- 1Psychology Department, Northumbria University, Newcastle upon Tyne, United Kingdom
- 2Abertay cyberQuarter, School of Design and Informatics, Abertay University, Dundee, United Kingdom
- 3Open Lab, School of Computing, Newcastle University, Newcastle upon Tyne, United Kingdom
Background: Health-related stigma can act as a barrier to seeking treatment and can negatively impact wellbeing. Comparing stigma communication across different conditions may generate insights previously lacking from condition-specific approaches and help to broaden our understanding of health stigma as a whole.
Method: A sequential explanatory mixed-methods approach was used to investigate the prevalence and type of health-related stigma on Twitter by extracting 1.8 million tweets referring to five potentially stigmatized health conditions and disorders (PSHCDs): Human Immunodeficiency Virus (HIV)/Acquired Immunodeficiency Syndrome (AIDS), Diabetes, Eating Disorders, Alcoholism, and Substance Use Disorders (SUD). Firstly, 1,500 tweets were manually coded by stigma communication type, followed by a larger sentiment analysis (n = 250,000). Finally, the most prevalent category of tweets, “Anti-Stigma and Advice” (n = 273), was thematically analyzed to contextualize and explain its prevalence.
Results: We found differences in stigma communication between PSHCDs. Tweets referring to substance use disorders were frequently accompanied by messages of societal peril. Whereas, HIV/AIDS related tweets were most associated with potential labels of stigma communication. We found consistencies between automatic tools for sentiment analysis and manual coding of stigma communication. Finally, the themes identified by our thematic analysis of anti-stigma and advice were Social Understanding, Need for Change, Encouragement and Support, and Information and Advice.
Conclusions: Despite one third of health-related tweets being manually coded as potentially stigmatizing, the notable presence of anti-stigma suggests that efforts are being made by users to counter online health stigma. The negative sentiment and societal peril associated with substance use disorders reflects recent suggestions that, though attitudes have improved toward physical diseases in recent years, stigma around addiction has seen little decline. Finally, consistencies between our manual coding and automatic tools for identifying language features of harmful content, suggest that machine learning approaches may be a reasonable next step for identifying general health-related stigma online.
Introduction
Experiences of stigma can have a detrimental effect on the lives of people living with a range of health conditions and disorders. A recent study in the UK found that over half of participants living with a long-term health condition reported having experienced stigma associated with their condition (Brown et al., 2022a). Modern conceptualizations of stigma suggest that it is a social, cultural and moral phenomenon; a process typically involving labeling, negative stereotyping, linguistic separation (whereby the target of stigma is referred to as distinct from the person communicating the stigma), and with an asymmetry of power between those communicating and receiving stigma (Major and O'brien, 2005; Kleinman and Hall-Clifford, 2009; Andersen et al., 2022). Experiences of stigma can act as a barrier to sharing health information making individuals less likely to seek treatment and advice, which may inhibit them from receiving an appropriate level of care (Earnshaw et al., 2011; Sheehan and Corrigan, 2020; Simpson et al., 2021; Brown et al., 2022b). Experiences of health stigma have also been found to negatively impact employment, income and can have adverse economic effects (Sharac et al., 2010).
Stigma can accompany conditions or disorders that are associated with lifestyle behaviors. The concept of “lifestyle diseases” has been strongly criticized because it can incorrectly allocate both blame and choice to those who experience ill health (Whyte, 2016). Nevertheless, assumptions are frequently made about an individual's lifestyle in the presence of ill health, which can assign individual responsibility for the development of the condition or disorder (Seeberg and Meinert, 2015). The perceived origin of a condition is key to health stigma, and centers on the construct of onset controllability (Pachankis et al., 2018). For example, health conditions relating to alcohol and other substance use disorders may be considered stigmatizing due to the association of their origin with chosen lifestyle behaviors around drinking. Human Immunodeficiency Virus (HIV) is a long-term health condition that remains highly stigmatized in society, as does Acquired Immunodeficiency Syndrome (AIDS). Pachankis et al. (2018), when evaluating an extensive range of potentially stigmatizing conditions, found that alcoholism, drug dependency and HIV status were all rated high in controllable origin. In previous decades, public policy and media discourse cultivated a stigmatizing narrative around HIV and AIDS as a sexually transmitted, fatal disease associated with lifestyle choices (Khan, 2020). Typically held stereotypes about the lifestyle of people living with HIV and AIDS were that they are likely to be homosexual men, prostitutes or drug users (Earnshaw et al., 2012). Eating disorders have also been considered potentially stigmatized due to their association with lifestyle behaviors. For example, Anorexia Nervosa has been linked to lifestyle factors such as dietary habits, pursuit of the “thin ideal” (Zipfel et al., 2015), and a need for control (Branley-Bell et al., 2023). Extreme “Pro-ana” (pro-anorexia) groups may have exacerbated stigma by suggesting that Anorexia is a lifestyle choice in place of an illness (Richardson and Cherry, 2011). Bulimia and Binge Eating Disorder are also often associated with lifestyle choices such as intentional overeating and desired weight loss (Mehler and Rylander, 2015; Hutson et al., 2018). Finally, type 2 Diabetes may be considered potentially stigmatizing as it is frequently classified as a lifestyle disease, often being associated with factors such as laziness and poor dietary choices (Browne et al., 2013). Experiences of health stigma can be very hurtful and can have a damaging impact on long-term health and wellbeing (Entwistle, 2008; Clair et al., 2016; Lawrence et al., 2022).
Existing research into health stigma has typically adopted a siloed approach in which specific conditions and disorders are investigated separately (Stangl et al., 2019). This approach has stifled comparisons between stigmatized conditions, potentially limiting the broader understanding of health stigma as a whole (Pachankis et al., 2018; Stangl et al., 2019). This siloed approach to health stigma research means that the insights drawn from investigating condition-specific stigma are not always brought to the attention of academics and public health communicators working to address stigma in other areas (Millum et al., 2019). Therefore, jointly investigating and comparing potentially stigmatized conditions may offer additional insights previously lacking from condition-specific approaches. For example, such comparisons may provide knowledge concerning potential differences between physical, mental, and behavioral conditions, with respect to instances of stigma. This may help to develop interventions aimed at tackling health stigma with a more informed application across multiple conditions.
In order to investigate the true impact of health stigma, it is vital to understand how it is communicated. Smith's Model of Stigma Communication describes four types of content relevant to stigma communication: Marks, Labels, Responsibility, and Peril (Smith, 2007, 2011, 2014). There are parallels between the modern conceptualization of stigma provided above and Smith's Model of Stigma Communication. For example, using labels to refer to a particular social group is employed to denote both what stigma is and how it is communicated. Stigma is described as a process involving the separation of those assigning and receiving stigma (such that the receivers of stigma are “othered” from the dominant social group). Stigma communication conveys this separation by referring to the negative features of the “othered” group. This is communicated by highlighting the societal peril caused by the stigmatized group and the responsibility they carry for belonging to this group. With respect to the Model of Stigma Communication, Marks describe potential ways to identify members of a stigmatized group. To be most effective, marks should be visible and unsightly features of health, so that they can be identified rapidly. These may include clearly visible facial marks, a notable physical movement or tic, such as those associated with Tourette's syndrome (Smith, 2007). Labels are terms used to refer to a group. Labels often present the danger associated with a group by arousing social cognitions, such as considering the identified persons as a distinct group and encouraging stereotypes. For example, instead of stating that a person has epilepsy, the use of a label may refer to an individual as an “epileptic,” denoting that the person is the disease and a member of a separate group (Smith, 2007). Responsibility is content that describes a person's own agency, and assigns choice and blame for belonging to a certain stigmatized group. Responsibility may even suggest that a person voluntarily decided to deviate from social norms to engage in taboo activities. For example, someone living with a sexually transmitted infection may experience stigma from others who attribute individual responsibility for the causal origin of the infection (Yoo and Jang, 2012). Finally, Peril is content that describes the physical or social threat to a community's functioning. Peril often highlights painful, fatal, or socially taboo consequences of belonging to a stigmatized group. For example, HIV/AIDS may be stigmatized by portraying experiences of pain and death, associated with sexual promiscuity or injecting illegal drugs. Smith's model has been recently extended for the purpose of identifying health stigma on Twitter to highlight additional features such as experiences of self-stigma (i.e., feeling negative attitudes toward oneself, or about one's condition), wishing harm upon others, and generally seeking to devalue the lives of those living with a particular condition (Robinson et al., 2019; Bacsu et al., 2022).
Today, much of the public communication and discussion about health conditions and the associated stigma occurs on social media platforms. This article focusses on health messages posted on the social media platform Twitter (X). Twitter was recently rebranded as “X,” however in this article we refer to “Twitter” and “tweets” because data were collected between March and May 2022, prior to the rebranding in July 2023 (BBC News, 2023). Twitter is a widely used social media platform on which users can communicate their thoughts and opinions on almost any topic, including those associated with potentially stigmatizing health conditions. Twitter is therefore a relevant communicative context for investigating health stigma, providing researchers with an ideal source of both quantitative and qualitative data (Kim et al., 2021). Health researchers have used Twitter to collect large quantities of potentially stigmatizing messages associated with a number of health concerns, including mental health disorders (Robinson et al., 2019), dementia (Bacsu et al., 2022), HIV pre-exposure prophylaxis treatment (Schwartz and Grimm, 2017), and eating disorders (Arseniev-Koehler et al., 2016; Talbot and Branley-Bell, 2022). Many health stigma studies have chosen to qualitatively analyze samples of tweets to identify themes, categories and content features associated with specific types of stigma (Reavley and Pilkington, 2014; Bacsu et al., 2022; Najafizada et al., 2022). Using sentiment analysis and other forms of natural language processing can also provide insights into the patterns of health-related stigma by considering the language features of a tweet and whether or not content characteristics vary by condition. Social media platforms such as Twitter have come under heavy criticism for potentially incentivizing the spread of provocative or polarizing content (Branley and Covey, 2017; Rathje et al., 2021). Further investigation of tweets containing health stigma may help to understand the degree to which potentially stigmatizing narratives are present online. This may help health communicators to “cut through the noise” by providing accurate and effective health messaging to counter harmful and potentially stigmatizing content.
