- 1Curriculum and Instruction Department, Purdue University, West Lafayette, IN, United States
- 2Teacher Education and Learning Sciences Department, North Carolina State University, Raleigh, NC, United States
Interaction is an essential element of online learning and researchers had use Social Learning Analytics (SLA) to understand the characteristics of meaningful interaction. While the potential for network analysis in education (i.e., SLA) is valuable, limited research has considered how best to use this emerging field to inform meaningful interaction in online settings. Online learning researchers need a concise and simplified framework for SLA to support interaction in online learning environments. Therefore, we present a conceptual framework to make SLA accessible for researchers investigating learners' interactions in online learning. The framework includes concepts from network theory and the online learning literature integrated into a new perspective to analyze learners' online behaviors and interactions. We analyzed existing models and frameworks to show how network analysis has been used in online learning resulting in a conceptual environment to investigate learner interaction. The proposed i-SUN framework has four main steps: (1) interaction, (2) social presence alignment, (3) unit of analysis definition, and (4) network statistics and inferential analysis selection. We also identified five ways in which the i-SUN model contributes to the advancement of SLA in online interaction research and provide recommendations for empirical validation. As part of a sequence of manuscripts, we seek to offer a unique perspective to online learning researchers and practitioners by focusing on the social and pedagogical implications of applying network analysis to understand online learning interaction.
1. Introduction
Although online learning has been established as an effective learning mode compared to face-to-face learning (Pei and Wu, 2019), challenges to facilitating online learning persist. Instructors and learners perceive difficulties in meaningfully connecting with one another, establishing an effective presence, and developing communication strategies that support intentional relationships, and eventually, learning (Richardson et al., 2015). As learning is generally considered a social activity (Vygotsky, 1978b; Bruner, 1990; Bandura, 2002; Lowyck, 2014), these challenges can greatly impact student success in online environments. Learning analytics (LA) has been one way in which researchers consider the effectiveness of online learners' interactions in supporting student success by combining education and data science to inform instructional design (Ifenthaler and Yau, 2020).
The social nature of learning demands external support (i.e., scaffolding; Wood et al., 1976; Vygotsky, 1978a) and interaction (Moore, 1989) among instructors, peers, or the learning environment. Yet, the assumptions of traditional statistical analysis (i.e., independence of observations) limit research questions and can be misaligned with the interdependency that emerges in online learning. As a result, Social Learning Analytics (SLA) has emerged as a sub-field of LA, offering educators and researchers new opportunities for analyzing learning interactions from a perspective that reflects the interdependent nature of learning. SLA focuses on “understanding connectivity and the development of social relationships, and how this can be used to promote learning through social interaction” (De Laat and Prinsen, 2014). Ferguson and Buckingham Ferguson and Shum, 2012 explain that SLA is a socio-constructivist approach to learning analytics in which learning occurs through interaction and collaboration. However, previous research on interaction in online learning using SLA has relied primarily on descriptive analysis rather than inferential SLA (Jan et al., 2019). Inferential SLA analysis allows researchers to estimate learners' interactions over time (e.g., Zhang et al., 2016; Poquet and Jovanovic, 2020; Castellanos-Reyes, 2021) or statistically compare different types of learner networks (e.g., Kellogg et al., 2014). Nevertheless, SLA faces the interdisciplinary challenge of bridging the social network analysis field that rooted in graph theory and sociology with the educational research field. Lack of rigor in the implementation of SLA results in unsystematic reporting of network measures without sound theoretical foundation on what they mean within the learning context resembling to what Poquet and Joksimovic (2022) call a “cacophony of networks.”
Methodological approaches that acknowledge the social nature of learning are not enough to support meaningful connections in online learning because they lack the theoretical guidelines to identify which interactions are relevant for establishing a sense of community. Researchers and practitioners have used the social presence construct to understand affective interactions in online learning environments and design online learning experiences that foster such interactions (Fiock, 2020). Social presence posits those members of an online learning community can project themselves as real to others in the online environment (Swan, 2021). Traditionally, social presence interactions have been analyzed from a learner-learner point of view for mutual construction of meaning (e.g., Kyei-Blankson et al., 2019; Castellanos-Reyes, 2021; Lim, 2023). Yet, online learning environments allow for other types of interaction like those between learners and instructors, learners and content (Moore, 1989), learners and network (i.e., social media) (Dennen, 2013), and learners and the rules that govern the broader community (e.g., netiquette, curricula, institutional guidelines) (Jonassen and Rohrer-Murphy, 1999; Engeström, 2001; Yamagata-Lynch, 2010). It is the coherence between the methodological approach, in this case SLA, and the theoretical perspective of social presence that supports educational researchers to draw rigorous and sound conclusions. Thus, it is urgent to have guidelines for online learning researchers conducting SLA that are theoretically sound and contextualized to the complexity of distance education.
While the potential for using SLA and network analysis methods to investigate learning is great, limited research has considered how best to use this emerging field to inform evidence-based practices in online settings. Furthermore, existing frameworks on SLA in online learning focus only on learner-learner interaction to form online communities (e.g., Jan and Vlachopoulos, 2019) without accounting for other types of interaction like learner-interface. For example, collaborative annotation of textbooks for community development (Sun et al., 2023) or intelligent tutoring systems (Ebadi and Amini, 2022). One potential reason for limited research in this area is a lack of conceptual frameworks that can be used to guide the implementation of SLA and a subsequent robust research process. Online learning researchers and practitioners need a concise and simplified SLA framework to support interaction in online learning environments.
The multiplicity of network analysis terms combined with the lack of theoretical integration of online learning interaction and social presence theory compounds the challenge of implementing SLA. In response to the call for “refinement and rigor” in SLA (Poquet and Joksimovic, 2022), we propose a conceptual framework for educators and researchers to understand online learning interaction through SLA and social presence. To guide the development of this framework, we used concepts from communication networks theory and online learning literature integrated into a new perspective to analyze and assess learners' online behaviors and interactions. We first synthesize and critically evaluate the existing literature about SLA frameworks and interaction frameworks in relation to social presence. Then, we provide specific network analysis indicators for researching interaction from a social presence perspective. Next, we discuss the benefits and challenges of implementing the proposed SLA conceptual framework to investigate online learning interaction. Finally, we provide suggestions for future research that could serve as guidelines in this area for researchers.
2. Literature review
The literature review section is divided into five main sections. First, we define social presence in the online learning context. Then, we evaluate the foundational interaction theories and frameworks for online learning in the light of social presence. In the third section, we address recent frameworks in SLA specific to online learning. The last two sections include a table synthesizing the relationship between the frameworks and social presence to support SLA while providing recommendations on how research can use SLA measures.
2.1. Social presence in online learning
Grounding in the telecommunications field (Short et al., 1976), Gunawardena and Zittle (1997) introduced social presence to distance education as “the degree to which a person is perceived as real in mediated communication” (p. 9). This definition has been increasingly used in the online learning field by practitioners and researchers alike (Castellanos-Reyes, 2020) and incorporated as part of the Community of Inquiry (CoI) framework. The CoI is a constructivist process-model that describes the components of an online learning experience (Garrison, 2017a; Swan, 2021). Since its inclusion as part of the CoI, social presence has been widely used to research online learning and guide the design of high-quality learning experiences (Fiock, 2020). Nevertheless, the conceptualization of social presence among researchers is not without controversy. For example, Kreijns et al. (2022) challenged the conceptualization of social presence, arguing that researchers confound the social presence's definition with the technology affordances and the interpersonal connections among students.
Despite the definition of social presence being widely debated (Kreijns et al., 2022; Shea et al., 2022), we adhere to the definition of social presence as the extent to which online learners perceive themselves and others as “real people” (Garrison et al., 2000; Rourke et al., 2001; Shea et al., 2022). Social presence is theorized to include three components: open communication (e.g., replying on a discussion board), affective communication (e.g., expressing emotions or liking others' work), and cohesive responses (i.e., addressing peers by name) (Garrison, 2017b; Shea et al., 2022). Social presence is essential in online learning because it influences student satisfaction (Richardson et al., 2017) and performance (Garrison and Arbaugh, 2007; Cui et al., 2013; Joksimović et al., 2015). Social presence has been used to explain interaction in computer-mediated environments, for example, interactions among members of an online community and their interactions with the course activities in the learning experience (i.e., “meet your classmates” activity, discussion board, reading assignments) (Richardson and Swan, 2003).
2.2. Models of online learning interaction and their limitations
In this section, we surveyed prominent models of online learning interaction frameworks as indicated by the Handbook of Distance Education (Dennen, 2019). We reviewed three foundational interactions theories and frameworks in relation to online learning. First, Moore's theory of transactional distance (1998) because of its longstanding history to explain distance learning interaction (Bernard et al., 2009). Second, the Interaction Analysis Model (IAM) (Gunawardena et al., 1997) because it was conceptualized on text-based communication which was the foundation of distance education delivery (Stewart et al., 2023). Third, we introduce activity theory, which was initially conceptualized to understand complex learning environments. Although activity theory was conceptualized for traditional in-person education (Jonassen and Rohrer-Murphy, 1999; Engeström, 2001; Yamagata-Lynch, 2010). Dennen (2019) contextualized the theory to online learning environments, offering a framework for considering additional elements of the distance learning ecosystem. We guide our review based on the social presence construct. Table 1 synthesizes this section.
