- 1Department of Design and Communication, University of Southern Denmark, Kolding, Denmark
- 2Department of Mechanical and Electrical Engineering, Centre for Industrial Electronics, University of Southern Denmark, Sonderborg, Denmark
- 3Department of Business Development and Technology, Aarhus University, Herning, Denmark
This study examines whether a social robot can enable team creativity and increase team performance depending on its speaking style. The aim is to provide insight into human teams' creativity and performance when exposed to different ways of speaking by a social robot, that is, when the robotic creativity facilitator is using different acoustic-prosodic features. In one condition, participants received their instructions from the robot in a “charismatic” speaking style, in the other, the robot used a less engaging way of speaking. The results show that when the robot's speech is based on charismatic speech characteristics, it is significantly better at enhancing team creativity and performance than when its speech uses fewer charismatic speech characteristics. The robot's speaking style thus influences its effectiveness as team creativity facilitator.
1. Introduction
Social robots are increasingly used in contexts in which they inform or instruct children and adults, for example, as museum guides (Shin et al., 2016), tutors (Han et al., 2008), or collaborators (Barrett et al., 2012). There is a growing interest in how robots can shape the social dynamics of teams, often with the goal to improve collaborative outcomes (De Visser et al., 2020). Teams are the most common form of collaboration and play a crucial role in enhancing organizational innovation and performance (Salas et al., 2008; Fay et al., 2015; McDowell et al., 2016). Research on teams has received extensive attention from, inter alia, organizational, psychology, sociology, communication and information science scholars. Across these disciplines, teams are typically defined as consisting of two or more individuals, having a common goal, performing tasks that interdependent in terms of workflow, goals and outcomes, and being embedded in an organizational context (Alderfer, 1977; Hackman, 1992; Salas et al., 1992; Kozlowski and Bell, 2003). Another important characteristic of teams is that they interact socially, face-to-face or virtually, and can be collocated, geographically dispersed or hybrid (Hinds and Bailey, 2003; Cousins et al., 2007).
Compared with individuals, teams are better at facilitating and improving the creative process (Leavitt, 1974), (Puranam, 2018). As teams are so central to creativity and collaboration, research has started to inquire into how machines, such as virtually and physically embodied robots, may affect teamwork (Sebo et al., 2020), (Yan et al., 2020). We define creativity in terms of outcomes, namely as “the production of novel and useful ideas in any domain” (Amabile et al., 1996, p. 1155) or more simply put as “novel, potentially useful ideas” (Seibt et al., 2020, p. 934). Many organizations are dependent on developments driven by creative ideas, designs, solutions, products, and services (Kozlowski and Bell, 2003). In these contexts, robots should enhance, rather than hinder, human performance.
While research on individual creativity has been investigated extensively, research on team creativity lags behind (Gilson et al., 2015). This is, to a certain extent, attributed to the fact that investigating creativity at the team level is more complex as team creativity is not warranted even in the presence of enabling conditions as other factors might be at play (Gilson et al., 2019). Nonetheless, team processes such as communication and coordination are essential for team performance, including team creativity (Leavitt, 1974; LePine et al., 2008). Indeed, the way team members communicate with each other may motivate them to share knowledge with the team and help them develop positive team relationships (Camden and Kennedy, 1986; Inglis, 1993; Hinds and Pfeffer, 2003). This study thus examines whether such effects of communication extend to team creativity, or, more specifically, whether and how speaking styles of a robotic facilitator affect team creativity.
While human-robot interaction research has demonstrated that robots may be successful as creativity facilitators (e.g., Kahn et al., 2016), a robot design choice that has hitherto received little attention is its speech characteristics. Verbal communication is one of the most natural ways for humans to communicate (Crumpton and Bethel, 2015). Similarly, it plays an important role in many human-robot interaction scenarios and has been shown to have a substantial effect on the persuasiveness and impressionistic evaluation of robots as well (Fischer et al., 2020). Despite this important role, robot designers have paid relatively little attention to the voices used on robots (McGinn and Torre, 2019), and specifically not in relation to teamwork and creativity.
There is however reason to assume that charismatic speech may have pragmatic and cognitive effects that lead to improved team creativity; in particular, there is empirical evidence that charismatic speech increases the speaker's perceived competence, self-confidence, and passion. For instance, Niebuhr and Michalsky (2019) found that speaking rate and silent pause duration are mostly related to perceived speaker competence, while pitch range and emphatic-accent frequency are related to passion and a higher level of arousal, which in turn can lead to heightened attention and hence more engagement (cf. Niebuhr, 2021). These features could thus also prove beneficial for increasing team creativity. Moreover, charismatic leadership is known to have many positive effects on their listeners, and charismatic leadership has been found to promote task performance (Holladay and Coombs, 1994; Cho and Cho, 2014) and team creativity (Murphy and Ensher, 2008; Sørensen, 2013).
In this study, we therefore examine how a robot's speech characteristics can influence the creativity of human teams in order to inform social robot design choices in the future. This leads us to the research question which guides the present study: How does a social robot's charismatic vs. non-charismatic speaking style influence team creativity and performance?
2. Previous work
Previous work concerns studies addressing how robots can enhance team creativity, and work on the effect of charismatic speaking styles.
2.1. Robots as creativity facilitators
Many studies have shown that robots can function as creativity facilitators; for instance, Kahn et al. (2016) show that a robot leads to more creative results than a self-paced presentation, for instance. Alderfer (1977), Ali et al. (2019) show that social robots are principally suited to facilitate creativity in children. Similarly, Alves-Oliveira et al. (2020) had a robot engage children in joint storytelling activities and found that verbal creativity levels increased, that children produced more objective ideas, and that they elaborated on their ideas more. Concerning adults, Geerts et al. (2021) found no difference between human and robot creativity facilitators on participants' productivity in a brainstorming task. Thus, previous work shows that robots are generally suited to engage people in creativity tasks, and that they perform better than other technologies and not worse than human facilitators.
Concerning the determining factors of creativity facilitation, Elgarf et al. (2021) found that the degree to which a robot is creative does not influence the extent to which children are creative during a joint storytelling task. Hu et al. (Howell, 2010) address factors that may impact the effects of robot behaviors on people's creativity. In their study with adults, they found both internal (e.g., personality, knowledge and motivation) and external factors (e.g., cultural differences) to influence the extent to which a robot can enhance creativity. The role of speech characteristics of the robot facilitator have not yet been addressed, and the studies have so far concentrated on investigating creativity at the individual level, not on team creativity.
In contrast, much previous work investigates whether robots can influence the performance of teams. For instance, Jung et al. (2015) explore whether robot interventions can positively influence conflict dynamics by addressing interpersonal violations that occur during a problem-solving task. They found that the robot's interventions increased the groups' awareness of conflict, thus acting against the tendency to suppress the conflict. These findings suggest that robots can help a team by the management of occurrences such as conflict. In a recent study by Rosenberg-Kima et al. (2020), a social robot facilitated a small collaborative group activity of students in higher education to examine the effects of facilitation on attitudes toward the activity facilitation, the group activity, and the robot. Students perceived the robot positively, as friendly and responsive, even though the robot did not directly respond to the students' verbal communications. Furthermore, the robot was perceived to have advantages over a human facilitator, such as better time management, objectivity, and efficiency. Similarly, De Visser et al. (2020) suggest that robots may influence trust in human–robot teams through “relationship equity”. Thus, robots have been shown to be able to influence team performance positively in general.