The aim of this study is to investigate Twitter discourse around potentially stigmatizing health conditions and disorders (PSHCDs). We will examine the prevalence of different types of stigma, and explore differences and/or similarities between PSHCDs. Furthermore, by conducting a sentiment analysis of tweets, we will compare natural language processing approaches to identifying potential features of health stigma communication online with manual coding conducted by subject experts. Finally, a deeper qualitative analysis of tweets will look to contextualize and explain the presence of stigma communication online. Therefore, our research questions are as follows: (1) What are the most prevalent types of stigma communication among tweets that refer to PSHCDs, and are there differences between conditions; (2) Using natural language processing, do tweets about PSHCDs that are manually coded as potentially stigmatizing differ from non-stigmatizing tweets in terms of sentiment and toxicity; and (3) What themes of discourse feature among the most prevalent categories of stigma communication.
Method
We conducted a mixed methods study into health stigma on Twitter by extracting tweets referring to five PSHCDs (Human Immunodeficiency Virus (HIV)/Acquired Immunodeficiency Syndrome (AIDS), Diabetes, Eating Disorders, Alcoholism, and Substance Use Disorders). These health conditions and disorders were selected because of their perceived associations with lifestyle behaviors (see above). People living with conditions associated with lifestyle behaviors may experience stigma due to external perceptions of the controllability of the origin of their condition or disorder (Pachankis et al., 2018). We manually coded a subset of tweets by stigma communication type, using natural language processing to analyze sentiment and other language features of tweets, and thematically analyzing an additional subset of tweets to further contextualize and explain health stigma communication online. Our study was approved by the Department of Psychology Ethics Committee at Northumbria University (ethical approval number 52832). For a flowchart of study phases, see Figure 1.
Twitter data
The R package “academictwitteR” was used to extract tweets via Twitter's API (Barrie and Ho, 2021). This package requires accredited access to Twitter's “Academic Research Product Track v2” which allows academic researchers to search the full history of public Tweets (Twitter, 2022). Tweets associated with each PSHCD were extracted using a pre-determined list of search terms (see Supplementary Table S1). This list of terms was created by first consulting the Medical Subject Headings (MeSH) thesaurus (National Library of Medicine, 2021) to find common terms related to each of the five target PSHCDs (HIV/AIDS, Diabetes, Eating Disorders, Alcoholism, and Substance Use Disorders). For each PSHCD, we consulted previous research and subject experts within the research team to refine our list of search terms. For example, in the UK, the four most common categories of eating disorder are Bulimia Nervosa, Anorexia Nervosa, Binge Eating Disorder, and Other Specified Feeding or Eating Disorder (OSFED) (Priory Group, 2022). Therefore, the names for these categories of eating disorder were included as additional search terms, along with related words and expressions identified by previous research (Branley and Covey, 2017). Group discussion and consultation with subject experts within the research team aided further refinement of search terms.
We extracted English language tweets published during March-May of 2022 without territory restrictions for the origin of tweets. This time period was chosen to avoid major international awareness days and campaigns for each of the five PSHCDs. Such dates were avoided to limit the effect of intermittent spikes in twitter activity on the prevalence and content of health-related tweets. We extracted 1,841,375 tweets in total: HIV/AIDS = 568,632 tweets, Diabetes = 496,614 tweets, Alcoholism = 339,391 tweets, Substance Use Disorders = 239,056 tweets, and Eating Disorders = 197,682 tweets. Due to limits to both researcher time and computational power, random subsets of the extracted tweets were created to enable further analysis. A subset of 1,500 tweets (300 per PSHCD) was created for manual coding by stigma communication type. A subset of 250,000 (50,000 tweets per PSHCD) was created for further analysis using natural language processing.
Analysis
Mixed methods approach
We adopted a sequential explanatory mixed-methods approach. First, we manually coded tweets by stigma type. We then conducted a sentiment analysis of the coded tweets to enable a comparison between the manual coding of stigma communication types and automatic sentiment analysis. A further sentiment analysis of the larger subset of tweets was then conducted to compare differences in language features between conditions. Finally, thematic analysis was used to explain and contextualize earlier findings by qualitatively investigating the prevalent category of coded tweets (Anti-Stigma and Advice; see section “Manual Coding” below). This follows previous theoretical guidelines for mixed-methods research in which sequential explanatory designs allow researchers to utilize a qualitative approach to expound former findings (Bishop, 2015; Creswell and Clark, 2017). For example, a related mixed-methods approach has previously been used to study mental health stigma on Twitter (Pavlova and Berkers, 2020).
Manual coding
To manually code tweets by stigma communication type, we created a Stigma Communication Type Codebook (see Supplementary Table S2). This codebook was informed by prior theoretical research. First, we included categories from Smith's Model of Stigma Communication: Marks, Labels, Responsibility, and Peril (Smith, 2007, 2011, 2014). After discussions among the full research team, additional categories were included to capture common features of Twitter communication, i.e., insults, entertainment and advice, as well as the role that stigma can play in devaluing the lives of those with a particular health condition or disorder (Robinson et al., 2019; Bacsu et al., 2022). A draft codebook was assessed by the research team by applying it to an initial subset of the extracted Twitter data (n = 100 tweets). During this initial assessment of tweets, researchers identified a notable presence of messages aimed at combatting online health stigma. This included content that referred to features of stigma as an attempt to counter existing narratives or to inform and advise the public on the relevant PSHCD. Due to the prevalence of this category, and its relevance to health stigma communication, the research team decided to add it as a stigma communication type. This additional category was defined as Anti-Stigma and Advice. The final codebook consisted of seven categories for coding tweets by stigma communication type (Labels, Marks, Responsibility, Peril, Insults, Entertainment, and Anti-Stigma and Advice).
Tweets were divided among five coders who applied the codebook to manually categorize tweets. Coders flagged tweets as irrelevant if they did not refer to a PSHCD and coded tweets as “No Stigma” where no stigma was present. If stigma was present, coders indicated the type(s) of stigma communication within the tweet and selected the main type represented. Finally, coders were free to code tweets as “other” and to define further categories. For each coder, 15% of their sample of tweets was also coded by a second researcher, pursuant to guidelines for ensuring inter-rater reliability among coders (Syed and Nelson, 2015). From this sample of twice-coded tweets, Krippendorff's alpha scores were calculated, α = 0.83 indicating an acceptable level of interrater reliability (Lombard et al., 2010).
An a priori power analysis indicated that a sample of 1,120 tweets would be sufficient to detect a small to medium effect size of 0.15 for a Chi-square test with power = 0.8, significance level = 0.05, df = 32, to investigate whether or not there is a statistically significant relationship between PSHCD and stigma communication type. After irrelevant and uncategorizable tweets were removed, our manually coded sample consisted of 1,288 tweets.
Quantitative analysis
All quantitative analyses were performed using R (R Core, 2021). The following packages were used for data processing, analysis, and visualization: academictwitteR (Barrie and Ho, 2021), devtools (Hadley et al., 2021), dplyr (Wickham et al., 2022), effectsize (Ben-Shachar et al., 2020), forcats (Wickham, 2021), ggplot2 (Wickham, 2016), ggrepel (Slowikowski, 2018), krippendorffsalpha (Hughes, 2021), peRspective (Votta, 2021), plotly (Sievert, 2020), plyr (Wickham et al., 2022), psych (Revelle, 2021), pwr (Champely et al., 2018), tidyr (Wickham and RStudio, 2021), tidytext (Silge and Robinson, 2016), tidyverse (Wickham et al., 2019), and wordcloud2 (Lang and Chien, 2022).
Wordclouds and n-grams were generated to identify common terms and language features among tweets referring to different PSHCDs. Sentiment scores were calculated to determine whether sentiment differs between tweets manually coded as stigmatized, not stigmatized, or anti-stigma. Further analysis was conducted to investigate differences in sentiment between PSHCDs using the larger sample of tweets (see Figure 1). Sentiment scores were calculated for tweets using an existing Opinion Lexicon (Hu and Liu, 2004) which categorizes approximately 6,800 words as positive or negative. Sentiment scores are calculated by determining the number of words contained within a tweet that are positive or negative according to the Opinion Lexicon. In a comprehensive comparison of approaches to sentiment analysis, Van Atteveldt et al. (2021) found Hu and Liu's lexicon to have the highest correlation with their human coded “gold standard” criteria for sentiment analysis, compared to other “off-the-shelf ” dictionaries.
Additionally, we generated language feature scores using Perspective API to compare our manual coding of health stigma with an automatic tool commonly used for identifying harmful content online. An increasing number of machine learning solutions are being offered to help monitor and combat harmful online content. Google's Perspective API processes online text and provides scores for a range of attributes potentially relevant to stigma communication, most notably “Toxicity,” “Identity Attack,” “Threat,” “Sexual Explicit,” “Insult,” and “Profanity.” By automatically generating scores for these dimensions, researchers have used Perspective API to study hate speech and other harmful content, social use of language, and online behaviors on Twitter (Jiang and Vosoughi, 2020; Narayanan, 2020; Aleksandric et al., 2022). We compare these dimensions among tweets manually coded as stigmatized, not stigmatized, or anti-stigma to explore the suitability and effectiveness of automatic tools for identifying potential features of health stigma communication.
Qualitative analysis
Reflexive Thematic Analysis (Braun and Clarke, 2019) was used to analyze tweets manually categorized as, “Anti-Stigma and Advice” (n = 273). This category of stigma communication was included in the Stigma Communication Type Codebook due to its notable presence among health-related stigma content (see section “Manual Coding” above). Our manual coding of tweets found Anti-Stigma and Advice to be the most prevalent category from our codebook. Therefore, researchers decided to conduct a qualitative analysis of this category of tweets in order to contextualize and explain this finding, pursuant to a sequential explanatory mixed-methods approach (Bishop, 2015; Cresswell et al., 2019). Thematic analysis has previously been used to analyze tweets associated with a range of stigmatized health conditions such as diabetes (Blackwood et al., 2022) and mental health disorders (Berry et al., 2017; Jansli et al., 2022). In our study, one researcher examined and analyzed tweets using NVivo 12 to create an initial thematic structure. This hierarchical structure of descriptive headings and subheadings was used to compare themes across all PSHCDs. Initial themes and representative tweets were discussed among the full research team to create the final thematic framework collaboratively.
