- Amsterdam Center for Language and Communication, University of Amsterdam, Amsterdam, Netherlands
Introduction: Research indicates that statistical learning plays a role in word learning by enabling the learner to track the co-occurrences between words and their visual referents, a process that is named cross-situational word learning. Word learning is problematic for children with developmental language disorder (DLD), and a deficit in statistical learning has been suggested to contribute to the language difficulties in these children. Therefore, we investigate whether children with DLD have more difficulty than TD children with learning novel word–referent pairs based on cross-situational statistics in an implicit task, and whether this ability is related to their lexical-semantic skills. Moreover, we look at the role of variability of the learning environment.
Methods: In our implicit cross-situational word learning task, each trial in the exposure phase was in itself ambiguous: two pictures of unknown objects were shown at the same time and two novel words were played consecutively, without indicating which word referred to which object. However, as every word occurred with its correct referent consistently, the children could learn the word–referent pairs across trials. The children were not explicitly instructed to learn the names of new objects. As an on-line measure of learning, eye-movements were recorded during the exposure phase. After exposure, word–referent knowledge was also tested using multiple choice questions. Several measures of lexical-semantic knowledge were administered to the children with DLD, as well as tasks measuring non-verbal intelligence and phonological processing. Contextual variability (the number of different distractors with which a particular word–referent pair occurs across trials) was manipulated between subjects by constructing two types of exposure conditions: low contextual diversity vs. high contextual diversity.
Results: Both groups of children performed significantly above chance level on the test phase, but the TD children significantly outperformed the children with DLD. We found no significant effect of contextual diversity. The eye-tracking data revealed some evidence of on-line learning, but no differences between groups. Finally, the regression analyses did not reveal any significant predictors of off-line or on-line cross-situational word learning ability.
Discussion: Our results indicate that although children with DLD are able to learn word-referent pairs in an implicit task, they have more difficulty than TD children. Possibly they need more input to achieve the same level.
1. Introduction
Young children learn a large number of words in a relatively short period of time, knowing an estimate of 14.000 words at 6 years old (Suanda et al., 2014). How are they able to do this? This question is especially puzzling considering the referential ambiguity problem that children often experience (Quine, 1960): children hear a word unknown to them and see multiple potential referents at the same time. How do they learn to match words to their correct referents? Recent research into cross-situational word learning has indicated that statistical learning plays a role in tracking co-occurrences between words and their corresponding referents. This type of learning may thus be important for word learning (Yu and Smith, 2007; Smith and Yu, 2008; Kachergis et al., 2014; Suanda et al., 2014; Yurovsky et al., 2014).
Learning words requires more effort for some children than for others. Children with developmental language disorder (DLD) often have difficulties with the development of word knowledge (Brackenbury and Pye, 2005; Sheng and McGregor, 2010; McGregor et al., 2013; Nation, 2014). Evidence suggests that an impairment in statistical learning, a learning mechanism that supports the extraction of patterns and regularities from sensory input, contributes to the language difficulties in these children (Evans et al., 2009; Hedenius et al., 2011; Hsu and Bishop, 2014; Mainela-Arnold and Evans, 2014; Haebig et al., 2017; Lammertink et al., 2017). The current study aims to investigate whether a cross-situational word learning deficit might (partially) explain their hampering lexical acquisition. Difficulty in tracking the co-occurrences between words and their corresponding referents might result in a problematic vocabulary development in children with DLD.
On the basis of accuracy and eye-tracking data, we investigate whether children with DLD have more difficulty than typically developing (TD) children when learning word–referent pairs in a cross-situational word learning experiment, as well as whether this cross-situational word learning ability is related to different types of vocabulary knowledge in children with DLD. Moreover, as previous research has shown that high variability in the learning environment might enhance statistical learning (Grunow et al., 2006; von Koss Torkildsen et al., 2013), we manipulate the contextual diversity of the to-be-learned word–referent pairs in our experiment to investigate whether this affects cross-situational word learning in children with and without DLD.
The acquisition of vocabulary starts in early infancy, continuing throughout life. The acquisition of a rich vocabulary entails several competences, including (but not limited to) the discovery of word forms, learning about concepts and word meanings, word–meaning association and the expansion of lexical representations (Yu and Ballard, 2007; Ralli et al., 2010). Previous studies have shown that statistical learning likely contributes to at least part of these processes. For example, segmenting words from running speech in which word boundaries are not consistently indicated is supported by statistical learning mechanisms (Saffran et al., 1996, 1997; Graf Estes, 2009). In addition to defining a word, children also need to map those word forms to their corresponding referents in the real world. The ability of fast mapping is important in early word learning (see Horst and Samuelson, 2008 for a review). Fast mapping is described as an “all-or-none” learning mechanism for which one single exposure to a new word and its corresponding referent is sufficient to link them in memory. However, this is just the start of building elaborate lexical entries. After fast mapping, the meaning and scope of the word needs to be further specified and the words needs to be placed in a broader network of related words, a process also called “slow mapping” (Carey, 1978; Blythe et al., 2010), which requires repeated exposure to words. Moreover, the research by Horst and Samuelson (2008) suggests that fast mapping is not sufficient for long-term word knowledge: after a mere 5 min, the 24-month-old infants in their fast-mapping experiment could no longer express what they had learned.
Moreover, word-learning contexts outside the lab are usually much more ambiguous than in controlled experiments. The fast speech stream and the visual world that young children encounter contain many (new) words and many different potential referents. How does a child learn the correct word–referent mappings? Referential ambiguity, or the word-to-world mapping problem, has been described by Quine (1960) and many others: “In any naming event, a novel word can refer to any object present, its properties, the speaker's feelings or intentions for it, an impending action, or something else altogether” (McMurray et al., 2012, p. 832). Thus, real-life learning situations might often not be ideal fast-mapping situations.
Another factor that needs consideration is that the number of possible referents is constrained by built-in or learned biases. For example, words usually refer to whole objects rather than parts or properties of an object (MacNamara, 1972), and children know that a novel word should not be linked to a referent that already is linked to another word (mutual exclusivity; Markman and Wachtel, 1988). Moreover, identifying the attentional focus of the speaker (Baldwin, 1991) and syntactic bootstrapping (Gleitman et al., 2005) reduces referential ambiguity (Blythe et al., 2010). Although these biases play an important role in word learning, evidence suggests that statistical learning mechanisms could be used on top of that to exploit an environment in which there is often a degree of (referential) uncertainty. Word–referent mapping could be viewed as a gradual, accumulative process: the learner can reduce referential uncertainty and extend meaning representations when a word is encountered in different contexts. This would mean that children can make use of ambiguous learning situations rather than only learn when there is no ambiguity at all (Yurovsky et al., 2014). Thus, words are not (always) learned in one single, unambiguous event. Rather, children use statistical learning, that is the ability to use information about the co-occurrence of words and referents from many different encounters, to acquire a vocabulary network.
