- 1Yale University, New Haven, CT, United States
- 2Oxford University, Oxford, United Kingdom
- 3University of Toronto, Toronto, ON, Canada
- 4Department of Computer Science, University of Toronto, Toronto, ON, Canada
- 5Department of Translation and Language Sciences, Universitat Pompeu Fabra, Barcelona, Spain
- 6Department of Computer Science, Cognitive Science Program, University of Toronto, Toronto, ON, Canada
Semantic change is attested commonly in the historical development of lexicons across the world's languages. Extensive research has sought to characterize regularity in semantic change, but existing studies have typically relied on manual approaches or the analysis of a restricted set of languages. We present a large-scale computational analysis to explore regular patterns in word meaning change shared across many languages. We focus on two levels of analysis: (1) regularity in directionality, which we explore by inferring the historical direction of semantic change between a source meaning and a target meaning; (2) regularity in source-target mapping, which we explore by inferring the target meaning given a source meaning. We work with DatSemShift, the world's largest public database of semantic change that records thousands of meaning changes from over hundreds of languages. For directionality inference, we find that concreteness explains directionality in more than 70% of the attested cases of semantic change and is the strongest predictor among the alternatives including frequency and valence. For target inference, we find that a parallelogram-style analogy model based on contextual embeddings predicts the attested source-target mappings substantially better than chance and similarity-based models. Clustering the meaning pairs of semantic change reveals regular meaning shiftings between domains, such as body parts to geological formations. Our study provides an automated approach and large-scale evidence for multifaceted regularity in semantic change across languages.
1. Introduction
Natural languages rely on a finite lexicon to express a potentially infinite range of emerging meanings. One important consequence of this finite-infinite tension is semantic change, where words often take on new meanings through time (Reisig, 1839; Bréal, 1897; Stern, 1931; Bloomfield, 1933; Ullmann, 1957; Blank, 1997). For example, in English the word face expressing “body part” at one point referred to “facial expression” later in time, and the word mouse extended its meaning from “rodent” to “computer mouse.” While historical semantic change might appear arbitrary due to random inventions and events in the world, it is believed to involve regular processes constrained by human cognition and communication (Sweetser, 1990; Geeraerts, 1997; Traugott and Dasher, 2001; Blank and Koch, 2013). Here we present a large-scale computational study to explore regular patterns of semantic change shared across languages.
Regularity in word meaning change has been a central focus in the study of historical semantics (Williams, 1976; Traugott and Dasher, 2001; Hopper and Traugott, 2003), and relatedly, discussed in the context of systematic metaphorical mapping and analogy making in human cognition (Lakoff and Johnson, 1980; Gentner, 1983; Gentner and Toupin, 1986; Sweetser, 1990). Here we consider regularity in semantic change to reflect recurring or predictable patterns in the historical shifts of word meaning, particularly as a new target meaning is derived from an existing source meaning over time. There may be dual motivations for semantic change to take place in regular ways from the perspectives of speaker and listener. From a speaker's view, regular semantic change might facilitate the grounding or structuring of new meaning given existing words (Srinivasan et al., 2019), and hence easing the process of creating and learning meaning change. From a listener's view, regular meaning change might facilitate the interpretation or construal of novel meaning, provided the speaker and listener have some shared knowledge about the world and the situation (Clark and Clark, 1979; Traugott and Dasher, 2001). Importantly, we believe that regularity may be manifested and understood in different aspects in the context of semantic change across languages.
One aspect of regularity pertains to the directionality of semantic change, namely whether there is an asymmetry in which meanings serve as the source or the target in historical semantic change of words (Winter and Srinivasan, 2022). Past studies have suggested metaphorical mapping to be a key device in structuring semantic change (Sweetser, 1990) and that people tend to extend words to abstract meanings by deriving those in relation to concrete meanings (Lakoff and Johnson, 1980; Xu et al., 2017) (e.g., we relate the abstract meaning “understand” of grasp, as in grasp an idea, to its concrete meaning “clench,” as in grasp a cup). We therefore expect an asymmetry in metaphoric semantic change such that source meanings should be generally more concrete than target meanings (Xu et al., 2017). Concreteness might not be the only factor that determines the directionality of word meaning change. For instance, recent work has shown that frequency, or how often a concept is talked about or as appears in language use, is a predictor of the directionality in meaning change alternative to concreteness (Winter and Srinivasan, 2022). However, it is not clearly understood how broadly factors such as concreteness and frequency apply to predicting the directionality of semantic change in its diverse forms which involve metaphor but also other processes such as metonymy.
Another aspect of regularity pertains to the mapping between source meaning and target meaning in semantic change, or how new meanings are structured in relation to existing meanings of a word. In particular, extensive work on polysemy has suggested that there are systematic patterns in how different senses of a word relate to each other [e.g., words originally denoting “animal” may be used to express “meat (of that animal),” as in English words fish, chicken], resulting in regular or logical polysemy (Copestake and Briscoe, 1995; Pustejovsky, 1998; Xu et al., 2020; Khishigsuren et al., 2022). Recent work has also shown that regular polysemy patterns hold crosslinguistically as they are examined in a synchronic, cross-sectional setting (Srinivasan and Rabagliati, 2015). However, it has not been comprehensively investigated whether there is shared regularity in source-target mapping of diachronic semantic change across languages. It is also an open question whether and how new mappings between a source meaning and a target meaning can be automatically inferred in semantic change. Characterizing these fine-grained, regular meaning mappings in semantic change can help inform the generative processes that give rise to novel instances of semantic change.
Our emphasis on exploring crosslinguistically shared regularity in semantic change is related to both theoretical and computational diachronic studies of word meaning. For instance, recent work has explored regularity (Bowern, 2008) and taken a functional approach in the study of grammaticalization (Hopper and Traugott, 2003). Other work has explored typological patterns in the lexicon (Kouteva et al., 2019; Thanasis et al., 2021) and taken a usage-based approach to account for the processes involved in language change (Bybee, 2015). Also related to our study is work in computational linguistics and natural language processing that has developed scalable methods for quantifying and modeling semantic change in historical text corpora (Gulordava and Baroni, 2011; Dubossarsky et al., 2016; Frermann and Lapata, 2016; Rosenfeld and Erk, 2018; Hu et al., 2019; Giulianelli et al., 2020; Schlechtweg et al., 2020), detecting semantic change (Cook and Stevenson, 2010; Sagi et al., 2011; Schlechtweg et al., 2021), and characterizing laws and regularity of semantic change (Dubossarsky et al., 2015, 2017; Xu and Kemp, 2015; Hamilton et al., 2016b; Luo et al., 2019; Xu et al., 2021) although typically within a restricted set of languages.
Extending these previous studies, we analyze a large database of historical semantic shifts recorded by linguists that include thousands of meaning change in the form of source-target meaning pairs. We develop automatic methods to learn regular patterns of semantic change that are relevant to a diverse range of languages, and our investigation helps broaden the scope of state-of-the-art analysis in this area and creates a new set of computational tools for understanding the typology of semantic change in the world's languages. To characterize regularity of semantic change in a multifaceted way, we consider two levels of analysis to explore the two aspects of regularity that we described (see Figures 1A, B for illustration).