Gold (2020) notes the importance of recognizing that Twitter data is unlike many datasets used for secondary analysis in that it is dynamic. Users may choose to delete previously posted tweets or remove their account entirely. Producing verbatim extracts of tweets in research makes it possible to connect tweets to individual users through internet search engines. Despite tweets being available in the public domain, we employed a process of anonymization to avoid any potential unwanted identification of tweet authors. Twitter handles and potentially identifying information were removed from tweets. Furthermore, when citing representative tweets in our thematic analysis, message content was altered and reconstructed where necessary in order to (1) ensure that the user could not be identified, and (2) ensure that the message accurately represented the original tweet. This follows previous guidelines in qualitative research whereby direct quotations may be paraphrased to hide idiosyncratic speech patterns (Social Research Association, 2021). This protection of user identity is pursuant to the British Psychological Society's “Ethics guidelines for internet-mediated research” which highlights the variety of expectations around data privacy online, and indicates the need to protect individuals posting or referred to in tweets (British Psychological Society, 2021).
Results
Our results are divided by the three phases of our analytic procedure (see Figure 1). First, we report the findings from our manual coding of stigma communication types. Second, we report the results from our sentiment analysis of both our manually coded sample of tweets, and larger subset of tweets. Finally, we present the findings from our thematic analysis of the most prevalent category from our manual coding of tweets, “Anti-Stigma and Advice.”
Manual coding of tweets by stigma communication type
A chi-square test of independence was performed to examine the relationship between PSHCD and the main category of stigma identified for each tweet. The relation between these variables was significant, x2 (32, n = 1,288) = 192.15, p = < 0.001. Of the 1,288 included in our manual coding, 43% (n = 562) were coded as not containing potentially stigmatizing content, 21% were considered to be “Anti-Stigma or Advice,” and the remaining 35% (n = 453) were divided among our defined categories of stigma communication (see Figure 2).
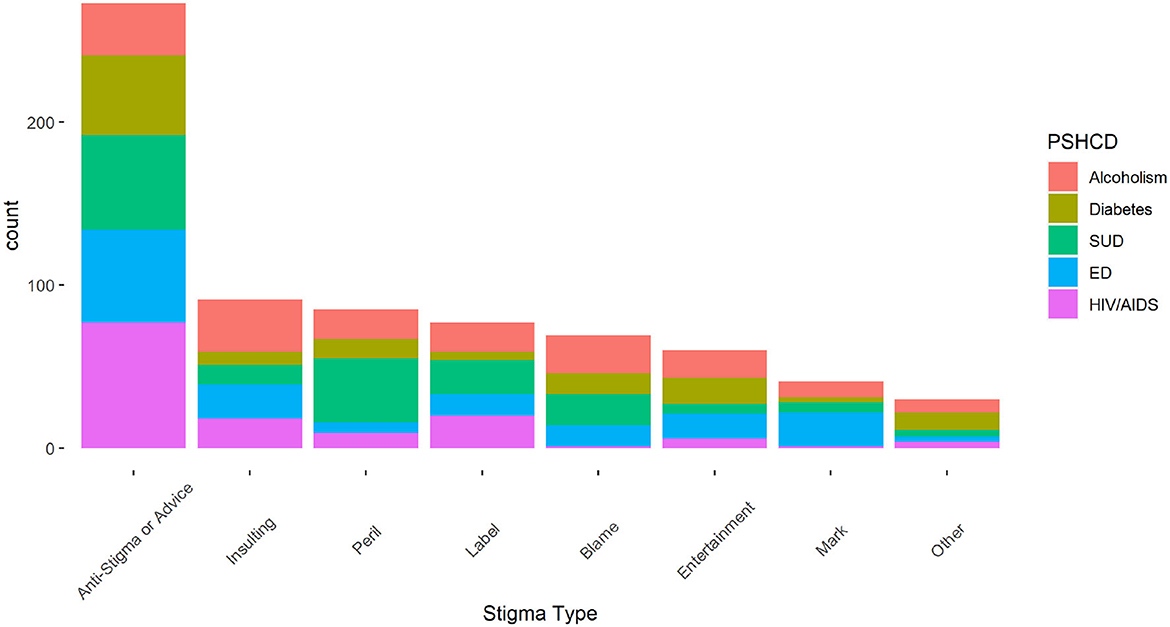
Figure 2. Number of manually coded tweets for each stigma communication type by condition (n = 726 tweets).
Of the tweets identified by researchers as potentially stigmatizing (n = 453), the percentage of tweets containing “Labels” of stigma was highest among those referring to HIV/AIDS (33.90% of HIV/AIDS tweets). The portion of tweets containing “Marks” of stigma was highest for tweets referring to eating disorders (22.58%). “Responsibility” was highest among tweets referring to diabetes (19.12%), “Entertainment” was also highest among diabetes tweets (23.53%), “Insults” among HIV/AIDS-related tweets (30.51%) and “Peril” among substance use disorder tweets (36.45%; see Figure 3 below).
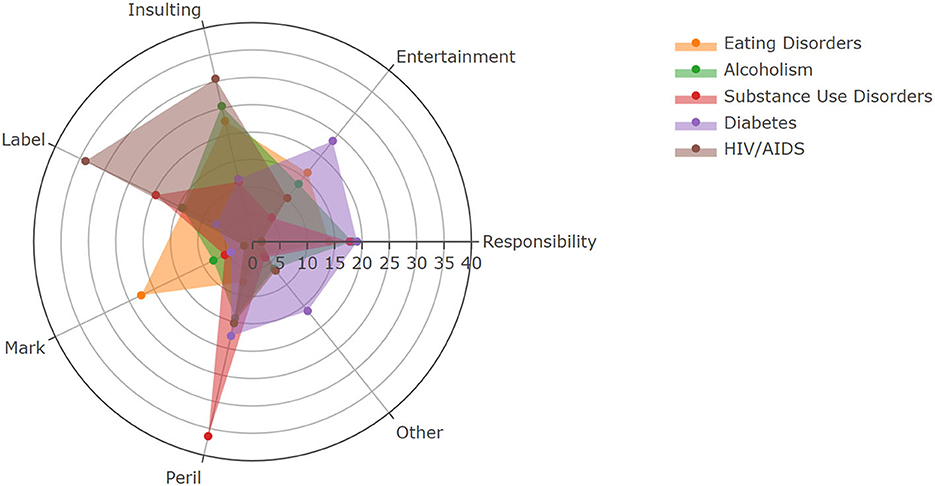
Figure 3. Radar chart showing the percentage of tweets containing each stigma communication type for each condition's sample, excluding non-stigmatizing tweets and anti-stigma (n = 453).
Sentiment analysis of manually coded tweets
Of the manually coded tweets (n = 1,288) the mean sentiment score was −1.76 (SD = 2.65, range = −13 to 8) indicating a negative sentiment. A one-way ANOVA was performed to compare sentiment scores across tweets manually coded as containing stigma, no stigma, or anti-stigma and advice. The ANOVA indicated a statistically significant difference in sentiment score between these groups of tweets [F(2, 1, 285) = 43.003, p < 0.001]. Mean tweet sentiment scores were lowest for tweets manually coded as containing stigma (M = −2.65, SD = 2.15), compared to tweets coded as “No Stigma” (M = −1.36, SD = 2.26) and “Anti-Stigma or Advice” (M = −1.11, SD = 3.59; see Figure 4 below).
A MANCOVA test was conducted to investigate differences in six Perspective API language dimensions for tweets manually coded as containing stigma, no stigma, or anti-stigma and advice. The Pillai's Trace statistic was significant [Pillai's Trace = 0.21, F(2, 1, 285) = 24.89, p < 0.001], suggesting an overall difference in Perspective API scores between groups of tweets. The measure of effect size (Partial Eta Squared; ηp2) is 0.10 suggesting there is a small effect of manually coded tweet group on Perspective API scores. Mean scores for five of the six generated Perspective dimensions were highest for tweets manually coded as containing stigma compared to “No Stigma” and “Anti-Stigma or Advice” (the exception being for scores for “Identity Attack”; see Figure 5 below).