In cross-situational word-learning tasks (Yu and Smith, 2007; Smith and Yu, 2008), participants are usually exposed to multiple novel words and multiple novel objects per learning trial. In these tasks, each individual learning trial is in itself ambiguous, as multiple words and referents appear simultaneously, with no indication as to which word should be mapped to which referent. However, as the correct word–referent pairs do consistently occur together, the correct mappings can be learned by accumulating evidence across trials. This task is a (strongly) simplified simulation of real word-learning situations in which there is often some amount of referential ambiguity. Studies have shown that adults (Yu and Smith, 2007; Fitneva and Christiansen, 2011; Smith et al., 2011; Suanda and Namy, 2012; Kachergis et al., 2014), infants (Smith and Yu, 2008; Yu and Smith, 2011; Vlach and Johnson, 2013) and 5–7 year old children (Suanda et al., 2014; Vlach and DeBrock, 2017) are able to learn word–referent pairs in this paradigm, after only a few minutes of exposure. Eye-tracking has been used as a measure of on-line learning in cross-situational word-learning tasks in adults (Fitneva and Christiansen, 2011; Yu et al., 2012), infants (Yu and Smith, 2011), children with and without autism (Venker, 2019) and children with and without DLD (Ahufinger et al., 2021), allowing for more fine grained analyses of learning. Besides behavioral experiments, computational models show that cross-situational learning mechanisms could explain learning large vocabularies in a relatively short amount of time in spite of referential ambiguity (Blythe et al., 2010; Yu and Smith, 2012), although it is still unclear whether associative learning, hypothesis testing strategies or both may underlie this ability.
Children with DLD have evident problems in the development of the lexicon, although grammatical problems are generally more apparent (Brackenbury and Pye, 2005; Nation, 2014; Jackson et al., 2019b). These reported vocabulary problems may last into adulthood (McGregor et al., 2017) and include smaller vocabulary size and more superficial word knowledge (McGregor et al., 2013), less accurate word naming (Leonard et al., 1983; McGregor, 1997; Lahey and Edwards, 1999; Dockrell et al., 2001; McGregor et al., 2002), impoverished semantic representations (Marinellie and Johnson, 2002; Dockrell et al., 2003; Mainela-Arnold et al., 2010; Drljan and Vuković, 2019) and less efficient lexical-semantic networks (Sheng and McGregor, 2010; McGregor et al., 2012; Drljan and Vuković, 2019; Sandgren et al., 2021).
Learning new words is generally problematic in this population (Nash and Donaldson, 2005; Alt and Plante, 2006; Kan and Windsor, 2010; Haebig et al., 2017; Kapa and Erikson, 2020). For example, Alt and Plante (2006) reported that children with DLD have difficulty with learning phonological forms of words as well as learning about semantic properties of words such as color and shape. Evidence also suggests that fast mapping is difficult for children with DLD (Rice et al., 1994; Alt et al., 2004; Gray, 2004; Haebig et al., 2017; Jackson et al., 2019a; see Jackson et al., 2019b for a review of the different types of word learning experiments that have been tested in individuals with DLD).
Thus, the lexical-semantic development in children with DLD is affected, but the underlying cause of these difficulties is still under debate. Phonological short-term memory has often been reported as being more limited in children with DLD compared to TD children, and has been hypothesized to contribute to their lexical-semantic deficits (see Montgomery et al., 2010 for a review). Indeed, phonological short-term memory has been shown to impact fast mapping in children with DLD (Alt and Plante, 2006; Jackson et al., 2019a), and results by Quam et al. (2020) indicate that sound discrimination ability affects word–object mapping in children with DLD. Whether there is actually a causal relation between phonological memory and lexical abilities is still debated (Melby-Lervåg et al., 2012).
Another strand of DLD research focuses on learning mechanisms that are not specific to language. A growing body of evidence implies that impaired statistical learning underlies the language deficits in children with DLD. These children seem to have more difficulty with extracting patterns from their environment, for example when extracting words from running speech in a word segmentation task (Evans et al., 2009; Haebig et al., 2017), learning non-adjacent dependencies in an artificial grammar learning task (Hsu et al., 2014; Lammertink et al., 2019), or learning motor sequences in a serial reaction time task (Lukács and Kemény, 2014). Meta-analyses also point into the direction of a statistical learning deficit in children with DLD (Lum et al., 2014; Obeid et al., 2016; Lammertink et al., 2017). Please note, however, that some studies report no evidence for (or against) a statistical learning deficit (Aguilar and Plante, 2014; Noonan, 2018; Lammertink et al., 2020a,b). Importantly, statistical learning ability has been shown to be correlated with language abilities in TD children (Newman et al., 2006; Conway et al., 2010; Kaufman et al., 2010; Misyak et al., 2010; Kidd, 2012; Shafto et al., 2012; Ellis et al., 2014; Spencer et al., 2015; Kidd and Arciuli, 2016; Hamrick et al., 2018) and in children with DLD (Tomblin et al., 2007; Evans et al., 2009; Misyak et al., 2010; Hedenius et al., 2011; Mainela-Arnold and Evans, 2014).
As discussed above, statistical learning seems to play an important role in the development of the lexicon. This hypothesized relation is underlined by findings of positive correlations between statistical learning ability and vocabulary size (Spencer et al., 2015) and even more strongly by the finding of predictive relationships between statistical learning and later vocabulary development in longitudinal studies (Shafto et al., 2012; Singh et al., 2012; Ellis et al., 2014). In children with DLD, this relationship has also been found (Evans et al., 2009; Mainela-Arnold and Evans, 2014). However, the relationship between statistical learning and specifically the largely unexplained lexical-semantic difficulties in children with DLD is not yet clear. The cross-situational word-learning paradigm offers a way to investigate the role of statistical learning in finding a word's meaning.
Cross-situational word learning has only sparsely been investigated in children with DLD. However, incidental word learning has been studied using the quick incidental word learning (QUIL) paradigm, which aims to mimic naturalistic word learning (Rice et al., 1990). In these tasks, new words are not explicitly taught but embedded in video stories. Children with DLD learn fewer words in such tasks (see Chung and Yim, 2020 for a summary). Findings by Rice et al. (1994) indicate that children with DLD are able to learn new word–referent mappings in a QUIL task, but need more exposure to the words than TD children. Correlations between QUIL ability and language skills have been reported by Gordon et al. (1992) and Yang et al. (2013). In a recent study, Chung and Yim (2020) investigated QUIL in 4–6-year-old children with and without DLD, also measuring eye movements during learning. In the task, the children were exposed to a 5-min-long video story in which five novel words had been embedded in sentences, each word three times, without further instructions. Afterwards it was tested whether the children could pick the right object corresponding to the novel words. Results showed that children with DLD score lower on this task, suggesting that they learn fewer words from watching this video. Moreover, the eye-tracking data revealed that children with DLD fixate less often on these target objects over time, while the fixations of TD children increase over time, and their looks are more widely scattered in general. As fixation time predicts word learning, the gazing pattern of children with DLD seems to reflect their difficulty linking new words to their referents.