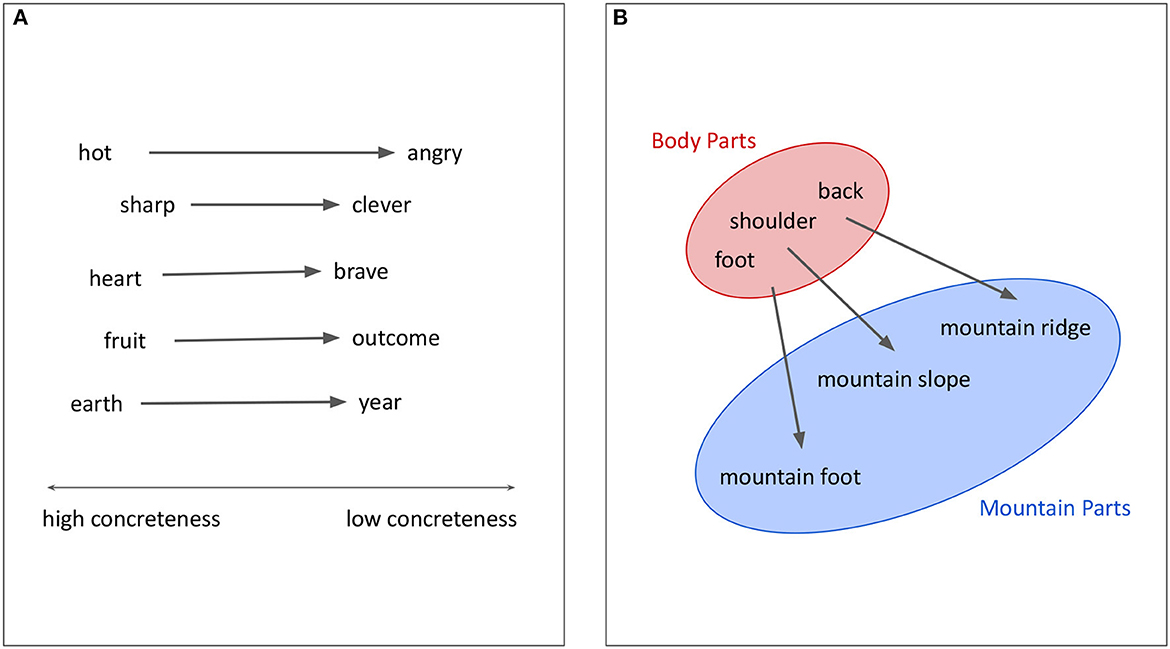
Figure 1. Illustration of the two aspects of regularity analyzed in semantic change across languages. Meanings or senses of a word are annotated in English words or phrases, and arrows indicate the direction of semantic change from a source meaning toward a target meaning. (A) Regularity in direction. (B) Regularity in source-target mapping.
We first explore shared regularity in the directionality of semantic change. We formulate this initial analysis in terms of the following inference problem: given a pair of meanings attested in historical semantic change, a source (i.e., original) meaning and a target (i.e., later acquired) meaning, can one infer the true direction of change (see Figure 1A)? We hypothesize that if semantic change exhibits a strong asymmetry in which meanings serve as source or target, there should be a high degree of regularity in the direction in which one meaning shifts toward another.
We also explore shared regularity in the source-target mapping of semantic change. We formulate this second analysis in terms of the following inference problem: given a source meaning, can one infer plausible target meanings that the source will shift toward (see Figure 1B)? We hypothesize that if semantic change draws on regular mechanisms for source-target mapping, there should be high predictability in possible (future) target meanings given a source meaning.
We formulate both of these two problems computationally, and we evaluate our computational models against Database of Semantic Shifts (Zalizniak et al., 2020), the world's largest crosslinguistic database of semantic change. To preview our results, we find strong evidence for shared regularity both in the directionality and source-target mapping of semantic change. We discuss the implications and limitations of our work, and how it synergizes with open issues and challenges for future research on the scientific study of semantic change across languages.
2. Materials and methods
We formulate our analyzes of shared regularity in semantic change as two inference problems: predicting directionality and source-target mapping. We first describe the formulation of these problems and the computational models that we develop to make inferences about regular patterns in semantic change. We then describe the crosslinguistic dataset that we use for model evaluation.
2.1. Inference of directionality of semantic change
The first problem we consider is to infer the directionality of historical semantic change between a pair of meanings. Here we adopt a discrete notion of meaning (concordant with the crosslinguistic database of semantic change we analyze) and refer to a meaning equivalently as a sense signified by a word.
We denote semantic shift from sense si to sense sj as si → sj, when a word in some language had meaning si at some point in time but not meaning sj, and then evolved to take on meaning sj at a later point in time. This process could result in meaning extension whereby a word ends up coexpressing both si and sj, or meaning replacement whereby sj takes over the original meaning si. Our analysis does not focus on this distinction and treats these cases equally as instances of semantic shift or meaning change.
Let P(si → sj) be the probability that semantic change occurs from si to sj. We can formulate this probability via the Bayes' rule as follows:
Here P(si) is the prior probability that si is a source sense, and F(sj|si) is the likelihood function that captures the semantic relatedness between the two senses si and sj, which we assume to be symmetric such that S(sj, si) = S(si, sj). Using the same logic, P(sj → si) representing probability in the inverse direction can be formulated as follows:
To infer directionality, we mask the attested historical direction of change between a pair of senses and predict the more likely direction by calculating the probability ratio of the two possible scenarios si → sj and sj → si:
If r is >1, then there is a higher probability that semantic shift was from si to sj, and vice versa. Combining Equations (1) and (2), the probability ratio is equivalent to the ratio of prior probabilities of the two senses being source of semantic change:
As an example, suppose that there exists a semantic shift between the senses “rodent” and “computer mouse” and the model is to predict which shifting direction is most likely, we would compare the probabilities P(rodent → computer mouse) and P(computer mouse → rodent), as follows:
Therefore, the problem simplifies to which of the senses “rodent” or “computer mouse” is more likely to be a source sense in semantic shift. To operationalize this source probability, we consider a set of hypotheses regarding the properties of source sense inspired by existing work from the literature. Each of these hypotheses can be taken as a predictor for directionality inference.
Following work that investigated metaphorical mapping and asymmetry of semantic change in English (Xu et al., 2017; Winter and Srinivasan, 2022), we postulate that a sense is likely to serve as a source if it is more concrete, more neutral in sentiment (i.e., valence), or more frequent in language use. We summarize the specific hypotheses as follows and draw further connections with the literature:
More concrete → less concrete: Concepts that are highly concrete may serve as representations or symbols for concepts that are less concrete (Osgood et al., 1957; Ullmann, 1957).
Less valenced → more valenced: Concepts with a neutral meaning may adopt a meaning with higher emotional valence (Osgood et al., 1957; Ullmann, 1957).
More frequent → less frequent: Since concepts that are frequently mentioned are easier for speakers to access, they might adopt new meanings more easily than those that are rarely talked about (Harmon and Kapatsinski, 2017).
2.1.1. Models and their operationalization
With the hypotheses described, we develop the following simple models to predict the direction of semantic change that occurs between senses si and sj. In each case, we take the source probability ratio of a pair of senses in Equation (4) to be proportional to the ratio of their values under the predictor variables in question.
• Concreteness: If the concreteness of si is higher than sj, we predict the direction to be si → sj. Otherwise, we predict sj → si. Here source probability is proportional to how concrete a concept is.
• Frequency: If the frequency of si is higher than sj, we predict the direction to be si → sj. Otherwise, we predict sj → si. Here source probability is proportional to how frequent a concept is.
• Valence: If the valence of si is closer to neutral than sj, we predict the direction to be si → sj. Otherwise, we predict sj → si. Here source probability is proportional to how neutral a concept is.
• Combined model: We also consider a logistic regression model that combines concreteness, frequency, and valence to predict direction. This allows us to examine whether the three predictors contain complementary information beyond the individual predictors alone.