2.2.1. Moore's theory of transactional distance (1993)
Moore's theory aimed to explicitly identify interaction and clarify traditionally vague and ambiguous concepts such as distance, independence, and interaction (1989). Moore explained that transactional distance referred to the psychological and communications space that separates the learner and the instructor (Moore, 1993). Moore (1993) formulated the concept of transactional distance as a function of dialogue, instructional structure, and learners' autonomy. Further, he explained that transactional distance is greater in pre-recorded sessions with little learner-instructor dialogue, but internal dialogue among learners is greater. Conversely, transactional distance reduces in instructional programs designed as live virtual meetings where there's a two-way interaction between learners and instructors, with less structure and more learner-instructor dialogue. Based on this, Moore proposed three main types of interaction: learner-content, learner-instructor, and learner-learner interaction (1989). There is a need to balance the kind of interaction based on the learner's capacity and needs, the instructors' teaching philosophy, and the nature of the subject (Moore, 1993; Falloon, 2011). Scholars have expanded Moore's original concept of transactional distance to include the interaction between learner and the interface (Hillman et al., 1994) and learner and the network (Dennen, 2013). Specifically, learner-network interactions refer to those behaviors that occur external to the class environment (Dennen, 2019) like communicating with others outside the class who might share similar interests or expertise (Dennen, 2013).
Moore's theory does not address the affective and cohesive components of social presence. Instead, it adopts a learner-centered approach as a focus. In other words, how the individual learner interacts with content, instructor, and other learners, almost singularly or in a linear fashion. Such linearity restricts the complexity of an online learning experience, especially one that includes multiple means of communication. Moore (1993) acknowledges that highly interactive media allow for more intensive and dynamic dialogue. The social presence construct is a helpful lens to evaluate Moore's theory because it includes a community aspect that allows for a more dynamic and complex explanation of interaction in online learning.
2.2.2. Gunawardena et al. (1997) interaction analysis model
Gunawardena et al. (1997) made the case that quantitative participation analysis and self-reported satisfaction within an online learning conference environment are not sufficient for determining the quality of interaction and the quality of the learning experience. As such, they proposed content analysis or interaction analysis of transcripts as being essential to evaluating the quality of interaction. Interaction in this model (Gunawardena et al., 1997) is rooted in the learning sciences in the work of Jordan and Henderson (1995), who described interaction analysis as human-human interaction and human interaction with objects in their environment. Interaction is, therefore, an “ongoing social process” in which people collaborate, learn, and recognize what they have learned (Gunawardena et al., 1997, p. 403). There is not hierarchical relationship among participants in this model, and therefore, it is not teacher centered. Here, participants are equal in the hierarchy. Interaction is also viewed as informal and voluntary as the learning process naturally unfolds among participants, as a co-creative process rather than an assessment of student performance. Ultimately, interaction is described as a “totality of interconnected and mutually-responsive messages… ‘interaction' is the entire gestalt formed by the online communications among the participants...in relation to each other and in a manner which reflects each other's presence and influence” (Gunawardena et al., 1997, p. 407). Gunawardena (1995) adopts a multi-constructed approach to social presence in describing how important and “real” a person “feels” in mediated communication through intimacy (e.g., eye contact, nonverbal cues) and immediacy (e.g., psychological distance, relatability). In relation to the Interaction Analysis Model, social presence is a potential predictor that influences learning outcomes positively. However, interaction is not reflected between the learner and the content, nor is the relationship between the learner and the interface highlighted.
2.2.3. Activity theory in online learning
Activity theory allows researchers to explore learning as an activity system and describes the elements that influence learning (Dennen, 2019). Activity systems “support a systematic and systemic approach to understanding human activities and interactions in real-world complex environments” (Yamagata-Lynch, 2010, p. 1). In activity theory, complex learning environments are conceptualized as “natural situations where multiple individuals are involved in shared activities within a single or multi-organized context” (Yamagata-Lynch, 2010, p. viii). Originating from Vygotskys' theoretical perspective, activity theory was developed by Egeström and later adapted to the educational context by Yamata-Lynch (Dennen, 2019). Engeström (1987) represented activity theory with a triangular model in which the vertices show the components of the complex system, and the sides, the tensions among them.
Despite activity theory being originally conceptualized to understand organizational change in educational contexts from a systemic perspective and to guide the design of constructivist learning environments (Yamagata-Lynch, 2010). Dennen (2019) explained that “activity theory encourages the view that online classes are complex ecosystems” (p. 252) in which tension among parts prompts learning. The online learning activity system framework comprises tools, rules, a community (e.g., students, instructors), and a division of labor (see Figure 1). Yet, the main subject of the system is the student, and the expected outcome is learning (Dennen, 2019). Activity theory allows researchers to comprehensively account for online learning elements (Dennen, 2019). It gives researchers conceptual tools to understand the interconnections among components of the complex system (e.g., learners, instructors, administrators) and their networks of interaction (as well as the juxtapositions of their objects (i.e., outcomes). One of the limitations of activity theory is that its methodologies are focused on qualitative research, mainly because it is through qualitative inquiry that researchers can deeply understand the complexity of a learning system. Furthermore, Bedny and Karwowski (2004) argue that researchers' interpretation of the components of activity theory is ambiguous due to translation challenges and “conflicting schools of thought” (p. 135). The diverse interpretations have obscured the use of activity theory challenging researchers to use to explain learning. Yet, we posit that activity theory serves as an analytical framework to better reflect the potential of SLA in online learning interaction. Unlike earlier LA methodologies that rely on quantitative data, new methods that involve SLA (e.g., Social Epistemic Network Signature, Gašević et al., 2019) are not limited to quantitative data.
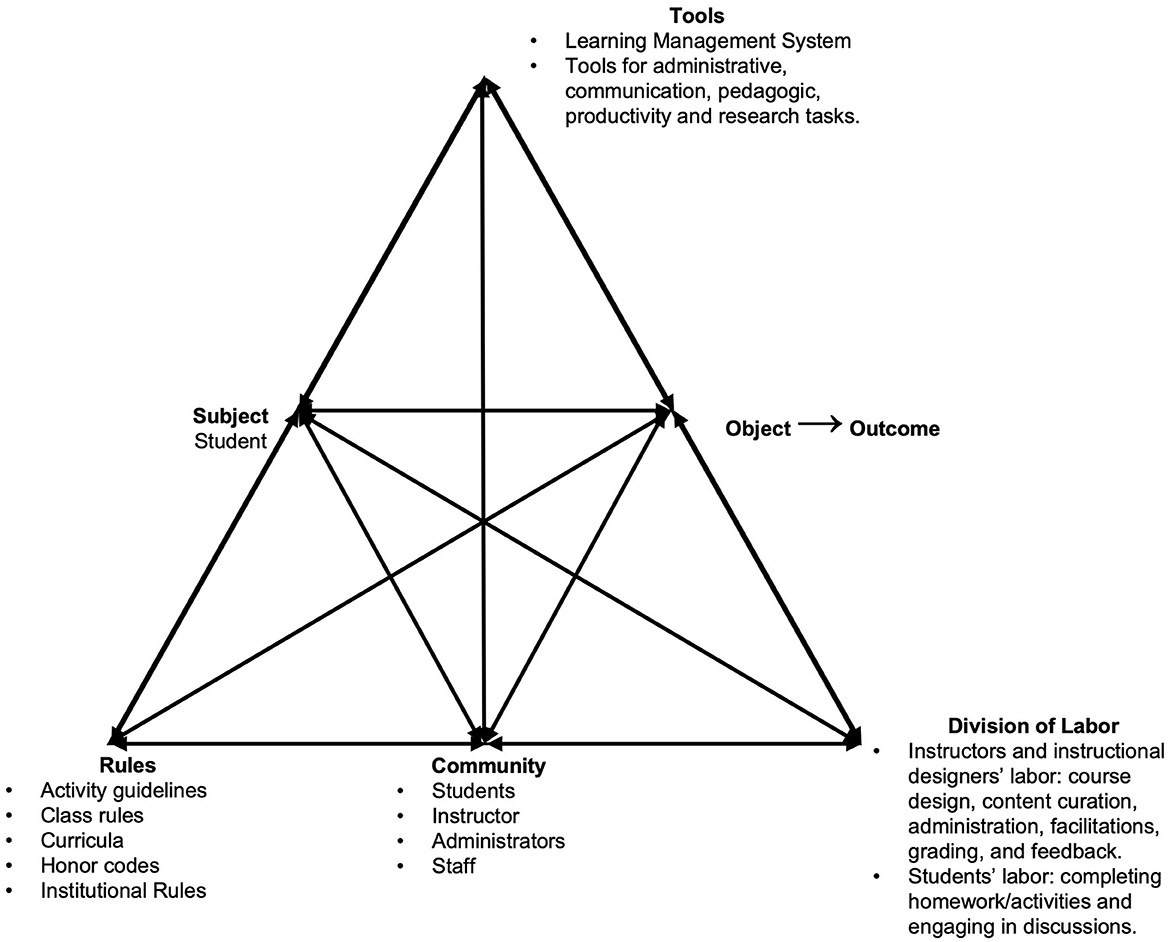
Figure 1. Adapted representation of Dennen's (2013) online learning as an activity system.
This framework considers social presence in terms of affective association and instructor investment since it explores learners' and instructors' individual and group constructs through interrelationships between tools, the subject, rules, community, and division of labor. However, the main gap exists in exploring the interface's functionality and how social presence is enabled in online environments through interaction intensity, cohesion within the community, and how affective outcomes are achieved. As such, exploration is needed to consider how the individual, and by extension, communities of learners interact with the interface to achieve the outcomes.