The study by Ahufinger et al. (2021) was the first to directly test children with DLD on the ability of tracking the co-occurrences between multiple words and visual referents in a cross-situational word learning task. In their experiment, children with and without DLD were subjected to a familiarization phase in which they could learn the names for eight robot-like figures in 16 trials. In each learning trial, the participants saw two pictures and heard two words, without any indication as to which word referred to which picture. After familiarization, the children were tested twice on each word–referent pair using four-alternative forced choice questions. Moreover, eye movements were measured during the familiarization phase and the testing phase. Although both groups of children performed significantly above chance level on the testing phase, the children with DLD had learned significantly fewer word–referent pairs than the TD children. The eye-tracking data did not reveal any preferences for target or distractor items during the familiarization phase, nor significant group differences in looking behavior. Eye-movements during the test phase were interpreted by the authors as a measure of the confidence children had in their answer. When only trials in which the participant had given the right answer were included in the analysis, the TD children looked significantly longer toward the target image than the children with DLD, indicating that TD children are more confident in their answer than children with DLD.
McGregor et al. (2022) investigated cross-situational word learning in children with DLD, with the aim of examining how learner characteristics influence this ability. In their cross-situational word learning task, overt responses were recorded during the learning phase of the experiment, enabling the researchers to track learning during the exposure to word–referent pairs. Accuracy on word–form retention (recognizing a trained word from three possibilities, for example zote, zoke or zofe) and word–referent retention (matching the correct picture to a trained word) was also measured after a 5 min interval. In every learning trial, the children saw two pictures and heard one word, and were prompted to choose the correct picture. Results show that children with DLD are less accurate at picking the correct referent during the learning phase compared to TD children. A significant main effect of Trial indicates that children get better at picking the right picture during the learning phase. However, there was no evidence for a slower learning trajectory for children with DLD, as the interaction between Trial and Group was not significant. In the test phase, the TD children also significantly outperformed the children with DLD. This was the case for both word form recognition and word–referent link recognition, and there was no evidence for a difference in performance on those two tasks. Moreover, links between vocabulary, attention, phonological working memory and cross-situational word learning were investigated. Vocabulary was the strongest predictor of cross situational word learning. A relative importance analysis, a type of analysis that can be used to determine unique variance in a dependent variable and is suitable when predictors are correlated, indicates that this link is stronger for TD children than for children with DLD. As sustained attention was a significant predictor of the children with DLD's performance in the final learning block, this ability appears to contribute to cross-situational word learning in children with DLD, but please note that sustained attention in itself was not a significant predictor of performance in the relative importance analysis.
The studies of Ahufinger et al. (2021) and McGregor et al. (2022) show that children with DLD indeed have more difficulty with statistical word–referent mapping. As the task of Ahufinger et al. (2021) included an extensive explanation and practice phase before familiarization, we do not yet know how children with DLD perform compared to TD children on implicit cross-situational word learning (see Evans et al., 2009 for a study on implicit word segmentation in children with and without DLD). As Ahufinger et al. state themselves, “(…), these explicit instructions may have triggered a compensatory mechanism (Ullman and Pullman, 2015) to help children with DLD to perform above chance. This hypothesis, however, should be further investigated by assessing the accuracy in this population in a CSSL task with no explicit instructions and no explicit response” (p. 14). Moreover, our study addresses the relationship between cross-situational word learning ability and different measures of lexical-semantic knowledge in children with DLD. McGregor et al. (2022) implemented a behavioral measure of online learning. As we aim to investigate implicit cross situational word learning, measuring eye-tracking is more suitable for our study.
Variability in the learning environment seems to enhance statistical learning: previous research shows that people often learn better on tasks tapping statistical learning when variability is increased in some way. For example, Gómez (2002) tested artificial grammar learning in adults and 18-month old infants. The grammar consisted of non-adjacent dependencies (for example: pel X jic). Participants were significantly better at learning the dependency relation between pel and jic when the intervening element (X) had 24 unique forms, than when X had only 12 different forms. Using the same task, Grunow et al. (2006) found that high variability of the X element also seems to have a positive effect in adults with and without language-based abilities. Other studies also indicate that variability has a positive effect on learning on both individuals with and without language-based disorders, and that more variability results in better generalization of the learned information (Perry et al., 2010; von Koss Torkildsen et al., 2013; Plante et al., 2014; Aguilar et al., 2017; Desmottes et al., 2017). Variability in the learning environment (but not in the to-be-learned target itself) might cause the invariable target or pattern to stand out more and therefore it becomes easier to learn.
Increasing variability in the learning context has also been applied to cross-situational learning tasks, by manipulating the contextual diversity of the to-be-learned word–object pairs. Contextual diversity in this case is defined as “the number of different sets of stimuli with which each word–object pairing co-occurs across learning trials” (Suanda et al., 2014, p. 397). Suanda and Namy (2012) found that greater contextual diversity enhances the learning of word–object mappings in adults: items that occur in more variable contexts (with more different distractor items) are easier to learn than items in a less variable context. Similarly, Suanda et al. (2014) made a comparison between high, moderate and low contextual diversity conditions in a cross-situational learning experiment, and found that contextual diversity enhances cross-situational learning in children of 5–7 years old. In the current research, it is tested whether contextual variability enhances cross-situational word learning differently in TD children than in children with DLD.
2. The current study
Our study aims to shed light on the relationship between cross-situational word learning and lexical semantic knowledge in children with and without DLD. To this end, we investigate implicit cross-situational word learning in 7–9-year-old children with and without DLD, as well as the relation between this ability and various lexical-semantic skills in children with DLD. To investigate cross situational word learning, we use off-line as well as on-line measures. The children's eye movements are measured during the familiarization phase to gain insight of how learning of word–referent pairs unfolds. There has been the need for on-line measures of statistical learning, because off-line measures such as performance on a testing phase are not always a reliable measure of statistical learning ability (Siegelman et al., 2017). However, to the best of our knowledge, previous studies have not looked at the development of looking times toward the target image across trials. We consider this measure as reflecting learning of word–referent pairs during the experiment. We aim to answer the following research questions1:
- RQ1A: do children with DLD have more difficulty than TD children with learning word–referent pairs in an implicit cross-situational word learning task?
- RQ1B: do children with DLD show weaker on-line learning than TD children during implicit cross-situational word learning?
- RQ2: is cross-situational word learning ability related to lexical-semantic skills in children with DLD?
- RQ3A: does higher contextual diversity enhance cross-situational word learning?
- RQ3B: does contextual diversity impact cross-situational word learning differently in TD children than in children with DLD?