Similar to work on directionality inference for semantic change in English (Xu et al., 2017; Winter and Srinivasan, 2022), we obtained concreteness and valence ratings of concepts from public datasets based on large-scale psycholinguistic experiments (Brysbaert et al., 2014; Mohammad, 2018), and frequency information from spoken English in the Corpus of Contemporary American English (Davies, 2010), similarly to that used in the work by Winter and Srinivasan (2022). Following work in this tradition, we also made two assumptions: (1) since we work with discrete senses, we take a sense to be the English gloss that describes its underlying conceptual meaning, and we measure the concreteness, valence, and frequency of that concept based on the corresponding English word(s); we therefore assume that these English words would be sufficiently representative of meanings, which we use to make predictions across different languages; (2) since it is infeasible to obtain people's judgment of concreteness, valence, or frequency of concepts in a historical setting, we approximate these variables with their contemporary values; we therefore assume that, for instance, the concreteness of a concept is stable over time. We acknowledge that these assumptions are limiting and may not always be warranted, but we adopt them to facilitate practically feasible and scalable analysis of semantic change in a crosslinguistic setting.
If semantic change across languages exhibits shared regularity in directionality, we expect the predictor variables we described to infer or recapitulate the historically attested directions of semantic change across languages substantially better than chance. We are also interested in understanding whether some predictor would dominate in directionality inference over other predictors.
2.2. Inference of source-target mapping of semantic change
The second problem we consider goes beyond the properties of source senses and explores shared regularity in the historical mappings between source meaning and target meaning. Here we ask whether given a source meaning si, one can automatically infer the target meaning sj as attested in the historical change si → sj.
We develop two main models for this target inference problem, described as follows.
• Similarity: We consider a similarity model that chooses the target meaning which bears the smallest semantic distance from the source meaning. This model predicts that source-target mapping in semantic change is purely based on the semantic similarity between source meaning and emerging meaning. We quantify semantic distance as Euclidean distance measured in high-dimensional semantic space to be described later.
• Analogy: We also consider an analogy model, where we model recurring types of semantic change as parallelograms in a high-dimensional semantic space resembling analogical inference in word embeddings (Mikolov et al., 2013a,b). Different from the similarity model where semantic distance is measured between a single source and a single target, here we measure semantic distance of a pair of source and target meanings and compare that to those of the attested pairs of source-target in semantic change. The idea is that the model would be able to detect new shifts that are analogous to the recurring or regular shifts already observed (e.g., over the past). For instance, if “Mercury” shifting to “Wednesday” has been already observed, the analogy model might infer that “Jupiter” is likely to shift to “Thursday,” and therefore the vector differences “Mercury” → “Wednesday” and “Jupiter” → “Thursday” are parallel and hence form a parallelogram-like structure in high-dimensional space.
2.2.1. Evaluation schemes for target meaning prediction
In principle, we can tackle the target inference problem described by calculating the probability of all possible meanings as candidate target sj for a given source meaning si. In practice, the possible set of meanings can be very large, so we operationalize this inference task by using models to choose an appropriate target meaning from a small yet controlled pool of alternative meanings for a given source meaning.
Consider an attested semantic shift si → sj, where sj is the ground-truth target meaning that we want to predict. We take k alternative senses A1, ⋯ , Ak independently from the distribution of source-target pairs d(si, sj) in a large database of semantic change. As an example, suppose the number of alternative targets is set to k = 4 and d(si, sj) is the uniform distribution over all senses in a database. We construct test cases by drawing an attested shift si → sj at random from the set of all available shifts, and alternatives A1, …, A4 at random from the set of all senses. Suppose the ground-truth shift we want to predict target for si → sj is from “rodent” to “computer mouse”, k = 4, and the alternative senses A1, …, Ak are “blue”, “heavy”, “strange feeling”, and “drum.” Our model is successful on this test case if it predicts that “computer mouse” has the highest probability of being the target sense compared to the alternatives. In this case, the model needs to make a choice out of five alternatives, one of which is the ground-truth target.
We consider two schemes for constructing the alternative pool of target candidates for evaluating our models: (1) d(si, sj) is uniformly sampled, where a random set of alternative target candidates is chosen from the database of si, sj pairs; (2) a semi-random set of alternative target candidates which bear similarity to si within some threshold. We describe these two different schemes of constructing alternative target candidates as follows.
Random selection. Under this scheme, we select target candidates randomly from the test set which is a random subset of the entire dataset, distinct from the training set that we use for model construction. We select from the test database instead of the entire database in case any of our models would give an advantage to senses that were already attested as a target in the train set.
Similarity-adjusted selection. Under this scheme, we select alternative targets from the test set that are as close as possible in similarity to the source as the actual target. In other words, we control for semantic similarity in the pool of candidate targets. For example, consider the source “Jupiter” and the target “Thursday,” which have some distance in semantic space. We would select candidate targets such as “latitude,” “molar tooth,” “place,” and “blood,” which are all the same distance from “Jupiter” in semantic space as “Thursday,” respectively. These potential targets might not be similar to the ground-truth target, but it is important that they are all near-equally similar to the source.
This similarity-based scheme for constructing the alternative target pool would help us better differentiate whether source-target mapping relies on merely similarity between source and a possible target, or something beyond such as analogical inference based on regular “types” of semantic change. If source-target mappings exhibit regularity, we expect the analogy model to better predict target meaning in comparison to the similarity model and chance.
2.3. Treatment of crosslinguistic data of semantic change
To evaluate our models, we work with Database of Semantic Shifts, abbreviated as DatSemShift (Zalizniak et al., 2020), which is a catalog of over 20,000 realizations (or cases identified by linguists) of semantic shifts across 1,179 languages. Each entry in the database is a realization of a specific attested semantic shift, and it contains information about the source and target senses annotated in English, the direction of the shift, the source and target languages and lexemes (which may differ in the case of language or morphological evolution), the status of the shift (whether it has been accepted by the database or not) and the type of shift. There are five different types of shifts: synchronic polysemy (the source and target words are meanings of one polysemous word), diachronic semantic evolution (a word evolves in meaning due to the evolution of the language), morphological derivation (the target meaning is represented by a word that is a morphological derivative of a word representing the source meaning), cognates (two words have the same ancestor), and borrowing (one word was borrowed into another language and adopted a different meaning in that language).
For our purposes, we filtered the data to focus on unidirectional shifts with the same language and word for both the source and target senses, and we focused on the shifts of the types synchronic polysemy and semantic evolution. These types include cases in which there are no morphological processes involved (contra derivation) and the processes happen within the same language (contra borrowing and cognate). We do not distinguish between synchronic polysemy and semantic evolution since there is practically no difference in these types for the purpose of our analysis. We then grouped the data together so that each shift between two senses was treated as one example of semantic shift, no matter how many realizations were attested of the shift in different languages. This brought us to 2,941 pairs of senses, each of which contains a source sense and a target sense annotated in English, as well as a number of realizations and a list of languages in which the shift was attested.
Since senses are annotated as phrases with multiple words (such as “to calculate or count”), we estimated the concreteness, valence, frequency of these senses through the following process. We split the phrase by the word “or” (e.g., “to calculate,” “count”). We removed function words such as “to” from the beginning of any phrase (e.g., “calculate,” “count”), since all verbs in DatSemShift were indicated by a leading “to.” We then matched each of the resulting phrases to the desired variable, discarding the phrase if it didn't match any entry in the concreteness, valence, or frequency datasets. We finally averaged the values of concreteness and valence variables for each phrase and took the average of the logarithmic frequency values because frequencies can be power-law distributed and thus highly skewed. Here we used the averaging method for calculating all the three predictors for simplicity and consistency, although we acknowledge that there might be other alternative methods.
