- 1Department of Linguistics and Scandinavian Studies, University of Oslo, Oslo, Norway
- 2The Alan Turing Institute, London, United Kingdom
- 3St Hugh's College, University of Oxford, Oxford, United Kingdom
In this paper, we explore the semantic map of the English temporal connective when and its parallels in more than 1,000 languages drawn from a parallel corpus of New Testament translations. We show that there is robust evidence for a cross-linguistic distinction between universal and existential WHEN. We also see tentative evidence that innovation in this area involves recruiting new items for universal WHEN which gradually can take over the existential usage. Another possible distinction that we see is between serialized events, which tend to be expressed with non-lexified constructions and framing/backgrounding constructions, which favor an explicit subordinator.
1 Introduction
What does it mean to claim that something happened WHEN1 something else happened? As a first approximation, it seems we are claiming that the two events overlapped temporally, but in fact there is a lot more going on if we look more closely at the range of situations covered by the English word when, which has been extensively studied. For example, it has been known in the literature at least since Partee (1984) and Hinrichs (1986) that when is compatible not just with overlap, but also with temporal inclusion, precedence and posteriority, while Sandström (1993) pointed out that when does not only express a temporal relation but also requires a certain discourse coherence relation (consequentiality, enablement, or similar) between the two events. In many respects, when functions as an unmarked temporal subordinator in partial competition with more explicit choices such as while, because, after etc.
In the following, we focus on two other distinctions relevant to WHEN that have been less well studied, probably because they are less salient in English grammar. First, there is the distinction between existential (1) and universal (2) readings, following the terminology of Sæbø (2011).2
(1) When I went to bed yesterday, I took a long time to sleep.
(2) When I went to bed, I usually took a long time to sleep.
In English, we can use adverbs like yesterday and usually to make clear what reading we intend. In German, for example, the same difference can be brought out by the choice of subordinator alone.3
(3) Als ich ins Bett ging, konnte ich nicht einschlafen. when.EX I in bed went, could I not sleep
(4) Wenn ich ins Bett ging, konnte ich nicht einschlafen when.UNIV I in bed went, could I not sleep
Second, temporal subordination through when can alternate with converb constructions,4 or with juxtaposition of two main clauses. In these cases, the temporal relation is brought out morphosyntactically (through the ing-form in a certain syntactic configuration), as in (6) or simply by the discourse configuration, as in (7). In neither case is the temporal relation lexicalized.
(5) When he arrived in Gaza Friday, Kandil pledged his support for the Palestinians.
(6) Arriving in Gaza Friday, Kandil pledged his support for the Palestinians.
(7) Kandil arrived in Gaza Friday. He pledged his support for the Palestinians.
In this paper we use Mayer and Cysouw's (2014) massively parallel corpus, which contains the New Testament in more than 1,400 languages, to explore the expression of WHEN cross-linguistically and see how the ground covered by English when is expressed across languages. The dataset we use is presented in Section 2.
To explore the data, we use probabilistic semantic maps, which are now a well-established tool in language typology for capturing universal correspondences between classes of forms and ranges of highly similar situational meanings across “massively cross-linguistic” datasets (Wälchli and Cysow, 2012). Probabilistic semantic maps can deal with very large datasets containing great degrees of variation within and across languages (Croft and Poole, 2008), and unlike traditional implicational semantic maps, they do not rely on a limited set of posited abstract functions and translational equivalents. These methods are described in more details in Section 3.
Finally, in Section 4 we analyze the semantic map and show how interesting cross-linguistic generalizations emerge, in particular regarding the distinction between existential and universal WHEN, and the use of competing constructions without a subordinator, such as main clauses and converbs. Section 5 summarizes and concludes.
2 Data
Mayer and Cysouw's (2014) massively-parallel Bible corpus comprises translations representing 1,465 ISO 639-3 language codes.5 As noted in Good and Cysouw (2013), an ISO 639-3 code should be understood as referring to a LANGUOID, a generalization of the term language referring to the grouping of varieties as represented in specific resources (DOCULECTS) without the common constraints associated with the definition of language, dialect or family. This is crucial for avoiding incurring into the misconception that the “languages” represented in our dataset are defined as such in virtue of their sociolinguistic status. Rather, each of them can be considered as sets of DOCULECTS at some level of hierarchical grouping. For practical purposes we will refer to the variety represented by each Bible translation in our parallel corpus as a “language”, with the caveat in mind that not all the varieties referred to by the ISO 639-3 codes will equally correspond to what is generally considered a “language”.6
Several of the languages in Mayer and Cysouw's (2014) parallel corpus have multiple translations and a few contain only (or predominantly) the Old Testament. To obtain the best textual coverage for the largest number of varieties possible, we only considered languages with a version of the New Testament. For languages with multiple translations, we first selected the New Testament version with the widest coverage in terms of verses. If the difference in coverage between versions was of <2,000 verses, the different versions were considered as having the same coverage, in which case the most recent one was selected.
Although Mayer and Cysouw's corpus already contains versions for some historical languages, for Ancient Greek, Church Slavonic, Latin, Gothic, and Classical Armenian we have used their versions from the PROIEL Treebank (Haug and Jøhndal, 2008) to facilitate the potential integration of their several layers of linguistic annotations in the semantic maps in future research.
Our final dataset comprises 1,444 languages (around 19% of the world's languages), representing, following the Glottolog classification, 121 families and 16 language isolates. In comparison, the world's languages are currently classified into 233 families and 167 isolates.7 Table 1 gives an overview of the language families most represented in our dataset compared to their frequency in the world's languages according to the Glottolog database. The top three families among the world's languages, the Atlantic-Congo, Austronesian, and Indo-European occupy the same position in our dataset and show similar relative frequencies to those found in Glottolog. We also see that the Nuclear Trans New Guinea, Quechuan, Uto-Aztecan and Mayan language families are overrepresented in our dataset compared to the world's languages. On the other hand, the Afro-Asiatic family is rather heavily underreprestented in our dataset, and the same goes for Pama-Nyungan, Austroasiatic, and Tai-Kadai families (not shown in the table). The families not represented at all in our dataset constitute around 48% of the world's families and comprise, for the most part, families with fewer than 10 languages.8
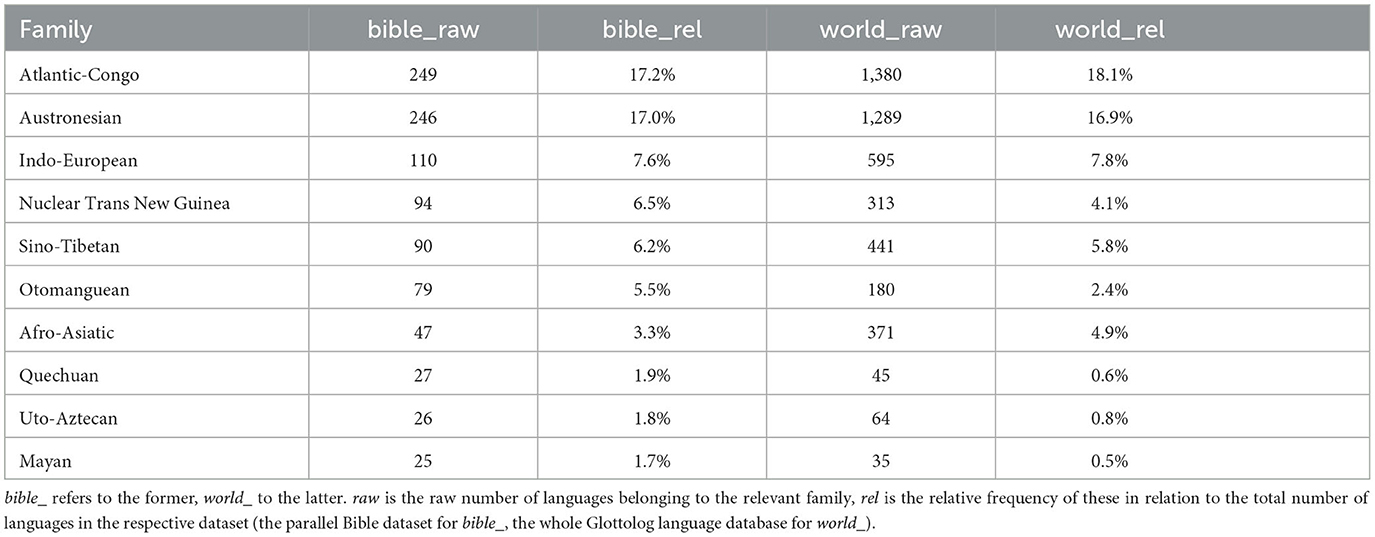
Table 1. The 10 most frequent language families in our dataset compared to the 10 most frequent families among the world's languages according to the Glottolog classification.
In terms of areal distribution, following the Glottolog classification into six main macro-areas (Africa, Australia, Eurasia, North America, South America, Papunesia), as Table 2 shows, languages from Africa and Australia are underrepresented in our dataset, while languages from the Americas are somewhat overrepresented. Figure 1 maps the distribution of the languages in our dataset among the world's languages.9 We see that although North America as such is overrepresented, most North American languages in the dataset are from Mexico or further south, and languages of the USA and Canada are underrepresented.
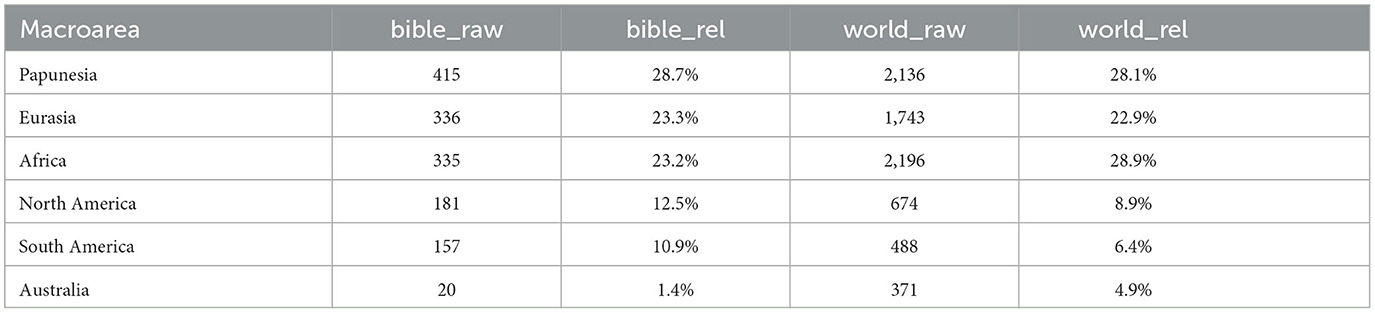
Table 2. Areal distribution of the languages in our dataset compared to the world's languages, following the classification into macroareas from Glottolog.