2.2.4. Limitations across interaction theories
The theory of transactional distance (Moore, 1993) is a valuable framework for examining interactions among online learners, their instructors, and the content, while activity theory (Engeström, 1987) is much broader and explores a systematic interplay of “tools, rules, people, and work” (p. 254) that comprise the online learning ecosystem. Within the traditional conceptualization of online learning (Stewart et al., 2023), the way people interact via text-based communication leads to an exchange of cultural insights, which in turn ignite knowledge as a collective social construction (Gunawardena et al., 1997; Gunawardena, 2013).
We propose that existing interaction theories do not fully reflect the complexity of online learning. On the one hand, transactional theory (Moore, 1989, 1993) focuses on interpersonal interaction and interaction with content. However, it does not include the interaction and tensions between other members of the online learning community (i.e., administrators and staff) and the available tools for online learning (i.e., learning management systems, external technology). On the other hand, the Interaction Analysis Model (Gunawardena et al., 1997) does not consider the interaction between the learner and the interface, as well as the learner and content. Furthermore, it relies on text-based interaction a as means of knowledge construction in online education. Yet, the adoption of Emergency Remote Teaching (ERT) (Hodges et al., 2020), leveraged synchronous communication as part of the response to the COVID-19 pandemic and the sustained and generalized use after (Stewart et al., 2023) showed the need to include other forms of delivery and interaction beyond text-based communication as central elements of online education. Finally, activity theory in online learning (Engeström, 1987; Yamagata-Lynch, 2010) presents a holistic, rich, qualitative approach to understanding interaction as a complex interrelation among learners, communities, tools, objects, and subjects. Still, there is a need to integrate facets of the online environment and how it affords interaction by including the online learning interface and other online interaction elements (i.e., network, rules, community) using SLA concepts. Therefore, the focus here is to share a conceptual framework that comprehensively reflects the interactions in online learning at (1) an interpersonal level and (2) the larger learning environment, while accounting for the potential of SLA and network analysis supported by the social presence construct.
2.3. Social learning analytics for online learning
Ifenthaler (2015, p. 447) defines LA as “the use, assessment, elicitation and analysis of static and dynamic information about learners and learning environments, for the real-time modeling, prediction and optimisation of learning processes, and learning environments, as well as for educational decision making.” LA in higher education has primarily focused on supporting student success (Sclater et al., 2016; Ifenthaler and Yau, 2020). For example, LA has been used to predict performance (Xing et al., 2015; Aulck et al., 2017), identify at-risk students (e.g., students who may dropout) (Aguiar et al., 2014; Cohen, 2017), analyze student dropout to support retention (Aguiar et al., 2014), or improve course design, and student engagement (Lockyer and Dawson, 2012). LA for online learning environments primarily uses learners' data (e.g., frequency of LMS logons, course resources downloads, or module accesses) to account for their navigation patterns, preferences, and behaviors (Ifenthaler and Widanapathirana, 2014). However, LA's potential has yet to be realized, as research commonly focuses on aggregated quantitative representations and does not fully consider the social dynamics necessary for meaningful learning in online environments. Furthermore, LA has been described as part of an “algorithmically pervaded society” (p. 17) in which theory has been discarded in place of data analysis (Knight and Buckingham Shum, 2017). Relying only on data analysis results in the under-exploration of the relationship between LA and its application and a lack of understanding of LA among educators and researchers (Drachsler and Greller, 2012). Viberg et al. (2018) found in a literature review on LA in higher education that despite the potential for LA to improve learning practice, very little LA application is realized in higher education practice.
Given that learning is inherently a social activity that requires external support (i.e., scaffolding) and interaction from instructors, peers, or the learning environment; a subfield of LA emerged —Social Learning Analytics (SLA). SLA offers educators and researchers new opportunities to overcome challenges in online teaching and learning like feelings of isolation and community building (Hart, 2012; Richardson et al., 2015) under the assumption that social learning contributes to the “quality of learning and student experiences” (Poquet and Joksimovic, 2022, p. 38). SLA focuses on understanding the interdependency of social relationships and how this can be used to promote learning through social interaction. SLA takes a socio-constructivist approach to learning, in which learning occurs through interaction and collaboration. As a part of SLA, researchers use network analysis to understand learners' interactions (Aviv et al., 2005) in online learning environments (Jan et al., 2019). Network analysis uses statistics and graph theory to study the relationships of entities (e.g., learners), assuming dependency on those with whom they connect and those within the same group (Monge and Contractor, 2003).
2.4. Conceptual frameworks for social learning analytics and their limitations
Next, we analyzed three main conceptual frameworks of SLA. To guide this process, we used the social presence construct to examine SLA frameworks from a perspective relevant to online learning. First, we begin with Ferguson and Shum's (2012) framework that defines the main elements of SLA. We use Buckingham and Shum's SLA framework, which pioneered the identification of the main elements of SLA, because (a) they propose an analytical approach that takes a social learning theory approach, (b) it includes the use of social networks incorporating the network element that Dennen (2019) adds to the transactional distance theory, and (c) it takes a community-centered approach which agrees with the social presence perspective of perceiving and projecting each other as real (Lang et al., 2022). Then, we analyzed Jan and Vlachopoulos's (2019) exploration of the concept of communities based on online discussion boards. Given the historical roots of text-based communication in distance learning, we included Jan and Vlachopoulos's (2019) framework that focuses on discussion boards and capitalizes on the socio-constructivist lens of social presence to apply network analysis to understand learning. Finally, we discuss interaction per Kent and Rechavi's (2020) framework as it pertains to usage in online environments because they account for students who are less active in discussion boards. Kent and Rechavi's (2020) perspective is essential to account for those unobserved interactions in online learning that still foster social presence perceptions. Table 2 synthesizes this section.
2.4.1. Ferguson and Shum (2012)
Ferguson and Shum (2012) recognized the importance of LA in improving learner outcomes, noting great potential arising from an “unprecedented” volume of data about learner activities and interests. Within a learning design space, the authors propose that SLA should be implemented to distinguish the concept and unique features of a social learning environment. Ferguson and Shum (2012) also suggested the need for defining the possibilities of SLA; as being inherently social (as a behavior and discourse analysis) or socialized (as an application to a broader setting; content, disposition, and context). Finally, the applications of SLA demand an ethical perspective by considering its limitations and abuses.
The underlying socio-cultural philosophy of Buckingham Shum and Ferguson draws on the distinction that SLA is a unique subset of LA that rests on the premise that novel skills and ideas are a result of interaction and collaboration. The authors maintain that five conditions or phenomena influence the learning context: (1) technology, (2) open access, (3) knowledge age skills, (4) social learning as a catalyst for innovation, and (5) challenges to educational institutions.
Social learning is characterized by “changing affordances” in which social activity occurs “at a distance, in mediated forms” (Ferguson and Shum, 2012, p. 8). Social learning occurs when intentions are clarified, learning is grounded, and learners are engaged in conversations to increase their understanding. Ferguson and Shum (2012) refined the conceptualization of SLA by specifying a five levels taxonomy: social network analytics, discourse analytics, content analytics, disposition analytics, and context analytics. From the social presence perspective, Buckingham Shum and Ferguson account only for the open communication element when addressing network analysis in SLA to investigate learners' interactions and relationship development, without considering affective communication or cohesive responses.
2.4.2. Jan and Vlachopoulos (2019)–Integrated methodological framework
Jan and Vlachopoulos (2019) proposed an Integrated Methodological Framework (IMF) that combines Community of Inquiry (CoI) and Community of Practice (CoP) frameworks through the lenses of SLA. The CoI is a constructivist process-model for collaborative discourse that integrates three “presences” that constitute a successful online learning experience (Garrison et al., 2000; Swan, 2021)–among those presences is social presence. A CoP includes a group of people with shared interests and different levels of expertise and interest in a shared domain (Wenger, 1998, 2004; Farnsworth et al., 2016) that relates closely to informal and professional learning experiences (Dennen, 2019). Although CoI and CoP differ in participants' expected level of commitment and participation–in which the former expects higher commitment and the latter encourages more autonomy–both are forms of collaborative and interdependent online learning (Dennen, 2019). After conducting a systematic review of research that investigated CoI/CoP through social network analysis, Jan et al. (2019) found the need to provide a conceptual framework to guide the identification of online learning communities. They argue that all communities are networks, and therefore, an SLA approach is appropriate. Their purpose is to build a framework to identify learning communities in higher education online learning. Jan and Vlachopoulos (2019) argue that there is a lack of quantitative research using both CoP and CoI frameworks, which are widely used in online learning (Castellanos-Reyes, 2020). Despite the potential of SLA to identify online communities, little research exists considering CoP and CoI through SLA. Although, to our knowledge, Jan and Vlachopoulos (2019) are the pioneers of proposing a conceptual framework that integrates online learning theory and SLA, their SLA approach is still descriptive, limiting researchers' possibilities. For instance, they focus on descriptive aggregated network analysis, leaving behind inferential analysis (e.g., exponential random graph models). Narrowing down Jan and Vlachopoulos's (2019) approach from all the constructs of CoI and CoP to social presence would better serve researchers investigating interactions in online learning using SLA given the potential complexity of network analysis.