We performed a focused inspection of the data from three languages that the authors have expertise in: English, German, and Spanish. We evaluated 25 random data entries from each language. The quality of the sense pairs in each entry was found to be high. However, we acknowledge that these three languages are all very well documented. Accordingly, our inspection may not necessarily speak to the quality of data from languages with sparser historical records. This is a recurrent issue for large-scale cross-linguistic research since data for some languages draws from a single source, with no point of comparison being available for quality assessment. With this in mind, the way we analyze this dataset is tolerant to some degree of noise, in the sense that we focus on differences in performance on models trained on the same dataset rather than on their precise numeric loadings.
For directionality inference, we assigned values to shifts si → sj for each predictor in question by subtracting the value of si from the value of sj. We then normalized these shift values by dividing each by half of their range across all shifts. Since we discarded all shifts where either source or target did not have an available value for concreteness, frequency, or valence, we analyzed a reduced set of 859 semantic change pairs. Alternative methods of assigning values to senses that retain more data points can be found in Supplementary material, and our results hold robustly in that more exhaustive dataset.
To train the logistic regression model combining all the three predictors, we reversed each of the semantic shifts with 50% probability, and trained the models to classify whether a shift had been reversed or not. We performed a 5-fold cross-validation and averaged the accuracy to measure model performance.
For target inference, we embedded the senses in DatSemShift using contextual embeddings particularly phrase-BERT (Wang et al., 2021). This involved replacing obscure words and spellings with common ones and removing some punctuation. More details of data preprocessing are described in Supplementary material. Phrase-BERT is an adapted version of the BERT model (Devlin et al., 2019) that embeds word and phrase meanings in a shared high-dimensional space, informed by context in natural language use. We took this semantic space as a common representation for operationalizing the similarity and analogy models. Supplementary material provides details of alternative semantic representations.
We wanted to develop and evaluate our models for predicting how the meaning of a word would change based on a crosslinguistic dataset of attested semantic changes. To do so, we split our dataset randomly into a training set (80%) and a test set (20%). For each of the sources in the test set, we selected five alternative candidate targets from the list of targets in the test set, where one of the targets is the ground truth. We performed this analysis five times for a 5-fold cross-validation.
3. Results
We evaluate the proposed models that infer the directionality and source-target mapping of semantic change against DatSemShift using data in aggregate and from individual languages that contain at least 100 attested cases of semantic change as recorded in the database. We first present crosslinguistic evidence for shared regularity in directionality. We then present further evidence for regularity in source-target mapping.
3.1. Crosslinguistic evidence for regularity in directionality
Figure 2 summarizes the results from directionality inference with aggregated data across languages. Overall, we observed that concreteness alone accounts for these data with 73.6% accuracy, which substantially exceeds the chance-level accuracy (50%) and the accuracy of the two alternative predictors (9.9% better than valence and 19.4% better than frequency). Importantly, the concreteness model predicts even better than the combined model that integrates all three predictors, suggesting that it is a dominant factor in determining source of semantic change across languages. Apart from these observations, valence has the next highest accuracy, whereas frequency performs poorly having an accuracy slightly above chance. None of the predictor variables was perfect, and Table 1 shows examples of semantic change that were assigned with correct and incorrect directions by each of the variables.
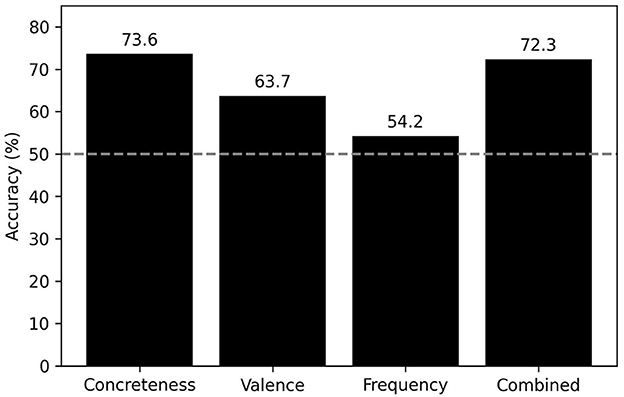
Figure 2. Predictive accuracy of concreteness, frequency, and valence in inferring directionality of semantic change. “Combined” refers to the logistic regression model that combines the three variables. Dashed line indicates chance accuracy (50%).
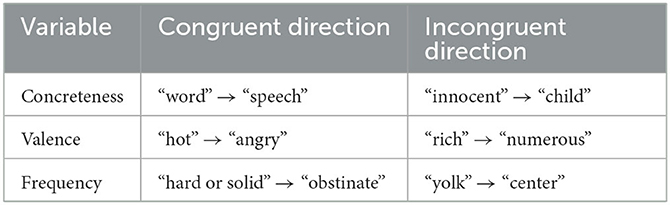
Table 1. Examples of semantic change (source meaning → target meaning) with directions congruent and incongruent with the prediction made under each variable.
To better and formally account for variability across languages, we repeated our analysis using Generalized Linear Mixed Model (GLMM) with the direction of the shift as a response variable, concreteness, valence, and frequency as predictor variables for the respective models, and language as a random effect. We used the Python package “gpboost” for the GLMM. The results of appear in Table 2. We observed that the covariant factors of random effects are small. This observation further supports our hypothesis that the trends in semantic change direction with regards to concreteness, frequency, and valence of the source and target words are shared and not language-specific.
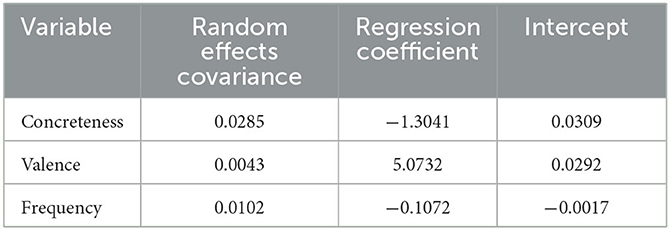
Table 2. Results of directionality inference from generalized linear mixed modeling with language as a random effect.
We also repeated our analysis across individual languages, and found the trend to persist (see Figure 3): concreteness performs the best, followed by valence. Frequency still has low accuracy around the random baseline. There are, however, some variation in the accuracy across languages. For instance, concreteness predicts directionality in the Japanese dataset almost perfectly, and it has very high accuracy for languages such as Lezgian, Tigryinya, and Mandarin Chinese. However, concreteness predicts directionality only slightly better than chance for Italian. This suggests that the languages themselves have a process of semantic change either abnormally related or abnormally unrelated to concreteness, or that the dataset might contain biases in those languages in terms of concrete to abstract semantic shifts. Nevertheless, the overall trend in accuracy by language is consistent, suggesting that the factors driving semantic change are common across languages. In fact, out of 29 languages we examined here that have more than 100 shifts in DatSemShift, 26 have directionality best predicted by concreteness, and the other three have directionality best predicted by valence.