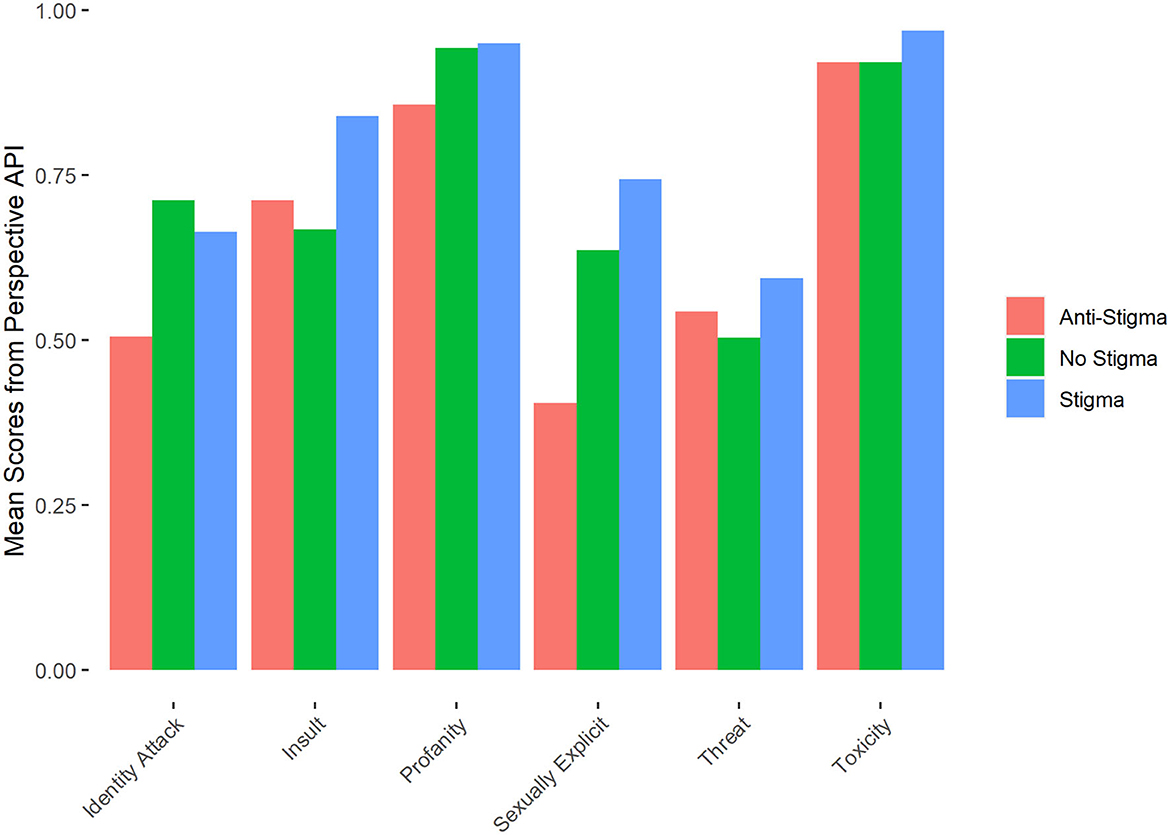
Figure 5. Bar chart showing differences in Perspective API dimension scores between groups of manually coded tweets (n = 1,288).
Comparison of sentiment scores across PSHCDs from the larger sample of tweets
A one-way ANOVA was performed to compare sentiment scores across the larger sample of tweets by PSHCD (n = 248,600). The ANOVA indicated a statistically significant difference in sentiment score between health conditions [F(4, 248, 595) = 5,911.3, p < 0.001]. Mean tweet sentiment scores were most positive for tweets referring to HIV/AIDS (M = 0.00, SD = 1.81), and least positive for tweets referring to substance use disorders (M = −1.69 SD = 2.07; see Figure 6 below). For an account of the frequency of the most common terms contained within tweets for each PSHCDs, see Supplementary Table S3 and Figures S1–S5.
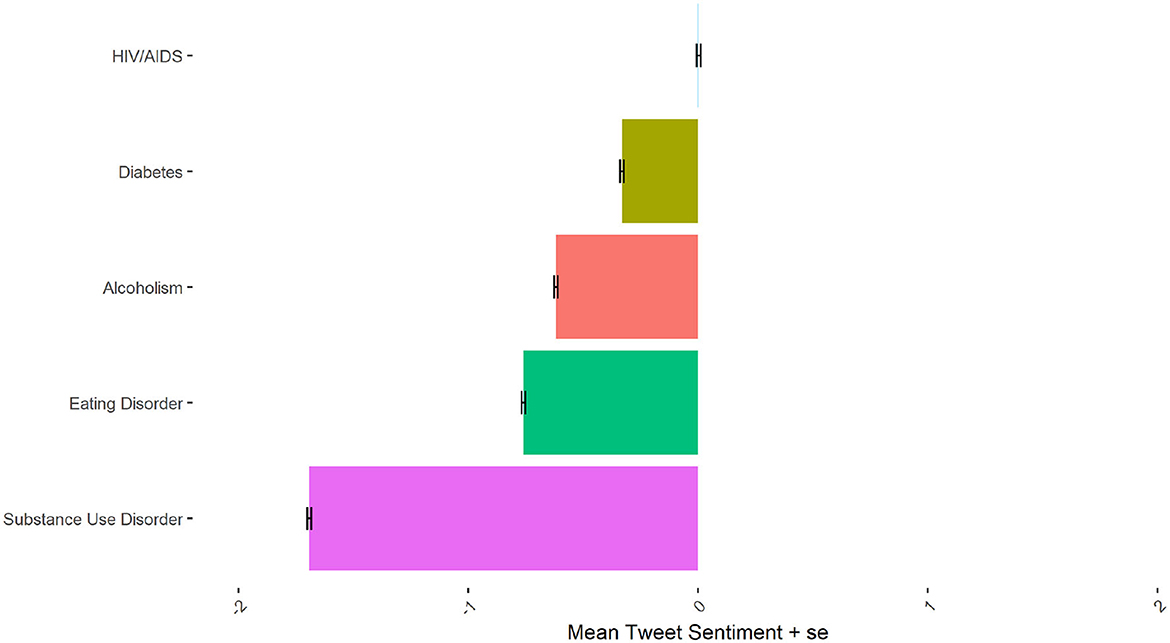
Figure 6. Mean sentiment scores for each PSHCD across the full quantitative sample of health tweets (n = 248,600).
Qualitative analysis
We report the findings from our reflexive thematic analysis of tweets manually coded as “Anti-Stigma and Advice” (n = 273). An overview of each theme, accompanied by representative tweets from our sample, are presented in Table 1. The prevalence of each theme across the five PSHCDs is reported in Table 2. Each theme is presented, defined and explained in further detail below.
Social understanding
This theme represents content aimed at combatting perceived stigma associated with PSCHDs by attempting to alter perspectives of ill health and personal responsibility for health. In particular, tweets challenged individual stereotypes associated with certain conditions, and looked to diminish the attribution of responsibility to those living with stigmatized conditions. Messages that attempted to improve the public's understanding of the experiences of those living with a PSHCD were most common among tweets about eating disorders. Tweets attempting to improve understanding by challenging popular stereotypes were exemplified most by content highlighting that PSHCDs are not restricted to a certain sex, age bracket, body shape or sexuality.
“Many PEOPLE suffer with eating disorders. Men and women. There is definitely a misogynistic part to the fatphobic diet industry, but men can suffer too. Fat people can have anorexia. It is not atypical—it is anorexia.”
“you can have an eating disorder at any weight and people of all sizes deserve treatment. when we perpetuate the idea that eating disorders have a specific look (usually young, thin, White women), we harm so many who suffer in silence.”
“The comments are really disturbing, HIV and other blood born viruses don't discriminate based on sexual orientation.”
Tweets referring to eating disorders challenged common associations between health and specific physiological characteristics (sex, age, body shape) whereas HIV/AIDS tweets often highlighted that the virus is not exclusive to homosexual men, arguably challenging established cultural stereotypes. In addition to separating experiences of ill health from the personal characteristics of individuals, tweets attempted to improve understanding of PSHCDs by detaching blame and responsibility from the individual.
“It's crazy- you should not be made to feel guilty about that. It's not like any of us WANT to be diabetic!”
“Treat hard drug addiction as a medical issue and not a criminal one.”
“Drug use doesn't equal addiction & addiction is a medical issue, not a moral failing.”
“Not a lifestyle choice, deal with the problems, don't punish! #substanceabuse.”
Though subsets of tweets for each PSHCD included content looking to counter messages of blame, this was most frequent when referring to substance use disorders. These tweets highlighted tensions between substance use disorders as a medical issue and the legal and societal frameworks around drugs. This tension is also found in tweets that stressed a need for change with respect to laws and attitudes toward addiction.
Need for change
This theme represents calls for change with respect to the degree of support given to people suffering from PSHCDs and the need for improved use of language when communicating about various conditions. Tweets about substance use disorders highlighted the myriad of causes that can lead to addiction and the importance of better provision and support.
“We need to do more to assist people whose lives are in danger from substance abuse. Many have chaotic lives and not the best start in life. We cannot turn our backs on them just because we don't agree with their lifestyle.”
“I reckon this tweet does a hell of a lot more to tackle the stigma of addiction by highlighting the history of drug use, the total hypocrisy of societal attitude to use of any substances, & the discriminatory drug laws across the world. More debate instigated by humour required.”
General calls for greater action to address structural causes of addiction were similar to the various rallying cries among the HIV/AIDS tweets seeking to limit the spread of the virus and improve treatment.
“Important we all pledge and commit to ending #stigma faced by people living with #HIV. Together, we can get to zero stigma and zero new HIV infections.”
“No more stalling. No more shifting responsibility. No more totally preventable HIV cases. However, this is not our end goal. We must simultaneously work towards finding PrEP a proper home in healthcare. It is now time to put aside the challenges of the last few years and collaborate.”
Finally, tweets about HIV/AIDS and Diabetes stressed the need for careful use of language when referring to a PSHCD and communicating with individuals about their health.
“What with the question ‘are you clean?'. I just had a shower, so I am clean thank you very much. Instead, use ‘what is your HIV status?'.”
“pointing out the hypocrisy of asking someone about one aspect of their medical history, apparently acceptable and necessary, when to ask that question about HIV would be inappropriate and insensitive.”
“Diabetes guru says in order to provide the best healthcare it's important to use the right language. Great resource for #Diabetes care #languagemstters.”
These tweets suggest the role that language can play in perpetuating stigma and highlight the importance of using terms mindfully for both individuals living with a specific condition, as well as for health communicators.
Encouragement and support
This theme captures messages that may inspire or encourage others either through celebrating instances of recovery or offering words of support to those living with PSHCDs. A common category of tweets across PSHCDs was that of celebrating recovery or successful management of health. These tweets often highlighted the ongoing nature of recovery and self-management of health, and focused on positive steps taken by the individual.
“I'm officially [number of days] self harm & emotional binge eating free!! it might not seem long but I've been struggling a lot & it takes a lot not to relapse! I'm v proud of myself !!”
“honestly I'm making so much progress here, still pretty much relapsing with anorexia but I'm working so much on myself.”
“I set out using Twitter as a personal journey diary. Was going to post my sobriety progress monthly to prove to myself. Figured if I could make it a year then I'm out of the woods as an alcoholic! I succeeded. And no longer need to keep track. thank you for your love.”