2.4.3. Kent and Rechavi (2020)–Deconstructing online social learning
Like Jan and Vlachopoulos (2019) and Kent and Rechavi (2020) also focused on the potential of SLA to support online discussions from a community perspective. Kent and Rechavi advocate for more types of interactions apart from “speaking” interactions (e.g., direct communication via discussion board posts). They explain that a majority of participants in online learning are considered inactive or passive. Therefore, suggesting speaking interactions as the most valid type of interaction is a misconception because learners also engage in other behaviors like interacting with content. Furthermore, discussion boards elicit anxious feelings in learners who doubt the significance of their contributions (Koehler and Meech, 2022). Guided by a collaborative learning paradigm, Kent and Rechavi (2020) describe the learners' interaction networks in online communities based on three types of interactions: (1) creational, (2) consumption, and (3) organizational. Kent and Rechavi (2020) allegorize creational interactions as “digitally speaking,” implying proactive interactions from learners (e.g., posting, editing posts). “Digitally listening” exemplifies consumption interactions like following peers, watching videos, or reading. The authors argue that learners' consuming interactions are usually overlooked due to the complexity of data extraction and an over-emphasis on individual assessment rather than collaborative work. Kent and Rechavi argue that consumption interactions serve to consider passive learners, also known as lurkers. Finally, stemming from Ausubel's advance organizers (1968), learners' activities with content organizational interactions refer to organizing their content through tags and bookmarks. While Kent and Rechavi (2020) include student-content interaction, a more apparent distinction between consuming interaction (i.e., interaction with content) and organization interaction (i.e., sorting content) is needed to better reflect the types of networks that researchers can study through SLA. Furthermore, despite Kent and Rechavi's work addressing learners that are usually overlooked (i.e., lurkers), they fall short on how to analyze the creational content that learners make in their interactions. Furthermore, as social presence is a precursor of a trustworthy environment for knowledge construction, understanding how “digitally listening” (student-content) interactions contribute to this foundation by supporting knowledge construction is necessary. As Richardson and Swan (2003) asserted “social presence permeates not only the activities generally designated as social activities but also those activities usually designated as individual activities” (p. 80). Furthermore, the SNA measures they examined, like transitivity and betweenness, can be related to the social presence construct “group cohesion” because they estimate how a network of learners is well connected. Such are summary statistics that describe the interactions within the network. Still, they do not account for students' perceptions of the social presence or their ability to display themselves as real. We ask, what aspects of the creational interactions that Kent and Rechavi propose make learners feel part of an online community to learn socially? Specifically, we suggest that adding the perspective of affective communication from the social presence construct is much needed to understand online learning interaction deeply. For example, include indicators that account for students' feelings during discussion boards or when interacting with the content.
3. Defining the i-SUN conceptual framework
The above models and frameworks demonstrate that interaction is a critical aspect of collaborative online learning and, consequently, of social presence to sustain online learning. Aside from learning occurring individually (Anderson, 2003), accounting for social presence enhances online learners' experiences and interactions by positively influencing their perceived learning and satisfaction (Richardson et al., 2017). As such, interaction can be among learners, instructors, content (Moore, 1989); learner and the interface (Hillman et al., 1994); and even between learner and the network (Dennen, 2013). We also underscore the need to account for the interaction of online learners who do not interact directly with peers or instructors (Kent and Rechavi, 2020)-in other words, who prefer to interact with the interface, the content, or the network only. Previous work described the unique role of discourse as essential to examining the quality of the interactions within the online learning experience (Gunawardena et al., 1997). As explained by Dennen (2019), Engeström captures the complexity of online ecosystems by describing tensions among elements. Taking all these perspectives together, we propose using a network analysis lens to help researchers explain the complexity of interactions in online learning on what is called SLA (e.g., Ferguson and Shum, 2012; Jan and Vlachopoulos, 2019). We posit an integration of social presence, SLA, and network analysis to research online learning interaction. To that end, we first offer a visualization of the proposed steps to research online learning interaction using SLA through the i-SUN process (see Figure 1). Then, we address the indicators of SLA measures for researchers to investigate online learning interaction through SLA and the social presence construct lens (Table 3).
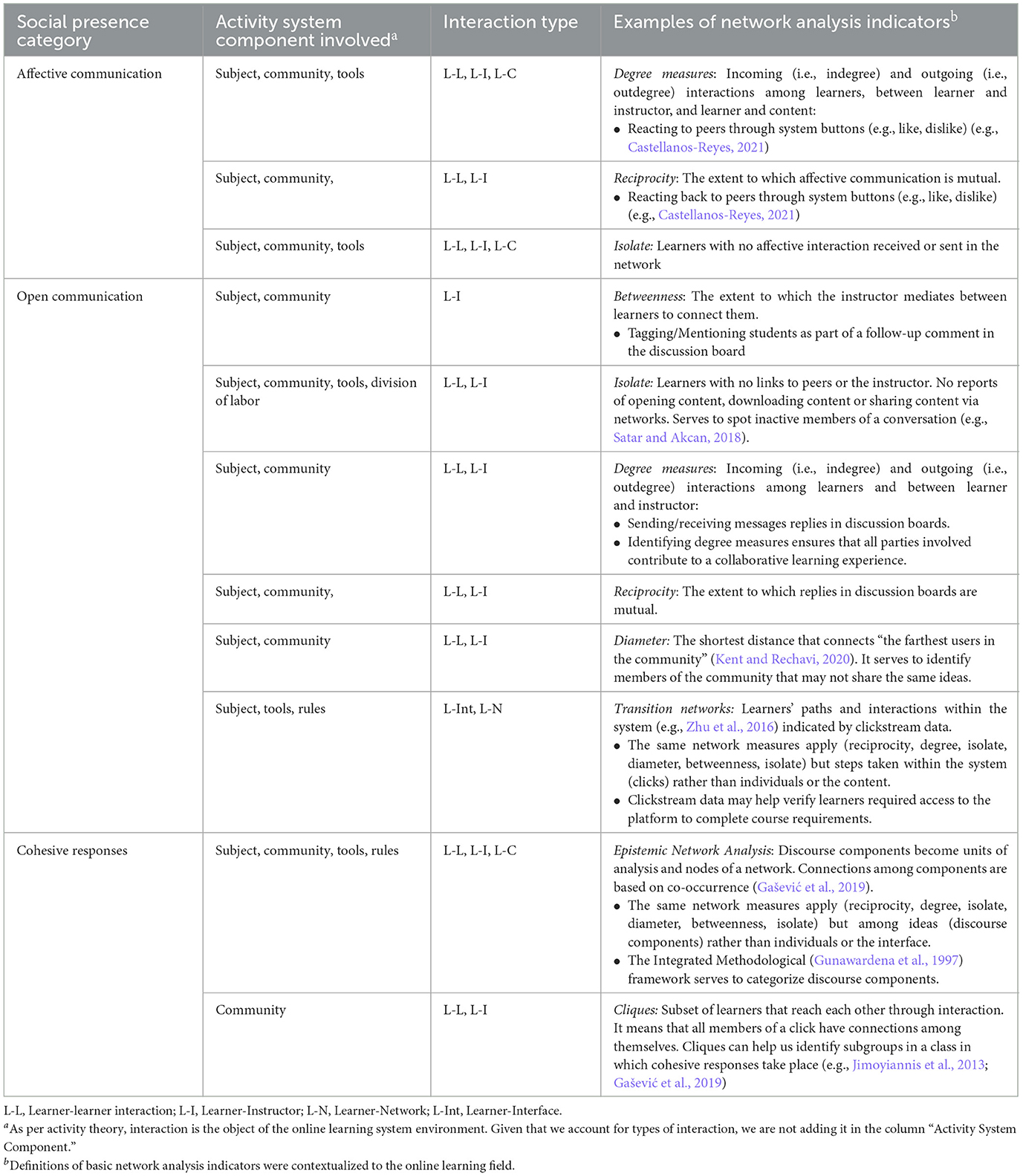
Table 3. Social learning analytics indicators to research interaction from a social presence perspective.
3.1. The i-SUN process
We propose a four-step process using social learning analytics for researching online learning interaction supported by social presence (Figure 2) with the purpose of fostering rigorous application of online learning theory when using complex data and methods. The first step focuses on choosing the interaction of interest. Second, researchers are suggested to align the social presence subconstruct with the selected interaction to guide their inquiry. Third, based on the previous selections, researchers are prompted to choose the units of analysis, as well as the type of network they would like to research. Finally, a set of descriptive network statistics can be selected to understand the interaction and, if necessary, inferential network analysis tests.
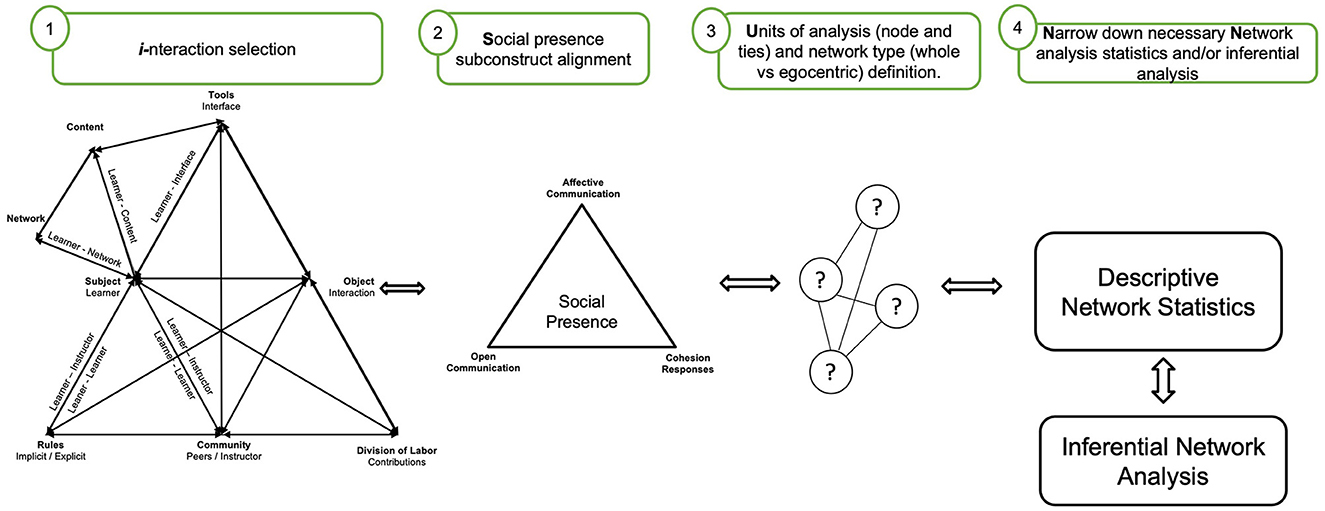
Figure 2. The i-SUN process to use social learning analytics to research online learning interaction guided by social presence.