We expect to find that children with DLD are less proficient in cross-situational word learning than TD children, which will be reflected by both behavioral and eye-tracking data. Moreover, we expect that cross-situational word learning ability is related to lexical-semantic knowledge in children with DLD. Finally, we expect that contextual diversity enhances learning in both groups of children. We have no hypothesis about a group difference on this enhancing effect of contextual diversity and thus explore whether this is the case. We expect that a potential group difference in sensitivity to contextual diversity could go both ways: if cross-situational word learning is really difficult for children with DLD, contextual diversity would not possibly make much difference for them, while it does for TD children. But it could also be the case that TD children do not show much effect of contextual diversity as cross-situational word learning is not too difficult for them, while children with DLD can utilize it to learn better.
3. Materials and methods
The task of the current study, based on Smith and Yu (2008) and Suanda et al. (2014), amongst others, is designed to measure cross-situational word learning in school-aged children (7–9 years old). Learning is tested off-line (test phase after familiarization) and on-line (eye-tracking during familiarization). Moreover, the influence of contextual diversity on word learning is investigated.
3.1. Participants
Twenty-six children diagnosed with DLD (18 boys and eight girls) between the age of 7; 2 (years; months) and 9; 3 were tested (average: 8; 1). As a control group, we used previously collected data of 26 TD children (15 boys and 11 girls) between 7; 6 and 8; 11 (average: 8; 2).2 The subgroup was selected from a larger sample to match with the DLD group regarding age, gender and the condition of the experiment (contextual diversity). All children had normal or to-normal-corrected vision, and did not have hearing loss or a diagnosis of AD(H)D or ASD. At least one of the caretakers had acquired Dutch as a native language. The TD group did not have any history of language disorders or dyslexia. The Ethical Committee of the Faculty of Humanities of the University of Amsterdam approved the experiment. Caretakers of the children gave written informed consent for their participation.
All children in the DLD group had been previously diagnosed with DLD by a professional speech and language therapist and met the inclusion and exclusion criteria used within the institution from which they were recruited (Pento, Royal Dutch Auris Group, and VierTaal). Using data collected by the institutions, it was checked that all children scored at least 1.5 standard deviations below the age norm on at least two out of four language domains (speech, auditory processing, grammar, vocabulary), measured using standardized tests. Furthermore, their language problems were not secondary to neurological of physiological disorders such as ASD, ADHD, a severe form of dyspraxia, hearing difficulties or genetic syndromes like Down syndrome or 22q11 syndrome.
3.2. Stimuli
Eight novel words and eight novel objects were used to form word–referent pairs. The novel objects were taken from the database of Kachergis et al. (2014), with permission from the authors. All objects were uncommon, difficult to name objects (see Figure 1).

Figure 1. Novel objects used in the experiment (from Kachergis et al., 2014).
The novel words (/dita/, /loχa/, /mıp/, /kasi/, /υεfəl/, /sulεp/, /reχεs/ and /χɔp/) were based on the novel words conducted by Lammertink et al. (2019). All words sounded like Dutch words and were recorded in a neutral manner by a female native speaker of Dutch.
3.3. Design
The familiarization phase consisted of 28 trials, in which eight word–referent pairs could be learned (see Figure 2). A word co-occurred with its referent on seven trials in total. In each trial two word-referent pairs were presented. Each trial in itself was ambiguous in the sense that it was not indicated which of the two words referred to which of the two referents. The position of the objects (left/right) and the order of the words (said first/second) was varied: in half of the trials the first word corresponded to the left object and the second word to the right object (“congruent” trials, named so because the reading direction in Dutch is from left to right), while in the other half of the trials the first word corresponded to the right object and the second word to the left object (“incongruent” trials). Each word consistently appeared with its corresponding referent.
For every participant, words were paired with objects randomly. Thus, for one participant/dita/could refer to the spiral-like object, while for another it could refer to the white round object. The order of the learning trials was pseudo-randomized such that an object could not occur on the same side of the screen two times in a row and a word could not occur as the first/second word two times in a row. Trials lasted 5 s in total, resulting in a familiarization phase of ~2 min and 20 s, but please notice that the exact duration of the learning phase varied between participants, as they could only proceed to the next learning trial if they were looking toward the screen. In every trial, the two objects appeared on the screen 2 s before the first word played. All words had a duration of 1 s, and a 1-s silence was placed between the two words. The trial structure is similar to that used by Smith and Yu (2008), but the time before the onset of the first word and the time between words was extended, so that participants had more time to process the words and the objects. Eye movements were measured during the familiarization phase to measure on-line learning.
Contextual diversity was manipulated between participants. In both conditions, eight word–referent pairs could be learned across trials, but the conditions differed in the variability of the environment in which the word–referent pairs occurred. In each learning trial, two word–referent pairs were presented simultaneously. In the high contextual diversity condition (high-CD), a particular word–referent pair (for example word 1 and picture 1, pair 1–1) occurred with a different word–referent pair each time across trials (with pairs 2–2, 3–3, 4–4, 5–5, 6–6, 7–7, and 8–8). However, in the low contextual diversity condition (low-CD), the accompanying word–referent pairs were sometimes the same. For example, pair 1–1 occurred with pair 2–2 three times, with pair 3–3 three times, and with 4–4 once. Thus, in the low-CD condition there was less diversity across trials. See Table 2 for the combinations of word–referent pairs in the two familiarization conditions.
In the test phase, all eight word–referent pairs were tested once. Participants heard a word three times and had to choose between four objects which was the correct one. The same audio files as in the familiarization phase were used. In the high-CD condition, three random objects are chosen as foils. All these foils had occurred with the word equally often (once). In the low-CD condition, the three foils that had occurred with the word are chosen as foils. Two of the foils had occurred with the word three times, and one foil had occurred with the word once (see Table 1).
3.4. Apparatus
The experiment ran in E-Prime 3.0 (Psychology Software Tools Inc, 2016) on a Windows laptop computer with a 17-inch monitor. Eye movements of the participants were measured with a Tobii Pro X2-120 mobile eye-tracker which was attached to a laptop. Gaze data were recorded at 120 Hz (120 samples per second).
3.5. Background measures
The cross-situational word learning task was part of a larger test battery. A number of background measures that tap into different types of linguistic skills and other cognitive skills were administered to the children with DLD (please see Appendix for more information).
3.5.1. Language measures
In the language domain we tested different types of lexical skills and morphosyntactic skills using subtests of the CELF-4-NL (Clinical Evaluation of Language Fundamentals: Core Language Scales, Dutch version; Semel et al., 2010) and the Peabody Picture Vocabulary Task-III-NL (PPVT; Schlichting, 2005). Regarding the lexical skills, we used the Expressive Vocabulary task to measure expressive vocabulary, the Word Classes task (part 1 or 2, depending on the age of the participant) to measure the ability to express relationships between words, the Word Associations task to measure the ability of recalling words in a certain semantic category (all three subtasks of the CELF-4-NL), and the PPVT to measure passive vocabulary size. Moreover, morphosyntactic knowledge was measured using the Sentence Recall subtest from the CELF-4-NL, and the non-word repetition task (Rispens and Baker, 2012) was administered to test verbal short-term memory.