“20 years ago today my life changed, my family's life changed. Diabetes, you may be here for the long haul. But you ain't stopping me. Has it made me who I am? Yes. Would I change it? Yes, but no. #NeverGiveUp #Type1Diabetes.”
“It's been almost 21yrs, living with HIV. It's gone from, a terminal illness, to a manageable illness. I'm undetectable, which means, untransmittable.”
These tweets may contribute toward combatting health stigma by presenting cases where individuals have progressed toward a better state of health and improved their general wellbeing. These tweets frame the individual in a positive light and may dampen negative attitudes around conditions by highlighting the possibility of positive change. This positive sentiment was also present among tweets offering more general messages of support and encouragement to seek treatment.
“It is sad to find out young people died from OD… If you're in the same situation (i.e drug addict) please do go and get help. It's never too late x.”
“Alcoholism is progressive but so is Recovery.”
Information and advice
This theme captures messages that aimed to increase awareness of PSHCD campaigns and events, signpost services, and offer advice. This theme is most represented by tweets that highlight specific events or services and provide information about accessing more information and support. This was most featured among HIV/AIDS and Diabetes tweets, but was also present among other PSHCDs.
“HIV treatment and care is free in the U.K. and most part of Europe, regardless of your immigration status, sexual orientation and gender identity. It's important to find and join the appropriate HIV peer support group. We can assist you.”
“Are you worried you may have an eating disorder? Talk to your GP or read more information from Royal College of Psychiatrists.”
“Our last awareness session for diabetes awareness week so if your struggling to manage your diabetes join us to learn lifestyle management tips by health care professionals”
Finally, of the Diabetes Anti-Stigma and Advice tweets (n = 49) only 17 indicated which type of diabetes they referred to (Type 1 = 12 tweets, Type 2 = 5 tweets). Though it was common among the Anti-Stigma and Advice tweets to contain references to personal experiences of PSHCDs, when specifying diabetes type, 9/12 references to type 1 diabetes were made by the individual living with the condition, whereas this was 1/5 for type 2 diabetes, with most references not indicating personal experience by the author of the tweet.
“They are two very different illnesses. I'm Type 1 and when I see a rise in diabetes due to bad diet, it frustrates me.”
“Years of managing my own T1d helped me focus. Emotionally, was in bits. Now know best to let it all out and ask for help.”
“Here I show how helping #T2D patients cut sugar and starchy carbs helps reduce our use of drugs for diabetes in primary care.”
“Something to glean from fab info on how to reduce type 2 diabetes with a focus on BMI & weight loss, an area for community pharmacy to play a big part in, come on pharmacy need to listen to these guys more!”
This may suggest that people with type 1 diabetes are more willing to specify the category of condition they experience, compared to those living with type 2 diabetes. Alternatively, this may reflect that greater efforts are being made by health communicators to raise awareness of type 2 diabetes given the increased behavioral component compared to type 1.
Discussion
This study used a sequential explanatory mixed-methods approach to investigate the prevalence and type of health-related stigma communication on Twitter. Tweets were manually coded for stigma communication type. Key differences were found between PSHCDs in terms of the prevalence of stigma communication categories. We also found consistencies between our manual coding of stigma communication and natural language processing analysis that captured tweet sentiment, toxicity and related dimensions from Google's Perspective API. Finally, despite one-third of tweets being coded as containing potentially stigmatizing content, our sample of tweets demonstrated a strong presence of anti-stigma and advice. The key features of discourse that captured this category were Improving Understanding, Need for Change, Encouragement and Support, and Information and Advice.
Prevalence of health stigma communication on twitter
From our manually coded sample, almost half of all tweets were categorized by researchers as not containing any potentially stigmatizing content nor any anti-stigma content. A third of tweets contained some form of potentially stigmatizing content and the remainder (over one fifth of all tweets) comprised messages of anti-stigma and advice. It is notable that this category “Anti-Stigma or Advice” was more prevalent than any individual stigma communication type. By studying numerous health conditions within the same study, we were able to provide an indication of the prevalence of health-related stigma communication on Twitter. Best and Arseniev-Koehler (2023) have suggested that assessments of the prevalence of health-related stigma may have been obscured due to research typically only addressing those individual conditions and disorders that remain highly stigmatized (most notably mental illnesses such as schizophrenia). By investigating the prevalence of stigma across several conditions, we find that potentially stigmatizing content is notably present on Twitter, but less common than non-stigmatizing content. We also highlight the significant presence of tweets directed at countering health-related stigma on Twitter.
Commonalities and differences between PSHCDs in stigma communication types
Each of our defined stigma communication types were found across all PSHCDs, though the prevalence of each category varied by condition. This suggests that there are commonalities between PSHCDs in the communication of stigma. Recent research has suggested that “health-related stigma” should be studied as a viable concept in its own right, due to similarities in features of stigma across various conditions and disorders (van Brakel et al., 2019). Features of stigma communication are likely to be common across conditions because all forms of stigma share a common framework as a social phenomenon arising from shared perceptions and relationships throughout society (Pescosolido and Martin, 2015). Despite the expected commonalities, our manual coding revealed notable differences between PSHCDs in the prevalence of certain stigma communication types.
From those tweets manually coded by researchers as containing potentially stigmatizing content, labels of stigma were most prevalent among HIV/AIDS tweets. Our analysis of the larger subset of HIV/AIDS tweets found that “gay” was one of the most associated terms with this PSHCD. Previous research has found that gender, race, and sexual orientation often intersect with HIV-related stigma (Logie et al., 2011). Similarly, the percentage of tweets containing marks of stigma was highest among those referring to eating disorders. Further analysis of the larger subset of eating disorder tweets found “weight,” “fat,” and “skinny” to be among the most frequently tweeted terms associated with this category of PSHCD. The close association between eating disorders and marks of stigma (physically identifying characteristics) found within our sample may reinforce commonly held stereotypes concerning disordered eating. This is problematic because perpetuating stereotypes associated with eating disorders can lead to disparities in treatment where the individual does not have the “marks” most commonly associated with disordered eating (thin, white, female) (Head, 2019). Finally, over a third of potentially stigmatizing tweets that referred to substance use disorders alluded to the “peril” associated with this PSHCD. Further analysis of the larger subset of tweets referring to substance use disorder reported that terms such as “crime,” “criminal,” “homelessness” and references to money and family issues were common. Previous research has suggested that substance use disorders are typically discussed as moral and criminal issues, rather than as a health concern (Mattoo et al., 2015). Research has also connected substance use disorder with reports of “peril” due to perceptions of societal danger, and suggestions of “poor moral character” (Stringer and Baker, 2018). In addition to substance use disorder tweets being coded as containing greater peril and perceived danger, this category of tweets reported a markedly lower average sentiment score compared to other PSHCDs. A key component of substance use-related stigma previously used to explain the negative connotations associated with this PSHCD is “socially deviant” behavior (Millum et al., 2019). Furthermore, in an analysis of changes in health-related stigma since the 1980s, Best and Arseniev-Koehler (2023) suggest that most physical diseases have experienced a marked decline in negative connotations, whereas mental illnesses, eating disorders, and addiction have seen little change in levels of stigma. Individual activism and informational campaigns are suggested to explain some, but not all, of the variation in condition-related stigma (Best and Arseniev-Koehler, 2023).
Anti-stigma and advice
To contextualize and explain the notable prevalence of “Anti-Stigma and Advice” within our manually coded sample of tweets, we conducted a thematic analysis to identify common features within this category. We identified the themes Social Understanding, Need for Change, Encouragement and Support, and Information and Advice. We found that alcoholism and substance use disorders were the least represented disorders among tweets categorized as anti-stigma or advice. As described above, conditions and disorders often associated with addiction and “socially deviant” behaviors have seen little decline in public stigma in recent years (Best and Arseniev-Koehler, 2023). Whereas, HIV/AIDS related tweets contained the highest portion of anti-stigma and most positive sentiment compared to other PSHCDs.
Tweets that represented attempts to improve public understanding of PSHCDs were most common when referring to eating disorders. Messages often highlighted that eating disorders are not restricted to those of a particular age bracket, sex or body shape and stressed the damage that can come from perpetuating such stereotypes. This message of anti-stigma appears to be in response to the reported “marks” of stigma communication commonly associated with eating disorders. Though the prevalence of this category of anti-stigma among tweets referring to eating disorders is positive, our finding that stigma “marks” are prevalent among tweets about this PSHCD suggests that continued efforts are required to combat stereotypes online. A previous evaluation of past and present approaches to stigma change divided strategies into attempts to “protest,” “educate,” and “contact” (Corrigan and Penn, 1999; Corrigan, 2016). We consider our themes for defining anti-stigma and advice in light of this theoretical categorization. Our theme Social Understanding coincides with Corrigan's “education” category—attempts to decrease stigmatizing myths of ill health and combatting stereotypes by presenting facts. The category “protest,” which highlights calls to suppress thoughts of moralizing health issues and disrespecting those that suffer from ill health, also overlaps with our theme “Need for Change.” Despite lower reports of anti-stigma among substance use-related tweets, there were notable calls for a need for change with respect to attitudes toward this PSHCD. These calls highlighted the need for greater efforts to address the structural causes of ill health and to ensure that addiction is viewed as a medical, not moral issue. Corrigan's final category “contact” (attempts to eradicate stigma through interactions between people living with a specific condition and the broader public) differs somewhat from our remaining themes Encouragement and Support, and Information and Advice.
Messages of encouragement and support featured across PSHCDs, often celebrating an individual's recovery or successful management of health. This echoes previous research into communication about eating disorders which highlighted the common presence of “Pro-Recovery” content online that looks to share and inspire recovery (Branley and Covey, 2017). However, the most common theme from our sample of anti-stigma and advice was “Information and Advice.” van Brakel et al. (2019) previously suggested that information-based approaches are the most common strategy to counteracting public stigma associated with any condition. Public health campaigns and activism are suggested to be effective in contributing toward the decline of stigma associated with certain conditions (Best and Arseniev-Koehler, 2023).