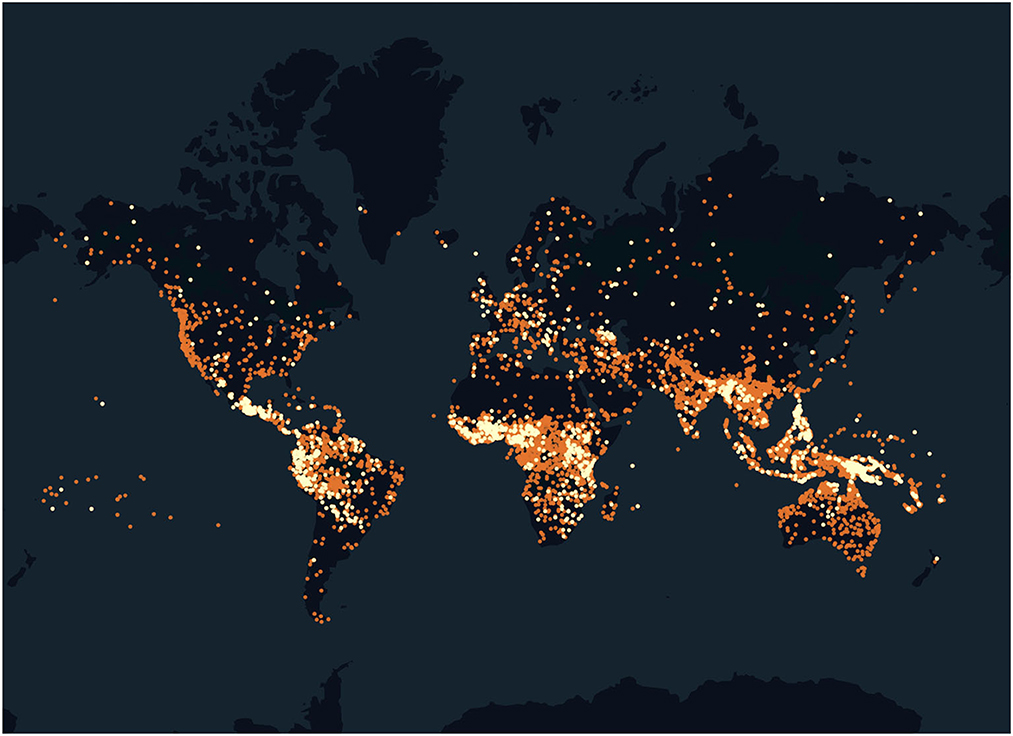
Figure 1. Approximate areal distribution of the languages in our dataset (light yellow) among the world's languages (orange).
3 Methods
We chose English, and in particular the word when as the source item because it is especially well-studied and known to be very general, i.e. cover a large semantic domain, as we saw in Section 1. The choice of trigger is important because it defines the domain of study: if we chose a subordinator that e.g. was only used with past tense, we would only be able to find cross-linguistic differences within that domain. Our choice of English when therefore has a similar motivation as Wälchli (2014)'s choice of Polish nie as a maximally general negation marker (without competetion from words like like nobody, nothing, never, cannot, as in English).
A potential weakness of starting from English when is that we are missing out on cases where English itself uses another means, such as a simple juxtaposition, or an ing-form as a converb. However, these constructions are extremely polysemous and cannot be reliably extracted from English alone. On the other hand, starting from English when allows us to identify cases where juxtaposition and converbs are used in this meaning across other languages, as we will see in Section 4.2.
The texts in the target languages were aligned to the English text at word-level, using SyMGIZA++ (Junczys-Dowmunt and Szał, 2012), a modification of the well-known GIZA++ program (Och and Ney, 2003) that allows training two-directed word alignment models in parallel. The results are one-to-one alignment models, namely one token in the source language corresponding to only one token, or no token at all, in the target language (as opposed to a one-to-many or many-to-one alignment).
SyMGIZA++ was first compared to the popular and much faster FastAlign model (Dyer et al., 2013), but the former was chosen after applying some heuristics to gauge the quality of their results. Rather than evaluating the alignment across the board,10 we checked a randomly selected subsample (10%) of all the sentences (= 876) containing when-clauses in English and calculated the accuracy of the alignment between the token when and its respective forms, or lack there of, in the Norwegian and Italian versions. SyMGIZA++ yielded 96.5% accuracy on Norwegian test set and 77.9% on the Italian one, whereas FastAlign only yielded 77.9 and 59.3%, respectively. Overall, SyMGIZA++ and FastAlign performed similarly at identifying the correct parallel when the target language uses a subordinator (e.g., when, while or after), but FastAlign generally aligned when to some other token in the absence of a direct parallel (e.g., to a conjunction or an auxiliary verb), whereas SyMGIZA++ more often explicitly indicated the lack of a parallel with a “NULL” alignment, which intuitively means that the target language uses a construction with no subordination (e.g., an independent clause) or a construction where the subordination is expressed morphologically (e.g., a converb).
Before training the final models with SyMGIZA++, minimal preprocessing (lowercasing and punctuation removal) was applied. We then extracted when and its parallels in all the target languages. Each occurrence of when and its parallels was treated as one usage point or, as we will say, one context for the hypothesized semantic concept WHEN, whose feature vector consists of the word forms used by each language, as shown in Table 3. Each row represents a context for the use of the concept WHEN. To measure the similarity between pairs of contexts, we use the Hamming distance, i.e., the number of language-specific word choices that you would have to change to make the contexts identical. For example, based on the six languages shown, the distance between the two contexts is 3, because they differ in the word choice in Maori (mri), Finnish (fin), and Kazakh (kaz).
In this way, we turn the alignment data into a matrix recording similarity between pairs of contexts. We then use classical Multidimensional scaling (MDS), as implemented by R's cmdscale function, as a way of rendering this distance matrix in a two-dimensional map. It should be noted that it is not possible to render our distance matrix faithfully in only two dimensions. MDS works in such a way as to order the dimensions by the amount of distance data that they capture, without any regard to human interpretability of the dimensions. In our case, the first two dimensions only capture around 15% of the distance data.11 This suggests that there is a lot of cross-linguistic variation in the use of WHEN that is not captured in the maps we analyze here. Nevertheless, we believe our approach is justified because it turns out that there is a relatively clear human interpretation of the map, as we will argue in Section 4. The fact that there are additional, orthogonal dimensions that influence the lexical realization does not invalidate this interpretation. Moreover, pairwise plotting of dimensions (3,4), (5,6), (7,8) and so on up to (19,20) shows that from dimension 9 onwards, the map looks like normally distributed (i.e., random) data, suggesting that it reflects free choice on the part of the translator. Therefore, in the rest of this paper, we only work on the first two dimensions of the MDS matrix. These can be plotted on a map as in Figure 2. Each dot represents context for WHEN (i.e., a Bible verse). If two dots are far apart, they tend to be expressed with different lexical items across the languages in the corpus.
Clusters of semantically similar observations are identified and analyzed in two main ways. First, similarly to Hartmann et al. (2014), starting from the MDS matrix, we apply Kriging as an interpolation method that uses a limited set of sampled data points (each observation in the target languages) to estimate the value of a variable in an unsampled location. As an example, Figure 3 shows the resulting semantic map for Doyayo (Atlantic-Congo, Africa) after applying Kriging to the MDS matrix by using the parallels to English when in the language to interpolate the areas shown in green, red, and yellow in the figure. Unlike Hartmann et al. (2014), we started from one single means (when), without pre-emptively assigning a semantic label to the different when-situations in English, so that the discernible Kriging-areas in the semantic maps of the target languages must be interpreted on the basis of comparison between similar cross-linguistic patterns. Like Hartmann et al. (2014), we used the function Krig from the R package fields (Nychka et al., 2021) to draw lines at different levels of probability distributions (35, 32, and 29%). Unlike traditional semantic maps, where boundaries are drawn around all observations of the same type (i.e., the same means in a given language), the lines in the Kriging map in Figure 3 represent the probability for a means to occur within those lines. This is why, for instance, red points in Figure 3 can also be found outside the red area identified by Kriging, but it also explains why relatively large areas can overlap, as the points between the red and yellow areas in the figure show.
Second, we fit a Gaussian Mixture Model (GMM) to the first two dimensions of the MDS matrix, to identify clusters which are more likely to correspond to separate universal functions of WHEN, regardless of how much variation a particular language shows within any of the clusters (which could go from no variation across the whole map or across one cluster, to several linguistic means in a single cluster).
GMM assigns data to a given number of clusters based on probability distributions rather than on the distance from a centroid, as in other well-established clustering algorithms (e.g., k-means). This allows for elliptical clusters, which may better approximate the semantic map of competing constructions, which are, by definition, more of a continuum than a set of clearly separate and spherical areas. The number of clusters (“components”) for the GMM models are chosen using the Silhouette score (Rousseeuw, 1987), the Akaike information criterion (AIC) (Akaike, 1974), and the Bayesian information criterion (BIC) (Schwarz, 1978). These methods are meant to indicate how many clusters are needed for the best trade-off between model fit and complexity, namely how many clusters can be generated while keeping them maximally separate from each other and internally consistent. However, empirically, we know that the temporal constructions under consideration are often competing and that their scopes are not at all clear-cut. With this caveat in mind, we focussed on the GMM model consisting of six clusters (Figure 4), which is the optimal number suggested by all three methods. While keeping this into account, we focussed on the GMM model consisting of six clusters (Figure 4), given the agreement between the Silhouette and the BIC/AIC scores.
Each cluster in Figure 4 potentially corresponds to a specific functional domain of WHEN. To test whether this is in fact the case, we check to what extent the languages have lexical items that align well with the GMM cluster. Concretely, we first extracted all the attested means used by each language for each particular cluster. For each attested means, we counted its occurrences in that cluster as true positives, its occurrences outside that cluster as false positives, and the occurrences of other means in that cluster as false negatives. The precision of a means as a rendering of the when-clauses corresponding to that cluster, then, is the number of true positives divided by the sum of true positives and false positives; the recall is the number of true positives divided by the sum of true positives and false negatives. We then computed the F1 score (harmonic mean of precision and recall) for each means and, for each language we plotted the precision and recall of the word with the highest F1 scores. A means with a high F1 score will correspond to a likely lexification of the relevant GMM cluster in its particular language. A frequent high F1 score across several languages may instead indicate a common lexification pattern, as we will see in Section 4.
The result is shown in Figure 5. Notice that a high precision item does not necessarily correspond to a likely expression of that GMM cluster if the recall is low. In many cases, these are just rare items (possibly false alignments by the model) that happen to be distributed inside one of the clusters. On the other hand, high recall with low precision means that the item in question expresses a more general concept than the GMM cluster. The extreme case of this is English when, which, due to how the data was sampled, has recall 1.0 for all clusters and a precision for each cluster that corresponds directly to its relative size.