3.1.1. Step 1: i-nteraction selection
The main triangle in Figure 2 depicts the tension between the online learner (i.e., subject) and the complex environment (i.e., tools, object, division of labor, community, and rules) as captured by Engeström's (2001) Activity Theory adapted to online learning (Dennen, 2013). This structure includes the subject who is the student engaged in activities within the online learning system resulting in interaction. Students are the actors or nodes initiating the interaction toward instructors, other learners, content, the interface, and the network (i.e., social media). It is worth noting that the learner-network and the learner-community are not interchangeable, as learner-network interaction refers to interactions with “outsider” individuals (Dennen, 2013) whereas learner-community refers to interactions with those who are a part of the institutional academic community (i.e., instructor and peers). The rules are “Implicit and explicit guidelines that constraint the activity” (Xing et al., 2015). The interface or the instructor could impose these rules. For example, instructors usually enforce implicit rules like netiquette in discussion boards. Explicit rules might include interface affordances to include (or not) liking buttons to acknowledge announcements and comments. Furthermore, ethical considerations about the data gathered for SLA fall within this category. Community involves the interaction between learners and peers and learners and instructors that create a sense of community. Given that direct exchange of information may not be necessary for all learners to establish a sound sense of community. Therefore, the tension between the learner and tools is also relevant. Tools are the instruments that mediate the learning environment (e.g., learning management systems, institutional email).
The two smaller triangles adjacent to the main structure add the components of the network and the content that refer to the interaction between the subject (learner) and the content and the network as proposed by Hillman et al. (1994) and Dennen (2013). The three components (tools, content, and network) are aligned as non-human elements from which SLA gathers data to support online learning interactions. Finally, the object is learning interaction itself with the components of the online learning activity system.
3.1.2. Step 2: social presence subconstructs alignment
After choosing the object of the system, which is the interaction itself, researchers need to align such interaction with at least one of the social presence elements to guide their inquiry. This interaction is supported by the three elements of social presence: open communication, affective communication, and cohesive discourse. Researchers can either use the three components of social presence separately like Tirado-Morueta et al. (2016) did or use the social presence construct as a whole, following the example of Shea et al. (2014). Although researchers are encouraged to use the social presence construct holistically, sometimes, the available interaction data are not comparable to all the elements of social presence, for example, in the case of Castellanos-Reyes (2021), who faced the limitation of using clickstream data to investigate the interaction and consequently focused only on affective communication and open communication.
3.1.3. Step 3: units of analysis and network type definition
This step focuses on defining who are the nodes or actors in the interaction of interest and which type of network better suits that interaction. Most research using SLA so far has focused on learner-learner interaction. Therefore, the nodes in the analysis are the learners themselves. If researchers focus on single online courses, their analysis can use whole networks. In other words, they can study the interactions of the entire system. Researchers can also focus on longitudinal analysis of learners' networks. For example, the work of Saqr and López-Pernas (2021b) who follow the interactions of online students over an entire program.
3.1.4. Step 4: narrow down network analysis statistics and inferential analysis
Although network analysis provides a plethora of network statistics to choose from, researchers would benefit from keeping an efficient rather than extensive attitude toward using network analysis measures. For example, Castellanos-Reyes (2021) used the indegree measure (i.e., the number of incoming interactions received by an actor) to estimate affective and open communication. On the one hand, the number of likes received on a comment on a discussion board was equivalent to affective communication. On the other hand, the number of comments that a student received was operationalized as open communication (Castellanos-Reyes, 2021).
Table 3 integrates the different elements of the proposed framework with specific measures to guide researchers when using SLA to examine online learning interactions. The first column refers to the categories of social presence, while second column refers to the components of the complex activity system that play a role in each social presence element. The third column refers to the type of interaction. Given that SLA uses network analysis measures to explain learners' interactions (Aviv et al., 2005), a central component of the i-SUN framework is to contextualize the definition of basic network statistics frequently used in SLA for online learning. As such, the fourth column refers to the specific SLA measures adapted. The network analysis indicators described stems from theories of communication networks (Monge and Contractor, 2003) and foundational work on social network analysis (Marin and Wellman, 2011). Furthermore, in the fourth column specific examples of each SLA indicators are provided for guidance. Some network analysis measures repeat given that researchers may use one measure to explore different aspects of interaction and social presence. Readers interested in a more detailed description of network analysis measures, please refer to Monge and Contractor (2003) and to Carolan (2014) for contextualization in broader educational research.
4. Discussion and applications
The conceptual framework and indicators presented in this paper will serve researchers who want to understand online learning interactions through SLA. Currently, SLA is dominated by a small group of researchers, and this framework can help create an opportunity for others to join that conversation. Perhaps, one reason for this might be the perceived complexity of the methods used. Therefore, we believe that a conceptual framework may allow other researchers to join this conversation by elaborating on the meaning of standard SLA measures in online learning. This conceptual framework adds value to the field by connecting online learning constructs to network analysis measures used in SLA. Previous researchers have conceptualized network analysis for educational research (Ferguson and Shum, 2012; Jan and Vlachopoulos, 2019; Kent and Rechavi, 2020). However, existing frameworks are still too obscure for researchers unfamiliar with network analysis to follow. Yet, the proposed framework merits consideration, given its focus on a step-by-step basis and its integration of inferential network analysis. Furthermore, we have expanded upon the pioneering work of Jan and Vlachopoulos (2019) by including interactions among members of the community within discussion forums and other elements of the activity system (i.e., content, network, interface).
The presented conceptual framework offers practical guidelines to researchers who foster interaction through social presence. Therefore, the proposed framework may support future endeavors that conceptualize other elements of online learning communities apart from social presence, like cognitive and teaching presence. Future work could expand the proposed framework using the exemplary work of Sadaf and Olesova (2020) who used SLA to explore the Practical Inquiry Model in relation to social presence. Given that the Practical Inquiry Model is used to operationalize cognitive presence, we foresee that future work on SLA and interaction will take upon the challenge of addressing these other constructs. Therefore, the i-SUN model could potentially be applied to other online learning constructs such as cognitive interactions.
4.1. Applications of the i-SUN conceptual framework
We argue that through SLA, network analytic methods are a coherent approach to online learning because they can illuminate the interdependencies of the learning ecosystem. However, the complexity of network measures and their indiscriminate use in educational research poses interpretative challenges for researchers leading to a “cacophony of networks” (Poquet and Joksimovic, 2022). Therefore, this framework does not focus on further analyzing empirical data but rather on “integrating existing perspectives into a more holistic view” (McGregor, 2019, p. 7) that connects the bodies of literature of online learning interaction, SLA, and network analysis. As conceptual frameworks do not generally include empirical data (McGregor, 2019), this framework provides a list of potential applications of the i-SUN Conceptual Framework that merit empirical validation. These examples serve the online learning field as guidelines for rigorous SLA implementation.
4.1.1. Fostering community using task-centered approaches
When learners interact with each other through the interface, active participation is facilitated by achieving common goals through division of labor and open communication. Yet, we recommend studying the specific mechanism and instructional methods that drive such facilitation. A good example is Tirado-Morueta, Maraver-López, Pérez-Rodríguez and Hernando-Gómez (2020) work in which facilitation tasks are explored using network analysis measures (i.e., density and centralization) in the light of social presence. Researchers could integrate instructional elements using Molenda and Subramony's (2021) update of the elements of instruction framework which provides a set of communication configurations that can be explored through SLA and social presence.
4.1.2. Use of learner-network interaction to foster knowledge transfer through egocentric approaches
The addition of learner-network interaction proposed by Dennen (2013) allows researchers to add authentic learning experiences to online learning interactions. For instance, registering learners' interactions with individuals outside the formal online learning community, such as experts on Twitter (Castellanos-Reyes et al., 2021). Suppose researchers are looking to investigate learner-network interaction. In that case, they might not have access to all learners available in the network. So, although the unit of analysis is still learners, they might want to focus on an egocentric approach to online learning. An analysis of egocentric networks, learners are asked for information about those with whom they interact. However, those at the other end of the interaction are not part of the research. A potential application is to ask learners who are the top three Twitter users who make them feel affectively connected to the community.