Automatic tools for identifying potential features of stigma communication
To compare the effectiveness of automatic tools for identifying potential language features of stigmatizing health content, we divided our manually coded sample into tweets categorized as stigmatizing, not stigmatizing, or anti-stigma and advice. We found that stigmatizing tweets were more negative in sentiment and higher in scores for five out of six of Perspective APIs dimensions for identifying harmful speech. This provides an initial indication that automatic tools may offer a possible means for assisting in the identification of general health-related stigma at a larger scale. In a detailed comparison of manual and automatic approaches to analyzing online text, Van Atteveldt et al. (2021) determined that the best performance for measuring the sentiment of text is still achieved by human coders, compared to lexicon approaches and machine learning (ML). However, numerous attempts have been made in recent years to identify health-related stigma associated with specific conditions online using ML approaches. Oscar et al. (2017) used a supervised ML approach to classify Alzheimer's disease stigma on Twitter. Similarly, Budenz et al. (2020) used a ML model to classify stigma on Twitter associated with bipolar disorder. Most recently, Jilka et al. (2022) provided a proof of principle supervised ML model for identifying schizophrenia stigma on Twitter. This suggests that ML approaches may be effective in identifying health stigma at a large scale, which may prove useful for measuring the success of attempts to reduce online stigma. To our knowledge, no attempts have been made to apply ML approaches to identifying general health-related stigma online. The consistency between our automatic sentiment analysis and manual coding may suggest that ML models should be an appropriate next step for attempting to classify general health-related stigma online.
Limitations
The results of this study are not without limitation. Firstly, we extracted tweets published during March-May of 2022, however this extraction was conducted in August 2022. The delay between the publication and extraction of tweets may have affected our data. For example, during this period of delay, tweets may have been censored by twitter administrators, accounts removed, or content deleted by users in response to public comment. This may have prevented researchers from identifying certain aspects of health stigma communication present on Twitter in real time. Furthermore, we manually coded single tweets in isolation of replies and retweets. This may have limited researchers from being able to accurately interpret the context of tweets. However, users often passively scroll through their twitter feeds when consuming social media (Song et al., 2021), suggesting that it is typical of Twitter users to read a tweet without fully understanding the surrounding context. Therefore, it is possible that an assessment of individual tweets in isolation reflects the standard interaction between users and content on Twitter. Our data were manually coded by researchers and subject experts relevant to our chosen PSHCDs. However, instances of stigma communication may be interpreted differently by those living with a particular health condition. Recent research has suggested the need for pools of specialized raters (consisting of members from marginalized communities) when annotating content used to design automatic tools for identifying harmful content online (Goyal et al., 2022). It is important that future research captures the perspectives of those living with a range of potentially stigmatized conditions in order to refine our understanding of health stigma communication. Additionally, colloquial terms referring to health conditions, especially those related to alcohol and drug use, are diverse and rapidly evolving. While researchers attempted to include a variety of search terms to capture a comprehensive sample of tweets, some might have been overlooked. Future studies could benefit from in-depth consultations with individuals who have lived experience of specific conditions, ensuring a broader spectrum of search terms is used to extract online messages.
Finally, it is important to question the extent to which the views expressed in our sample of tweets are representative of broader online narratives. We did not apply territory restrictions during data collection. However, our English language requirement resulted in the majority of tweets coming from the US and UK, limiting the generalizability of our findings to other populations. Furthermore, online health narratives can differ between social media platforms. For example, attitudes toward COVID-19 vaccinations, and the corresponding arguments for and against, differ between platforms (Wawrzuta et al., 2022). The Pew Research Center have reported that the 22% of American adults who use Twitter are representative of the nation's broader population in certain ways, but not in others (Wojcik and Hughes, 2019). For example, they reported that Twitter users are generally younger and more likely to be Democrats than the general public. Furthermore, a large majority of tweets (80%) come from a small minority of tweeters (10%). Given the prevalence of health-related stigma communication on Twitter, future research might look to conduct a detailed comparison of the prevalence and types of stigma communication in non-English language populations, and to explore the differences across social media platforms.
Conclusion
This study investigated the prevalence and type of stigma communication among health-related tweets. We found that each of our defined categories of stigma communication were present across all PSHCDs, though there were notable differences between conditions. From our sample of potentially stigmatizing tweets, those referring to substance use disorders were frequently accompanied by messages of societal peril. Whereas, HIV/AIDS related tweets were most associated with reference to potential labels of stigma communication (such as sexual orientation). Sentiment scores for substance use disorder tweets were more negative than any other PSHCD, reflecting recent suggestions that, though negative connotations associated with physical diseases have diminished in recent years, stigma around addiction has seen little decline. Despite one third of health-related tweets being manually coded as potentially stigmatizing by researchers, we found a notable presence of content directed at counteracting online stigma. Our thematic analysis found that themes related to providing “Information and Advice” and “Social Understanding” were common across PSHCDs. Finally, the consistency between automatic tools for identifying features of harmful text online and our manual coding of stigma communication, suggests that ML approaches may be a reasonable next step for identifying general health-related stigma online.
Data availability statement
The data that support the findings of this study are available from Twitter (X)'s application programming interface. Restrictions apply to the availability of these data. Data are available from Twitter (X) at https://developer.twitter.com/en/products/twitter-api with the permission of Twitter (X).
Ethics statement
The studies involving humans were approved by Northumbria University, Psychology Department Ethics Committee. The studies were conducted in accordance with the local legislation and institutional requirements. Written informed consent for participation was not required from the participants or the participants' legal guardians/next of kin in accordance with the national legislation and institutional requirements.
Author contributions
RB: Conceptualization, Formal analysis, Methodology, Project administration, Visualization, Writing—original draft, Writing—review and editing. ES: Conceptualization, Supervision, Writing—original draft, Writing—review and editing. LC: Conceptualization, Funding acquisition, Supervision, Writing—review and editing. DB-B: Conceptualization, Supervision, Writing—review and editing. CM-M: Conceptualization, Investigation, Writing—review and editing. AD: Conceptualization, Funding acquisition, Supervision, Writing—review and editing.
Funding
The author(s) declare financial support was received for the research, authorship, and/or publication of this article. This research was supported by funding from EPSRC (EP/R033900/2 and EP/T022582/1).
Conflict of interest
The authors declare that the research was conducted in the absence of any commercial or financial relationships that could be construed as a potential conflict of interest.
Publisher's note
All claims expressed in this article are solely those of the authors and do not necessarily represent those of their affiliated organizations, or those of the publisher, the editors and the reviewers. Any product that may be evaluated in this article, or claim that may be made by its manufacturer, is not guaranteed or endorsed by the publisher.
Supplementary material
The Supplementary Material for this article can be found online at: https://www.frontiersin.org/articles/10.3389/fcomm.2023.1264373/full#supplementary-material
References
Aleksandric, A., Singhal, M., Groggel, A., and Nilizadeh, S. (2022). Understanding the bystander effect on toxic Twitter conversations. arXiv. [preprint]. doi: 10.48550/arXiv.2211.10764
Andersen, M. M., Varga, S., and Folker, A. P. (2022). On the definition of stigma. J. Eval. Clin. Pract. 28, 847–853. doi: 10.1111/jep.13684
Arseniev-Koehler, A., Lee, H., McCormick, T., and Moreno, M. A. (2016). # Proana: pro-eating disorder socialization on Twitter. J. Adolesc. Health 58, 659–664. doi: 10.1016/j.jadohealth.2016.02.012
Bacsu, J.-D., Fraser, S., Chasteen, A. L., Cammer, A., Grewal, K. S., Bechard, L. E., et al. (2022). Using Twitter to examine stigma against people with dementia during COVID-19: infodemiology study. JMIR Aging 5, e35677. doi: 10.2196/35677
Barrie, C., and Ho, J. C.-t. (2021). academictwitteR: an R package to access the Twitter academic research product track v2 API endpoint. J. Open Source Softw. 6, 3272. doi: 10.21105/joss.03272
Ben-Shachar, M. S., Lüdecke, D., and Makowski, D. (2020). effectsize: estimation of effect size indices and standardized parameters. J. Open Source Softw. 5, 2815. doi: 10.21105/joss.02815
Berry, N., Lobban, F., Belousov, M., Emsley, R., Nenadic, G., and Bucci, S. (2017). # WhyWeTweetMH: understanding why people use Twitter to discuss mental health problems. J. Med. Internet Res. 19, e6173. doi: 10.2196/jmir.6173
Best, R. K., and Arseniev-Koehler, A. (2023). The stigma of diseases: unequal burden, uneven decline. Am. Sociol. Rev. 80. doi: 10.1177/00031224231197436
Bishop, F. L. (2015). Using mixed methods research designs in health psychology: an illustrated discussion from a pragmatist perspective. Br. J. Health Psychol. 20, 5–20. doi: 10.1111/bjhp.12122
Blackwood, L., Gavin, J., Arnott, E., Barnett, J., Dack, C., and Johansen, J. (2022). # DiabetesOnAPlate: the everyday deployment and contestation of diabetes stigma in an online setting. Crit. Public Health 33, 1–14. doi: 10.1080/09581596.2022.2077548
Branley, D. B., and Covey, J. (2017). Pro-ana versus pro-recovery: a content analytic comparison of social media users' communication about eating disorders on Twitter and Tumblr. Front. Psychol. 8, 1356. doi: 10.3389/fpsyg.2017.01356
Branley-Bell, D., Talbot, C. V., Downs, J., Figueras, C., Green, J., McGilley, B., et al. (2023). It's not all about control: challenging mainstream framing of eating disorders. J. Eat Disord. 11, 25. doi: 10.1186/s40337-023-00752-9
Braun, V., and Clarke, V. (2019). Reflecting on reflexive thematic analysis. Qual. Res. Sport Exerc. Health 11, 589–597. doi: 10.1080/2159676X.2019.1628806
British Psychological Society (2021). Ethics Guidelines for Internet-mediated Research. Leicester: British Psychological Society. doi: 10.53841/bpsrep.2021.rep155
Brown, R., Coventry, L., Sillence, E., Blythe, J., Stumpf, S., Bird, J., et al. (2022a). Collecting and sharing self-generated health and lifestyle data: understanding barriers for people living with long-term health conditions – a survey study. Digit. Health 8, 20552076221084458. doi: 10.1177/20552076221084458
Brown, R., Sillence, E., Coventry, L., Simpson, E., Gibbs, J., Tariq, S., et al. (2022b). Understanding the attitudes and experiences of people living with potentially stigmatised long-term health conditions with respect to collecting and sharing health and lifestyle data. Digit. Health 8, 20552076221089798. doi: 10.1177/20552076221089798
Browne, J. L., Ventura, A., Mosely, K., and Speight, J. (2013). ‘I call it the blame and shame disease': a qualitative study about perceptions of social stigma surrounding type 2 diabetes. BMJ Open 3, e003384. doi: 10.1136/bmjopen-2013-003384
Budenz, A., Klassen, A., Purtle, J., Tov, E. Y., Yudell, M., and Massey, P. (2020). Mental illness and bipolar disorder on Twitter: implications for stigma and social support. J. Ment. Health 29, 191–199. doi: 10.1080/09638237.2019.1677878
Champely, S., Ekstrom, C., Dalgaard, P., Gill, J., Weibelzahl, S., Anandkumar, A., et al (2018). Package ‘pwr'. R package version, 1(2). Available online at: https://nyuscholars.nyu.edu/en/publications/pwr-basic-functions-for-power-analysis
Clair, M., Daniel, C., and Lamont, M. (2016). Destigmatization and health: cultural constructions, the long-term reduction of stigma. Soc. Sci. Med. 165, 223–232. doi: 10.1016/j.socscimed.2016.03.021
Corrigan, P. W. (2016). Lessons learned from unintended consequences about erasing the stigma of mental illness. World Psychiatry 15, 67–73. doi: 10.1002/wps.20295
Corrigan, P. W., and Penn, D. L. (1999). Lessons from social psychology on discrediting psychiatric stigma. Am. Psychol. 54, 765. doi: 10.1037/0003-066X.54.9.765
Cresswell, K., McKinstry, B., Wolters, M., Shah, A., and Sheikh, A. (2019). Five key strategic priorities of integrating patient generated health data into United Kingdom electronic health records. J. Innov. Health Inform. 25, 254–259. doi: 10.14236/jhi.v25i4.1068
Creswell, J. W., and Clark, V. L. P. (2017). Designing and Conducting Mixed Methods Research. London: Sage publications.