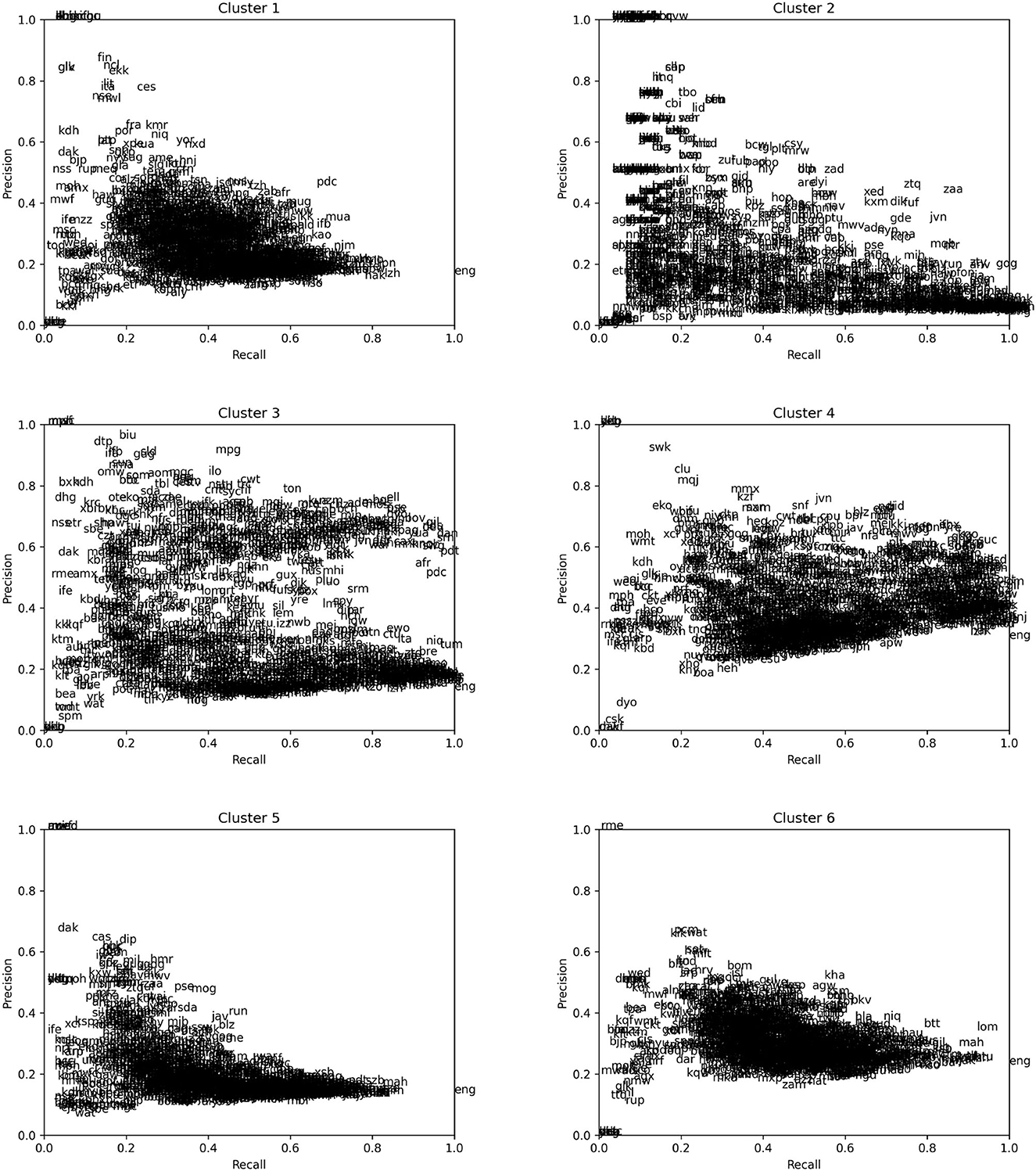
Figure 5. Precision and recall for the six GMM clusters shown in Figure 4. The x and y values (recall and precision, respectively) for each language in each of the subplots correspond to the recall and precision of the item with the highest F1 score in that language for that cluster (compared to all other items in that language occurring at least once within that cluster).
Finally, leveraging the advantages of both the Kriging and the GMM methods, we identified the Kriging areas that best correspond to each GMM cluster in each language. As we will see in Section 4, this will allow us to study patterns of coexpression across languages. The alignment of Kriging areas and GMM clusters runs as follows.
1. For each of n number clusters, across which cross-linguistic variation in coexpression is to be investigated, calculate its centroid. This is the sum of the coordinates of the points belonging to each cluster, divided by the number of points in the cluster, namely:
where j is the number of points in a GMM cluster, x are the x-coordinates (i.e., dimension 1 of the MDS matrix) and y the y-coordinates (i.e., dimension 2 of the MDS matrix). The centroid of a GMM cluster is preliminarily assumed to be the best representation of that cluster. Note that it is unlikely to correspond to an actual observation in the target languages.12
2. For each GMM cluster, extract k actual observations corresponding to the k-nearest neighbors of the centroid of that cluster. The value k should be adjusted in a trial-and-error fashion; we set ours to 30, i.e., 30 points are extracted for each cluster. The nearest neighbors were identified using the balltree approach (Omohundro, 1989), a space partitioning system which can be applied to multi-dimensional space for nearest neighbor search.13 The result of the search is a group of “core” points surrounding the centroid of the GMM cluster. Figure 6 shows the three groups from our experiment.
3. For each language, check which Kriging area, if any, contains each of the groups in Figure 6 and, for each language, create a dictionary to take note of the mapping between groups and Kriging areas.14 For example, the group of points corresponding to GMM cluster 3 in Figure 6 are contained within the Kriging area for Doyayo gɔ, those corresponding to GMM cluster 2 and 4 are contained within the Kriging area for yɔ. The resulting dictionary for Doyayo, then, is {group-3: gɔ, group-2: yɔ, group-4: yɔ}, meaning that all the points of each group are contained within one Kriging area only. This is the simpler scenario.
The more complex scenario is one in which more than one Kriging area include points from the same group. For example, the dictionary for Patep (Austronesian, Papunesia) is {group-3: [obêc, buc], group-2: buc, group-4: NULL}, meaning that the Kriging area for buc contains points from groups 2 and group 3, but points from group 3 are also found in the Kriging area for obêc. In such cases, we apply the following heuristics to infer whether more than one Kriging area should be considered meaningful in that group for the purpose of looking at patterns of coexpression.
a. If one of the two Kriging areas is unique to a given group (e.g., obêc in the Patep example), while the other is not (e.g., buc in the Patep example), consider the former as meaningful, regardless of how many points from that group it contains. Instead, consider the latter as meaningful only after running a test of proportion with the competing Kriging area. If it contains significantly more points than the competing area, or if the difference in proportion is not statistically significant, then both Kriging areas are kept in the dictionary. To determine this, we use a Fisher's exact test with α = 0.01. In the Patep example above, obêc is considered a meaningful Kriging area for group 3 because it is only found there. On the other hand, to decide whether to also keep the Kriging area for buc, we run a Fisher's test, which indicates that the difference in proportion is not significant (26 out of 30 points are found in the Kriging area for obêc, 21 out of 30 in the one for buc; p = 0.32), so both Kriging areas are considered meaningful lexifications for group 3.
b. If neither of two competing Kriging areas is unique to a particular group, then a Fisher's test is used to establish which one to consider meaningful. For example, the dictionary for Yucatec Maya (Mayan, North America) is {group-3: ken, group-2: [ken, ka], group-4: ka}. A Fisher's test indicates that the Kriging area for ken contains significantly more points from group 2 than the Kriging area for ka (p < 0.01), so the dictionary is modified to {group-3: ken, group-2: ken, group-4: ka}.
c. Give lexical items a greater weight than NULLs. Only consider a NULL Kriging area as meaningful if it is the only one containing a particular group. For example, the dictionary for Manam (Austronesian, Papunesia) is {group-3: [bong, NULL], group-2: bong, group-4: [bong, NULL]}, which, for the purpose of looking at lexification patterns is then modified to {group-3: bong, group-2: bong, group-4: bong}. On the other hand, the dictionary for Hills Karbi (Sino-Tibetan, Eurasia) is {group-3: ahut, group-2: ahut, group-4: NULL}, in which case the only Kriging area containing points from group 4 is a NULL area.
4. Assign patterns of lexification based on the Kriging areas considered meaningful for each group.
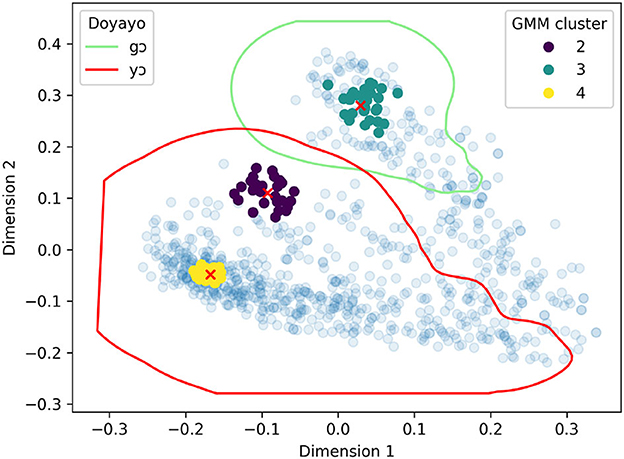
Figure 6. Result of the 30-nearest-neighbor search using the balltree method, with an example of its application to Doyayo (Atlantic-Congo, Africa). The red marks are the centroid of the respective GMM clusters (as represented in Figure 4), while the points in which they are embedded are their 30 nearest neighbors. The contour lines in green and red correspond to the Kriging areas for Doyayo gɔ and yɔ at 29% probability.
As shown in Figure 6, for example, the points in group 3 all fall within the Kriging area for Doyayo ɡɔ, while those in both group 2 and 4 are all contained within the Kriging area for yɔ. On the basis of this, we can assign languages behaving like Doyayo to a pattern in which the top left area (cluster 3) is the domain of one word, whereas the mid and bottom left areas are colexified by a different word. This—which we will call “pattern C” in the next Section—is one of five basic patterns which can be observed on the basis of the three groups of core points represented in Figure 6 (one for each possible logical combinations between the groups). An overview of the patterns will be given in Section 4.3.
4 Analysis
4.1 Grams
A gram, according to Bybee and Dahl (1989) is a linguistic item—a bound morpheme, a lexical item or a complex construction—with a specific function or meaning. The goal of our study is to identify grams that are similar across languages, what Dahl and Wälchli (2016) call a gram type, i.e., “a cluster of language-specific grams whose closeness in meanings and functions is reflected in similar distributions in a parallel corpus”.15 Together, the gram types make up the semantic atoms in the grammatical space that English when covers.
Kriging maps such as Figure 3 clearly bring out language-specific grams. Do the GMM clusters similarly reflect cross-linguistic gram types? We measured the fit of linguistic items in our corpus to the GMM cluster through precision and recall measures as shown in Figure 5. Elements that combine high precision and recall are good candidates as expressions of a GMM cluster, and if we find such candidates across many languages, we may reasonably conclude that the cluster represents a gram type. Looking at Figure 5, this is not the case with clusters 1, 5, and 6: the languages of these plots are quite dense, with little variation between languages, and almost no items with a high precision. Clusters 2, 3, and 4 are different and may better correspond gram types, which we will study more closely in Sections 4.3–4.5.