4.1.3. Go beyond threaded discussion data
Most research using network analysis to examine social presence centers on text-based communication. However, focusing only on discussion boards leaves behind those vicarious learners who benefit from reading without engaging in conversation, the so-called lurkers (Sun et al., 2014; Bozkurt et al., 2020). Koehler and Meech (2022) found that discussion boards can overwhelm learners, producing anxiety about what to post and the merit of their thoughts compared to peers' contributions. Furthermore, social presence behaviors might occur in other aspects of the course, like communications via email as part of group work. Again, the vast amount of data collected through learning management systems can enrich researchers' conclusions about students' social presence. For example, researchers might also integrate the Community of Inquiry instrument (Arbaugh et al., 2008), specifically the SP subscale, as covariates of their SLA research. Combining the self-reported measures of SP as covariate measures would shed light on how the network configuration of online learning communities reflects students' internal psychological states. Furthermore, ERT (Hodges et al., 2020) employed widely during the COVID-19 pandemic highlighted other formats of distance education delivery, (e.g., synchronous interaction via videoconference) that should be examined. Although mandatory viewing of pre-recorded lectures and videoconferencing are uncommon in formal distance education (Stewart et al., 2023), they became the heart of ERT, eventually taking an essential role in online learning post-pandemic. Future research could explore the relationship between synchronous communication via videoconferencing and constructs like connectedness and community building (Belt and Lowenthal, 2023), and eventually social presence interactions as the object of the learning experience.
4.1.4. Being intentional with network analysis indicators
Network analysis offers a plethora of network statistics to understand and explain the structure of a network. Furthermore, network analysis allows researchers to define what each statistic means in their contexts. Yet, it does not imply the unsystematic use of statistics for the sake of reporting. Saqr and López-Pernas's (2021a) meta-analysis of network analysis centrality measures used to investigate collaborative learning argue that previous work shoes inconclusive and contradictory results. Their analysis recommends using degree and eigenvector centrality measures as performance indicators but discourage the use of closeness and betweenness centrality. We invite researchers to be intentional about the statistics used in SLA measures, and above all, guide their definitions with a theory like the social presence construct. For example, previous work by Shea et al. (2010, 2013) have suggested that measures like centrality are significantly related to social presence.
4.1.5. Explore correlational and inferential network analysis
The merit of descriptive work is indisputable. It is descriptive analysis and observations what drive researchers to formulate hypotheses to explain the educational phenomenon. Yet, we encourage researchers to make the leap from descriptive SLA to inferential SLA to better understand online interaction and social presence. Inferential SLA does not need to involve complex longitudinal (Castellanos-Reyes, 2021) or epistemic (Gašević et al., 2019) research designs. For instance, parallel to traditional t-tests, researchers could compare two different relations of the same set of learners. For example, researchers could explore if a social presence network obtained from online learners' collaboration shows patterns that deviate from a comparable random network (Borgatti et al., 2018).
4.1.6. Question the boundaries of an online community and who belongs to it
Social presence in online learning is conceptualized in this manuscript from the CoI lens. Although ERT has driven researchers various delivery forms of online learning like synchronous communication through the CoI lens (Shea et al., 2022), the CoI was conceptualized to address text-based computer-mediated communication in formal higher education, which assumes that all students enrolled in a course are members of the community. Nevertheless, issues like passive students or lurkers (Sun et al., 2014; Bozkurt et al., 2020) and the inclusion of intelligent tutoring via artificial intelligence (Ebadi and Amini, 2022; Huang et al., 2022) make us push the question of the boundaries of an online community. Related to the suggested application of going beyond discussion boards, we invite the community to apply the i-SUN process to identify who belongs to an online community by comparing observed data from discussion boards with students' reports on who they consider being in their communities. Taking such an approach might shift the focus to individual students' connections without assuming that the community includes everyone enrolled in a course.
5. Conclusions
There is a need for a SLA taxonomy to analyze online learning interaction that fosters a rigorous application of online learning interaction theory combined with network analysis methods. As such, the expansion of the i-SUN conceptual framework into specific measures used in SLA is a concrete tool for researchers. Future work on SLA should have an intersection and consideration of cultural contexts and nuances. Examples include exploring the relationships between culture and learning through measures such as collective quantitative proficiency. For example, process data collected from computer assessment environments are analyzed through transition networks. Conceptual frameworks require an application to explore how the offered operationalizations stand in a real-world setting. Therefore, future work should focus on how the provided SLA measures reflect online learning interaction. We hope to apply the proposed measures in a prospective case study.
Author contributions
DCR contributed to conceptualization, writing of initial draft, creation of visualization, and reviewing and editing. AK and JR contributed with critical review, and commentary or revision. All authors contributed to the article, manuscript revision, read, and approved the submitted version.
Funding
We want to thank the National Academy of Education, National Academy of Education/Spencer Dissertation Fellowship Program and the Bilsland Dissertation Fellowship for funding this research.
Acknowledgments
DCR thanks God for his support and the fruitful discussions with Prof. Dr. Dirk Ifenthaler, Shivani Ramoutar, Sally Meech, and Dr. Rathziel Roncancio on initial drafts of this manuscript. All authors want to thank the reviewers for their detailed feedback. We are grateful for the support of the Purdue University Libraries Open Access Publishing Fund.
Conflict of interest
The authors declare that the research was conducted in the absence of any commercial or financial relationships that could be construed as a potential conflict of interest.
Publisher's note
All claims expressed in this article are solely those of the authors and do not necessarily represent those of their affiliated organizations, or those of the publisher, the editors and the reviewers. Any product that may be evaluated in this article, or claim that may be made by its manufacturer, is not guaranteed or endorsed by the publisher.
References
Aguiar, E., Ambrose, G. A. A., Chawla, N. V., Goodrich, V., and Brockman, J. (2014). Engagement vs performance: using electronic portfolios to predict first semester engineering student persistence. Learn. Anal. 1, 7–33. doi: 10.18608/jla.2014.13.3
Anderson, T. (2003). Getting the Mix Right Again: An Updated and Theoretical Rationale for Interaction. IRRODL 4. doi: 10.19173/irrodl.v4i2.149
Arbaugh, J. B., Cleveland-Innes, M., Diaz, S. R., Garrison, D. R., Ice, P., Richardson, J. C., et al. (2008). Developing a community of inquiry instrument: testing a measure of the Community of Inquiry framework using a multi-institutional sample. Internet High. Educ. 11, 133–136. doi: 10.1016/j.iheduc.2008.06.003
Aulck, L., Aras, R., Li, L., L'Heureux, C., Lu, P., and West, J. (2017). STEM-Ming the Tide: Predicting STEM Attrition Using Student Transcript Data. Nova Scotia, 10.
Aviv, R., Erlich, Z., and Ravid, G. (2005). Response neighborhoods in online learning networks: a quantitative analysis. Educ. Technol. Soc. 8, 90–99.
Bandura, A. (2002). Social cognitive theory in cultural context. Appl. Psychol. 51, 269–290. doi: 10.1111/1464-0597.00092
Bedny, G. Z., and Karwowski, W. (2004). Activity theory as a basis for the study of work. Ergonomics 47, 134–153. doi: 10.1080/00140130310001617921
Belt, E. S., and Lowenthal, P. R. (2023). Synchronous video-based communication and online learning: an exploration of instructors' perceptions and experiences. Educ. Inf. Technol. 28, 4941–4964. doi: 10.1007/s10639-022-11360-6
Bernard, R. M., Abrami, P. C., Borokhovski, E., Wade, C. A., Tamim, R. M., Surkes, M. A., et al. (2009). A meta-analysis of three types of interaction treatments in distance education. Rev. Educ. Res. 79, 1243–1289. doi: 10.3102/0034654309333844
Borgatti, S. P., Everett, M. G., and Johnson, J. (2018). Analyzing Social Networks. 2nd ed. Thousand Oaks, CA: SAGE.
Bozkurt, A., Koutropoulos, A., Singh, L., and Honeychurch, S. (2020). On lurking: multiple perspectives on lurking within an educational community. Internet High. Educ. 44:100709. doi: 10.1016/j.iheduc.2019.100709
Carolan, B. (2014). Social Network Analysis and Education Theory, Methods and Applications. Thousand Oaks, CA: SAGE Publications. doi: 10.4135/9781452270104
Castellanos-Reyes, D. (2020). 20 years of the community of inquiry framework. TechTrends, 64, 557–560. doi: 10.1007/s11528-020-00491-7
Castellanos-Reyes, D. (2021). The dynamics of a MOOC's learner-learner interaction over time: a longitudinal network analysis. Comput. Human Behav. 123:106880. doi: 10.1016/j.chb.2021.106880
Castellanos-Reyes, D., Maeda, Y., and Richardson, J. (2021). “The relationship between social network sites and perceived learning and satisfaction for educational purposes: A systematic review and meta-analysis,” in Social Media: Influences in Education. p. 283–314.
Cohen, A. (2017). Analysis of student activity in web-supported courses as a tool for predicting dropout. Educ. Tech. Res. Dev. 65, 1285–1304. doi: 10.1007/s11423-017-9524-3
Cui, G., Lockee, B., and Meng, C. (2013). Building modern online social presence: a review of social presence theory and its instructional design implications for future trends. Educ. Inform. Technol. 18, 661–685. doi: 10.1007/s10639-012-9192-1
Dennen, V. (2019). “Frameworks for designing and analyzing learning activity interactions in online courses,” in Handbook of Distance Education, eds M. G. Moore and W. Diehl (New Yor, NY: Routledge). 245–259. doi: 10.4324/9781315296135-20
Dennen, V. P. (2013). “Activity design and instruction in online learning,” in Handbook of Distance Education, ed M. G. Moore (New York, NY: Routledge).