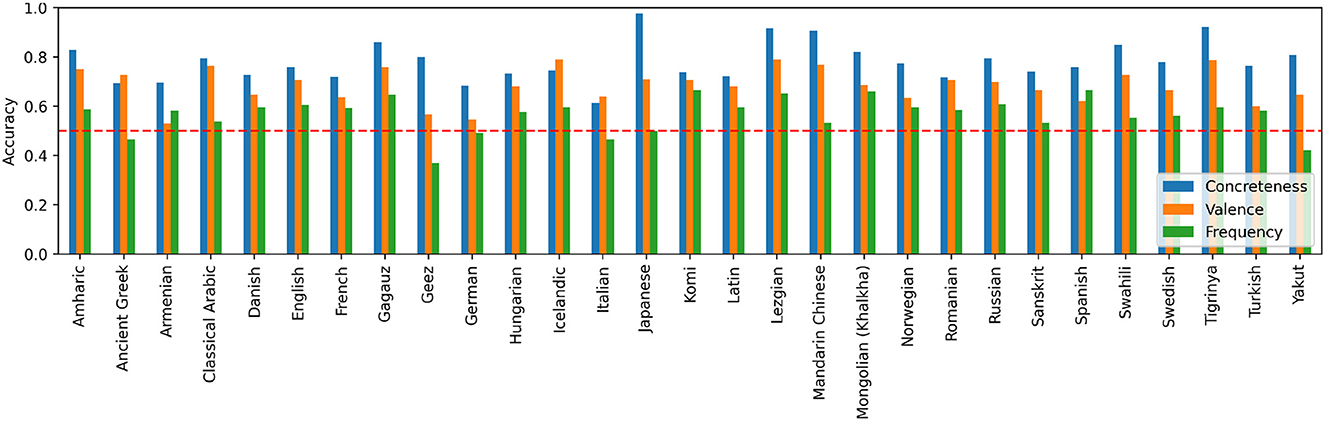
Figure 3. Predictive accuracy of concreteness, frequency, and valence in inferring directionality of semantic change for each individual language with at least 100 samples. Dashed line indicates chance accuracy (50%).
This set of results resonates with previous work on metaphoric semantic change in English suggesting that concreteness is a dominant factor in determining the directionality, or the source of change (Xu et al., 2017). However, our current analysis extends the existing finding toward regularity in semantic change in general (not just metaphoric change) and across many languages. Our findings support the view that there is broad-scale regularity in the directionality of semantic change across languages: more concrete meanings tend to serve as source of change, and historical meaning change tends to move from concrete meanings toward abstract ones.
3.2. Crosslinguistic evidence for regularity in source-target mapping
Figure 4 summarizes the results from target inference with aggregated data across languages. The gray bars indicate predictive accuracy of the similarity and analogy models evaluated respectively on random selections of alternative target candidates. In each of these test cases, a source meaning is given, and models are applied to infer its ground-truth target meaning among four alternative candidate meanings (with chance accuracy being 20%). The black bars indicate the same except on similarity-adjusted selections of alternative target candidates.
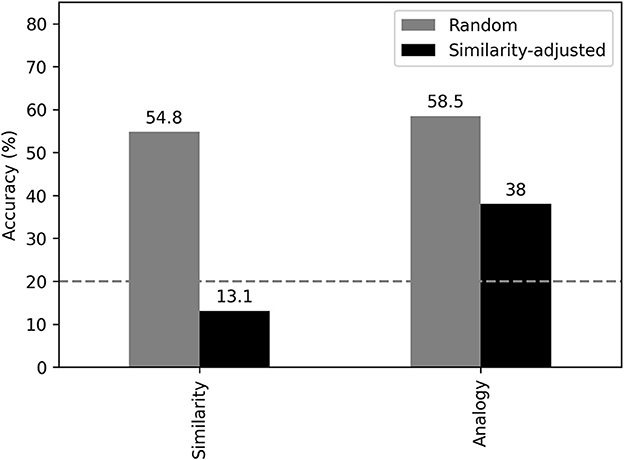
Figure 4. Predictive accuracy of the analogy model and the similarity model in inferring target meanings of semantic change. Models are evaluated based on randomly chosen and similarity-adjusted target candidates. The dashed line indicates chance accuracy (20%).
For the randomly selected targets, both similarity and analogy models performed well above the chance level. This observation indicates that the similarity between source and target is an important factor in determining the appropriateness of semantic shift between a pair of meanings. However, this finding is not that surprising, since from the similarity-model point of view, a target meaning that is most similar to the source meaning is most likely to be chosen among the random alternatives, since we know that semantic change is not arbitrary and often relies on relatedness of senses. From the analogy-model point of view, a potential semantic change between a source and a target that are very similar—that is, a shift that moves a short distance in semantic space—is going to be relatively close to other short-distance shifts in the training set (i.e., meaning pairs that the model has been exposed to as past observations). But a potential shift that moves a long distance in semantic space is more likely to be in a sparse area of the train dataset. In this respect, the analogy model would likely choose a target that is similar to the source – that is, the same target that the similarity model is choosing. Therefore, this initial set of observations shows that similarity matters in semantic change, but it does not tease apart the difference in predictive power of the similarity model and the analogy model.
For the similarity-adjusted targets, it is clear that the similarity model is incompetent in choosing the ground-truth target among alternative targets that are semantically similar to the source meaning in question, and in fact, this model performs even worse than chance. This result is due to the fact that not all cases of semantic change necessarily involve a shift to the most similar meaning possible. The similarity model, however, always favors a target that bears high similarity with the source meaning, and thus assumes the target must be maximally similar to the source among the set of alternatives (which may not be true). In contrast, the analogy model performs well above chance and almost triples the accuracy of the similarity model by correctly identifying the target 38% of the time. This result suggests that semantic change relies on not just similarity between meaning, but recurring or regular meaning shifting strategies. In our case, the analogy model identifies parallel semantic shifts in the training set to be relatable to those in the test set, and is therefore able to generalize to novel instances of semantic change beyond those observed in the training set (note that there is no overlapping or duplicated cases of semantic change between the training and test sets).
To understand how robustly these results hold across languages, we repeated target inference in all individual languages with at least 100 attested semantic shifts. We trained the model on the set of all shifts that did not occur in a particular language, and tested the model on the shifts that did occur in that language. Figure 5 summarizes these results which confirm our findings at the aggregate level: the analogy model dominates in predicting source-target mappings in each of these languages. These findings provide comprehensive evidence that there are shared regular patterns in source-target mappings in historical semantic change across languages.
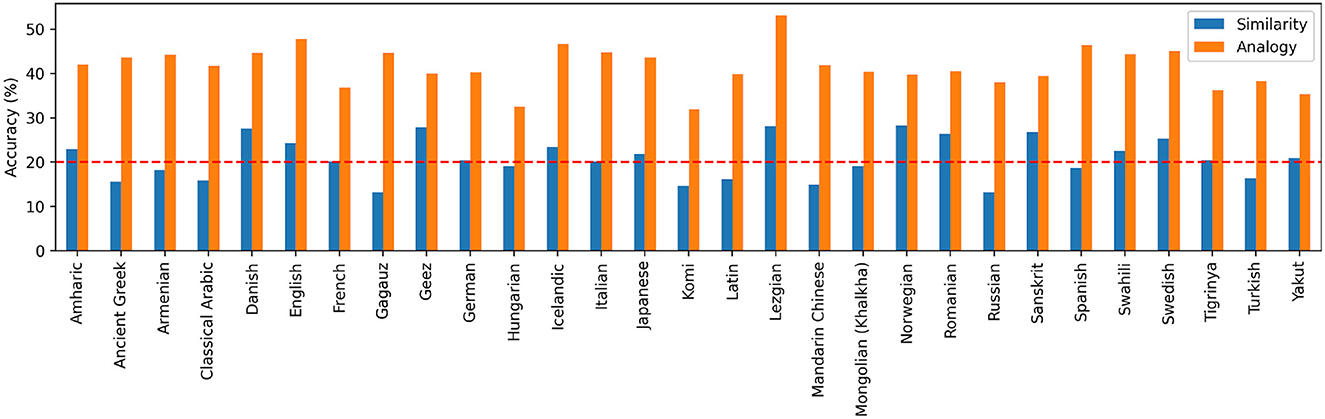
Figure 5. Predictive accuracy of the analogy model and the similarity model in inferring target meanings of semantic change for each individual language with at least 100 samples. The models are evaluated with the similarity-adjusted target candidates. Dashed line indicates chance accuracy (20%).