Approaching WHEN in terms of grams and gram types is in line with previous typological literature (Cristofaro, 2013), which defines WHEN-clauses in functional terms, classifying as such not only those introduced by specific temporal conjunctions (e.g., English when X did Y or when doing Y), but also clauses that are simply juxtaposed and whose function must be contextually inferred, as in (8).
(8) Canela-Krahô (Macro-Gê)
“My mother died when I was born” (Popjes and Popjes, 1986, p. 139, cited in Cristofaro, 2013).
In some other cases, languages may use specific verb forms to mark adverbial subordination, without, however, specifying their semantic relation to the main clause, This is the case of cross-linguistically well-attested converbs and predicative participles (Haspelmath and König, 1995), as in examples (9)–(10) from our data.
(9) Avar (North Caucasian)
“And when Jesus was baptized, immediately he went up from the water, and behold, the heavens were opened to him, and he saw the Spirit of God descending like a dove and coming to rest on him” (Matthew 3:16).
(10) Ancient Greek (Indo-European)
“And when they lifted up their eyes, they saw no one but Jesus only” (Matthew 17.8).
This morphosyntactic diversity strikes a clear chord with Dahl and Wälchli's (2016) remark that grams differ in how transparent they are, namely in how constant and isolable their form is, which has bearing on how easily they can be automatically identified via methods such as ours. English when is maximally transparent, as it is a single word with a constant form and can therefore be automatically identified with little obstacle. The Avar perfective converb marker -un (9) is much less transparent since it is not easily isolable and may be one of several possible converb markers in the language. Cases like the Ancient Greek predicative participle in (10) are maximally opaque since their form depends on the constituent in the matrix clause with which they agree. Moreover, while participles in their predicative function are similar to converbs, they can often also occur in other, e.g., attributive contexts.
4.2 Non-lexified constructions
In a large-scale study like ours, with no access to language-specific knowledge, it is difficult to identify such more opaque gram types and we make no attempt to do so. Instead such grams are captured as NULL alignments by our models, because there is no lexical item that can be aligned with when. Recall that such NULL alignments was the main advantage of the SyMGIZA++ tool.
A language with only NULL alignments should correspond to one which exclusively uses WHEN-clauses without any temporal connector. A language showing both NULLs and other means should indicate that the different WHEN-situations can be expressed either by a subordinate introduced by a connector such as when, or by juxtaposed verbal forms, potentially depending on the context or on the GRAM TYPE they belong to.
A question that immediately arises is whether NULL expressions/oblique gram types tend to cluster in a particular area of our semantic maps. The heatmap in Figure 7, which shows the concentration of languages expressing a particular data point with a NULL construction, indicates that this is in fact the case. We see that the closer we get to the lower right corner of the map, the more likely we are to get an oblique, non-lexified construction. Notice that the model does not “know” a priori that NULL values are in any sense ‘the same' across languages. Therefore, this clustering reveals that the model has detected a common usage pattern for non-lexified constructions. For example, 869 languages use a non-lexified construction in their equivalent to (11).
(11) And he took bread, and when he had given thanks, he broke it and gave it to them (Luke 22:19).
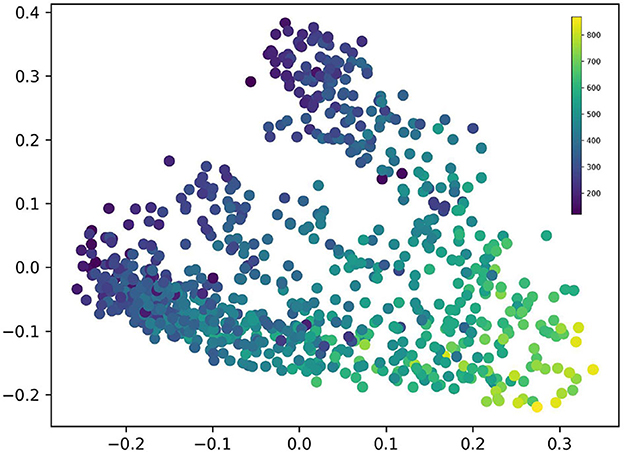
Figure 7. Heatmap showing the concentration of languages expressing a particular data point with a NULL/oblique construction.
Indeed many other English translations than the one we have in our dataset also use a non-lexified construction here (took the bread, gave thanks and broke it, and gave it to them).
By contrast, (12) is only expressed with an oblique construction in 120 languages.
(12) When all things are subjected to him, then the Son himself will also be subjected to him who put all things in subjection under him (1 Corinthians 15:28).
In the light of this, we interpret the left-right dimension of our semantic map as corresponding to a decreasing likelihood of lexified expression. It is likely that this reflect some semantic properties of the data points to the right, but since our corpus is not well suited for the study of oblique constructions, we leave this for future research and focus on the left-hand side of our map.
4.3 Distinctions on the left hand side
Looking now at the areas where a lexical construction is likely, we see that these stretch out from the lower right corner in three bands, whose end points correspond roughly to the GMM cluster centroids identified in Figure 6.
The Kriging maps show variation in how these areas are colexified in different languages. In Figure 8, for instance, there is an obvious overlap between Kako komɛ, Greek otan, Tuwuli ntɛ, and Kiribati ngkana. The Kriging area corresponding to these means suggests a relatively consistent cross-linguistic patterns of lexification. Similarly, there is some overlap between Kako ŋgimɔ and Tuwuli lɔkɔ, as well as between Kako ma, Tuwuli kĩ and Kiribati ngke. In this case, however, there seems to be more variation between the scopes of these overlapping means than between komɛ/otan/ntɛ/ngkana. There is also more variation in the colexification patterns among the mid and bottom left areas than at the very top of the map—Kiribati, for instance, colexifies the areas corresponding to Tuwuli lɔkɔ and kĩ, and to Kako ma and ŋgimɔ, whereas Greek ote colexifies the areas corresponding to lɔkɔ/ŋgimɔ and only part of the one for ma/kĩ.16 These examples of colexification from the Kriging maps are also reflected in the GMM model (Figure 4) to different extents. GMM cluster 3 clearly corresponds to a subset of the komɛ/otan/ntɛ/ngkana areas (Figure 8); cluster 2 to Tuwuli lɔkɔ and Kako ŋgimɔ; and so on.
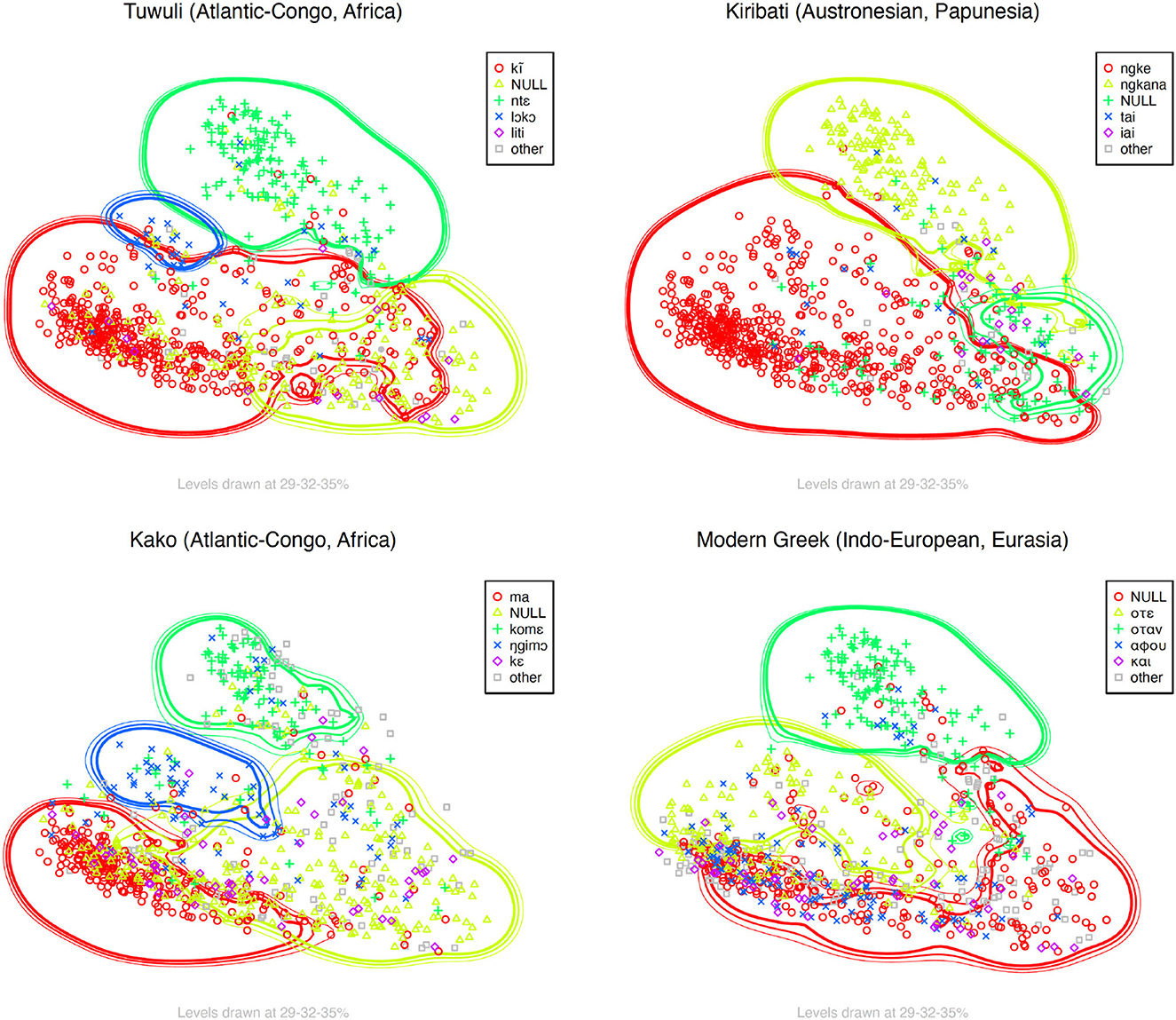
Figure 8. Kriging maps showing some of the variation in colexification between languages in the parallel corpus.
To understand these distinctions better, we examine the coexpression patterns in this area systematically. As explained in Section 3, we find for each language the Kriging area(s) that match the closest with the GMM areas we study (2, 3, and 4 from Figure 4) and extract the means that the languages use to express those Kriging areas.
In the majority of languages (1,165 out of 1,452), there is one Kriging area that is the best correspondence to each GMM area. For such languages, then, there are five possibilities concerning coexpression patterns. Table 4 shows these with their frequencies.
An additional 222 languages have significant competition with at least one more Kriging area within one of the three GMM cluster, but these can be subsumed to one of the five main patterns in Table 4, by considering whether each GMM cluster has at least one dedicated means (with its Kriging area) that is not also found in either of the other two GMM clusters. The updated frequencies with the addition of these 222 languages to the respective patterns are shown in Table 5.