3.5.2. Cognitive measures
We administered the Raven Progressive Matrices (Raven et al., 2003) to measure non-verbal intelligence. Auditory short-term memory (Number Repetition 1 from the CELF-4-NL, digit span task) and working memory (Number Repetition 2, from the CELF-4-NL, digit span backwards task) were administered as well.
3.6. Procedure
For every participant, the experiment consisted of a calibration phase, a familiarization phase and a test phase. Participants sat behind the computer screen. The calibration procedure was run with E Prime. As a first part of the calibration, it was checked whether participants' gaze was in the center of the screen, and if necessary the position of the laptop was adjusted. The calibration procedure included nine fixation points and took ~2 min. After calibration, the task was explained to the participants. A cute alien was shown on the screen and pre-recorded child-directed instructions were played. Participants were instructed to look carefully at the screen and listen carefully to the words, and they were told that there would be some questions at the end of the experiment. Thus, it was not explicitly explained that they should learn word–referent pairs.
Participants were then exposed to either the high-CD or the low-CD familiarization condition. Every learning trial started with a fixation cross (a + in the middle of the screen). Participants automatically proceeded to the learning trial if they looked at the cross for 200 consecutive milliseconds (24 samples). A cover task was added to the familiarization phase to make sure the participants kept paying attention. The same alien that gave them instructions appeared jumping on the screen at five random moments between trials in the familiarization phase. Participants were told to click on the alien as quickly as possible when they saw it.
After familiarization, participants did a test phase, during which all eight word–referent pairs were tested once. The test phase started with a practice item: the word hond (“dog”) was played and participants could choose between a picture of a dog, cat, a tree and a couch. The experimenter was allowed to provide feedback during this practice phase. There was no feedback during the actual test phase. As stated earlier, the cross-situational word learning task was part of a larger test battery.
Apart from the background measures, participants also did two other statistical learning tasks that are not discussed in this paper (see Broedelet et al., 2023; submitted). The order of the tasks was counterbalanced across participants.
3.7. Data processing
For the off-line test phase, the practice item was removed for further analysis. For every answer it was coded whether it was correct or incorrect.
The eye-tracking data were interpolated before analysis using a Praat (Boersma and Weenink, 2019) script. When at least one but at most nine consecutive samples (75 ms) in a row lacked eye-gaze information, the position of the eye in these missing samples was filled in by linear interpolation. The value of 75 ms as a maximum for a gap to be interpolated reflects a recommendation in the official Tobii manual (Tobii Pro AB, 2014). This value is chosen because it corresponds to the duration of a blink. 6.7% of the data was interpolated in this way.
After interpolation, we constructed two 1,000-ms time windows. As it takes ~200 ms to plan an eye movement in reaction to a spoken word (Viviani, 1990), time window 1 started 200 ms after the onset of the first word. Time window 2 started 200 ms after the onset of the second word. Data points from the fixation parts of the learning trials and when the pictures were shown but the words had not yet started were removed from the data.
Two Areas of Interest (AOI's) were defined, corresponding to the two pictures that were shown on the left side and the right side of the screen during the familiarization trials. For every sample it was computed whether the participant looked at the left picture or the right picture. Trials in which more than 50% of the samples were missing (no eye-gaze data) or irrelevant (looks at the screen but not at one of the two pictures) were removed from the data (433 trials). Then, we removed all remaining missing data (31,835 samples), leaving 210,925 samples for analysis. Unfortunately, the DLD group had more missing data than the TD group (84,423 DLD; 27,891 TD), which caused an imbalance in the remaining data: 139,612 samples for the TD group and 71,313 samples for the DLD group. On average, each participant contributed data from 19.6 trials.
3.8. Analysis
We used the packages lme4 (Bates et al., 2015) and eyetrackingR (Dink and Fergusson, 2015) from the free software R (R Core Team, 2020) for data analysis. For each sample of the eye-tracking data, it was computed whether the participant looked at the target picture or the distractor picture, which depended on the word that was played at that moment. Using the eyetrackingR package, the samples were binned into 50-ms time bins. For each time bin, the proportion of looks toward the target picture was computed by dividing the number of looks toward the target by the total number of looks toward the pictures. The dependent variable was then transformed using an adjusted logit transformation.3 In this transformed variable, a value of 0 means that a participant is looking equally often at both pictures while a positive value means s/he looks more toward the target picture. In our statistical analysis, we computed whether the proportion of looks toward the target picture depended on Group (TD/DLD), Condition (high-CD/low-CD) and Trial (1–28), keeping into account the factors Time within a trial, Age and Congruency (congruent vs. incongruent trials). To this end, we set up two linear mixed-effects models.
4. Results
4.1. Cross-situational word learning: Accuracy
All analyses were done in R. For the off-line data, the answers to all eight test items in the test phase were taken into account. There was no missing data. Using the package lme4, we constructed a generalized linear mixed-effects model. Accuracy was the dependent variable, Group and Condition were between-participant predictors. Age was included as a between-participant control variable. Group and Condition were binary predictors and were coded with orthogonal contrasts: Group was coded as –½ for DLD and + ½ for TD, Condition was coded as –½ for low-CD and + ½ for high-CD. The predictor Age was centered and scaled. The maximal model that included the main predictors and the interaction between them, random intercepts for Subject and Item as well as by-Item random slopes for Group, Condition and Age and all interactions between them resulted in a singular fit. Therefore we took out the by-item random slopes,4 resulting in the following model: Accuracy ~ Group * Condition * Age + (1 | Subject) + (1 | Item).
For answering research question 1A (“do children with DLD have more difficulty than TD children with learning word–referent pairs in an implicit cross-situational word learning task?”), the relevant effect is the main effect of Group: we expected that children with DLD learn fewer word–referent pairs on this test than TD children. For research questions 3A (“does higher contextual diversity enhance cross-situational word learning?”) and 3B (“does contextual diversity impact cross-situational word learning differently in TD children than in children with DLD?”), the relevant effects are the main effect of Condition and the interaction between Condition and Group, respectively: we expected more accurate responses for children in the high-CD condition compared to the low-CD condition. A significant interaction between Group and Condition would indicate that the Condition effect differed between the groups (we had no expectation about the existence or direction of such an interaction).