3.2.1. Visualization of regular mappings in semantic change
To better understand the regular patterns of source-target mapping in semantic change, we performed a qualitative interpretive analysis that visualizes the “types” of semantic change. To do so, we first embedded each attested semantic change using the vector difference between target and source embeddings via phrase-BERT. We then applied Gaussian Mixture Model (GMM) to group these shift embeddings into clusters. Since GMM is a soft clustering algorithm, it assigns a probability to each shift for being in each cluster. However, in practice, we observed that the majority of cases were assigned a high probability (>0.95) of being in one cluster and a low probability (<0.05) for the other clusters. We therefore visualized the resulting clusters as hard clusters and assigned each shift to the cluster for which it had the highest probability.
To facilitate effective visualization, we chose GMM to estimate 50 clusters to balance between interpretability and diversity. Figure 6 shows a subset of three clusters, in which we found both the set of source senses and the set of target senses to have clear interpretation. Most of these clusters are for noun senses, as shown by the green and blue clusters. The red cluster shows some variability where both its source and target senses are mainly verbs. Interestingly we observed that the shifts in this cluster are pejorative, with a clear drop in valence (e.g., from neutral to negative sentiment). Within each cluster, we observed regular patterns of meaning shift from one domain to another, such as meanings to do with body part shifting toward meanings to do with geological formation, and meanings to do with everyday actions shifting toward meanings to do with politically destructive actions.
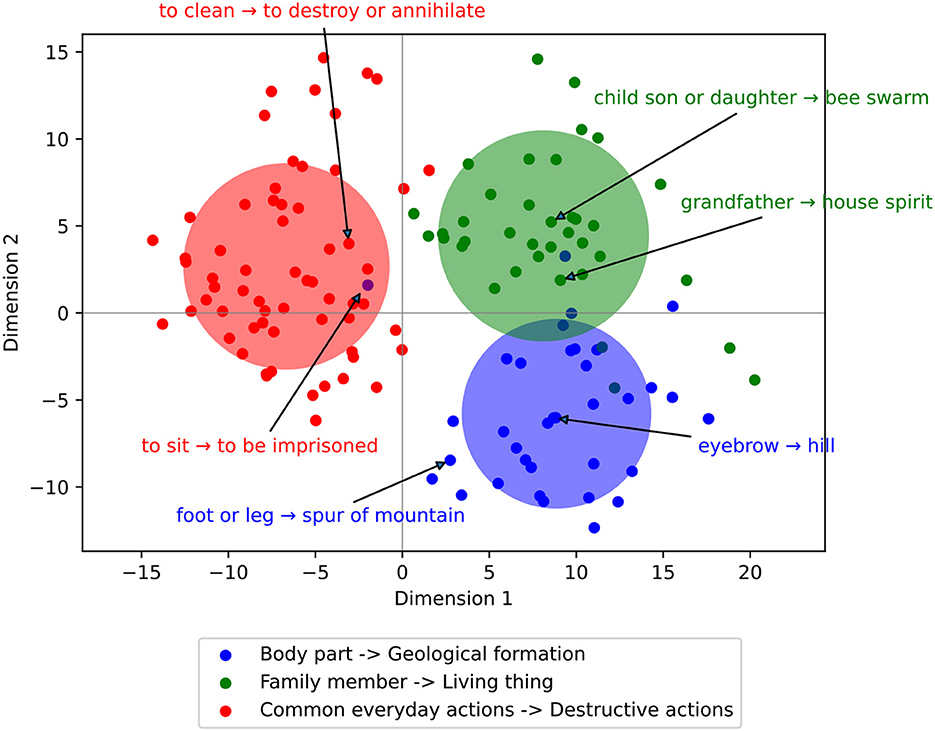
Figure 6. Example clusters of semantic change in dimension-reduced principal components space. Clusters are obtained via a Gaussian mixture model and illustrate regular domain-domain mappings, where attested samples of semantic shift (source meaning → target meaning) are annotated.
Our findings are consistent with existing crosslinguistic work on polysemy, where there are regular patterns in how word relate and express different meanings (Srinivasan and Rabagliati, 2015). However, our current analysis extends this line of research from a synchronic setting to a diachronic view and across many different languages. Our findings underscore the importance of analogical inference in the recurring patterns of historical semantic change across languages, where source-target mapping not only depends on the similarity between source and target themselves, but also on the similarity of a higher order that creates a novel pairing of source-target meanings based on its relations to the existing or attested pairings in semantic change.
4. Discussion and conclusion
We have presented a large-scale computational analysis of shared regular patterns in semantic change. Our analysis reveals that regularity in semantic change is not only multifaceted but also a shared property across many different languages.
We evaluated the roles of concreteness, valence, and frequency in predicting the direction of semantic change and identified concreteness as the most accurate predictor. This finding is consistent with earlier work from Xu et al. (2017) suggesting that a parsimonious set of variables can account for the directionality in metaphoric meaning change, but it extends the existing findings by explaining regular directions of semantic change beyond metaphoric change, and by considering semantic change in languages other than English. Our current finding holds broadly across the wide range of attested semantic shifts and crosslinguistically: in fact, we found concreteness to be the best predictor for directionality in 90% of the languages with more than 100 data points in DatSemShift. Our finding about directionality can be taken as evidence for common principles shared across languages, but it also naturally leads to questions regarding the crosslinguistic typology or variation in semantic change. One possible direction for future research is to characterize where languages differ in the patterns of semantic change, and therefore understand what might give rise to this variation. It is possible that culture-specific factors, such as history, religion, or technological innovation, and language-specific factors, such as vocabulary or syntax, might jointly contribute to semantic change (Hamilton et al., 2016a). Understanding how these factors interact and give rise to crosslinguistic variation in semantic change can be an important and fruitful avenue.
Apart from directionality, we also evaluated the degree of regularity in source-target mappings of semantic change. We found that similarity is a good predictor for inferring target sense of a semantic change, but when controlling explicitly for similarity, the analogy model that takes into account high-order similarity of source-target pairings performs much better than chance and the similarity model. Our results extend synchronic, cross-sectional findings from Srinivasan and Rabagliati (2015) suggesting that regular patterns of English polysemy exist in other languages toward a diachronic setting. Furthermore, through fine-grained target inference we also demonstrated how analogy may play a crucial role in shaping regular source-target mappings in historical semantic change across languages.
Our work has important implications to cognitive and computational approaches to characterizing semantic change. Separate lines of research ranging from cognitive science to computational linguistics have presented the converging view that word meaning often changes in incremental as opposed to abrupt ways (Frermann and Lapata, 2016; Bamler and Mandt, 2017; Ramiro et al., 2018). This incremental, gradual way of meaning change is typically captured by temporally smooth models that account for small changes in meaning space over time (Frermann and Lapata, 2016; Bamler and Mandt, 2017), or semantic chaining models that postulate new meanings to emerge by linking to existing meanings that are highly semantically similar (Xu et al., 2016; Ramiro et al., 2018; Habibi et al., 2020). Our current analysis paints a more complex picture of semantic change by suggesting that incremental or similarity-based processes alone are not sufficient to account for the diverse range of attested cases of semantic change. However, it is likely that semantic change relies on a combination of cognitive mechanisms that identify both surface similarity and structural (or analogy-based) similarity (Gentner, 1983) in meaning space. A fundamental challenge for future research is how to integrate these different kinds of processes in a coherent formal framework for generating the diverse range of semantic changes across languages.