Earnshaw, V. A., Quinn, D. M., and Park, C. L. (2011). Anticipated stigma and quality of life among people living with chronic illnesses. Chronic Illn. 8, 79–88. doi: 10.1177/1742395311429393
Earnshaw, V. A., Smith, L. R., Chaudoir, S. R., Lee, I.-C., and Copenhaver, M. M. (2012). Stereotypes about people living with HIV: implications for perceptions of HIV risk and testing frequency among at-risk populations. AIDS Educ. Prev. 24, 574. doi: 10.1521/aeap.2012.24.6.574
Entwistle, V. A. (2008). Hurtful comments are harmful comments: respectful communication is not just an optional extra in healthcare. Health Expectat. 11, 319. doi: 10.1111/j.1369-7625.2008.00527.x
Gold, N. (2020). Using Twitter Data in Research: Guidance for Researchers and Ethics Reviewers. Department of Computer Science, UCL. Available online at: https://www.ucl.ac.uk/data-protection/sites/data-protection/files/using-twitter-research-v1.0.pdf
Goyal, N., Kivlichan, I. D., Rosen, R., and Vasserman, L. (2022). Is your toxicity my toxicity? Exploring the impact of rater identity on toxicity annotation. Proc. ACM Hum.-Comput. Interact. 6(CSCW2), 1–28. doi: 10.1145/3555088
Hadley, W., Hester, J., and Chang, W. (2021). devtools: Tools to Make Developing R Packages Easier. R package version 2.4.0. Available online at: https://CRAN.R-project.org/package=devtools (accessed July 1, 2023).
Head, M. (2019). (In) visible: an examination of eating disorder detection in marginalized women as a function of weight status and ethnic group membership (Electronic Thesis and Dissertation Repository). University of Western Ontario. Available online at: https://ir.lib.uwo.ca/etd/6208
Hu, M., and Liu, B. (2004). “Mining and summarizing customer reviews,” in Proceedings of the Tenth ACM SIGKDD International Conference on Knowledge Discovery and Data Mining, 168–177.
Hughes, J. (2021). krippendorffsalpha: an R package for measuring agreement using Krippendorff's alpha coefficient. arXiv. [preprint]. doi: 10.48550/arXiv.2103.12170
Hutson, P. H., Balodis, I. M., and Potenza, M. N. (2018). Binge-eating disorder: clinical and therapeutic advances. Pharmacol. Ther. 182, 15–27. doi: 10.1016/j.pharmthera.2017.08.002
Jansli, S. M., Hudson, G., Negbenose, E., Erturk, S., Wykes, T., and Jilka, S. (2022). Investigating mental health service user views of stigma on Twitter during COVID-19: a mixed-methods study. J. Ment. Health 31, 576–584. doi: 10.1080/09638237.2022.2091763
Jiang, J., and Vosoughi, S. (2020). “Not judging a user by their cover: understanding harm in multi-modal processing within social media research,” in Proceedings of the 2nd International Workshop on Fairness, Accountability, Transparency and Ethics in Multimedia (New York, NY: ACM), 6–12. doi: 10.1145/3422841.3423534
Jilka, S., Odoi, C. M., van Bilsen, J., Morris, D., Erturk, S., Cummins, N., et al. (2022). Identifying schizophrenia stigma on Twitter: a proof of principle model using service user supervised machine learning. Schizophrenia 8, 1–8. doi: 10.1038/s41537-021-00197-6
Khan, S. (2020). Examining HIV/AIDS-related stigma at play: power, structure, and implications for HIV interventions. Health Commun. 35, 1509–1519. doi: 10.1080/10410236.2019.1652386
Kim, N. J., Lin, J., Hiller, C., Hildebrand, C., and Auerswald, C. (2021). Analyzing US tweets for stigma against people experiencing homelessness. Stigma Health 8, 187–195. doi: 10.1037/sah0000251
Kleinman, A., and Hall-Clifford, R. (2009). Stigma: a social, cultural and moral process. J. Epidemiol. Community Health 63, 418–419. doi: 10.1136/jech.2008.084277
Lang, D., and Chien, G. (2022). wordcloud2: Create Word Cloud by htmlWidget. R package version 0, 2.1. R Package. Available online at: https://cloud.r-project.org/web/packages/wordcloud2/wordcloud2.pdf
Lawrence, S. E., Puhl, R. M., Schwartz, M. B., Watson, R. J., and Foster, G. D. (2022). “The most hurtful thing I've ever experienced”: a qualitative examination of the nature of experiences of weight stigma by family members. SSM-Qual. Res. Health 2, 100073. doi: 10.1016/j.ssmqr.2022.100073
Logie, C. H., James, L., Tharao, W., and Loutfy, M. R. (2011). HIV, gender, race, sexual orientation, and sex work: a qualitative study of intersectional stigma experienced by HIV-positive women in Ontario, Canada. PLoS Med. 8, e1001124. doi: 10.1371/journal.pmed.1001124
Lombard, M., Snyder-Duch, J., and Bracken, C. C. (2010). Practical Resources for Assessing and Reporting Intercoder Reliability in Content Analysis Research Projects. Philadelphia, PA: Temple University. Available online at: https://www.researchgate.net/profile/Cheryl-Bracken/publication/242785900_Practical_Resources_for_Assessing_and_Reporting_Intercoder_Reliability_in_Content_Analysis_Research_Projects/links/0deec52e14791a0d6f000000/Practical-Resources-for-Assessing-and-Reporting-Intercoder-Reliability-in-Content-Analysis-Research-Projects.pdf
Major, B., and O'brien, L. T. (2005). The social psychology of stigma. Annu. Rev. Psychol. 56, 393–421. doi: 10.1146/annurev.psych.56.091103.070137
Mattoo, S. K., Sarkar, S., Gupta, S., Nebhinani, N., Parakh, P., and Basu, D. (2015). Stigma towards substance use: comparing treatment seeking alcohol and opioid dependent men. Int. J. Ment. Health Addict. 13, 73–81. doi: 10.1007/s11469-014-9514-1
Mehler, P. S., and Rylander, M. (2015). Bulimia Nervosa–medical complications. J. Eat. Disord. 3, 1–5. doi: 10.1186/s40337-015-0044-4
Millum, J., Campbell, M., Luna, F., Malekzadeh, A., and Karim, Q. A. (2019). Ethical challenges in global health-related stigma research. BMC Med. 17, 1–9. doi: 10.1186/s12916-019-1317-6
Najafizada, M., Rahman, A., Donnan, J., Dong, Z., and Bishop, L. (2022). Analyzing sentiments and themes on cannabis in Canada using 2018 to 2020 Twitter data. J. Cannabis Res. 4, 1–14. doi: 10.1186/s42238-022-00132-1
Narayanan, S. (2020). “Challenges in fighting hate speech, false propaganda and abuse on Twitter in Indian context,” in False Propaganda and Abuse on Twitter in Indian Context (January 31, 2020). doi: 10.2139/ssrn.3539292
National Library of Medicine (2021). Introduction to the Medical Subject Headings (MeSH) Thesaurus. Available online at: https://www.nlm.nih.gov/mesh/introduction.html (accessed July 1, 2023).