2.2. Robots and charismatic speech
Previous work on robot speech highlights the importance in considering robot speech characteristics and specifically the benefits of prosodic-expressive speech. Nass and Brave (2005), for instance, find that people prefer voice interfaces that match the listener in personality and emotional expression. Jung (Jung, 2017) suggests that robots need to align their emotional expression with users in order to participate in socially acceptable interactions with humans. Furthermore, the prosodic features of robot utterances may influence the robot's persuasiveness. Specifically, Fischer et al. (2020) show that robots are more persuasive when using speech characteristics similar to those used by charismatic speakers. They examine the effects of manipulating robot speech melody and other prosodic features on robot persuasiveness in three different studies. The robots' speech was manipulated to correspond to the speech characteristics of two famous CEO's, Steve Jobs or Mark Zuckerberg. Overall, the three studies demonstrate that robots that use Steve Jobs' speech characteristics, participants followed the respective robot's requests and implicit advice more often, and the robots were rated as more charismatic. Similarly, Fischer et al. (2021) find that the same manipulations in robot voices make students perform better in an (unrelated) foreign language learning task. Similarly, Westlund et al. (2015) used a more expressive speaking style produced by an actress, which led to better learning outcomes. Thus, a robot using a charismatic speaking style can encourage listeners to do more work or to do it more thoroughly.
These findings suggest that speech characteristics should be taken into account in robot speech creation and that they may potentially be quite powerful. At the same time, a recent study by Velner et al. (2020) suggests that simply copying general human speech characteristics may not lead to the expected results, so case by case analyses of the effects of speech characteristics in human-robot interaction seem warranted. Furthermore, in the studies reported on above, a broad range of acoustic-prosodic features was employed to manipulate the robot voices. While obviously effective, it is not clear from these studies which features contribute to the perception of the robot as charismatic. In the current study, we therefore focus on a minimum of manipulations to be able to better track the effects of particular speech characteristics.
3. The present study
Our study investigates the role of the robot's speech characteristics in promoting better team performance and creativity in a task that asks participants first to come up with associations about a given picture and then to jointly apply those associations to brainstorming ideas for a new product. The independent variable tested is therefore the robot's speaking style. The dependent variables concern robot perception, team performance and team creativity results from student teams completing a divergent-thinking task. Based on our literature review above, we expect:
H1: A robot acting as a creativity facilitator will be perceived more positively, as more charismatic and as having more interactive social capacities when it uses charismatic speech, compared to a robot that uses a less charismatic speaking style.
H2: Team performance will be perceived more positively when participants are exposed to a robot facilitator that uses charismatic speech, compared to a robot that uses a less charismatic speaking style.
H3: Creative outcomes will improve when group members are exposed to a robot facilitator that uses charismatic speech, such that they produce more, more original, more flexible, and more elaborate ideas, compared to a robot that uses a less charismatic speaking style.
4. Method
The study was carried out as a between-subject experiment, in which teams of students were instructed in a set of robot videos how to carry out a creativity task, where the robot used one speaking style in one condition and another in the other condition.
4.1. Participants
The participants of this study were students from five different Master and Bachelor programs in Philosophy, User Studies, Innovation Management, Communication and Design, based at four different universities in Denmark and Switzerland (convenience sample). The experiments were carried out during the COVID-19 pandemic, when all courses were held online. Team creativity was relevant in some way or other to the content of all of these courses, and the respective teachers had invited us in because they believed the activities involved to be interesting to their students. Altogether, there were 100 student participants in five different online classes; further demographic information was not elicited, in line with their identity as course participants. Each experiment session took place during their respective class lecture times via Zoom. The whole experiment from start to finish took about 30–40 min.
4.2. Experiment procedure
The experiment was presented to the student participants as a “Creativity Workshop”. The participants were not told anything about social robots, nor that a robot would be guiding their task; instead, they were told that the study was looking into how groups can work together creatively online.
In order to administer this Creativity Workshop, an online collective whiteboard Miro was used (see Figure 1). This allowed us to prepare a journey for them with different steps they had to perform, as well as the student groups to ideate together simultaneously using digital post-it notes and live mouse cursers that indicate a user's positions, movements, or contributions. This platform also helped the students with idea consolidation, where all ideas can be captured in one place (Brem, 2019).
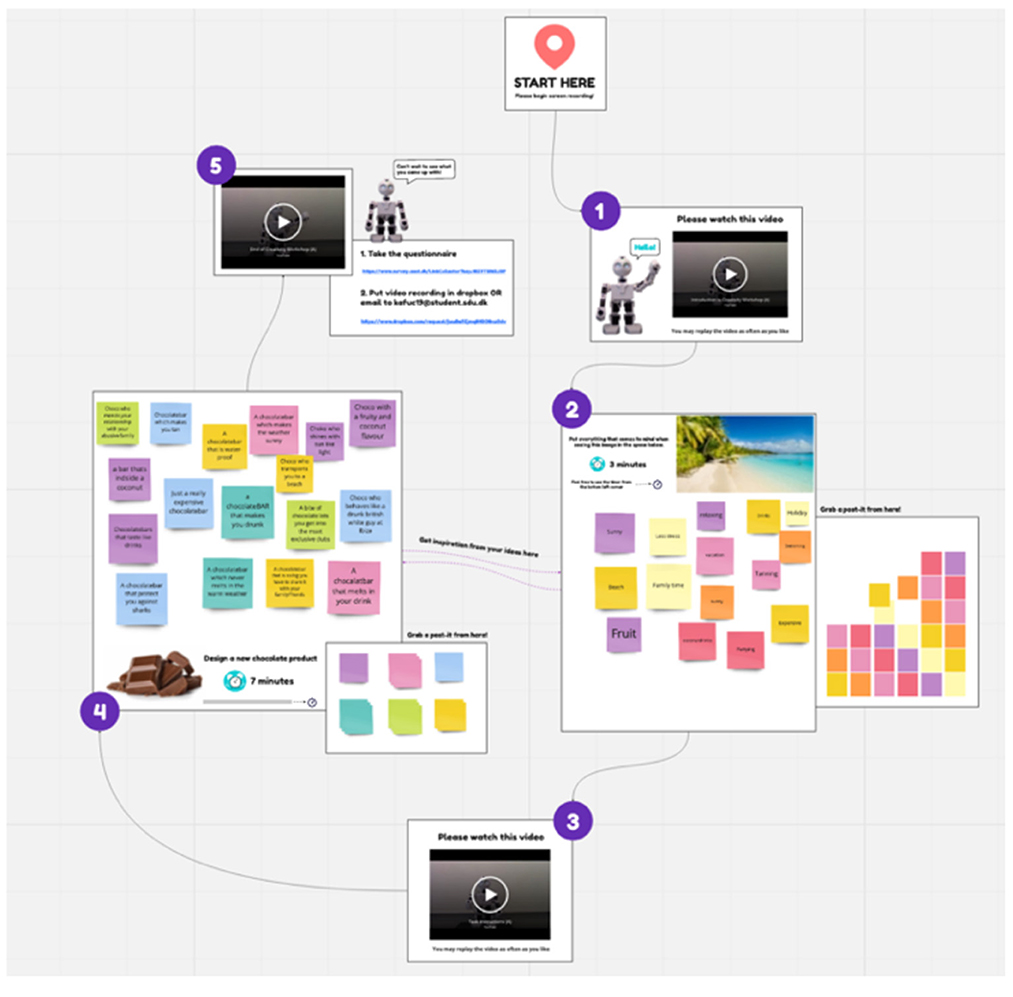
Figure 1. Experiment set-up on the miro board (3 robot videos, brainstorming task and idea generation task).