Drachsler, H., and Greller, W. (2012). “The pulse of learning analytics understandings and expectations from the stakeholders,” in Proceedings of the 2nd International Conference on Learning Analytics and Knowledge (Vancouver, BC: ACM), 120–129. doi: 10.1145/2330601.2330634
Ebadi, S., and Amini, A. (2022). Examining the roles of social presence and human-likeness on Iranian EFL learners' motivation using artificial intelligence technology: A case of CSIEC chatbot. Interact. Learn. Environ. 1–19. doi: 10.1080/10494820.2022.2096638
Engeström, Y. (1987). Learning by Expanding: An Activity-Theoretical Approach to Developmental Research. Helsinki: Orienta-Konsultit.
Engeström, Y. (2001). Expansive learning at work: toward an activity theoretical reconceptualization. J. Educ. Work 14, 133–156. doi: 10.1080/13639080020028747
Falloon, G. (2011). Making the connection: moore's theory of transactional distance and its relevance to the use of a virtual classroom in postgraduate online teacher education. J. Res. Technol. Educ. 43, 187–209. doi: 10.1080/15391523.2011.10782569
Farnsworth, V., Kleanthous, I., and Wenger-Trayner, E. (2016). Communities of practice as a social theory of learning: a conversation with etienne wenger. Br. J. Educ. Stud. 64, 139–160. doi: 10.1080/00071005.2015.1133799
Ferguson, R., and Shum, S. B. (2012). Social learning analytics. J. Educ. Technol. Soc. 15, 3–26. doi: 10.1145/2330601.2330616
Fiock, H. (2020). Designing a community of inquiry in online courses. Int. Rev. Res. Open Dis. Learn. 21, 135–153. doi: 10.19173/irrodl.v20i5.3985
Garrison, R. (2017a). E-Learning in the 21st century: A Community of Inquiry Framework for Research and Practice. Routledge. Available online at: https://ebookcentral.proquest.com
Garrison, R. (2017b). “Social presence,” in E-learning in the 21st Century: A Community of Inquiry framework for research and practice (New Yor, NY: Routledge), 35–49.
Garrison, R., Anderson, T., and Archer, W. (2000). Critical Inquiry in a text-based environment: computer conferencing in higher education. Internet High. Educ. 2, 87–105. doi: 10.1016/S1096-7516(00)00016-6
Garrison, R., and Arbaugh, J. B. (2007). Researching the community of inquiry framework: review, issues, and future directions. Internet High. Educ. 10, 157–172. doi: 10.1016/j.iheduc.2007.04.001
Gašević, D., Joksimović, S., Eagan, B. R., and Shaffer, D. W. (2019). SENS: network analytics to combine social and cognitive perspectives of collaborative learning. Comput. Human Behav. 92, 562–577. doi: 10.1016/j.chb.2018.07.003
Goel, L., Zhang, P., and Templeton, M. (2012). Transactional distance revisited: Bridging face and empirical validity. Comput. Human Behav. 28, 1122–1129. doi: 10.1016/j.chb.2012.01.020
Gunawardena, C. N. (1995). Social presence theory and implications for interaction and collaborative learning in computer conferences. Int. J. Educ. Telecommun. 1, 147–166.
Gunawardena, C. N. (2013). “Culture and online distance education,” in Handbook of Distance Education (New York, NY: Routledge), 185–200.
Gunawardena, C. N., Lowe, C. A., and Anderson, T. (1997). Analysis of a global online debate and the development of an interaction analysis model for examining social construction of knowledge in computer conferencing. J. Educ. Comput. Res. 17, 397–431. doi: 10.2190/7MQV-X9UJ-C7Q3-NRAG
Gunawardena, C. N., and Zittle, F. J. (1997). Social presence as a predictor of satisfaction within a computer-mediated conferencing environment. Am. J. Dist. Educ. 11, 8–26. doi: 10.1080/08923649709526970
Hart, C. (2012). Factors associated with student persistence in an online program of study: a review of the literature. J. Interact. Online Learn. 11, 19–42.
Hillman, D. C. A., Willis, D. J., and Gunawardena, C. N. (1994). Learner-interface interaction in distance education: an extension of contemporary models and strategies for practitioners. Am. J. Distance Educ. 8, 30–42. doi: 10.1080/08923649409526853
Hodges, C., Moore, S., Lockee, B., Trust, T., and Bond, A. (2020). The Difference Between Emergency Remote Teaching and Online Learning. Educause review.
Huang, H., Chen, Y., and Rau, P. -L. P. (2022). Exploring acceptance of intelligent tutoring system with pedagogical agent among high school students. Univ. Access Inf. Soc. 21, 381–392. doi: 10.1007/s10209-021-00835-x
Ifenthaler, D. (2015). “Learning analytics,” in The SAGE Encyclopedia of Educational Technology, Vol. 2, ed J. M. Spector (Thousand Oaks, CA: Sage), 447–451.
Ifenthaler, D., and Widanapathirana, C. (2014). Development and validation of a learning analytics framework: two case studies using support vector machines. Technol. Knowl. Learn. 19, 221–240. doi: 10.1007/s10758-014-9226-4
Ifenthaler, D., and Yau, J. Y. K. (2020). Utilising learning analytics to support study success in higher education: a systematic review. Educ. Technol. Res. Dev. 68, 1961–1990. doi: 10.1007/s11423-020-09788-z
Jan, S. K., and Vlachopoulos, P. (2019). Social network analysis: A framework for identifying communities in higher education online learning. Technol. Knowl. Learn. 24, 621–639. doi: 10.1007/s10758-018-9375-y
Jan, S. K., Vlachopoulos, P., and Parsell, M. (2019). Social network analysis and online learning in communities in higher education: a systematic literature review. Online Learn. 23, 249–265. doi: 10.24059/olj.v23i1.1398
Jimoyiannis, A., Tsiotakis, P., and Roussinos, D. (2013). Social network analysis of students' participation and presence in a community of educational blogging. Interact. Technol. Smart Educ. 10, 15–30. doi: 10.1108/17415651311326428
Joksimović, S., Gašević, D., Kovanović, V., Riecke, B. E., and Hatala, M. (2015). Social presence in online discussions as a process predictor of academic performance. J. Comput. Assist. Learn. 31, 638–654. doi: 10.1111/jcal.12107
Jonassen, D. H., and Rohrer-Murphy, L. (1999). Activity theory as a framework for designing constructivist learning environments. Educ. Technol. Res. Dev. 47, 61–79. doi: 10.1007/BF02299477
Jordan, B., and Henderson, A. (1995). Interaction analysis: foundations and practice. J. Learn. Sci. 4, 39–103. doi: 10.1207/s15327809jls0401_2
Kellogg, S. B., Booth, S., and Oliver, K. M. (2014). A social network perspective on peer support learning in MOOCs for educators. Int. Rev. Res. Open Distance Learn. 15, 263–289. doi: 10.19173/irrodl.v15i5.1852
Kent, C., and Rechavi, A. (2020). Deconstructing online social learning: network analysis of the creation, consumption and organization types of interactions. Int. J. Res. Method Educ. 43, 16–37. doi: 10.1080/1743727X.2018.1524867
Knight, S., and Buckingham Shum, S. (2017). “Theory and learning analytics,” in Handbook of Learning Analytics, eds C. Lang, G. Siemens, D. Gasevic (Vancouver, BC: University of Texas at Arlington, New York University, and Society for Learning Analytics Research (SoLAR)), 17–22. doi: 10.18608/hla17.001
Koehler, A. A., and Meech, S. (2022). Ungrading learner participation in a student-centered learning experience. TechTrends 66, 78–89. doi: 10.1007/s11528-021-00682-w
Kreijns, K., Xu, K., and Weidlich, J. (2022). Social presence: conceptualization and measurement. Educ. Psychol. Rev. 34, 139–170. doi: 10.1007/s10648-021-09623-8
Kyei-Blankson, L., Ntuli, E., and Donnelly, H. (2019). Establishing the importance of interaction and presence to student learning in online environments. J. Interact. Learn. Res. 30, 539–560. doi: 10.22158/wjer.v3n1p48
Lang, C., Siemens, G., Wise, A. F., Gasevic, D., and Merceron, A. (2022). Handbook of Learning Analytics. 2nd ed. (Vancouver, BC: Society for Learning Analytics Research (SoLAR)). doi: 10.18608/hla22
Lim, J. (2023). Exploring the relationships between interaction measures and learning outcomes through social network analysis: the mediating role of social presence. Int. J. Educ. Technol. High Educ. 20, 14. doi: 10.1186/s41239-023-00384-8
Lockyer, L., and Dawson, S. (2012). “Where learning analytics meets learning design,” in Proceedings of the 2nd International Conference on Learning Analytics and Knowledge (Vancouver, BC: ACM), 14–15. doi: 10.1145/2330601.2330609
Lowyck, J. (2014). Bridging Learning Theories and Technology-Enhanced Environments: A Critical Ppraisal of Its History. Handbook of Research on Educational Communications and Technology: Fourth Edition, 1–1005. doi: 10.1007/978-1-4614-3185-5_1
Marin, A., and Wellman, B. (2011). “Social network analysis: An introduction,” in The SAGE Handbook of Social Network Analysis., eds. J. Scott and P. Carrington (Thousand Oaks, CA: SAGE Publications).
McGregor, S. (2019). “Conceptual and theoretical papers,” in Understanding and Evaluating Research: A Critical Guide (Thousand Oaks, CA: SAGE Publications).