Our model estimates that TD children are 3.71 (95% CI: 1.73–7.98) times more likely to answer an item on the test phase correctly than children with DLD: z = 3.63, p = 0.0008. Moreover, our model estimates that children in the high-CD condition score 1.67 (95% CI: 0.79–3.54) times higher in the test phase than children in the low-CD condition, but this difference is not significant: z = 1.346, p = 0.18. Although the positive effect of contextual variability in the high-CD Condition was 1.1 (95% CI: 0.25–4.94) times stronger in the children with DLD than the TD children, this interaction between Group and Condition was not significant: z = −0.136, p = 0.89. To determine whether both groups scored higher than could be expected from chance, we also compared the performance of both groups to chance level (which was 0.25 as there were four possible answers on every test item). For the TD children, the estimation of the intercept, converted from log-odds to probabilities, was 0.83 (95% CI: 0.65–0.94). For the DLD children, the estimate of the intercept (converted from log-odds to probabilities) was 0.49 (95% CI: 0.39–0.60). For both groups, as the confidence intervals of the intercept do not include 0.25, this estimation was significantly higher than chance level. Thus, although both groups of children learned word–referent pairs in the experiment, as indicated by the above-chance performances, the children with DLD were significantly outperformed by the TD children, indicating that children with DLD have more difficulty with cross-situational word learning than TD children. We did not find evidence for or against an effect of contextual diversity on learning word–referent pairs. See Table 2 for the mean accuracy data, and Figures 3, 4 for a visual representation of the data.
4.2. Cross-situational word learning: Eye-tracking data
We conducted two separate linear mixed-effects models for the two time windows (Word1 and Word2) to test whether the proportion of looks toward the target picture was different for children with and without DLD and whether there was an influence of Condition and Trial, taking into account the variables Time, Age and Congruency. The dependent variable was the adjusted logit transformation of the proportion of looks toward the target picture of every 50-ms time bin. Between-participant variables were Group and Condition, within-participant variables were Trial, Time, Age, and Congruency. Before conducting a linear mixed-effects model, all binary variables were coded with sum-to-zero orthogonal contrasts (Group, Condition, and Congruency) and all numeric variables were centered and scaled (Trial, Time, and Age).
The model included all predictors and the interactions between them (except for Age), as well as random intercepts for Participant and Item. Also included were by-item random slopes (and the interactions between them) for Group and Condition as well as by-subject random slopes (and the interactions between them) for Time, Trial and Congruency. This resulted in the following model: Logit ~ Group * Condition * Time * Trial * Congruency + Age + (Group * Condition | Item) + (Time * Trial * Congruency | Participant). We are interested in the main effect of Group, Condition and Trial, as well as the interactions between Group and Condition and Group and Trial. A significant effect of Trial in the expected direction would show that children look more toward the target image as the experiment unfolds, what we interpret as an on-line learning effect. An interaction between Group and Trial would show that this on-line learning effect is different for the two groups. We are also interested whether the intercepts of the models are significant, which would indicate that children in general look more toward the target image than the distractor image.
4.2.1. Sanity checks and confirmatory results
In this section, we first discuss some sanity checks, and then the confirmatory results. We assume a significance criterium of p = 0.01. As a first sanity check, we computed whether the intercept is significantly higher than zero, which would mean that participants look more toward the target picture compared to the distractor picture. As a second sanity check, we computed whether Trial significantly influenced the proportion of looks toward the target picture, which would mean that participants look more toward the target picture as the experiment progressed (on-line learning effect). To answer our research questions 1B (“do children with DLD show weaker on-line learning than TD children during implicit cross-situational word learning?”), 3A (“does higher contextual diversity enhance cross-situational word learning?”) and 3B (“does contextual diversity impact cross-situational word learning differently in TD children than in children with DLD?”) we look at the effects of Group, the interaction between Group and Trial, the effect of Condition and the interaction between Group and Condition respectively.
4.2.1.1. Word 1
See Table 3 for the outcomes of the model for Word1. None of the relevant effects are significant, meaning there is no evidence that children look more toward the target picture than the distractor in general (Intercept) and whether the proportion of looks toward the target increases as the familiarization phase progresses (effect of Trial). As we do not find significant results for the sanity checks, we do not have clear evidence that the eye gaze patterns of our participants reflect on-line learning of word–referent pairs. We cannot answer our research questions, as the effects of Group, Condition, nor the interactions between Group and Trial and Group and Condition are significant. See Figure 5 for a plot depicting the model predictions and actual data of the proportion of looks toward the target picture in the first time window (Word1) for the children with and without DLD, and see Figure 6 for the effect of Trial across groups. A plot depicting individual differences can be found in the Supplementary material.
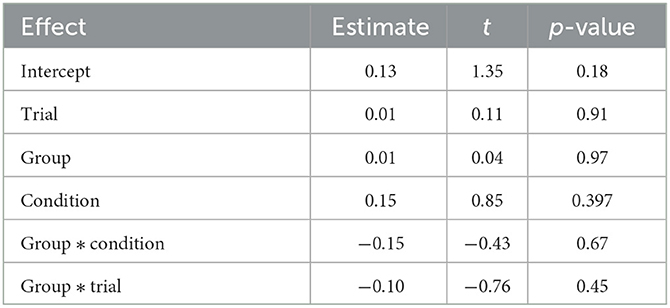
Table 3. Outcomes of the linear mixed-effects model for Word1: sanity checks and confirmatory results.
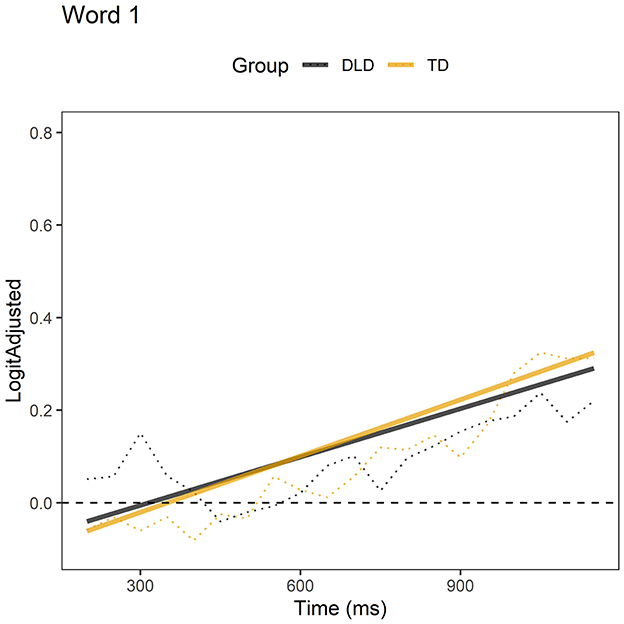
Figure 5. Graph depicting looks towards the target picture per Group for Word1. The dotted line represents the actual data, the solid line represents the prediction of the statistical model.