After a brief introduction to the “creativity workshop,” participants were divided into groups of 3–4 randomly using Zoom's breakout room feature, which moves users automatically into separate meeting rooms. Participants were then directed to Google Slides where they were given three sets of instructions: fill out a consent form, write their names down with their other group members next to their corresponding group number, and click on their designated Miro board link. Furthermore, we asked them to record their Miro board screen. The informed consent form was based on GDPR guidelines and stated that both participation and recording were voluntary (many groups chose not to record themselves); IRB evaluation was not provided since IRB evaluation is provided only for newly externally funded projects in the country in which the experiment was carried out. However, ethical considerations were discussed with an ethical advisor, the participants were provided with consent forms that fully conform with GDPR, and they were extensively debriefed.
When participants arrived at their designated Miro board, they began screen recording with video and audio. The boards were identical in both conditions, but different with respect to the videos provided. Each step of the task was numbered to help the student groups navigate the board.
The set-up of the experiment was created based on a creativity workshop technique described in Brem and Brem (2019) called visual synectics (see below), consisting of: idea collection and idea generation.
The first step was to watch the first video. In this video, the robot introduced itself and gave instructions on the first part of the task, with examples given to help avoid confusion. Participants were told to work together as a team and that there were no restrictions or evaluations on their ideas. They had 3 min to complete the first part of the task. The groups were provided with post-it notes on the side and a designated space for their idea generation. After the idea collection, participants were guided to the second video where the robot gave instructions for the second part of the task. Similar to the first video, groups were provided with examples, and they were explicitly told to be creative, to come up with as many ideas as possible and that there were no stupid ideas. This was done to prevent any possible limitations or fears of evaluation for the students. The groups had seven minutes to complete this portion of the task. When both parts of the task were completed, participants watched a final video in which the robot congratulated the group on their hard work and asked them to take the questionnaire. Then, participants were directed to complete the questionnaire. There were two identical questionnaires administered to the participants, one for those who were exposed to the first (charismatic) condition and the other for the second (non-charismatic) condition. Lastly, the groups were reminded to send a video of their screen recordings after the completion of the questionnaire.
All participants were then called back to the main session, where we asked them informally about their experience and about the robot. Finally, they were debriefed about the additional goals of the experiment (the official goal was to introduce them to a novel creativity technique, which it did).
4.3. Creativity task
A two-part divergent thinking task was developed for participants to complete based on a technique called Visual Synectics, which is a divergent thinking idea generating technique in which pictures are used as a visual stimulus to create useful ideas (Asanowicz, 2008). Successful creation depends on the ability to see metaphoric meaning, through a process called Conceptual Blending. Elements and relations between things are “blended” in a subconscious process through everyday thoughts and language. Insights gained from this process of blending promotes creative thinking and emphasizes the role of unpredictable associations and metaphors in creativity (Asanowicz, 2008).
In our study, each group was first given a photo of a beach as their visual element. They are then instructed to write down anything that comes to mind when seeing this image, such as attributes or feelings that arise in relation to the photo. For example, when seeing the image of the beach below, one may write down “palm trees”, “Hawaii”, or “family vacation”. After that, the groups were asked (in the second robot video) to develop a new chocolate product to be sold at grocery stores for the second part of the task. They were instructed to generate their ideas off of the descriptions they used for the first part of the task as inspiration. For example, one could think of “a chocolate bar in the shape of a palm tree” or “a chocolate bar wearing a lei in Hawaii”. It was encouraged that the group members should work together as a team when creating ideas in both parts of the task. This process is comparable to group brainstorming, because it can stimulate people to consider categories or thoughts that they might not otherwise think of Paulus et al. (2012).
The social robot acted as a creative workshop facilitator, where it explained the two-part task that participants in a group needed to complete. The social robot provided directions to the creativity task and some examples, and it explicitly encouraged the team members to be creative and work as a team together through direct and unambiguous instructions.
4.4. Experiment stimuli
The participants in each group watched three separate videos during the experiment. In the first video, the EZ-robot (see Figure 2) gave instructions for the first part of the task. In the second video, it provided the participants with instructions and examples for the second part of the divergent-thinking task. In the third video, the robot thanked the group for their hard work and reminded participants to fill out the questionnaire and to send their screen recording videos. A set of videos were made using the charismatic speech and another set of videos used the non-charismatic speech.
For the robot videos, the JD Humanoid robot “EZ-robot” was used, a small, stylized humanoid robot1 with 16 degrees of freedom and 16 servos, which can be operated by a simple interface. Its “eyes” consist of nine LEDs each. In the videos we recorded, the robot moved its arms and head slightly to match the content of the message in the corresponding manipulated audio file. Three videos were created, which were subsequently matched with two different versions of synthesized speech.
Two different versions of the robot's utterances were created from the same raw synthesized speech file called [en-us] Jack Bailey—S from a free Text to Speech Software (TTS) called wideo.co, which sounds like a male, adult American English speaker. Then, using the speech analysis software praat (Boersma and Weenink, 2001), the overall relationship between formants and pitch height was adjusted using the gender manipulation function in praat. For the more charismatic speaking style, the acoustic-prosodic features of the robot utterances were manipulated in the direction of a female speaker because this corresponds to a shorter vocal space. This in turn is related to smiling and higher arousal and hence engagement (Niebuhr, 2021). The manipulation was only adjusted by 5%, which is too little to make the male voice sound female. Rather, the result is a smiling-sounding male. For the non-charismatic speaking style, the manipulation was adjusted by 5% in the other direction. In other words, the voice is more male and less smiling sounding. The pitch level and pitch range were also changed, such that the charismatic voice is 15 hertz higher and the range is 3 semitones larger. In contrast, for the non-charismatic voice, the pitch height was lowered by 15 hertz and the range by 3 semitones. These manipulations are again related to speaker engagement and to higher flexibility (Berger et al., 2017). Furthermore, local manipulations of accents (i.e., emphasized syllables) in the charismatic prosody were done to increase the number of accents to 4–5 per minute by means of lengthening of voiced consonants, again for the sake of increasing the impression of engagement and flexibility. The accents in the synthesized voice were reduced to 0 for the non-charismatic prosody. It is important to note that the manipulated speech features in both conditions did not change the speaker's voice or voice quality itself. Also, both versions of the speech manipulation were clear, pleasant and understandable to users even though we refer to this condition as “non-charismatic”, as confirmed in the informal discussions with the participants after the experiments.2
4.5. Post-experimental questionnaire
In order to test the three hypotheses and determine if the charismatic prosody of a robot can influence creativity and team performance, previously validated scales were used in a questionnaire that participants took after watching the videos of the robot and completing the divergent thinking task. The measured variables used in the questionnaire include questions about charisma-related characteristics and the perceived sociality of the robot, and self-reported team performance. Most of the questions given to measure each variable used Likert-scales.
4.5.1. Subjective evaluation of the robot
The first part of the questionnaire asked participants to rate the robot with charisma-related questions as well as its perceived sociality. The charisma-related questions asked participants to rate the robot on a scale from 1 to 7 on the following adjectives, where 1 corresponds to “not at all” and 7 to “very much”. The adjectives used were: Enthusiastic, Charming, Convincing, Engaging, Boring, and Passionate. The scale from Fischer et al. (2020), was used, which in turn relied on measures used by Rosenberg and Hirschberg (2009) to study charismatic speech in humans. The adjectives “Self-confident”, “Uninspiring”, and “Charismatic” were added to the list of attributes to allow a more detailed analysis of the specific expected effects of the manipulations in speaking styles made.