We have restricted our current analysis of semantic change within individual words, and we acknowledge that this semasiological approach might not be fully representative of the onomasiological aspects of meaning change. In reality, meaning change results from a lexical competition process where words in the lexicon compete to express an emerging meaning (e.g., see work on chaining that formulates lexical competition as models of categorization as in Xu et al., 2016 and Habibi et al., 2020). Understanding these competing dynamics at the scale of the lexicon across languages may be challenging, since tracking the space of possible alternative lexical items can be infeasible due to its size but also the sparsity of crosslinguistic diachronic data. This is also complicated by the fact that multiple cognitive mechanisms (e.g., metaphor, metonymy) might be at work in the historical development of semantic change.
Our computational approach also differs substantially from a large body of work in natural language processing that uses historical corpora as a primary medium for investigating semantic change. It is an open issue how the regular patterns identified here with discrete word senses may be leveraged to develop novel algorithms for the automated inference and generation of semantic change in naturalistic settings.
In summary, we have offered an automated approach and a comprehensive evaluation of multifaceted regularity in semantic change across languages. We believe that our study paves the way for diversifying the scientific inquiry into semantic change beyond individual or a restricted set of languages. In doing so, we also hope that it will serve as an important stepping stone for fostering synergistic research across disciplines toward understanding the time-varying nature of the human lexicon.
Data availability statement
The original contributions presented in the study are included in the article/Supplementary material, further inquiries can be directed to the corresponding authors. Data and code repository: https://github.com/o-fugi/regularity-semantic-change.
Author contributions
OF, OH, RL, and YX conceptualized the study. OF, OH, and RL acquired and analyzed the data and drafted the initial manuscript. YX and LY provided supervision. All authors participated in the development of methodology, data interpretation, contributed to the revision, and writing of the manuscript. All authors contributed to the article and approved the submitted version.
Funding
This research was funded partly by the 2022 Fields Undergraduate Summer Research Program. YX was funded by a NSERC Alliance International-Catalyst Grant ALLRP 576149-22, a NSERC Discovery Grant RGPIN-2018-05872, a SSHRC Insight Grant #435190272, and an Ontario Early Researcher Award #ER19-15-050.
Acknowledgments
We thank the Fields Institute for financial support and facilitating the collaborative research project. We thank Gemma Boleda for discussion and feedback, Yiwei Luo and Aparna Balagopalan for sharing resources.
Conflict of interest
The authors declare that the research was conducted in the absence of any commercial or financial relationships that could be construed as a potential conflict of interest.
Publisher's note
All claims expressed in this article are solely those of the authors and do not necessarily represent those of their affiliated organizations, or those of the publisher, the editors and the reviewers. Any product that may be evaluated in this article, or claim that may be made by its manufacturer, is not guaranteed or endorsed by the publisher.
Supplementary material
The Supplementary Material for this article can be found online at: https://www.frontiersin.org/articles/10.3389/fcomm.2023.1136338/full#supplementary-material
References
Bamler, R., and Mandt, S. (2017). “Dynamic word embeddings,” in International Conference on Machine Learning (Sydney), 380–389.
Blank, A. (1997). Prinzipien des lexikalischen Bedeutungswandels am Beispiel der romanischen Sprachen. Tubingen: Niemeyer.
Bowern, C. (2008). The diachrony of complex predicates. Diachronica 25, 161–185. doi: 10.1075/dia.25.2.03bow
Brysbaert, M., Warriner, A. B., and Kuperman, V. (2014). Concreteness ratings for 40 thousand generally known english word lemmas. Behav. Res. 46, 904–911. doi: 10.3758/s13428-013-0403-5
Clark, E. V., and Clark, H. H. (1979). When nouns surface as verbs. Language 55, 767–811. doi: 10.2307/412745
Cook, P., and Stevenson, S. (2010). “Automatically identifying changes in the semantic orientation of words,” in Proceedings of the Seventh International Conference on Language Resources and Evaluation (LREC'10) (Malta).
Copestake, A., and Briscoe, T. (1995). Semi-productive polysemy and sense extension. J. Semant. 12, 15–67. doi: 10.1093/jos/12.1.15
Davies, M. (2010). The corpus of contemporary american english as the first reliable monitor corpus of english. Literary Linguist. Comput. 25, 447–464. doi: 10.1093/llc/fqq018
Devlin, J., Chang, M.-W., Lee, K., and Toutanova, K. (2019). “BERT: pre-training of deep bidirectional transformers for language understanding,” in Proceedings of the 2019 Conference of the North American Chapter of the Association for Computational Linguistics: Human Language Technologies, Volume 1 (Long and Short Papers) (Minneapolis, MN. Association for Computational Linguistics), 4171–4186.
Dubossarsky, H., Tsvetkov, Y., Dyer, C., and Grossman, E. (2015). “A bottom up approach to category mapping and meaning change,” in The European Network on Word Structure (Pisa).
Dubossarsky, H., Weinshall, D., and Grossman, E. (2016). Verbs change more than nouns: a bottom-up computational approach to semantic change. Lingue e Linguaggio 15, 7–28. doi: 10.1418/83652
Dubossarsky, H., Weinshall, D., and Grossman, E. (2017). “Outta control: laws of semantic change and inherent biases in word representation models,” in Proceedings of the 2017 Conference on Empirical Methods in Natural Language Processing, 1136–1145.
Frermann, L., and Lapata, M. (2016). A bayesian model of diachronic meaning change. Trans. Assoc. Comput. Linguist. 4, 31–45. doi: 10.1162/tacl_a_00081
Geeraerts, D. (1997). Diachronic Prototype Semantics: A Contribution to Historical Lexicology. Oxford: Oxford University Press.
Gentner, D. (1983). Structure-mapping: a theoretical framework for analogy. Cogn. Sci. 7, 155–170. doi: 10.1207/s15516709cog0702_3
Gentner, D., and Toupin, C. (1986). Systematicity and surface similarity in the development of analogy. Cogn. Sci. 10, 277–300. doi: 10.1207/s15516709cog1003_2
Giulianelli, M., Del Tredici, M., and Fernández, R. (2020). “Analysing lexical semantic change with contextualised word representations,” in Proceedings of the 58th Annual Meeting of the Association for Computational Linguistics. p. 3960–3973.
Gulordava, K., and Baroni, M. (2011). “A distributional similarity approach to the detection of semantic change in the google books ngram corpus,” in Proceedings of the GEMS 2011 Workshop on GEometrical Models of Natural Language Semantics, GEMS '11 (USA: Association for Computational Linguistics), 67–71.
Habibi, A. A., Kemp, C., and Xu, Y. (2020). Chaining and the growth of linguistic categories. Cognition 202, 104323. doi: 10.1016/j.cognition.2020.104323
Hamilton, W. L., Leskovec, J., and Jurafsky, D. (2016a). “Cultural shift or linguistic drift? comparing two computational measures of semantic change,” in Proceedings of the Conference on Empirical Methods in Natural Language Processing. Conference on Empirical Methods in Natural Language Processing, volume 2016 (Austin: NIH Public Access).
Hamilton, W. L., Leskovec, J., and Jurafsky, D. (2016b). “Diachronic word embeddings reveal statistical laws of semantic change,” in Proceedings of the 54th Annual Meeting of the Association for Computational Linguistics (Volume 1: Long Papers) (Berlin), 1489–1501.
Harmon, Z., and Kapatsinski, V. (2017). Putting old tools to novel uses: the role of form accessibility in semantic extension. Cogn. Psychol. 98, 22–44. doi: 10.1016/j.cogpsych.2017.08.002
Hopper, P. J., and Traugott, E. C. (2003). Grammaticalization. Cambridge: Cambridge University Press.
Hu, R., Li, S., and Liang, S. (2019). “Diachronic sense modeling with deep contextualized word embeddings: an ecological view,” in Proceedings of the 57th Annual Meeting of the Association for Computational Linguistics, 3899–3908.