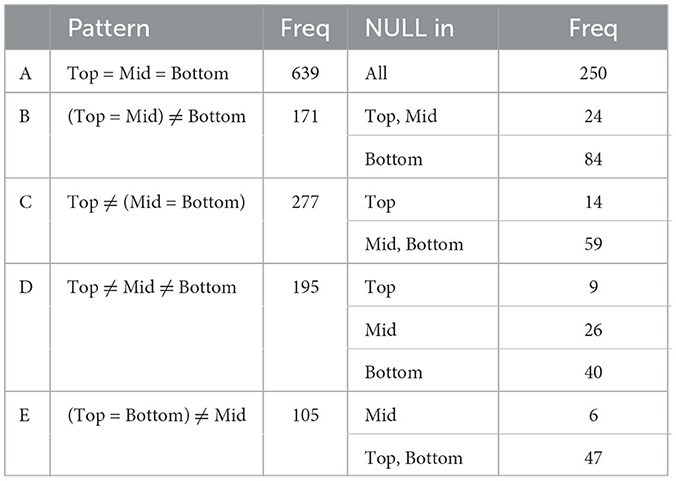
Table 5. Frequency of coexpression patterns, including subpatterns within a main pattern, across 1,387 languages.
Forty languages in the dataset have at least one GMM cluster in which there is no Kriging area (i.e., there is not one particular means that is significantly more prominent than others), so we are not able to assign them to any of the five main patterns. Finally, a small number of languages (17) have at least one main Kriging area per GMM cluster, but their pattern cannot be easily subsumed to any of the five main patterns.
We see that the most common case is that no distinctions are being made, i.e. pattern A (e.g. Serbian and Moose Cree in Figure 9). In about 40% of these languages, NULL is used for all three areas. Given the higher likelihood to use NULL constructions on the right-hand side of the map, it is likely that this is the case for the whole map for those language. Pattern D distinguishes all three areas. Here, NULL values are much less common, except in the lower area.
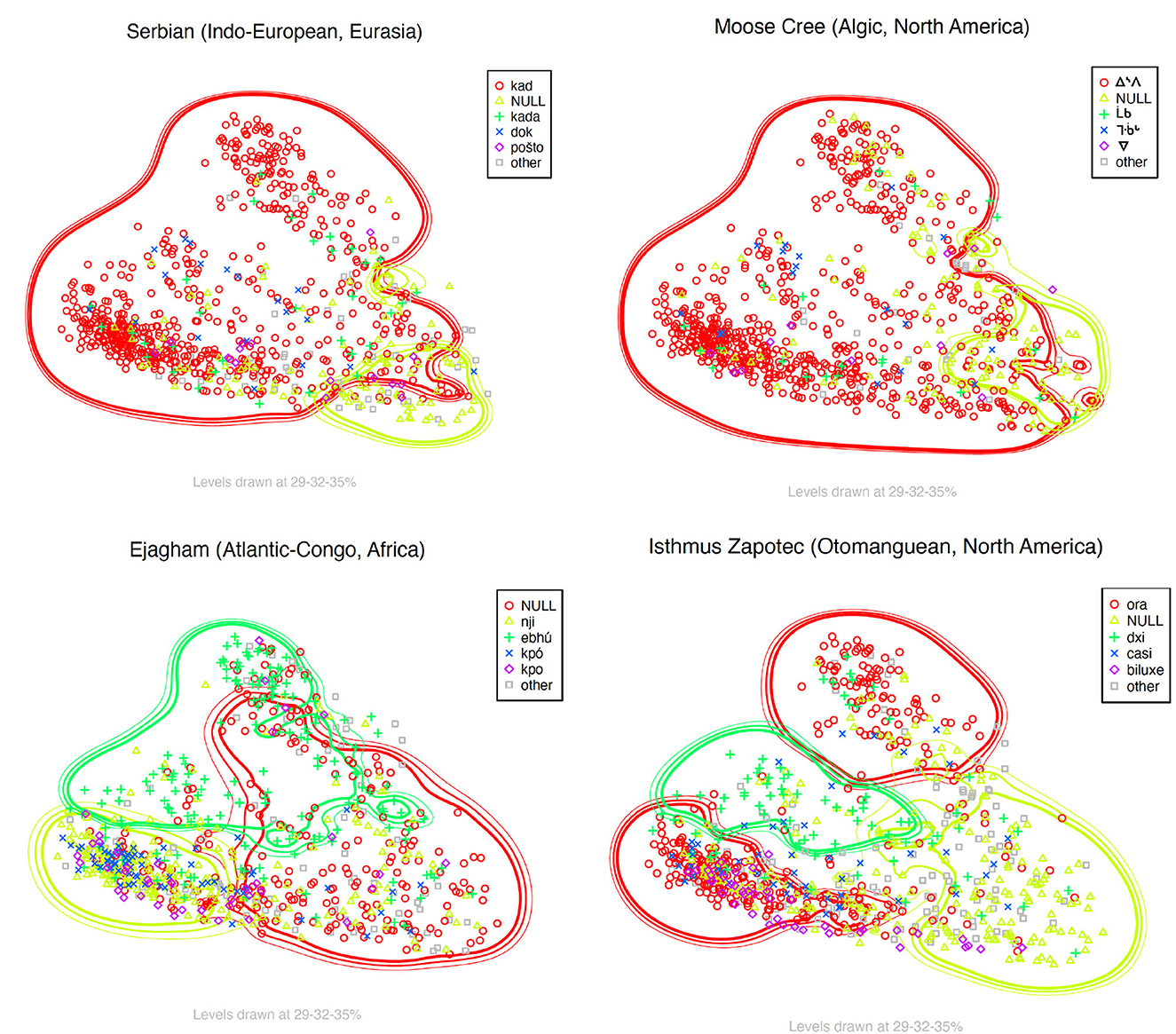
Figure 9. Examples of Kriging maps for pattern-A (Serbian and Moose Cree), -B (Ejagham) and -E (Isthmus Zapotec) languages.
Of the three patterns where two areas are colexified, the least common is E, where the top and the bottom area are expressed by the same means. This is as expected given that these two areas are not contiguous in our map. There is also an interesting asymmetry between patterns B and C, i.e., whether the middle area is colexified with the upper or the lower area. Two hundred and seventy-seven languages show pattern C, where the upper cluster has a dedicated expression, while 173 languages have pattern B with a dedicated expression for the lower cluster. Between these two patterns, the former is less common and in half of the pattern B languages, the dedicated expression for the lower cluster is NULL. In contrast, this is quite uncommon for pattern C, considering the overall frequency of the pattern among the languages.
In sum, this means that if a language has a separate, non-NULL lexification of one of the three areas on the left-hand side of the map, it is overwhelmingly more likely to be found in the upper area. Tentatively, we take this to mean that this is where where lexical items are often recruited. Given the much lower frequency of non-NULL items in the bottom, it is tempting to think that this pattern often results from the spread of an item that was orginally reserved for the top area down to the middle area as well. This pattern of change is attested in North Germanic: in Norwegian, the distinction between universal når and existential da is disappearing and it is the universal variant that is generalized. The same change happened in standard Modern Greek, as we saw in footnote 16. Our maps suggest that this may be a more common pattern than the opposite, but this must of course await confirmation.
4.4 Cluster 3: universal WHEN
The precision/recall plot for Cluster 3 in Figure 5 is especially interesting. The languages here divide into two bands, one with low precision (between 0.1 and 0.3) and one with high precision (≥ 0.5). Both bands stretch across the whole range of recall from 0 to 1. This clearly indicates that cluster 3 approximates a real gram type that exists in a range of languages but not in others.
Let us first observe that there are many language families represented among the items that have high precision and recall for cluster 3 (see Figure 10). We find items from Afro-Asiatic, Arawakan, Atlantic-Congo, Austroasiatic, Austronesian, Central Sudanic, Chibchan, Chiquitano, Eastern Trans-Fly, Indo-European (in particular Germanic and Greek), Kru, Lengua-Mascoy, Mande, Mayan, Nilotic, North Hamahera, Otomanguaen, Paba-Yagua, Sino-Tibetan, Songhay, and Ticuna-Yuri, as well as some creoles and isolates that have both precision and recall ≥ 0.5. This indicates that cluster 3 corresponds to a gram type that is relatively wide-spread across language families.
But while the GMM clusters are statistically optimal clusters that may approximate the cross-linguistic usage of a gram type, they tell us nothing about the meaning of that gram. For that we must inspect the items that match well with the relevant cluster. In the case of cluster 3, we see that some of the best matches are found across a range of Germanic languages, in particular Danish and Norwegian når and German wenn. These items express universal WHEN as in (2) and (4). What Figure 10 shows, then, is that this distinction is not a random feature of some Germanic languages, but actually found across the globe.
Since the GMM clustering is but a statistically optimal grouping of usages, it makes sense to instead use one of the words that best correspond to this cluster as an examplar. We choose the German word wenn. German is particularly interesting in this respect because it quite clearly carves up the semantic space of English when in two domains expressed by wenn and als, as shown in Figure 11. Notice that we are not actually using wenn/als as the source here, as we are still restricting attention to correspondents of English when: that is, we are looking at how often words of other languages correspond to German wenn in cases where both words correspond to English when, and we exclude e.g. cases where German wenn corresponds to ‘if', as it can also do.
To find the best correspondents to wenn, we proceed in the same way as for the GMM clusters. We treat the set of occurrences of wenn within the when-map as a cluster and we extract all the attested means used by each of the other languages for that cluster. For each attested means t, an instance in the when-map is then a true positive if it is rendered by t and s, a false positive if it is rendered by t but not s, a false negative if it is rendered by s but not t, and a true negative if it is not rendered by either s or t. We then compute the precision, recall and F1 score of t as a rendering of s, and, like before, we plotted the precision and recall of the word with the highest F1 score within each language. The result is shown in Figure 12.
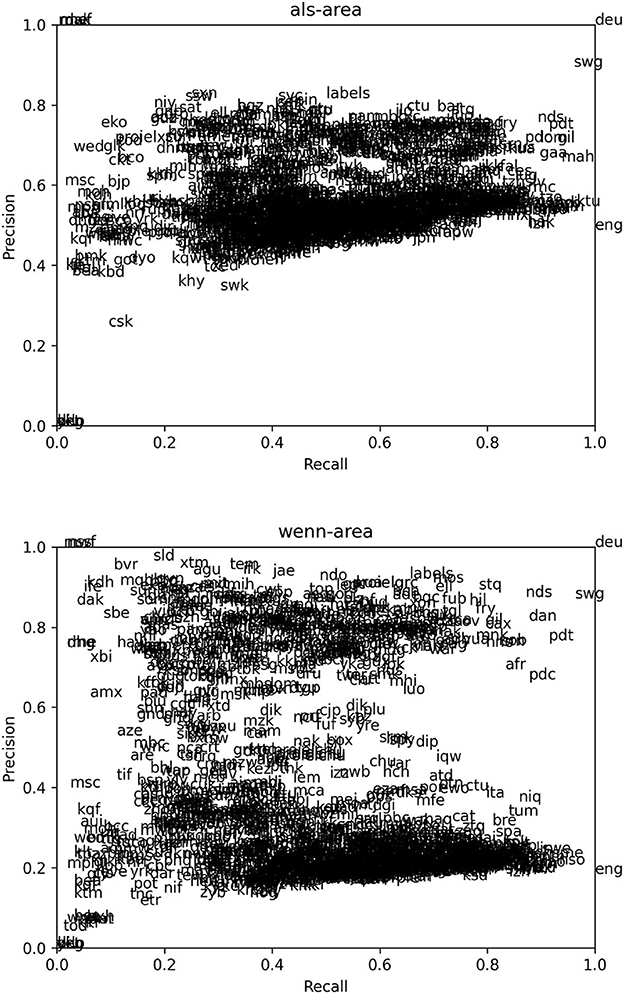
Figure 12. Precision and recall for the best match to German wenn and als in each language in the dataset.