4.5.2. Perceived sociality of the robot
The scale of perceived sociality measures the social robotic properties that can be related to the types of human experiences and interactive dispositions. The perceived sociality scale used in this study is based on previous research by Seibt et al. (2020), who argue that social interactions with robots are not always the result of anthropomorphizing, but of “sociomorphing”, i.e., the perception of actual non-human social capacities. The set of questions regarding perceived sociality asks what the participant anticipates from the social robot such as expectations of certain interactive capacities.
The participants rated six statements on a scale from 1 (strongly disagree) to 5 (strongly agree). The two statements, “The robot will understand what I feel” and “The robot will understand how I reason” ask about different types of expected empathy. The question, “The robot will want to be with me and close to me,” explores whether any capacity to experience and enjoy sociality is attributed to the robot. Whether participants attribute the social robot to the capacity of counter factional imagination is asked with the question, “The robot and I could try to imagine together what it would be like to be able to fly”. The statement, “If the robot were to walk off in all directions, I would keep it on a leash” is expected to indicate the relationship between capacities ascribed and moral dignity, which in turn helps to distinguish anthropomorphizing and sociomorphing (Seibt et al., 2020).
4.5.3. Perceived team performance
Team performance variables were measured using scales from previous work that relate to different team performance constructs: outcomes of teamwork as well as the team's process. Perceptions of outcomes of teamwork were measured using a 5-point Likert scale asking to rate their team's performance where 1 is “strongly disagree” and 5 is “strongly agree”. This scale used four subscales of teamwork outcomes: team effectiveness, team efficiency, team psychological safety, and team creative performance. Effectiveness refers to the degree to which team members meet the requirements and expectations of work quality as well as team collective goals (Velner et al., 2020). The items of team effectiveness were selected and slightly adapted from previous research on group effectiveness (Inglis, 1993; Velner et al., 2020). The questions include, “My team was effective in getting the task done” and “My team completed the task successfully”. Efficiency refers to the team's ability to adhere to means of producing satisfactory results with minimum inputs as well as the ability to complete tasks quickly (Velner et al., 2020). Efficiency-related questions include “Overall, my team did our task in a time-efficient way” and “My team completed this task smoothly”.
The subscale of team psychological safety is a construct that addresses a shared belief held by team members that the team is safe for interpersonal risk taking (De Visser et al., 2020). The term psychological safety is meant to suggest a sense of confidence that the team will not embarrass, reject, or punish someone for speaking up. Psychological safety is one of the key factors influencing team creativity performance (De Visser et al., 2020). Questions for psychological safety in this study include, “It was difficult to ask other members of this team for help”, “It is safe to take a risk on this team,” and “No one on this team would deliberately act in a way that would undermine my efforts.”
To measure a team's process, collective engagement was assessed using an Engagement Questionnaire (Salanova et al., 2003). It was slightly adapted for use in work groups. Collective engagement is central for creativity and occurs when group members stimulate one another's divergent thinking and their individual ideas are combined for the group's creative output (Harvey and Kou, 2013). The collective engagement measure is scored in three subgroups: Collective Vigor (seven items; e.g., “During the task, my group felt full of energy”), Collective Dedication (four items; e.g., “My group felt very motivated to do a good job”), and Collective Absorption (seven items; e.g., “Time was flying when my group was working”). The variables of the measure used a 5-point Likert scale asking to rate the questions from 1 to 5, where 1 is “strongly disagree” and 5 is “strongly agree”.
A selection of relevant questions based on a scale developed by Shin et al. (Shalley et al., 2004) was used to measure each team's self-perception w.r.t. their creative performance (Brem and Brem, 2019). There were two questions related to group perception: “My team members' work was original, adaptive, and practical,” and “My team members generated creative ideas”. Additionally, subjective creative perception questions were asked after they had worked with their teams in order to gauge their perceived creativity compared to the creativity task scoring. These two questions were, “How novel are your group's ideas,” and “How valuable are your group's ideas?”. Lastly, a 5-point slider question was presented on participants' perception of the extent to which they contributed to the group result, compared to their teammates.
4.5.4. Creativity analysis
Participants' productions were scored using Guilford's (Gilson et al., 2019) categories of divergent thinking perspectives: Fluency, Flexibility, Originality, and Elaboration. Fluency is the quantity of ideas a group generates. Therefore, if the group gave five responses, that would equal a score of 5. Flexibility is the number of different categories of relevant responses or the breadth of categories they cover with the ideas. Thus, if there were two categories identified, flexibility would be scored 2. Originality is the relative novelty and uniqueness of each answer. Each response is compared to the total amount of responses from all of the groups who participated in the task. Responses that were given by only 1% of the group are considered unique (1 point). Responses that were only given by that particular group are considered one of a kind (2 points). Higher total scores for a group indicate more original thinking. Elaboration is the amount of detail in the responses. For example, “a blue chocolate” = 0 points, but “a blue chocolate wearing sunglasses” = 1. An additional point would be given for any further details given, such as what the chocolate tastes like or details in the design. Since coding is highly mechanistic, in line with previous work [e.g., 26], we did not deem it necessary to calculate intercoder reliability.
Another indicator of divergent thinking, participation, was added for this study to see how much the group was collaborating together as a team. Participation was calculated by measuring the total amount of time participants spent talking compared to how long it took them to complete the two parts of the task. This was taken into account because more evenly distributed conversational turn-taking in a group can predict better performance, as well as a more active discussion leads to added innovative group performance (Tennent et al., 2019). The more time a group spent talking during the task, the better team performance is considered. The English subset of the screen recorded videos taken during the experiment were analyzed concerning the percentage of time that group members spent talking to one another in order to find their participation levels.
5. Results
The 100 student participants who attended the experiment formed 30 different groups who completed the task together as a team, and 76 students filled out the questionnaire completely; 40 students were exposed to condition 1 (C1, i.e., charismatic speech) and 36 students were exposed to condition 2 (C2, i.e., non-charismatic speech). In the inferential statistics below, MC1 andMC2 refer to the mean values of the two speaking-style or charisma conditions.
The questionnaire and behavioral data were analyzed using multivariate analyses of covariance (MANCOVAs). Three MANCONAs were performed, one per set of obtained rating data, i.e., creativity performance, subjective robot evaluation, and perceived sociality/team performance. Each MANCOVA aimed to test the effects of our independent variable, i.e., the two-level fixed factor Condition (speaking styles C1 vs. C2, see above), on the dependent variables represented by the rating scales in each set.
In order to reduce in these tests the statistical noise that has been introduced by distributing the overall experiment across several classes and subgroups of students, we made Class (the different courses in which the experiments were conducted) and Group (the small groups of 3–4 students in each class) covariates of the analysis. That is, Class and Group were random effects in our experimental procedure and we addressed them as such in the statistical tests. To that end, we split up Class and Group into categorical dummy variables, following the procedure suggested in the statistical-methods handbook by Howell (Howell, 2010, p. 600), and we defined both covariates as being categorical (non-continuous) in each MANCOVA. Note that this test design did not include interaction effects between the covariates and our dependent variables (ratings), but neither did we hypothesize any such interactions nor were relevant to the questions of our study.
