnic Institute of Viana do Castelo, PortugalTze Wei Liew, Multimedia University, Malaysia
Copyright © 2024 Sae-tae, Ling and Wang. This is an open-access article distributed under the terms of the Creative Commons Attribution License (CC BY). The use, distribution or reproduction in other forums is permitted, provided the original author(s) and the copyright owner(s) are credited and that the original publication in this journal is cited, in accordance with accepted academic practice. No use, distribution or reproduction is permitted which does not comply with these terms.
*Correspondence: Qian Wang, d2FuZ19xaWFuQHNqdHUuZWR1LmNu