Khishigsuren, T., Bella, G., Brochhagen, T., Marav, D., Giunchiglia, F., and Batsuren, K. (2022). Metonymy as a universal cognitive phenomenon: evidence from multilingual lexicons. PsyArXiv. doi: 10.31234/osf.io/f6yjt
Kouteva, T., Heine, B., Hong, B., Long, H., Narrog, H., and Rhee, S. (2019). World Lexicon of Grammaticalization. Cambridge: Cambridge University Press.
Luo, Y., Jurafsky, D., and Levin, B. (2019). “From insanely jealous to insanely delicious: computational models for the semantic bleaching of english intensifiers,” in Proceedings of the 1st International Workshop on Computational Approaches to Historical Language Change (Florence), 1–13.
Mikolov, T., Chen, K., Corrado, G., and Dean, J. (2013a). Efficient estimation of word representations in vector space. CoRR, abs/1301.3781. doi: 10.48550/arXiv.1301.3781
Mikolov, T., Sutskever, I., Chen, K., Corrado, G. S., and Dean, J. (2013b). “Distributed representations of words and phrases and their compositionality.,” in Advances in Neural Information Processing Systems, Vol. 26 (Lake Tahoe).
Mohammad, S. (2018). “Obtaining reliable human ratings of valence, arousal, and dominance for 20,000 English words,” in Proceedings of the 56th Annual Meeting of the Association for Computational Linguistics (Volume 1: Long Papers) (Melbourne, VIC: Association for Computational Linguistics), 174–184.
Osgood, C. E., Suci, G. J., and Tannenbaum, P. H. (1957). The Measurement of Meaning. Champaign, IL: University of Illinois Press.
Ramiro, C., Srinivasan, M., Malt, B. C., and Xu, Y. (2018). Algorithms in the historical emergence of word senses. Proc. Natl. Acad. Sci. U.S.A. 115, 2323–2328. doi: 10.1073/pnas.1714730115
Rosenfeld, A., and Erk, K. (2018). Deep “Neural models of semantic shift,” in Proceedings of the 2018 Conference of the North American Chapter of the Association for Computational Linguistics: Human Language Technologies, Volume 1 (Long Papers) (New Orleans, LA: Association for Computational Linguistics), 474–484.
Sagi, E., Kaufmann, S., and Clark, B. (2011). Tracing semantic change with latent semantic analysis. Curr. Methods Histor. Semant. 73, 161–183. doi: 10.1515/9783110252903.161
Schlechtweg, D., McGillivray, B., Hengchen, S., Dubossarsky, H., and Tahmasebi, N. (2020). “Semeval-2020 task 1: unsupervised lexical semantic change detection,” in Proceedings of the Fourteenth Workshop on Semantic Evaluation, 1–23.
Schlechtweg, D., Tahmasebi, N., Hengchen, S., Dubossarsky, H., and McGillivray, B. (2021). Dwug: a large resource of diachronic word usage graphs in four languages. EMNLP (Punta Cana). 2021, 567. doi: 10.18653/v1/2021.emnlp-main.567
Srinivasan, M., Berner, C., and Rabagliati, H. (2019). Children use polysemy to structure new word meanings. J. Exp. Psychol. Gen. 148, 926–942. doi: 10.1037/xge0000454
Srinivasan, M., and Rabagliati, H. (2015). How concepts and conventions structure the lexicon: cross-linguistic evidence from polysemy. Lingua 157, 124–152. doi: 10.1016/j.lingua.2014.12.004
Stern, G. (1931). Meaning and Change of Meaning, with Special Reference to the English Language. Gothenberg: Elanders Boktryckeri Aktiebolag.
Sweetser, E. (1990). From Etymology to Pragmatics: Metaphorical and Cultural Aspects of Semantic Structure. Cambridge: Cambridge University Press.
Thanasis, G., Grossman, E., Nikolaev, D., and Polis, S. (2021). Universal and macro-areal patterns in the lexicon: a case-study in the perception-cognition domain. Linguist. Typol. 26, 439–487. doi: 10.1515/lingty-2021-2088
Traugott, E. C., and Dasher, R. B. (2001). Regularity in Semantic Change. Cambridge: Cambridge University Press.
Wang, S., Thompson, L., and Iyyer, M. (2021). Phrase-bert: Improved phrase embeddings from BERT with an application to corpus exploration. CoRR, abs/2109.06304. doi: 10.18653/v1/2021.emnlp-main.846
Williams, J. M. (1976). Synaesthetic adjectives: A possible law of semantic change. Language 52, 461–478. doi: 10.2307/412571
Winter, B., and Srinivasan, M. (2022). Why is semantic change asymmetric? the role of concreteness and word frequency and metaphor and metonymy. Metaphor Symbol 37, 39–54. doi: 10.1080/10926488.2021.1945419
Xu, A., Stellar, J. E., and Xu, Y. (2021). Evolution of emotion semantics. Cognition 217, 104875. doi: 10.1016/j.cognition.2021.104875
Xu, Y., Duong, K., Malt, B. C., Jiang, S., and Srinivasan, M. (2020). Conceptual relations predict colexification across languages. Cognition 201, 104280. doi: 10.1016/j.cognition.2020.104280
Xu, Y., and Kemp, C. (2015). “A computational evaluation of two laws of semantic change,” in Proceedings of the 37th Annual Meeting of the Cognitive Science Society, CogSci 2015, eds D. C. Noelle, R. Dale, A. S. Warlaumont, J. Yoshimi, T. Matlock, C. D. Jennings and P. P. Maglio (Pasadena, CA: cognitivesciencesociety.org).
Xu, Y., Malt, B., and Srinivasan, M. (2017). Evolution of word meanings through metaphorical mapping: systematicity over the past millennium. Cogn. Psychol. 96, 41–53. doi: 10.1016/j.cogpsych.2017.05.005
Xu, Y., Regier, T., and Malt, B. C. (2016). Historical semantic chaining and efficient communication: the case of container names. Cogn. Sci. 40, 2081–2094. doi: 10.1111/cogs.12312
Keywords: word meaning, historical semantics, semantic change, crosslinguistic regularity, computational analysis
Citation: Fugikawa O, Hayman O, Liu R, Yu L, Brochhagen T and Xu Y (2023) A computational analysis of crosslinguistic regularity in semantic change. Front. Commun. 8:1136338. doi: 10.3389/fcomm.2023.1136338
Received: 02 January 2023; Accepted: 16 March 2023;
Published: 04 April 2023.
Edited by:
Gerd Carling, Lund University, SwedenReviewed by:
Mario Giulianelli, University of Amsterdam, NetherlandsEitan Grossman, Hebrew University of Jerusalem, Israel
Copyright © 2023 Fugikawa, Hayman, Liu, Yu, Brochhagen and Xu. This is an open-access article distributed under the terms of the Creative Commons Attribution License (CC BY). The use, distribution or reproduction in other forums is permitted, provided the original author(s) and the copyright owner(s) are credited and that the original publication in this journal is cited, in accordance with accepted academic practice. No use, distribution or reproduction is permitted which does not comply with these terms.
*Correspondence: Olivia Fugikawa, by5mdWdpa2F3YUBnbWFpbC5jb20=; Oliver Hayman, b2xpdmVyLmhheW1hbkBrZWJsZS5veC5hYy51aw==; Raymond Liu, cmF5bW9uZG0ubGl1QG1haWwudXRvcm9udG8uY2E=; Yang Xu, eWFuZ3h1QGNzLnRvcm9udG8uZWR1
†These authors have contributed equally to this work