With regard to the creativity performance (see Figure 3), we found a significant main effect of Condition (F[4, 69] = 6.893, p < 0.001, ηp2 = 0.286). The main effect is based on influences of speaking style on originality (M = 26.63, SD = 12.54 in the charismatic and M = 18.44, SD = 18.04 in the non-charismatic condition; F[1, 72] = 9.889, p = 0.002, ηp2 = 0.121) and elaboration (M = 9.95, SD = 5.82 vs. M = 7.33, SD = 4.15; F[1, 72] = 4.628, p = 0.035, ηp2 = 0.067). Both criteria scored 35–45% higher in connection with the robot's charismatic tone of voice. The MANCOVA also yielded significant main effects of both Class (F[4, 69] = 2.871, p = 0.029, ηp2 = 0.143) and Group (F[4, 69] = 7.758, p < 0.001, ηp2 = 0.310), however, these did not concern originality and elaboration. Classes differed in terms of flexibility (F[1, 72] = 13.019, p = 0.035, ηp2 = 0.153) and Groups in terms of Fluency (F[1, 72] = 4.127, p = 0.046, ηp2 = 0.054), with mainly one class or group standing out against the others.
Regarding the subjective evaluation of the robot (see Figure 4), Condition, i.e., speaking style, came out as the only significant main effect on participants' perception of the robot (F[9, 58] = 3.054, p = 0.005, ηp2 = 0.321). That is, ratings did not differ significantly as a function of the two covariates Class and Group. More specifically, the charismatic voice was found to make the robot sound more enthusiastic (M = 4.72, SD = 1.30 vs. M = 3.88, SD = 1.45; F[1, 66] = 10.257, p = 0.002, ηp2 = 0.135) and, above all, more passionate (M = 4.10, SD = 1.48 vs. M = 2.90, SD = 1.16; F[1, 66] = 13.622, p < 0.001, ηp2 = 0.171). Also, in line with these impressions, further statistical trends emerged showing that the robot with the charismatic speaking style tended to be perceived as less uninspiring (M = 2.9, SD = 0.26 vs. M = 3.54, SD = 0.29; F[1, 66] = 3.908, p = 0.052, ηp2 = 0.056) and as more charming (M = 4.6, SD = 0.29 vs. M = 3.9, SD = 0.32; F[1, 66] = 4.502, p = 0.079, ηp2 = 0.041).
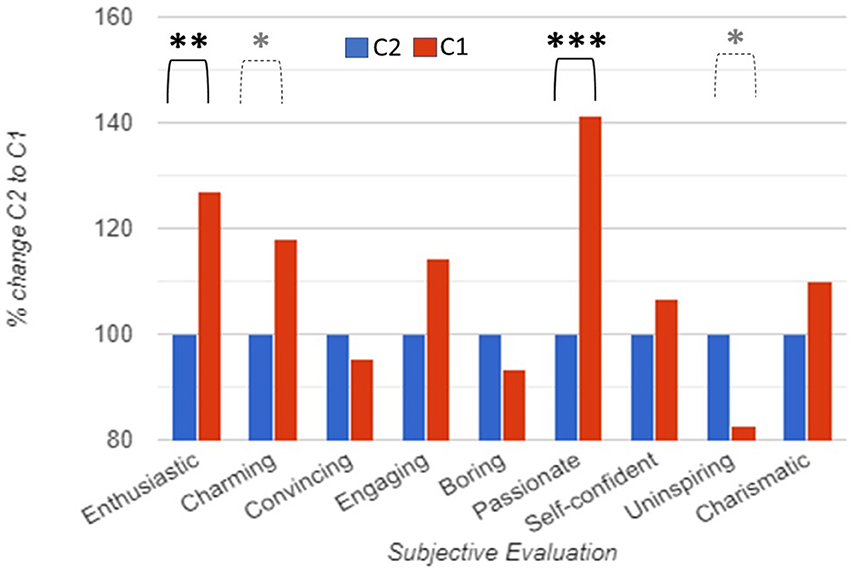
Figure 4. Relative differences in the subjective evaluation of the robot (C1: charismatic vs. C2: non-charismatic speech).
For the perceived sociality and perceived team performances, there was a main effect of Condition on four task- or output-oriented questions: “The robot will understand how I reason” (M = 2.1, SD = 0.21 vs. M = 2.6, SD = 0.23; F[1, 51] = 3.991, p = 0.047, ηp2=0.073), “My group felt very resilient during the task” (M = 3.05, SD = 0.93 for the charismatic and M = 3.47, SD = 0.84 for the non-charismatic condition; F[1, 51] = 4.087, p = 0.045, ηp2 = 0.074). Note that robot empathy and perceived team resilience were rated slightly better (higher) with the non-charismatic robot. In contrast, in the two questions on the perceived quality of ideas, it was the charismatic robot that caused the better (higher) team-performance ratings: “How valuable are your group's ideas” (M = 3.3, SD = 0.15 vs. M = 3.0, SD = 0.17; F[1, 51] = 4.270, p = 0.044, ηp2 = 0.077) and “How novel are your group's ideas” (M = 3.38, SD = 0.91 for the charismatic vs. M = 3.06, SD = 0.79 for the non-charismatic robot; F[1, 51] = 6.990, p = 0.011, ηp2 = 0.12).
Like for the creativity performance, team performance also differed significantly with respect to the two covariates, but only on one scale per covariate and again not along the same lines as for the factor Condition. The two affected scales concern group dynamics, i.e., what the team members thought about the group or the other group members. In the case of Class, differences emerged along the scale “My team members generated creative ideas” (F[1, 51] = 10.550, p = 0.002, ηp2 = 0.177). For Group, the affected scale was “My team completed this task smoothly” (F[1, 51] = 4.750, p = 0.034, ηp2 = 0.085).
We carried out a post hoc power analysis, based on the observed effect sizes of our main variable of 0.28 and 0.32; given our 76 participants, the estimated power is.99 for the creativity analyses and the subjective evaluation of the robot. However, given effect sizes of 0.07–0.12 for the perceived sociality and team performance questions, the study must be considered underpowered.
The analysis of the videos provided showed considerable differences between groups in terms of teamwork; some groups carried out the tasks silently and individually while others collaborated intensely, such that they built on each other's ideas and jointly discussed possible product innovations.
6. Discussion
In this study, the behavioral effects of a social robot's charismatic speech on human team members have been examined to see if creativity and team performance can be positively impacted. The results support our hypothesis (H3) that charismatic instructions by a robot improve team creativity concerning two of the four measures of creativity: “Originality” and “Elaboration.” Thus, the groups exhibited overall better creative processes and creative outcomes than groups that were facilitated by a non-charismatic speaker.
Similarly, if the social robot used a charismatic speaking style, it was perceived positively and was seen as having interactive social capacities, supporting the hypothesis (H1) that charismatic prosody leads to more positive attributions. Regarding the evaluation of the robot, participants viewed the robot that used the charismatic speech as more enthusiastic and passionate, and to a weaker extent also as less uninspiring and more charming. Remarkably, these attributions were consistent across courses and groups, confirming that charisma is “easy to sense but hard to define” (Niebuhr and Michalsky, 2019). The traits attributed to the more charismatic robot are likely to have an effect on the team members' creativity (see Niebuhr, 2021) by suggesting higher arousal levels and more engagement (the ‘smiling voice'), as well as more flexibility (more emphasized syllables and higher pitch range).
Similarly, regarding perceived sociality, team performance and perceived psychological safety, there are significantly better ratings for the charismatic robot, despite more (and significant) variation between courses and groups. All of these results point to the same conclusion, that when the robot uses charismatic speech characteristics, it is rated more positively with respect to traits that are associated to charisma than the robot with a non-charismatic prosody.