Oscar, N., Fox, P. A., Croucher, R., Wernick, R., Keune, J., and Hooker, K. (2017). Machine learning, sentiment analysis, and tweets: an examination of Alzheimer's disease stigma on Twitter. J. Gerontol. B Psychol. Sci. Soc. Sci. 72, 742–751. doi: 10.1093/geronb/gbx014
Pachankis, J. E., Hatzenbuehler, M. L., Wang, K., Burton, C. L., Crawford, F. W., Phelan, J. C., et al. (2018). The burden of stigma on health and well-being: a taxonomy of concealment, course, disruptiveness, aesthetics, origin, and peril across 93 stigmas. Pers. Soc. Psychol. Bull. 44, 451–474. doi: 10.1177/0146167217741313
Pavlova, A., and Berkers, P. (2020). Mental health discourse and social media: which mechanisms of cultural power drive discourse on Twitter. Soc. Sci. Med. 263, 113250. doi: 10.1016/j.socscimed.2020.113250
Pescosolido, B. A., and Martin, J. K. (2015). The stigma complex. Annu. Rev. Sociol. 41, 87. doi: 10.1146/annurev-soc-071312-145702
Priory Group (2022). Eating Disorder Statistics. Available online at: https://www.priorygroup.com/eating-disorders/eating-disorder-statistics (accessed July 1, 2023).
R Core (2021). Core R: A Language and Environment for Statistical Computing. Vienna: R Foundation for Statistical Computing.
Rathje, S., Van Bavel, J. J., and Van Der Linden, S. (2021). Out-group animosity drives engagement on social media. Proc. Nat. Acad. Sci. 118, e2024292118. doi: 10.1073/pnas.2024292118
Reavley, N. J., and Pilkington, P. D. (2014). Use of Twitter to monitor attitudes toward depression and schizophrenia: an exploratory study. PeerJ 2, e647. doi: 10.7717/peerj.647
Revelle, W. (2021). psych: Procedures for Personality and Psychological Research (version R package version 2.0, 12.). Evanston, IL: Northwestern University.
Richardson, A., and Cherry, E. (2011). “Anorexia as a choice,” in Embodied Resistance: Challenging the Norms, Breaking the Rules, eds C. Bobel, and S?. Kwan (Nashville, TN: Vanderbilt University Press), 119. doi: 10.2307/j.ctv16757rr.18
Robinson, P., Turk, D., Jilka, S., and Cella, M. (2019). Measuring attitudes towards mental health using social media: investigating stigma and trivialisation. Soc. Psychiatry Psychiatr. Epidemiol. 54, 51–58. doi: 10.1007/s00127-018-1571-5
Schwartz, J., and Grimm, J. (2017). PrEP on Twitter: information, barriers, and stigma. Health Commun. 32, 509–516. doi: 10.1080/10410236.2016.1140271
Seeberg, J., and Meinert, L. (2015). Can epidemics be noncommunicable? Med. Anthropol. Theory 2, 54. doi: 10.17157/mat.2.2.171
Sharac, J., Mccrone, P., Clement, S., and Thornicroft, C. (2010). The economic impact of mental health stigma and discrimination: a systematic review. Epidemiol. Psychiatr. Sci. 19, 223–232. doi: 10.1017/S1121189X00001159
Sheehan, L., and Corrigan, P. (2020). “Stigma of disease and its impact on health,” in The Wiley Encyclopedia of Health Psychology, eds K. Sweeny, M. L. Robbins, and L. M. Cohen (Hoboken, NJ: John Wiley & Sons Ltd), 57–65. doi: 10.1002/9781119057840.ch139
Sievert, C. (2020). Chapman and Hall/CRC; 2020. Interactive Web-Based Data Visualization with R, Plotly, and Shiny. New York, NY: Chapman and Hall/CRC. doi: 10.1201/9780429447273
Silge, J., and Robinson, D. (2016). tidytext: text mining and analysis using tidy data principles in R. J. Open Source Softw. 1, 37. doi: 10.21105/joss.00037
Simpson, E., Brown, R., Sillence, E., Coventry, L., Lloyd, K., Gibbs, J., et al. (2021). Understanding the barriers and facilitators to sharing patient-generated health data using digital technology for people living with long-term health conditions: a narrative review. Front. Public Health 9, 641424. doi: 10.3389/fpubh.2021.641424
Slowikowski, K. (2018). ggrepel: Automatically Position Non-overlapping Text Labels with “ggplot2.” R package version 0.8. 0 ed. R Package. Available online at: https://brieger.esalq.usp.br/CRAN/web/packages/ggrepel/ggrepel.pdf
Smith, R. A. (2007). Language of the lost: an explication of stigma communication. Commun. Theory 17, 462–485. doi: 10.1111/j.1468-2885.2007.00307.x
Smith, R. A. (2011). “Stigma, communication, and health,” in The Routledge Handbook of Health Communication, eds T. L. Thompson, R. Parrott, and J. F. Nussbaum (London: Routledge), 480–493.
Smith, R. A. (2014). Testing the model of stigma communication with a factorial experiment in an interpersonal context. Commun. Stud. 65, 154–173. doi: 10.1080/10510974.2013.851095
Song, K. W., Vivrekar, J., Yeom, L., Paulos, E., and Salehi, N. (2021). “Crank that feed: a physical intervention for active twitter users,” in Extended Abstracts of the 2021 CHI Conference on Human Factors in Computing Systems (New York, NY: ACM), 1–6. doi: 10.1145/3411763.3451817
Stangl, A. L., Earnshaw, V. A., Logie, C. H., van Brakel, W., Simbayi, L. C., Barré, I., et al. (2019). The Health Stigma and Discrimination Framework: a global, crosscutting framework to inform research, intervention development, and policy on health-related stigmas. BMC Med. 17, 1–13. doi: 10.1186/s12916-019-1271-3
Stringer, K. L., and Baker, E. H. (2018). Stigma as a barrier to substance abuse treatment among those with unmet need: an analysis of parenthood and marital status. J. Fam. Issues 39, 3–27. doi: 10.1177/0192513X15581659
Syed, M., and Nelson, S. C. (2015). Guidelines for establishing reliability when coding narrative data. Emerging Adulthood 3, 375–387. doi: 10.1177/2167696815587648
Talbot, C. V., and Branley-Bell, D. (2022). # BetterHealth: a qualitative analysis of reactions to the UK government's better health campaign. J. Health Psychol. 27, 1252–1258. doi: 10.1177/1359105320985576
Twitter (2022). Academic Research - Product Track Details. Available online at: https://developer.twitter.com/en/products/twitter-api/academic-research/product-details (accessed July 1, 2023).
Van Atteveldt, W., Van der Velden, M. A., and Boukes, M. (2021). The validity of sentiment analysis: comparing manual annotation, crowd-coding, dictionary approaches, and machine learning algorithms. Commun. Methods Meas. 15, 121–140. doi: 10.1080/19312458.2020.1869198
van Brakel, W. H., Cataldo, J., Grover, S., Kohrt, B. A., Nyblade, L., Stockton, M., et al. (2019). Out of the silos: identifying cross-cutting features of health-related stigma to advance measurement and intervention. BMC Med. 17, 1–17. doi: 10.1186/s12916-018-1245-x
Wawrzuta, D., Klejdysz, J., Jaworski, M., Gotlib, J., and Panczyk, M. (2022). Attitudes toward COVID-19 vaccination on social media: a cross-platform analysis. Vaccines 10, 1190. doi: 10.3390/vaccines10081190
Whyte, S. R. (2016). “Chronicity and control: framing ‘noncommunicable diseases' in Africa,” in Medical Anthropology in Europe, eds E. Hsu, and C. Potter (London: Routledge), 67–78.
Wickham, H. (2016). Package ‘ggplot2': Elegant Graphics for Data Analysis. New York, NY: Springer-Verlag.
Wickham, H. (2021). Forcats: Tools for Working with Categorical Variables (Factors) 2020. R Package Version 0.5. 0. Available online at: https://forcats.tidyverse.org/reference/forcats-package.html (accessed June 29, 2022).
Wickham, H., Averick, M., Bryan, J., Chang, W., McGowan, L., François, R., et al. (2019). Welcome to the Tidyverse. J Open Source Softw. 4, 1686. doi: 10.21105/joss.01686
Wickham, H., François, R., Henry, L., and Müller, K. (2022). RStudio.(2021). dplyr: A Grammar of Data Manipulation (1.0.7). R package. Available online at: https://cran.r-hub.io/web/packages/dplyr/dplyr.pdf
Wickham, H., and RStudio (2021). tidyr: Tidy Messy Data (1.1.3) [Computer Software]. R Package. Available online at: https://cran.utstat.utoronto.ca/web/packages/tidyr/tidyr.pdf
Wojcik, S., and Hughes, A. (2019). Sizing up Twitter users. Pew Res. Center 24, 1–23. Available online at: https://www.pewresearch.org/internet/2019/04/24/sizing-up-twitter-users/
Yoo, J. H., and Jang, S. (2012). An attributional analysis of stigma associated with sexually transmitted diseases and its relationship with communication efficacy. Glob. J. Health Sci. 4, 15. doi: 10.5539/gjhs.v4n4p15
Keywords: stigma, health communication, human computer interaction, long-term health conditions, social media, Twitter, X
Citation: Brown R, Sillence E, Coventry L, Branley-Bell D, Murphy-Morgan C and Durrant AC (2023) Health stigma on Twitter: investigating the prevalence and type of stigma communication in tweets about different conditions and disorders. Front. Commun. 8:1264373. doi: 10.3389/fcomm.2023.1264373
Received: 20 July 2023; Accepted: 29 September 2023;
Published: 13 October 2023.
Edited by:
Alexander Röhm, Technical University Dortmund, GermanyReviewed by:
Lindsay Sheehan, Illinois Institute of Technology, United StatesBryan Abendschein, Western Michigan University, United States
Copyright © 2023 Brown, Sillence, Coventry, Branley-Bell, Murphy-Morgan and Durrant. This is an open-access article distributed under the terms of the Creative Commons Attribution License (CC BY). The use, distribution or reproduction in other forums is permitted, provided the original author(s) and the copyright owner(s) are credited and that the original publication in this journal is cited, in accordance with accepted academic practice. No use, distribution or reproduction is permitted which does not comply with these terms.
*Correspondence: Richard Brown, cmljaGFyZDYuYnJvd24mI3gwMDA0MDtub3J0aHVtYnJpYS5hYy51aw==