The correspondents to wenn in Figure 10 show a similar split as the correspondents to cluster 3 in Figure 12, but much more pronounced. In Figure 10 the band of high-precision correspondents start at around 0.5, whereas in Figure 12 it starts at around 0.65. This suggests, not surprisingly, that the distribution of the German word wenn is a better approximation to the relevant cross-linguistic concept than the GMM-produced cluster. To see the distribution of wenn-equivalents across the world, we can use the F1 score to plot a heatmap of how good the best wenn-equivalent is in each language. The result is shown in Figure 13, where we observe clear areal clusters in Europe and in Indonesia/the Philippines as well as a less pronounced cluster in West Africa.
The higher correspondences to wenn, compared with the correspondence to GMM cluster 3, yields some practical justification for focusing on the German word. But of course it is a completely arbitrary choice and we could equally well have chosen Modern Greek (Indo-European, Eurasia) otan, Tuwuli (Atlantic-Congo, Africa) ntɛ, Kiribati (Austronesian, Papunesia) ngkana, or Tektiteko (Mayan, North America) oj, all of which have slightly higher F1-scores for cluster 3. On the other hand, it is unlikely that a different choice would yield a different result: after all, the F1 correspondence rates of these words both as measured to German wenn and to GMM cluster 3, were quite high.17
This shows that doing typology purely from parallel corpus data has both strengths and weaknesses. On the one hand, we are able to identify gram types of cross-linguistic relevance directly from the data: the two bands in Figure 5 tell us that there is a gram type that some languages (in the upper band) care about and other languages (in the lower band) do not care about. It does not, however, tell us anything about the meaning of that gram type: it is a purely extensional approach to gram types, identifying them with a set of usage points. In this sense, they are token-based comparative concepts (Haspelmath, 2019, p. 88) although we would like to stress that the tokens do not provide a concept; this rather comes from the post-hoc examination of the map by linguists. The raw map itself is inherently probabilistic: the usage points of language-specific grams may correspond more or less well to a gram type and if we slightly alter the set of usage points that represent the type, we will only slightly alter the match statistics. We do not have a priori access to, say, a comparative concept (Haspelmath, 2010) that could tell us whether to include a particular usage point in a gram type.
A convenient—but merely a convenient—way out of this is to pick a good correspondent from one of the sampled languages. As a very crude simplification, we could think that the meaning of cluster 3 is similar to the representative that we have been using, German wenn. However, since our study started from English when, meanings of wenn that are not translation equivalents of when are not captured, i.e., most prominently the conditional meaning of wenn “if”. Restricting attention to temporal wenn, this meaning is often described as referring to repeated events in past, present or future (i.e., what we have called “universal WHEN”), or singular events [i.e., what we have called “existential WHEN”, but only in the future (Fabricius-Hansen and Sæbø, 1983, p. 2)], since als is used for existential WHEN in the past. But since we picked German wenn more or less arbitrarily as a representative of cluster 3, we should be wary of assuming that it represents the meaning of this gram type cross-linguistically—the more so since its description is essentially disjunctive (wenn is existential in the future or universal in any tense).
To dig deeper, we can instead inspect the corpus underlying our study. This corpus can offer data about possible usages of a gram type: if a form occurs in a particular Bible verse in a particular language, that means it can express the relevant meaning. And so we may try to reconstruct the core meaning from the corpus sentences. To do so we proceed in two steps. First, we extract the item in each language that is the best match (as measured by F1) to cluster 3 and rank each data point by the number of such top-ranked items that are used to express it: the highest ranked items can be said to be prototypical usages of cluster 3. Based on these, we may then try to extract a comparative concept. In so doing, we leave the domains of quantitative typology and so we will not pursue this approach in depth here. But it is interesting to note that among the prototypical examples we find both universal WHEN in the present (generic) tense and existential WHEN in the future tense
(13) But when you give a feast, invite the poor, the crippled, the lame, the blind, (Luke 14:13).
(14) and he said Jesus remember me when you come into your kingdom (Luke 23:42).
This shows that the lumping together of the existential WHEN in the future with the universal WHEN, which could appear to be an accident of German, is actually found across the languages that make a distinction between existential and universal WHEN, suggesting that we should look for a unified concept. In this way, quantificational typology does bring up an issue that is relevant for the semantic analysis, although it does not resolve it.
4.5 Clusters 2 and 4
Just like German wenn is a good representative of GMM cluster 3, als is a good match for the union of clusters 2 and 4. However, the cross-linguistic correspondences to als show a very different pattern, as is clear from Figure 12. Where wenn clearly splits languages in two according to whether they have an equivalent or not, als does not induce such a clear split. Instead, all languages seem to have a reasonably good equivalent to als, though never as good as the equivalent to wenn.
One reason for this is plausibly that als covers more ground, and indeed corresponds to two of our GMM clusters. This functional heterogeneity is also visible in our inner-German semantic map. As is clear from Figure 11, the als area displays quite some variability; in addition to als, not only NULL values, but also und and da are reasonably frequent. By contrast, the wenn area is quite homogeneous: there are a few null values and two instances of und, but otherwise wenn reigns alone. In other words, wenn is (almost) obligatory as the expression of universal WHEN, whereas existential WHEN can be expressed in several different ways. Therefore, we cannot expect to find equally good matches to als as to wenn: if a language uses the same expression for most of the upper region of the map, that will be a good equivalent to wenn, but if it uses the same expression for most of the lower region, it will be a less good equivalent of als.
This may mean that we cannot expect the difference between cluster 2 and 4 to correspond to a clear-cut functional difference like the one we found for cluster 3, which is also more distant on the semantic map. Instead, we are probably dealing with a more gradual distinction. Given the analysis of the left-hand side in Section 4.3, it seems likely that as we move toward the bottom, i.e., cluster 4, we are more likely to find nonfinite forms.
Among the languages in which NULL constructions are predominant in GMM cluster 4, while the top and mid left areas are lexified (i.e., pattern-B or -D languages in our classification), we find that languages with converbs (or converb-like forms) or known for allowing serial constructions are particularly frequent. Among pattern-D languages, for instance, we find numerous West African languages, where extensive use of serial verb constructions is a well-known prominent feature (cf. Stahlke, 1970; Lord, 1973; Bamgboṣe, 1974; Awoyale, 1987; Givón, 2015), as well as Yabem (cf. Bisang, 1995) and several other Austronesian and Papuan languages (cf. Conrad and Wogiga, 1991; Senft, 2004), also oft-cited for their use of verb serialization. Among pattern-B languages we find several North and South American language families, such as Arawakan, Aymaran, Chibchan and Tupian, all of which have also been studied with respect to their use of serial constructions (cf. Aikhenvald and Muysken, 2010). Languages known to have converbs, such as Korean and Avar, or predicative participles functionally very similar to converbs, such as Ancient Greek, are also among pattern-B or -D languages in which NULL constructions are predominant in GMM cluster 4.
Our intuition is that the situations found at the bottom half of the semantic map are more likely to be found as part of a longer series of sequential events which can be expressed with serial verb constructions or clause chaining by the languages where these are possible.
As already mentioned, the way in which our data was sampled (i.e., starting from a single, albeit relatively underspecified, lexified means, namely English when) does not allow us to say much about cross-linguistic correspondences between different types of NULL constructions. However, we can formulate hypotheses on the basis of languages for which more granular information on NULL constructions is available, which is the case for the historical Indo-European languages in the PROIEL Treebank. Ancient Greek, for example, is well-known for making extensive use of participial forms which, when used co-predicatively, function much like converbs in that, among other things, they are most often controlled by the subject of the superordinate clause and their precise semantic relation to the main clause can only be contextually inferred (cf. Haspelmath, 1995, p. 17–20). Also often occurring as a parallel to English when in our data are so-called absolute constructions, which are similar to predicative participle constructions in that they involve a participle and function as “semantically indeterminate adverbial modifiers” (Haspelmath, 1995, p. 27), but unlike predicative participles their subject is not controlled by an argument of the matrix clause.
The discourse functions of co-predicative participle and absolute constructions in Ancient Greek can partly be inferred compositionally from the relative order and tense-aspect of participle and matrix clause (Haug, 2012). This allows us to single out their usage as foreground clauses (INDEPENDENT RHEMES in Bary and Haug's (2011) terminology), which are very similar to independent clauses from the discourse perspective and can be found stacked up in relatively long sequences leading up the finite matrix clause (i.e., clause chaining in the definition of Dooley, 2010, as in (15), and background clauses (FRAMES, in Bary and Haug's (2011) terminology), which set the stage for the matrix event and are thus not strictly part of the main line of events, as in (17).
(15)
(16)
The INDEPENDENT RHEMES which we can mainly expect to correspond to English when-clauses in our dataset are examples like (17), where the when-clause in the English Standard Version (i.e., the translation we used as source text) may also easily correspond to an independent clause in other English translations (as in the New International Version, provided in the example), since it is clearly part of a series of sequential, ordered events.
(17)
We can identify typical INDEPENDENT RHEMES (i.e., foreground participle clauses) and FRAMES (i.e., background participle clauses) among NULL alignments in Ancient Greek by using the linguistic annotation in PROIEL18 and test the intuition, offered above, that the situations found at the bottom half of the semantic map are more likely to be found in series of sequential foregrounded events.
Prenuclear perfective participles in the Ancient Greek New Testament are most typically INDEPENDENT RHEMES and were therefore labeled as such. Absolute constructions regularly occur sentence-initially, often introducing clause chaining constructions, and can instead be considered typical FRAMES regardless of tense-aspect (cf. Pedrazzini, 2022). We ran Kriging on the newly labeled data points and obtained the map in Figure 14 (the remaining NULL alignments are labeled as “other_NULL”). For ease of comparison, a map highlighting all and only the NULL observations belonging to the Kriging areas for FRAMES and INDEPENDENT RHEMES is also included.