The only exceptions to the positive effects of the more charismatic voice of the robot concern the extent to which the participants perceived how resilient their teams were and how empathic the robot was. That is, what makes a meeting subjectively more successful, namely discipline, perseverance and focus, was more strongly triggered by the less charismatic robot, while what makes a creative meeting objectively more successful, namely commitment, a change of perspective, and finding new ideas, was supported by the more charismatic robot. Here we can only speculate what causes this effect; one possibility may be that when a team leader is less charismatic, the team will stand more together. The finding is related to a similar observation made in Niebuhr et al. (2021) on “the sound of successful meetings”. Based on a corpus of 70 idea-generation meetings of teams of 3–4 US high-school students, the authors investigated whether and how the interlocutors' prosody correlates with the subjective and objective performance indicators of such creativity-oriented meetings. They find that subjectively more successful meetings have a “sound” that is, amongst other things, characterized by an overall lower voice-pitch level, a significantly smaller pitch range and less pitch variability (e.g., due to fewer accent-related pitch movements). That is, the “sound” is more matter-of-factly and less charismatic. However, the actually objectively more successful meetings (in terms of the number generated ideas/solutions and their level of feasibility) were characterized by a livelier, more charismatic intonation in the sense of higher voice-pitch levels and larger and more frequent pitch movements. Why exactly this is so and why subjective and objective perceptions and indicators correlate and diverge in this way and with voice is a completely new type of question that must be addressed in future studies.
Overall, the impressions of the social robot made by participants imply that charismatic speech characteristics should be taken into account for robot synthesis in the future. This is in line with work on human communication, where speaking style is considered an essential component of speech communication (Camden and Kennedy, 1986). Furthermore, our results are consistent with findings by Fischer et al. (2020), that speech characteristics of robot speech may influence both the persuasiveness and impressionistic evaluation of the robot, and with the findings by Fay et al. (2015), that charismatic robot speech may lead to better student performance.
The results furthermore demonstrate that very few prosodic parameters can have significant effects on how the respective speaker is perceived and on how effective the robot is as a facilitator of team creativity tasks. While previous studies that demonstrated significant effects of robot speaking styles have relied on a large range of prosodic features, in this study, only pitch height, pitch range and number of accents were manipulated, i.e., how high the voice was, how large its range was, and how often the speech melody indicated that there was an important word. Thus, the study results can contribute to narrowing down which speech features the effects of charismatic speaker are actually determined by.
A possible limitation of the study is the fact that not all student groups were equally affected by the robot's speaking style as far as the objective creativity performance results are concerned. That is, we found that the courses and groups, in which the experiments were conducted, differed significantly with respect to some of the measures; specifically, classes differed in terms of flexibility and groups in terms of fluency, which means that the range of different ideas created was significantly different between students who study philosophy, design, communication, innovation management and user studies. Similarly, classes differed with respect to the extent to which they thought that they produced creative ideas. In contrast, groups differed concerning the number of ideas they produced and with respect to the extent to which they thought that they fulfilled the task smoothly. We do not know what these differences are caused by, whether they are intercultural differences, age difference, interindividual or just coincidental differences; for instance, in one class that was held as a block seminar, students reported to be extremely exhausted already before the experiment. Moreover, given their different disciplinary backgrounds, the students were used to both creativity techniques and team work to different degrees, and it turned out in the discussions after the experiments, that they also had experience with filling out such questionnaires to very different degrees. For instance, in one class, students reported that they thought that we were pulling their legs with the questionnaire; that is, they were completely unfamiliar with the questionnaire items that came from standardized questionnaires in psychology and related disciplines. Thus, potentially many different factors may have caused the differences between classes and groups observed; as noted by Gilson et al. (2019), even in the presence of the conditions that are essential for team creativity, other variables may influence the outcome, as team creativity is a complex phenomenon. It may have thus been beneficial to include some additional individual- and team-level factors, such as communication competence of the members, team history, team members' attitudes toward teamwork, etc. to improve the reliability of the findings. Furthermore, the fact the study relied on self-reports to tap into the team process may pose limitations to the validity of these findings. Nonetheless, variables such as psychological safety are meant to be assessed subjectively. Future work will have to reveal what those differences between the five classes are likely to be caused by. Nevertheless, the subjective evaluation of the robot with the charismatic speaking style as more or less enthusiastic and passionate was similar across the different classes or groups.
One may also object that participants were exposed to the robot only briefly and in non-interactive videos; thus we do not know to what extent long-term exposure to the robot's speaking style and a more interactive encounter with the robot might have. However, given the effects of human charismatic leaders and charismatic teachers on team performance (Nass and Brave, 2005; Niebuhr et al., 2016), we can expect that with more exposure or interaction, the effect will rather increase than decrease. Furthermore, studies that compare the effects of creativity techniques online and onsite report little effects of the setting; for instance, Cho and Cho [15x] show that students are more satisfied with offline collaboration and perceive offline collaboration as more effective, but they find no significant difference in student performance online and offline. We can therefore assume that charismatic speech characteristics will have similar effects in onsite creativity teamwork at least in terms of results as in the online teamwork described in this study.
7. Conclusion
Our study has shown that a charismatic speaking style may impact team creativity; specifically, very small changes in mean pitch, number of accents and pitch range can have a considerable effect on how original and elaborate team members' ideas are in a visual synectics task. A team creativity facilitor's speaking style may thus play an important role in the creative results of such teamwork in terms of originality and elaboration of the design ideas. Furthermore, the facilitator's speaking will impact how they are perceived as well as how valuable or novel the team members understand their results to be. Speaking style should thus be taken into consideration when designing robots as creativity facilitators.
Furthermore, we can conclude that by using a robot facilitator, we were able to uncover that speaking style may potentially influence creativity teams in general, and it may be useful to consider speaking style also as relevant variable in team creativity exercises in general.
Data availability statement
The raw survey data supporting the conclusions of this article will be made available by the authors, without undue reservation.
Ethics statement
Ethical review and approval was not required for the study on human participants in accordance with the local legislation and institutional requirements. The patients/participants provided their written informed consent to participate in this study.
Author contributions
Under the supervision of KFi and KFu designed the text for the stimuli and the Miro board and carried out the investigation. KFu also analyzed the students' productions with respect to their creativity. ON created the speech stimuli and carried out the statistical analysis. SN, KFi, and KFu jointly developed the experimental study design and the questionnaires. All authors contributed to the writing of the paper.
Funding
The research reported here is part of the SFACTOR project (Grant Nr. 105081) supported by the Nordforsk Foundation.
Conflict of interest
ON is CEO of the speech technology company AllGoodSpeakers ApS. A full statement in this regard can be found at https://oliverniebuhr.com/conflict-of-interest.html.
Publisher's note
All claims expressed in this article are solely those of the authors and do not necessarily represent those of their affiliated organizations, or those of the publisher, the editors and the reviewers. Any product that may be evaluated in this article, or claim that may be made by its manufacturer, is not guaranteed or endorsed by the publisher.
Footnotes
1. ^https://www.ez-robot.com/learn-robotics-getting-started-humanoid-robot-kit.html
2. ^Links to the videos: Condition 1 (charismatic prosody).
Video 1: https://youtu.be/x5wvRzulbzo.
Video 2: https://youtu.be/dk-eEv849pM.
Video 3: https://youtu.be/ecEQw9CNmCY.
Condition 2 (non-charismatic prosody).
Video 1: https://youtu.be/MLsXNImwmuM.
Video 2: https://youtu.be/CqszFYzSCJI.
Video 3: https://youtu.be/G3eR-mzIPbI.
References
Alderfer, C. P. (1977). “Group and intergroup relations,” in J. R. Hackman and J. L. Suttle eds., Improving the quality of work life (Palisades, CA: Goodyear), p. 227–296.