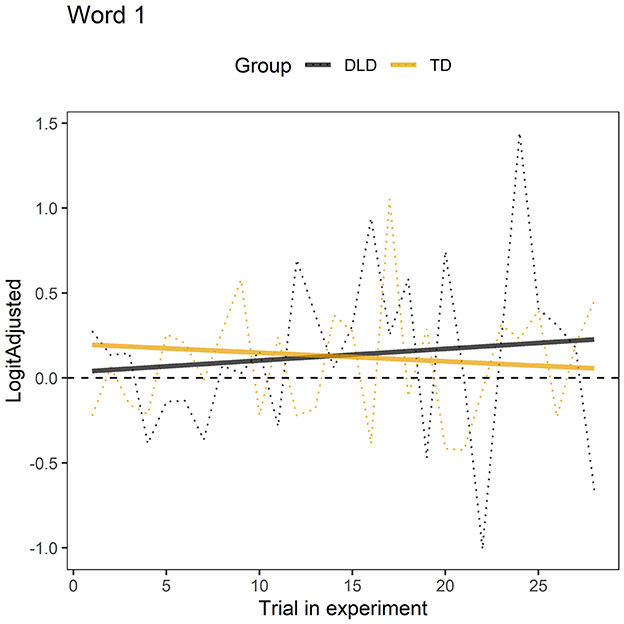
Figure 6. Graph depicting looks towards the target picture per Group and Trial for Word1. The dotted line represents the actual data, the solid line represents the prediction of the statistical model.
4.2.1.2. Word 2
See Table 4 for the outcomes of the model for Word2. The estimate for the intercept is 0.55 logit, which is significantly different from zero (t = 4.73, p = 0.00001), indicating that children on average look more toward the target picture than the distractor picture. The other relevant effects are not significant, although we might mention that the main effect of Group and the interaction between Group and Trial approach significance. Thus, we cannot answer our research questions about the effect of group or contextual variability on implicit cross-situational learning ability. See Figure 7 for a plot depicting the model predictions and actual data of the proportion of looks toward the target picture for the children with and without DLD and see Figure 8 for the effect of Trial across groups. A plot depicting individual differences can be found in the Supplementary material.
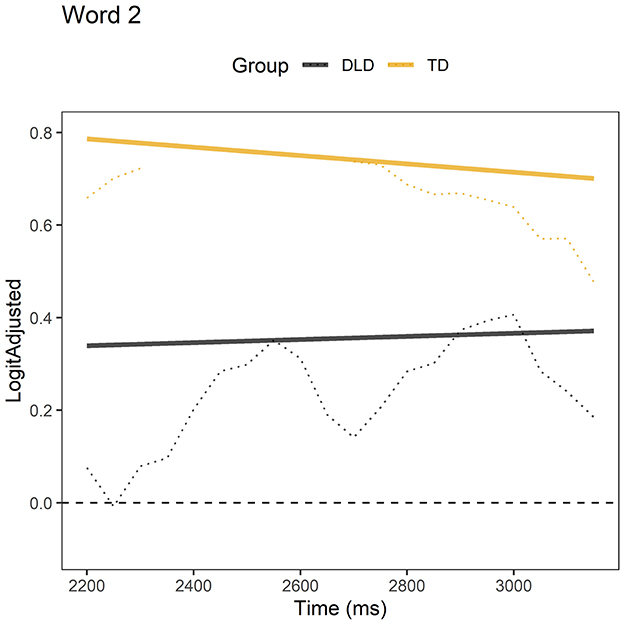
Figure 7. Graph depicting looks towards the target picture per Group for Word2. The dotted line represents the actual data, the solid line represents the prediction of the statistical model.
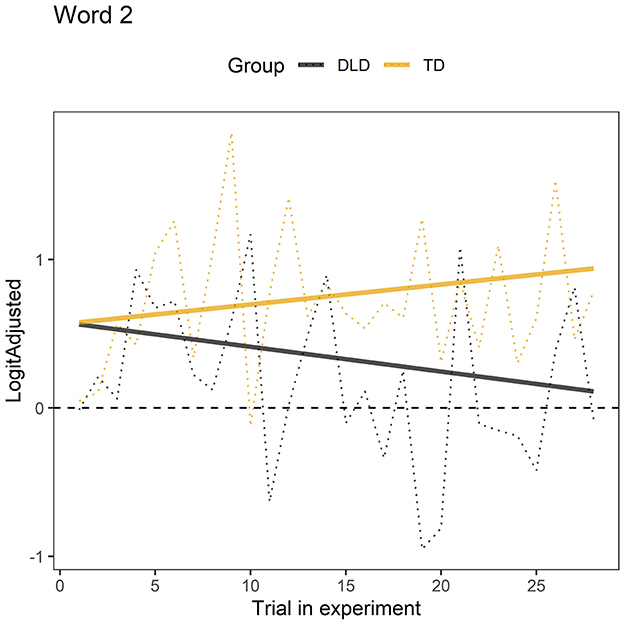
Figure 8. Graph depicting looks towards the target picture per Group and Trial for Word2. The dotted line represents the actual data, the solid line represents the prediction of the statistical model.
4.2.2. Exploratory results
As an exploratory analysis, we looked at the effects of Time (within trials) and Congruency (congruent/incongruent with reading order) on the proportion of looks toward the target. Word1. The children looked more toward the correct image later in a learning trial (i.e. as they have heard more of the word): estimate: 0.11, t = 2.30, p = 0.03. Moreover, children looked more toward the target picture when trials were congruent (first word refers to left image and second word to right image) than when they were incongruent (first word refers to right image and second word to left image): estimate: 0.40, t = 2.49, p = 0.015. Word2. For Word2, the effect of Time was small or non-existent (95% CI: −0.10 to −0.08, t = −0.18, p = 0.86). Again, children's looking times were influenced by Congruency: estimate: 0.49, t = 2.22, p = 0.03. It could be the case that time within a trial and the 577 order of the words and pictures influences looking behavior.
4.3. Regression analyses
To answer research question 2 (“is cross-situational word learning ability related to lexical-semantic skills in children with DLD?”), we performed regression analyses to investigate the relationship between cross-situational word learning ability on the one hand and existing lexical-semantic knowledge on the other hand. Dependent variables were the different measures of lexical-semantic knowledge, predictor variables were off-line and on-line measures of cross-situational word learning and several control measures of cognitive abilities, as well as age and SES. All variables were centered and scaled before analysis.
4.3.1. Principal component analysis
We constructed a principal component analysis (PCA) in R on the measures of non-verbal intelligence, digit span forwards, digit span backwards and non-word repetition to reduce the number of predictor variables. This resulted in four component scores, of which we decided to use the first three as they together explained 95% of the variance. See Table 5 for the standardized loadings of the component scores after varimax rotation. The scores represent phonological processing (digit span forwards, non-word repetition), non-verbal intelligence (Raven) and verbal working memory (digit span backwards) and were saved and used for as predictor scores in further analyses. We computed the correlations between the predictor variables (see Supplementary material).
We constructed four separate linear regression models for the four dependent variables, which are discussed one by one below. CSWL off-line represent the average accuracy on the test phase of the CSWL task, while CSWL on-line represents the mean proportion of looks toward the target picture during the familiarization phase of the CSWL task.