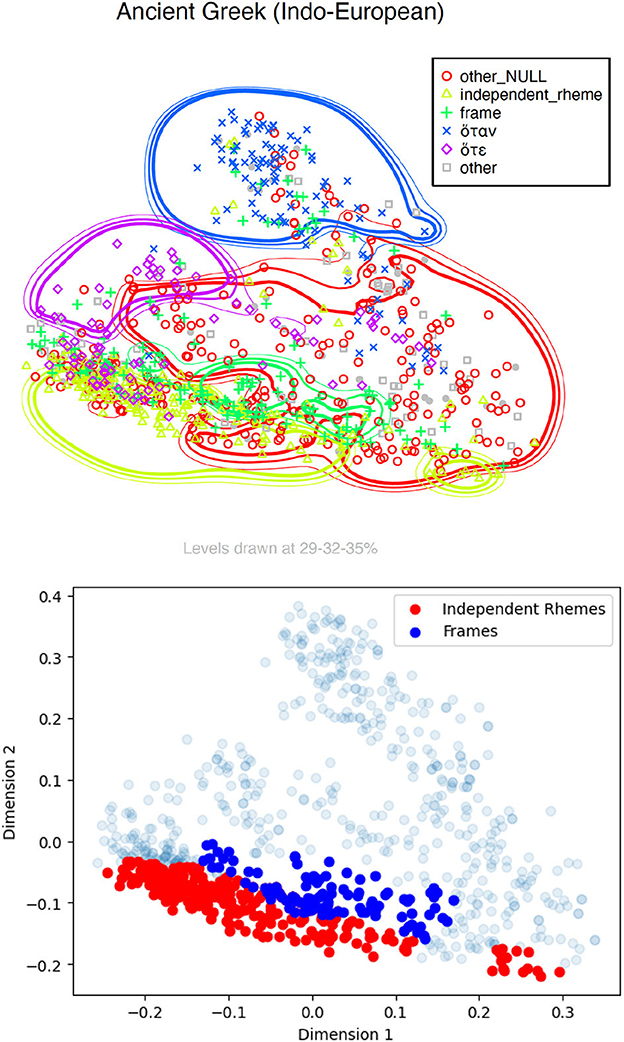
Figure 14. Kriging map for Ancient Greek with labeled FRAMES and INDEPENDENT RHEMES. The bottom map highlights only the points inside the Kriging areas for FRAMES (blue) and INDEPENDENT RHEMES (red).
As the figures show, our intuitions seem to be largely confirmed: typical INDEPENDENT RHEMES and FRAMES (or, in other terms, foreground and background matter, respectively) are predominant in a dedicated Kriging area at the bottom half of the map, stretching out from the area corresponding to GMM cluster 4 toward the right side of the map, where other, non-further-defined NULL constructions are found. INDEPENDENT RHEMES and FRAMES each receive a contiguous, but relatively well-defined Kriging area. It is interesting to notice that typical FRAMES are found above INDEPENDENT RHEMES in the map, i.e., closer to lexified WHEN-clauses. The connective when (and similarly lorsque and quand in the literature on French) have been widely recognized as “triggers” or “clues” for backgrounding rhetorical relations in formal frameworks of discourse representation (Reese et al., 2003; Asher, 2004; Prévot, 2004; Asher et al., 2007), namely as introducers of a background frame for a foregrounded event(uality). If an equivalence be made, in discourse-structural terms, between hote/hotan and when as Background-triggers, then the relative greater closeness of FRAMES (which are also background, but expressed by NULL forms in Ancient Greek) to hote and hotan in the map in Figure 14 adds a further layer of distinctions within the when-map—that between backgrounds and foregrounds.
These results are, of course, preliminary. More granular, larger-scale annotation on more constructions and for more languages will be needed to confirm whether the background-foreground distinction can help explain the distribution of NULL constructions in the when-map cross-linguistically.19
5 Conclusions
In this article, we have explored the semantic space of temporal connectives in a huge parallel corpus of Bible translations, starting from English when. We generated a distance matrix and applied multidimensional scaling to it following the by now standard method of generating probabilistic semantic maps from parallel data. We also explored these maps with the help of Kriging, following the methods used by Hartmann et al. (2014).
Because we start from a single means, English when and no further annotation, it is not trivial to get sense distinctions out of the data. We therefore tried to fit a GMM to the MDS map to identify clusters that might correspond to distinct universal functions of WHEN and used precision/recall-measure to gauge how well these clusters fit to the data.
To our knowledge, these method has not been used before to explore semantic maps from parallel language data. The results are tentative, but—we believe—promising. In particular, we find relatively clear evidence for a cross-linguistic gram type expressing universal WHEN. This gram type is well-known from Germanic languages, but our data show that it is present in a wide range of languages from a variety of language families as shown in the map in Figure 13. Moreover, a striking feature of the Germanic gram is that it is used both for repeated events in the past, present or future, and for singular events in the future. Other languages in our sample seem to follow the same pattern, suggesting that this colexification is not an accident.
Another clear finding in our data is that non-lexified constructions (e.g., converbs and simple main clause juxtaposition) do cluster in particular regions of the semantic map. This means that they are not equally viable as alternatives to any use of WHEN, but carry particular meanings that make them less suitable for some functions of WHEN. Our raw data are not well suited to further investigations in this area because we are unable to distinguish different non-lexified constructions. However, drawing on the PROIEL corpora, which have a richer annotation and contains the New Testament text in its Greek original (as well as several translations), we were able to suggest that non-lexified constructions are most likely to be foregrounded material whereas backgrounded (framing) material appear closer to explicitly subordinated sentences in Greek.
Our maps show no traces of other underspecified distinctions of English when, such as different temporal relations or coherence relations that are not purely temporal. We speculate that this is due to the way the data was sampled, since these are distinctions that when underspecifies, but for which there are explicit competitors (such as after, while, because etc.) that are not included in our data sample.
Finally, we also tried to match the GMM clustering and the Kriging to explore colexification patters across languages. We find tentative evidence that the top cluster (universal WHEN) spreads downwards toward existential WHEN more often than the opposite, but this must await independent confirmation.
Future research may build on our preliminary results by incorporating more detailed annotation on a number of areally and genealogically distinct languages for which the usage of different NULL construction has been studied, similarly to what we did for Ancient Greek in Figure 14. This might help make safer observations about the presence of one or several gram types within the high-variation semantic space corresponding to the bottom half of the semantic map analyzed in this paper.
Data availability statement
The datasets of English when and its parallels, as well as the code to reproduce the analysis and the Kriging maps for all the languages, can be found in the associated Figshare repository (https://doi.org/10.6084/m9.figshare.22072169).
Author contributions
All authors listed have made a substantial, direct, and intellectual contribution to the work and approved it for publication.
Acknowledgments
We grateful to Michael Cysouw for sharing the repository with the full parallel Bible corpus.
Conflict of interest
The authors declare that the research was conducted in the absence of any commercial or financial relationships that could be construed as a potential conflict of interest.
Publisher's note
All claims expressed in this article are solely those of the authors and do not necessarily represent those of their affiliated organizations, or those of the publisher, the editors and the reviewers. Any product that may be evaluated in this article, or claim that may be made by its manufacturer, is not guaranteed or endorsed by the publisher.
Footnotes
1. ^We use small caps WHEN to refer to the semantic concept, and italicized when for the English lexical item.
2. ^In effect, examples like (1) almost always refer to events that are known or inferrable from the previous discourse, so that definite may be more apt than existential but we stick with the previous terminology here.
3. ^The glossing abbreviations follow the Leipzig glossing rules, with the addition of AOR, aorist; EX, existential; IMPF, imperfect; PTC, particle; UNIV, universal.
4. ^We understand converb constructions in the sense of Haspelmath (1995, p. 3) as “nonfinite verb forms whose main function is to mark adverbial subordination”. Converbs are “part of the inflectional paradigm of verbs” and “cannot be analyzed as a verb plus a subordinator”, but are “inherently subordinate” (Haspelmath, 1995, p. 4).
5. ^As of January 2023.
6. ^The Glottolog database (https://glottolog.org; Nordhoff and Hammarström, 2011; Hammarström et al., 2023), for example, which adopts a DOCULECT-based approach while also grouping languoids into successively larger “levels” (such as subdialects, dialects, languages, subfamilies and families) classifies 15 of the languages in our dataset as dialects. Norwegian Bokmål (NOB) and Norwegian Nynorsk (NNO), for example, are considered “dialects” of Norwegian (NOR), even though the latter is in fact defined collectively by the combination of the former two (among other “dialects”). “Norwegian” (NOR), then, could therefore be considered as a languoid at a higher hierarchical level than the languoids Norwegian Bokmål and Norwegian Nynorsk.
7. ^These numbers do not include some of the “non-genealogical trees” to which some languages are assigned to by Glottolog, specifically UNCLASSIFIABLE, UNATTESTED, and SPEECH REGISTER. SIGN LANGUAGES, MIXED LANGUAGES, and PIDGINS are instead considered in the numbers and they are therefore counted in the frequencies in Table 1. So-called BOOKKEEPING LANGUOIDS are also excluded from the counts. These exclusions explain why the figures reported here are slightly different from those reported on the Glottolog webpage (https://glottolog.org/glottolog/glottologinformation).
8. ^The complete list of the world's language families used to extract the counts reported here, their frequency according to Glottolog and in our dataset can be found in the data repository (https://doi.org/10.6084/m9.figshare.22072169).
9. ^The points in the maps are obviously approximation of where a particular language is used. The coordinates for the map in Figure 1 are from Glottolog.
10. ^Because of the sheer number of languages in the parallel corpus, some bias in the evaluation method will necessarily be introduced, since it requires familiarity with both source and target language.
11. ^Since our distance data is not in fact embeddable in Euclidean space (of any dimension), the exact measure depends on how we treat negative eigenvalues in the decomposition of the centered distance matrix. The GOF measure in cmdscale report 15.7 and 15.9% when we replace negative eigenvalues by their absolute value or by zero, respectively.
12. ^The procedure can in principle have groups of observations drawn from clusters obtained with any method as a starting point. A group can also be made of one individual observation.
13. ^We used the implementation of balltree by Scikit-Learn (Pedregosa et al., 2011).
14. ^To obtain this information, we used Kriging areas at 29% of probability.
15. ^We speak loosely of gram types here, and do not want to claim that they are actually existing universal categories rather than grams that fall under some comparative concept that linguists find useful.
16. ^Notice incidentally that the Greek data illustrates the point that we are dealing with doculects here. In standard Modern Greek, ote has disappeared and has been replaced by otan in all contexts. However, the conservative Bible translation in our corpus still uses ote.
17. ^The precision and recall plot for the best correspondence to GMM cluster 3 in Modern Greek, Tuwuli, Kiribati, and Tektiteko (all indeed very similar to the plot for German) can be found in the data repository (https://doi.org/10.6084/m9.figshare.22072169).
18. ^PROIEL contains morpho-syntactic and dependency annotation, which allows us to easily identify absolute constructions and co-predicative usages of participles.