Ali, S., Moroso, T., and Breazeal, C. (2019). “Can Children Learn Creativity from a Social Robot?,” in Proceedings of the 2019 on Creativity and Cognition (CandC '19) Association for Computing Machinery, (New York, NY, USA), p. 359–368. doi: 10.1145/3325480.3325499
Alves-Oliveira, P., Arriaga, P., Cronin, M. A., and Paiva, A. (2020). “Creativity encounters between children and robots,” in Proceedings of the 2020 ACM/IEEE International Conference on Human-Robot Interaction (p. 379–388). doi: 10.1145/3319502.3374817
Amabile, T. M., Conti, R., Coon, H., Lazenby, J., and Herron, M. (1996). Assessing the work environment for creativity. Acad. Manage. J. 39, 1154–1184. doi: 10.2307/256995
Asanowicz, A. (2008). “How to Find an Idea?—Computer Aided Creativity,” Architecture in Computro [26th ECAADe Conference Proceedings], p. 735–742. http://papers.cumincad.org/cgi-bin/works/Show?ecaade2008_025 doi: 10.52842/conf.ecaade.2008.735
Barrett, M., Oborn, E., Orlikowski, W. J., and Yates, J. (2012). Reconfiguring boundary relations: robotic innovations in pharmacy work. Organiz Sci. 23, 1448–1466. doi: 10.1287/orsc.1100.0639
Berger, S., Niebuhr, O., and Peters, B. (2017). “Winning over an audience—a perception-based analysis of prosodic features of charismatic speech,” in Proceedings of the 43rd Annual Conference of The German Acoustical Society (p. 1454–1457).
Boersma, P., and Weenink, D. J. M. (2001). PRAAT, a system for doing phonetics by computer. Glot Int. 4, 341–345.
Brem, A. (2019). Creativity on demand: how to plan and execute successful innovation workshops. IEEE Eng Manage Rev. 47, 94–98. doi: 10.1109/EMR.2019.2896557
Brem, A., and Brem, S. (2019). Die Kreativ-Toolbox für Unternehmen. Ideen generieren und innovatives Denken fördern. Stuttgart.
Camden, C. T., and Kennedy, C. W. (1986). Manager communicative style and nurse morale. Hum. Commun. Res., 12, 551–563. doi: 10.1111/j.1468-2958.1986.tb00091.x
Cho, J. Y., and Cho, M. H. (2014). Student perceptions and performance in online and offline collaboration in an interior design studio. Int J Technol Des Educ 24, 473–491. doi: 10.1007/s10798-014-9265-0
Cousins, K. C., Robey, D., and Zigurs, I. (2007). Managing strategic contradictions in hybrid teams. Eur J Inform Syst. 16, 460–478. doi: 10.1057/palgrave.ejis.3000692
Crumpton, J., and Bethel, C. L. (2015). A survey of using vocal prosody to convey emotion in robot speech. Int. J. Soc. Robot. 8, 271–285. doi: 10.1007/s12369-015-0329-4
De Visser, E. J., Peeters, M. M., Jung, M. F., Kohn, S., Shaw, T. H., Pak, R., et al. (2020). Towards a theory of longitudinal trust calibration in human–robot teams. Int. J. Social Robot. 12, 459–478. doi: 10.1007/s12369-019-00596-x
Elgarf, M., Skantze, G., and Peters, C. (2021). “Once upon a story: can a creative storyteller robot stimulate creativity in children?,” in Proceedings of the 21th ACM International Conference on Intelligent Virtual Agents (p. 60–67). doi: 10.1145/3472306.3478359
Fay, D., Shipton, H., West, M. A., and Patterson, M. (2015), Teamwork organizational innovation. Creativity Innovat. Manage. 24, 261–277. doi: 10.1111/caim.12100
Fischer, K., Niebuhr, O., and Alm, M. (2021). Robots for foreign language learning: speaking style influences student performance. Front. Robot. AI, 273. doi: 10.3389/frobt.2021.680509
Fischer, K., Niebuhr, O., Jensen, L. C., and Bodenhagen, L. (2020). Speech melody matters—how robots profit from using charismatic speech. ACM Trans. Hum. Robot Interact. 9, 1–21. doi: 10.1145/3344274
Geerts, J., de Wit, J., and de Rooij, A (2021). Brainstorming with a social robot facilitator: better than human facilitation due to reduced evaluation apprehension?. Front. Robot. AI, 8, 156. doi: 10.3389/frobt.2021.657291
Gilson, L. L., Lee, Y. S. H., and Litchfield, R. C. (2019). Advances in Team Creativity Research. Oxford Research Encyclopedia of Business and Management. Oxford: Oxford University Press. doi: 10.1093/acrefore/9780190224851.013.171
Gilson, L. L., Lim, H. S., Litchfield, R. C., and Gilson, P. W. (2015). “Creativity in teams: A key building block for innovation and entrepreneurship,” in C.E. Shalley, M.A. Hitt, and J. Zhou (Eds.), The Oxford handbook of creativity, innovation, and entrepreneurship (Oxford University Press), p. 177–204.
Hackman, J. R. (1992). “Group influences on individuals in organizations,” in M. D. Dunnette and L. M. Hough, eds. Handbook of industrial and organizational psychology (Palo Alto, CA: Consulting Psychologist Press), p. 199–267.
Han, J-. H., Jo, M-. H., Jones, V., and Jo, Jun-H. (2008). Comparative study on the educational use of home robots for children. J. Inform. Process. Syst. 4, 159–168. doi: 10.3745/JIPS.2008.4.4.159
Harvey, S., and Kou, C-. Y. (2013). Collective engagement in creative tasks. Administrat. Sci.Quarterly, 58, 346–386. doi: 10.1177/0001839213498591
Hinds, P., and Pfeffer, J. (2003). “Why organizations don't “know what they know”: Cognitive and motivational factors affecting the transfer of expertise,” in M. Ackerman, V. Pipek, and V. Wulf, eds., Beyond knowledge management: Sharing expertise (Cambridge, MA: MIT Press), p. 3–26.
Hinds, P. J., and Bailey, D. E. (2003). Out of sight, out of sync: understanding conflict in distributed teams, organization. Science. 14, 615–632. doi: 10.1287/orsc.14.6.615.24872
Holladay, S. J., and Coombs, W. T. (1994). Speaking of visions and visions being spoken an exploration of the effects of content and delivery on perceptions of leader charisma. Manage. Commun. Quarterly, 8, 165–189. doi: 10.1177/0893318994008002002
Inglis, M. (1993). The communicator style measure applied to nonnative speaking teaching assistants. Int. J. Intercultural Relat. 17, 89–105. doi: 10.1016/0147-1767(93)90014-Y
Jung, M. F. (2017). “Affective grounding in human-robot interaction,” in Proceedings of the 2017 ACM/IEEE International Conference on Human-Robot Interaction. doi: 10.1145/2909824.3020224
Jung, M. F., Martelaro, N., and Hinds, P. J. (2015). “Using robots to moderate team conflict: the case of repairing violations,” in Proceedings of the Tenth Annual ACM/IEEE International Conference on Human-Robot Interaction (pp. 229–36). doi: 10.1145/2701973.2702094
Kahn, P. H., Kanda, T., Ishiguro, H., Gill, B. T., Shen, S., Ruckert, J. H., et al. (2016). “Human creativity can be facilitated through interacting with a social robot,” in 2016 11th ACM/IEEE International Conference on Human-Robot Interaction (HRI). doi: 10.1109/HRI.2016.7451749
Kozlowski, S. W. J., and Bell, B. S. (2003). “Work groups and teams in organizations,” in W.C. Borman, D. R. Ilgen, and R. J. Klimoski, eds. Handbook of psychology, Vol. 12. Industrial and organizational psychology (London: Wiley), p. 333–375. doi: 10.1002/0471264385.wei1214
Leavitt, H. J. (1974). Suppose We Took Groups Seriously…Prepared for Western Electrics Symposium on the Hawthorne Studies, pp. 1–20. https://eric.ed.gov/?id=ED103291.