Molenda, M., and Subramony, D. P. (2021). The Elements of Instruction: A Framework For the Age of Emerging Technologies. 1st ed. (New York, NY: Taylor and Francis). doi: 10.4324/9781315194721-1
Monge, P., and Contractor, N. (2003). Theories of Self-Interest and Collective Action. Cambridge, MA: Theories of Communication Networks, 141–172. doi: 10.1093/oso/9780195160369.003.0012
Moore, M. G. (1989). Editorial: three types of interaction. Am. J. Distance Educ. 3, 1–7. doi: 10.1080/08923648909526659
Moore, M. G. (1993). “Theory of transactional distance,” in Theoretical principles of Distance Education (bookRoutledge), 22–38.
Pei, L., and Wu, H. (2019). Does online learning work better than offline learning in undergraduate medical education? a systematic review and meta-analysis. Med. Educ. Online 24:1666538. doi: 10.1080/10872981.2019.1666538
Poquet, O., and Joksimovic, S. (2022). “Cacophony of metworks in learning analytics,” in Handbook of Learning Analytics, eds C. Lang, G. Siemens, A. Friend Wise, D. Gašević, and A. Merceron (Vancouver, BC: SoLAR). doi: 10.18608/hla22.004
Poquet, O., and Jovanovic, J. (2020). “Intergroup and interpersonal forum positioning in shared-thread and post-reply networks,” in ACM International Conference Proceeding Series, 187–196. doi: 10.1145/3375462.3375533
Reyes, J. (2013). Transactional distance theory: Is it here to stay? Dist. Learn. 10, 43–50. Available online at: https://www.thefreelibrary.com/_/print/PrintArticle.aspx?id=369914705
Richardson, J. C., Koehler, A. A., Besser, E. D., Caskurlu, S., Lim, J., and Mueller, C. M. (2015). Conceptualising and investigating instructor presence in online learning environments. Int. Rev. Res. Open Dis. Learn. 16, 256–297. doi: 10.19173/irrodl.v16i3.2123
Richardson, J. C., Maeda, Y., Lv, J., and Caskurlu, S. (2017). Social presence in relation to students' satisfaction and learning in the online environment: a meta-analysis. Comput. Human Behav. 71, 402–417. doi: 10.1016/j.chb.2017.02.001
Richardson, J. C., and Swan, K. (2003). Examining social presence in online courses in relation to students' perceived learning and satisfaction. JALN. 7, 68–88. doi: 10.24059/olj.v7i1.1864
Rourke, L., Anderson, T., Garrison, R., and Archer, W. (2001). Assessing social presence in asynchronous text-based computer conferencing. J. Distance Educ. 14, 50–71.
Sadaf, A., and Olesova, L. (2020). Exploring the relationship between interaction and the structure of questions in online discussions using learning analytics. Eur. J. Open Distance E-Learn. 23, 46–60. doi: 10.2478/eurodl-2020-0004
Saqr, M., and López-Pernas, S. (2021a). The curious case of centrality measures: a large-scale empirical investigation. J. Learn. Anal. 8, 1–23. doi: 10.18608/jla.2022.7415
Saqr, M., and López-Pernas, S. (2021b). The longitudinal trajectories of online engagement over a full program. Comput. Educ. 175:104325. doi: 10.1016/j.compedu.2021.104325
Satar, H. M., and Akcan, S. (2018). Pre-service EFL teachers' online participation, interaction, and social presence. Lang. Learn. Technol. 22, 157–184.
Sclater, N., Peasgood, A., and Mullan, J. (2016). Learning Analytics in Higher Education: A Review of UK and International Practice Full Report.
Shea, P., Hayes, S., Uzuner Smith, S., Vickers, J., Bidjerano, T., Gozza-Cohen, M., et al. (2013). Online learner self-regulation: learning presence viewed through quantitative content- and social network analysis. Int. Rev. Res. Open Dist. Learn. 14, 427–461. doi: 10.19173/irrodl.v14i3.1466
Shea, P., Hayes, S., Uzuner-Smith, S., Gozza-Cohen, M., Vickers, J., and Bidjerano, T. (2014). Reconceptualizing the community of inquiry framework: an exploratory analysis. Internet Higher Educ. 23, 9–17. doi: 10.1016/j.iheduc.2014.05.002
Shea, P., Hayes, S., Vickers, J., Gozza-Cohen, M., Uzuner, S., Mehta, R., et al. (2010). A re-examination of the community of inquiry framework: Social network and content analysis. Internet Higher Educ. 13, 10–21. doi: 10.1016/j.iheduc.2009.11.002
Shea, P., Richardson, J., and Swan, K. (2022). Building bridges to advance the community of inquiry framework for online learning. Educ. Psychol. 57, 148–161. doi: 10.1080/00461520.2022.2089989
Short, J., Williams, E., and Christie, B. (1976). The Social Psychology of Telecommunications. London: John Wiley and Sons.
Stewart, W. H., Lowenthal, P. R., and Richter, D. (2023). A model of remote teaching and learning under emergency and sustained crisis conditions: a description of novel distance educaiton context and manifestations. Turkish Online J. Dist. Educ. 24, 183–201. doi: 10.17718/tojde.1090810
Sun, C., Hwang, G., Yin, Z., Wang, Z., and Wang, Z. (2023). Trends and issues of social annotation in education: a systematic review from 2000 to 2020. Comput. Assist. Learn. 39, 329–350. doi: 10.1111/jcal.12764
Sun, N., Rau, P. P. L., and Ma, L. (2014). Understanding lurkers in online communities: a literature review. Comput. Human Behav. 38, 110–117. doi: 10.1016/j.chb.2014.05.022
Swan, K. (2021). “Teaching and learning in post-industrial distance education,” in An Introduction to Distance Education: Understanding Teaching and Learning in a New Era, eds M. F. Cleveland-Innes and R. D. Garrison (New York, NY: Routledge). doi: 10.4324/9781315166896-7
Tirado-Morueta, R., Maraver López, P., Hernando Gómez, Á., and Harris, V. W. (2016). Exploring social and cognitive presences in communities of inquiry to perform higher cognitive tasks. Internet High. Educ. 31, 122–131. doi: 10.1016/j.iheduc.2016.07.004
Tirado-Morueta, R., Maraver-López, P., Pérez-Rodríguez, A., and Hernando-Gómez, Á. (2020). Exploring social network structure patterns suitable to the community of inquiry model moderated by the task. J. Educ. Comput. Res. 58, 319–342. doi: 10.1177/0735633119845695
Viberg, O., Hatakka, M., Bälter, O., and Mavroudi, A. (2018). The current landscape of learning analytics in higher education. Comput. Human Behav. 89, 98–110. doi: 10.1016/j.chb.2018.07.027
Vygotsky, L. (1978a). “Interaction between learning and development,” in Readings on the Development of Children, eds.Gauvain and Cole (New York, NY: Scientific American Books), 34–40.
Vygotsky, L. (1978b). Mind in Society: The Development of Higher Psychological Processes, eds. M. Cole, V. John-Steiner, S. Scribner, and E. Souberman (London: Harvard University Press).
Wenger, E. (1998). Communities of Practice: Learning Meaning and Identity. Cambridge, MA: Cambridge University Press. doi: 10.1017/CBO9780511803932
Wenger, E. (2004). Knwledge Management as a Doughnut: Shaping Your Knowledge Strategy Through Communicites of Practice. Ivey Business Journal. Available online at: https://iveybusinessjournal.com/publication/knowledge-management-as-a-doughnut/
Wood, D., Bruner, J. S., and Ross, G. (1976). The role of tutoring in problem solving. J. Child Psychol. Psychiatry 17, 89–100. doi: 10.1111/j.1469-7610.1976.tb00381.x
Xing, W., Guo, R., Petakovic, E., and Goggins, S. (2015). Participation-based student final performance prediction model through interpretable genetic programming: integrating learning analytics, educational data mining and theory. Comput. Human Behav. 47, 168–181. doi: 10.1016/j.chb.2014.09.034
Yamagata-Lynch, L. C. (2010). Activity Systems Analysis Methods: Understanding Complex Learning Environments. New York, NY: Springer. doi: 10.1007/978-1-4419-6321-5
Zhang, J., Skryabin, M., and Song, X. (2016). Understanding the dynamics of MOOC discussion forums with simulation investigation for empirical network analysis (SIENA). Dist. Educ. 37, 270–286. doi: 10.1080/01587919.2016.1226230
Keywords: interaction, social presence, social learning analytics, network analysis, conceptual framework, online learning, distance education, social network analysis (SNA)
Citation: Castellanos-Reyes D, Koehler AA and Richardson JC (2023) The i-SUN process to use social learning analytics: a conceptual framework to research online learning interaction supported by social presence. Front. Commun. 8:1212324. doi: 10.3389/fcomm.2023.1212324
Received: 26 April 2023; Accepted: 11 July 2023;
Published: 07 August 2023.
Edited by:
Larisa Olesova, University of Florida, United StatesReviewed by:
Andreia de Bem Machado, Federal University of Santa Catarina, BrazilMingyue Fan, Jiangsu University, China
Patrick R. Lowenthal, Boise State University, United States
Copyright © 2023 Castellanos-Reyes, Koehler and Richardson. This is an open-access article distributed under the terms of the Creative Commons Attribution License (CC BY). The use, distribution or reproduction in other forums is permitted, provided the original author(s) and the copyright owner(s) are credited and that the original publication in this journal is cited, in accordance with accepted academic practice. No use, distribution or reproduction is permitted which does not comply with these terms.
*Correspondence: Daniela Castellanos-Reyes, Y2FzdGVsZEBwdXJkdWUuZWR1; ZGNhc3RlbDJAbmNzdS5lZHU=; Jennifer C. Richardson, amVubnJpY2hAcHVyZHVlLmVkdQ==