19. ^In this regard, we should highlight recent experiments in Pedrazzini (2023), where very similar patterns to the one in Figure 14 were also found in the when-map of clause-chaining languages such as Huichol (Uto-Aztecan) and Amele (Nuclear Trans New Guinea). Similarly to our Ancient Greek experiment, Pedrazzini (2023) automatically identified switch-reference markers in the semantic map of when and found that different-subject markers (widely attested to be also used independently as markers of background clauses and clause-linkage in clause chaining; cf. Stirling, 1993; AnderBois and Altshuler, 2022; AnderBois et al., 2023) and same-subject markers (also known to independently mark foreground clauses) largely overlap, respectively, to FRAMES and INDEPENDENT RHEMES as identified in the map in Figure 14.
References
Aikhenvald, A., and Muysken, P. (2010). Multi-verb Constructions: A View from the Americas. Leiden: Brill.
Akaike, H. (1974). A new look at the statistical model identification. IEEE Trans. Automat. Contr. 19, 716–723. doi: 10.1109/TAC.1974.1100705
AnderBois, S., and Altshuler, D. (2022). Coordination, coherence and A'ingae clause linkage. Proc. SALT 32, 793–813. doi: 10.3765/salt.v1i0.5331
AnderBois, S., Altshuler, D., and Silva, W. D. L. (2023). The forms and functions of switch reference in A'ingae. Languages 8. doi: 10.3390/languages8020137
Asher, N., Prévot, L., and Vieu, L. (2007). Setting the background in discourse. Discourse 1, 1–29. doi: 10.4000/discours.301
Bary, C., and Haug, D. T. (2011). Temporal anaphora across and inside sentences: the function of participles. Semant. Pragmat. 4, 1–56. doi: 10.3765/sp.4.8
Bisang, W. (1995). “Verb serialization and converbs – differences and similarities,” in The New Psychology of Language, eds M. Haspelmath, and E. König (Berlin; New York, NY: Mouton de Gruyter), 137–188.
Bybee, J. L., and Dahl, Ö. (1989). The creation of tense and aspect systems in the languages of the world. Stud. Lang. 13, 51–103. doi: 10.1075/sl.13.1.03byb
Conrad, R. J., and Wogiga, K. (1991). An Outline of Bukiyip Grammar. Canberra, ACT: Pacific Linguistics.
Cristofaro, S. (2013). “‘When' clauses,” in The World Atlas of Language Structures Online, eds M. S. Dryer, and M. Haspelmath (Leipzig: Max Planck Institute for Evolutionary Anthropology).
Croft, W., and Poole, K. T. (2008). Inferring universals from grammatical variation: multidimensional scaling for typological analysis. Theor. Linguist. 34, 1–37. doi: 10.1515/THLI.2008.001
Dahl, Ö., and Wälchli, B. (2016). Perfects and iamitives: two gram types in one grammatical space. Letras Hoje 51, 325–348. doi: 10.15448/1984-7726.2016.3.25454
Dyer, C., Chahuneau, V., and Smith, N. A. (2013). “A simple, fast, and effective reparameterization of IBM model 2,” in Proceedings of the 2013 Conference of the North American Chapter of the Association for Computational Linguistics: Human Language Technologies (Atlanta, GA): Association for Computational Linguistics), 644–648.
Fabricius-Hansen, C., and Sæbø, K. J. (1983). Das Chamäleon “wenn” und seine Umwelt. Linguist. Berichte 83, 1–35.
Givón, T., (ed.). (2015). “Chapter 7. Serial verbs and syntactic change: Niger-congo,” in The Diachrony of Grammar (Amsterdam; Philadelphia, PA: John Benjamins), 131–162.
Good, J., and Cysouw, M. (2013). Languoid, doculect and glossonym: formalizing the notion ‘language' Lang. Document. Conserv. 7, 331–359. Available online at: http://hdl.handle.net/10125/4606
Hammarström, H., Forkel, R., Haspelmath, M., and Bank, S. (2023). Glottolog 4.8. Leipzig: Max Planck Institute for Evolutionary Anthropology. doi: 10.5281/zenodo.8131084
Hartmann, I., Haspelmath, M., and Cysouw, M. (2014). Identifying semantic role clusters and alignment types via microrole coexpression tendencies. Stud. Lang. 38, 463–484. doi: 10.1075/sl.38.3.02har
Haspelmath, M. (1995). The Converb as a Cross-Linguistically Valid Category. Berlin; Boston, MA: De Gruyter Mouton, 1–56.
Haspelmath, M. (2010). Comparative concepts and descriptive categories in crosslinguistic studies. Language 86, 663–687. doi: 10.1353/lan.2010.0021
Haspelmath, M. (2019). “How comparative concepts and descriptive linguistic categories are different,” in Aspects of Linguistic Variation, eds D. Van Olmen, T. Mortelmans, and F. Brisard (Berlin; Boston, MA: De Gruyter Mouton), 83–113.
Haspelmath, M., and König, E. (1995). Converbs in Cross-Linguistic Perspective. Structure and Meaning of Adverbial Verb Forms-Adverbial Participles, Gerunds. Berlin; New York, NY: Mouton de Gruyter.
Haug, D. T. T. (2012). “Open verb-based adjuncts in New Testament Greek and the Latin of the Vulgate,” in Big Events and Small Clauses, eds C. Fabricius-Hansen, and D. T. T. Haug (Berlin; Boston, MA: De Gruyter), 287–321.
Haug, D. T. T., and Jøhndal, M. L. (2008). “Creating a parallel treebank of the old Indo-European Bible translations,” in Proceedings of the Second Workshop on Language Technology for Cultural Heritage Data (LaTeCH 2008) (Marrakech), 27–34.
Hinrichs, E. (1986). Temporal anaphora in discourses of English. Linguist. Philos. 9, 63–82. doi: 10.1007/BF00627435
Junczys-Dowmunt, M., and Szał, A. (2012). “SyMGiza++: symmetrized word alignment models for machine translation,” in Security and Intelligent Information Systems (SIIS), volume 7053 of Lecture Notes in Computer Science, eds P. Bouvry, M. A. Klopotek, F. Leprévost, M. Marciniak, A. Mykowiecka, and H. Rybinski (Warsaw: Springer), 379–390.
Mayer, T., and Cysouw, M. (2014). “Creating a massively parallel Bible corpus,” in Proceedings of the Ninth International Conference on Language Resources and Evaluation (LREC'14) [Reykjavik: European Language Resources Association (ELRA)], 3158–3163.
Nordhoff, S., and Hammarström, H. (2011). “Glottolog/langdoc: defining dialects, languages, and language families as collections of resources,” in Proceedings of the First International Workshop on Linked Science, eds T. Kauppinen, L. C. Pouchard, and C. KeSSler, 1–7.
Nychka, D., Furrer, R., Paige, J., and Sain, S. (2021). Fields: Tools for Spatial Data. R package Version 14.1 [Dataset].
Och, F. J., and Ney, H. (2003). A systematic comparison of various statistical alignment models. Comp. Linguist. 29, 19–51. doi: 10.1162/089120103321337421
Omohundro, S. M. (1989). Five Balltree Construction Algorithms. Technical Report. Berkeley, CA: International Computer Science Institute Berkeley.
Partee, B. H. (1984). Nominal and temporal anaphora. Linguist. Philos. 7, 243–286. doi: 10.1007/BF00627707
Pedrazzini, N. (2023). A Quantitative and Typological Study of Early Slavic Participle Clauses and Their Competition (PhD thesis), University of Oxford, Oxford, United Kingdom.
Pedrazzini, N. (2022). One question, different annotation depths: a case study in Early Slavic. J. Hist. Synt. 6, 1–40. doi: 10.18148/hs/2022.v6i4-11.96
Pedregosa, F., Varoquaux, G., Gramfort, A., Michel, V., Thirion, B., Grisel, O., et al. (2011). Scikit-learn: machine learning in Python. J. Mach. Learn. Res. 12, 2825–2830.
Popjes, J., and Popjes, J. (1986). “Canela-Krahô,” in Handbook of Amazonian Languages, vol. 1, eds D. C. Derbyshire and G. K. Pullum (Berlin: Mouton de Gruyter), 128–199.
Prévot, L. (2004). Structures sémantiques et pragmatiques pour la modélisation de la cohérence dans des dialogues finalisés (PhD thesis), Toulouse: Université Paul Sabatier.
Reese, B., Hunter, J., Asher, N., Denis, P., and Baldridge, J. (2003). Reference Manual for the Analysis and Annotation of Rhetorical Structure (v 1.0). Technical Report. Austin, TX: University of Texas.
Rousseeuw, P. J. (1987). Silhouettes: a graphical aid to the interpretation and validation of cluster analysis. J. Comput. Appl. Math. 20, 53–65. doi: 10.1016/0377-0427(87)90125-7
Sæbø, K. J. (2011). “Adverbial clauses,” in Semantics. An International Handbook of Natural Language Meaning, Vol. 2, eds K. von Heusinger, C. Maienborn, and P. Portner (Berlin: Mouton de Gruyter), 1420–1441.
Sandström, G. (1993). When-Clauses and the Temporal Interpretation of Narrative Discourse (PhD thesis), Umeå: University of Umeå.
Schwarz, G. (1978). Estimating the dimension of a model. Ann. Stat. 6, 461–464. doi: 10.1214/aos/1176344136
Senft, G. (2004). “What do we really know about serial verb constructions in Austronesian and Papuan languages?” in Complex Predicates in Oceanic Languages, eds I. Bril and F. Ozanne-Rivierre (Berlin; Boston, MA: De Gruyter Mouton), 49-64. doi: 10.1515/9783110913286.49
Stirling, L. (1993). Switch-Reference and Discourse Representation. Cambridge: Cambridge University Press.
Wälchli, B. (2014). “Algorithmic typology and going from known to similar unknown categories within and across languages, in Aggregating Dialectology, Typology, and Register Analysis, eds B. Szmrecsanyi and B. Wälchli (Berlin; Boston, MA: De Gruyter), 355–393. doi: 10.1515/9783110317558.355
Keywords: temporal connectors, parallel corpora, word alignment, multidimensional scaling, semantic maps, Kriging, colexification, gram types
Citation: Haug D and Pedrazzini N (2023) The semantic map of when and its typological parallels. Front. Commun. 8:1163431. doi: 10.3389/fcomm.2023.1163431
Received: 10 February 2023; Accepted: 17 October 2023;
Published: 08 December 2023.
Edited by:
Annemarie Verkerk, Saarland University, GermanyReviewed by:
Anna Kapuścińska, Kazimierz Wielki University of Bydgoszcz, PolandLuigi Talamo, Saarland University, Germany
Copyright © 2023 Haug and Pedrazzini. This is an open-access article distributed under the terms of the Creative Commons Attribution License (CC BY). The use, distribution or reproduction in other forums is permitted, provided the original author(s) and the copyright owner(s) are credited and that the original publication in this journal is cited, in accordance with accepted academic practice. No use, distribution or reproduction is permitted which does not comply with these terms.
*Correspondence: Dag Haug, ZGFnaGF1ZyYjeDAwMDQwO3Vpby5ubw==