LePine, J.A., Piccolo, R.F., Jackson, C.L., Mathieu, J.E., and Saul, J.R. (2008). A meta-analysis of teamwork processes: tests of a multidimensional model and relationships with team effectiveness criteria. Pers. Psychol. 61, 273–307. doi: 10.1111/j.1744-6570.2008.00114.x
McDowell, T., Agarwal, D., Miller, D., Okamoto, T., and Page, T. (2016). “Organizational design: The rise of teams,” in Global Human Capital Trends 2016, Deloitte Insights.
McGinn, C., and Torre, I. (2019). “Can you Tell the Robot by the Voice? An Exploratory Study on the Role of Voice in the Perception of Robots,” in 2019 14th ACM/IEEE International Conference on Human-Robot Interaction (HRI). doi: 10.1109/HRI.2019.8673305
Murphy, S. E., and Ensher, E. A. (2008). A qualitative analysis of charismatic leadership in creative teams: the case of television directors. Leadership Quarterly 19, 335–352. doi: 10.1016/j.leaqua.2008.03.006
Nass, C., and Brave, S. (2005). Wired for Speech: How Voice Activates and Advances the Human-Computer Relationship. Cambridge, MA: MIT Press.
Niebuhr, O. (2021). “Advancing Higher-Education Practice by Analyzing and Training Students' Vocal Charisma: Evidence from a Danish Field Study,” in 7th International Conference on Higher Education Advances, Spain. p. 743–751. doi: 10.4995/HEAd21.2021.12827
Niebuhr, O., Böck, R., and Allen, J. R. (2021). “On the Sound of Successful Meetings: How Speech Prosody Predicts Meeting Performance,” in ACM Proc. 23rd International Conference on Multimodal Interaction (Montreal, Canada), p. 1–10. doi: 10.1145/3461615.3485412
Niebuhr, O., Brem, A., Novák-Tót, E., and Voße, J. (2016). “Charisma in business speeches: A contrastive acoustic-prosodic analysis of Steve Jobs and Mark Zuckerberg,” in J. Barnes, A. Brugos, S. Shattuck-Hufnagel, and N. Veilleux (Eds.) Proceedings of the 8th International Conference of Speech Prosody (Speech Prosody Special Interest Group. Speech Prosody), p. 79.
Niebuhr, O., and Michalsky, J. (2019). “Computer-generated speaker charisma and its effects on human actions in a car-navigation system experiment—or how steve jobs' tone of voice can take you anywhere,” in International Conference on Computational Science and its Applications (Cham: Springer), p. 375–390. doi: 10.1007/978-3-030-24296-1_31
Paulus, P. B., Dzindolet, M., and Kohn, N. W. (2012). Collaborative creativity—group creativity and team innovation. Handbook Organiz. Creativity 327–357. doi: 10.1016/B978-0-12-374714-3.00014-8
Puranam, P. (2018). The Microstructure of Organizations. Oxford, England: Oxford University Press. doi: 10.1093/oso/9780199672363.001.0001
Rosenberg, A., and Hirschberg, J. (2009). Charisma perception from text and speech. Speech Commun. 51, 640–655. doi: 10.1016/j.specom.2008.11.001
Rosenberg-Kima, R. B., Koren, Y., and Gordon, G. (2020). Robot-supported collaborative learning (rscl): social robots as teaching assistants for higher education small group facilitation. Front. Robot. 6, 148. doi: 10.3389/frobt.2019.00148
Salanova, M., Llorens, S., Cifre, E., Martínez, I. M., and Schaufeli, W. B. (2003). Perceived collective efficacy, subjective well-being and task performance among electronic work groups. Small Group Res. 34, 43–73. doi: 10.1177/1046496402239577
Salas, E., Cooke, N. J., and Rosen, M. A. (2008). On teams, teamwork, and team performance: discoveries and developments. Human Factors J. Hum. Factors Ergonomics Soc. 50, 540–547. doi: 10.1518/001872008X288457
Salas, E., Dickinson, T. L., Converse, S. A., and Tannenbaum, S. I. (1992). “Toward an understanding of team performance and training,” in: R.W. Swezey and E. Salas, eds. Teams: Their training and performance (Norwood, NJ: Ablex), p. 3–29.
Sebo, S., Stoll, B., Scassellati, B., and Jung, M. F. (2020). Robots in groups and teams: a literature review. Proceed. ACM Hum. Computer Interact. 4, 1–36. doi: 10.1145/3415247
Seibt, J., Vestergaard, C., and Damholdt, M. F. (2020). Sociomorphing, not anthropomorphizing: towards a typology of experienced sociality. Front. Artif. Intell. Appl. 335, 51–67. doi: 10.3233/FAIA200900
Shalley, C. E., Zhou, J., and Oldham, G. R. (2004). The effects of personal and contextual characteristics on creativity: where should we go from here? J. Manage. 30, 933–958. doi: 10.1016/j.jm.2004.06.007
Shin, Y., Kim, M., and Lee, S-. H. (2016). Reflection toward creativity: team reflexivity as a linking mechanism between team goal orientation and team creative performance. J. Bus. Psychol. 32, 655–671. doi: 10.1007/s10869-016-9462-9
Sørensen, L. S. (2013). How to grow an apple: Did Steve Jobs speak Apple to success?- An analysis of Steve Jobs' rhetorical and linguistic development in relation to Apple's organizational performance. Masterthesis. Aalborg University.
Tennent, H., Shen, S., and Jung, M. (2019). “Micbot: a peripheral robotic object to shape conversational dynamics and team performance,” in 2019 14th ACM/IEEE International Conference on Human-Robot Interaction (HRI). doi: 10.1109/HRI.2019.8673013
Velner, E., Boersma, P. P., and de Graaf, M. M. (2020). “Intonation in robot speech: does it work the same as with people?,” in Proceedings of the 2020 ACM/IEEE International Conference on Human-Robot Interaction (p. 569–578). doi: 10.1145/3319502.3374801
Westlund, J. K., Gordon, G., Spaulding, S., Lee, J. J., Plummer, L., Martinez, M., et al. (2015). Learning a second language with a socially assistive robot. Almere, The Netherlands.
Keywords: charismatic speech, human-robot interaction, team creativity, robot speech, speech prosody
Citation: Fucinato K, Niebuhr O, Nørskov S and Fischer K (2023) Charismatic speech features in robot instructions enhance team creativity. Front. Commun. 8:1115360. doi: 10.3389/fcomm.2023.1115360
Received: 03 December 2022; Accepted: 10 April 2023;
Published: 22 May 2023.
Edited by:
Sandra Madureira, PUCSP, BrazilCopyright © 2023 Fucinato, Niebuhr, Nørskov and Fischer. This is an open-access article distributed under the terms of the Creative Commons Attribution License (CC BY). The use, distribution or reproduction in other forums is permitted, provided the original author(s) and the copyright owner(s) are credited and that the original publication in this journal is cited, in accordance with accepted academic practice. No use, distribution or reproduction is permitted which does not comply with these terms.
*Correspondence: Kerstin Fischer, kerstin@sdu.dk