4.3.2. Passive vocabulary
The linear model with passive vocabulary as the dependent variable as a whole was not significant (F = 0.6801, p = 0.69, adjusted R2 = −0.098), meaning that the full model did not predict the dependent variable better than a null model without any predictors. The contributions of the individual predictors can be found in the Supplementary material. None of the predictors contributed significantly to variance in passive vocabulary size in the children with DLD.
4.3.3. Active vocabulary
The linear model with active vocabulary as the dependent variable as a whole was not significant (F = 1.196, p = 0.35, adjusted R2 = 0.05). The contributions of the individual predictors can be found in the Supplementary material. None of the predictors significantly contributed to the children's active vocabulary score.
4.3.4. Word categories
The linear model with word categories score are the dependent variable as a whole was not significant (F = 1.827, p = 0.14, adjusted R2 = 0.19). The contributions of the individual predictors can be found in the Supplementary material. None of the predictors significantly contributed to the children's word categories score.
4.3.5. Word associations
The linear model with word associations score as the dependent variable as a whole was not significant (F = 0.799, p = 0.59, adjusted R2 = −0.06). The contributions of the individual predictors can be found in the Supplementary material. None of the predictors significantly contributed to variance in word associations score in the children with DLD.
5. Discussion
The current study aimed to investigate implicit cross-situational word learning in children with and without DLD and its relation to lexical-semantic knowledge. We will discuss the results per research question in the sections below.
5.1. RQ1: Are children with DLD less proficient in cross-situational word learning?
Results from the analysis of the off-line test phase show that both our groups were able to pick up the mappings between novel objects and novel pictures, while they had not received instructions to do so. This indicates that children with and without DLD can use statistical learning mechanisms to link words and referents implicitly. However, as our children with DLD performed significantly lower than our TD children (p = 0.0008), we can conclude that children with DLD likely are not able to profit from statistical learning to the same extent as children without DLD do. These results are in line with the findings of Ahufinger et al. (2021) and McGregor et al. (2022). The latter also report above-chance performance for both TD children and children with DLD, but poorer performance in the last group in a more explicit learning condition. Our study extends this finding to implicit cross-situational word learning.
We also aimed to measure the process of learning using eye-tracking. We expected that children would start to look more toward the target image as the experiment progressed, reflecting learning of word–referent pairs during the exposure phase of the experiment. Moreover, we expected to find group differences in looking behavior. One finding seems to reflect on-line learning: the intercept for the model of the second word in a learning trial was significant, showing that children have a preference for the target picture as opposed to the distractor picture, corresponding to the finding of above chance on the off-line test phase. This is an extension of the eye-tracking results of Ahufinger et al. (2021), who did not report any evidence for a preference for the target image. However, the remaining predictors did not significantly influence looking behavior. The effect of Trial was not significant for the first word or the second word, meaning we have no evidence for an on-line learning effect across trials. Since neither the main effect of Group nor the interaction between Group and Trial was significant, we have no evidence that children with DLD look less often toward the target picture in general or that they show less strong on-line learning. Exploratory analyses might indicate that time within a trial and the congruency of the order of the words and pictures (congruent with reading order or incongruent) influenced the proportion of looks toward the target, but we cannot draw any conclusions from exploratory findings.
As can be seen in the Supplementary material, the amount of individual variation is large, especially within the DLD group. Moreover, as discussed in section 3.7, the contribution of data points between the two groups is highly skewed: the TD children provided many more data points than the children with DLD. In the Supplementary material, graphs are provided that show the number of data points per group, split up for the predictors Time, Condition, Congruency and Trial. Besides the overall imbalance between the groups, data for the predictors Condition, Congruency and Trial are also skewed for the DLD group. While for the TD children the data is roughly equally divided, for the children with DLD there is more data for the low-CD condition, for the incongruent trials and the earlier trials in the experiment than there is for their counterparts. These imbalances are caused by the large number of missing data in our DLD group, but also relatively many “irrelevant” looks (looks at the screen but outside the AOIs; 39,677 samples in the DLD data vs. 12,461 samples in the TD data). It could be the case that the eye-tracker worked less efficiently for these children, but is likely that they looked less well at the screen overall. This could be related to attention difficulties, which have been established in children with DLD (for a review, see Smolak et al., 2020), and McGregor et al. (2022) report that sustained attention predicts cross-situational word learning ability in children with DLD. It is possible that weaker attentional ability in children with DLD contributed to poorer learning of word-referent pairs as measured with the off-line test phase. The skewness of the data and the large individual variation possibly weakened statistical power, which could partly explain the absence of significant effects in the eye-tracking analysis. Future studies should aim to test larger groups of participants.
One could argue that learning could have faltered at the level of phonology for the children with DLD. As children with DLD are shown to have difficulty with phonological short-term memory and seem to store less specified phonological representations (Mainela-Arnold et al., 2010), it might be hard for them to disentangle the new words in their memory, resulting in poorer learning. To reduce the chance that children would confuse the words, we chose to have more variation in phonological structure then is often implemented: the words in our experiment have different (simple) phonological structures (CVC, CVCV, and CVCVC) and every word starts with a different consonant. Still, as Bogaerts et al. (2021) argue, it would be fruitful for future studies to set up experiments that can show a contrast between impaired statistical learning and intact performance on a task that does not entail statistical learning.
5.2. RQ2: Is cross-situational word learning ability related to lexical-semantic skills in children with DLD?
We expected to find that cross-situational word learning ability significantly contributes to lexical semantic knowledge in children with DLD. Besides segmenting words from running speech, tracking the co-occurrences between auditory words and visual referents contributes to gaining lexical-semantic knowledge. For children with DLD, it might be the case that this type of implicit word learning works less efficiently and hampers lexical-semantic development. However, as none of the multiple linear regression models we conducted was significant, we cannot conclude anything about the relation between implicit cross-situational word learning and existing lexical-semantic knowledge in children with DLD, nor the influence of age, SES, phonological processing, non-verbal intelligence and verbal working memory. Future studies, besides testing larger participant groups, could investigate this relationship by setting up longitudinal experiments.
Previous work has shown a relationship between cross-situational word learning ability and vocabulary size in young TD children (22–66 months; Vlach and DeBrock, 2017). Kemény and Lukács (2021) report a significant independent contribution of probabilistic statistical learning ability (weather prediction task) to vocabulary size in TD children, while short-term memory did not independently contribute to vocabulary. However, in their children with DLD, this pattern was reversed: short-term memory independently contributed to vocabulary size, but statistical learning ability did not. The authors interpret the results as indicating that different cognitive abilities underlie lexical development in TD children and children with DLD, although it is important to note that this interpretation is based on a p-value comparison. McGregor et al. (2022) report that vocabulary is a predictor of cross-situational word learning ability, and that this relationship is stronger in TD children compared to children with DLD, based on a relative importance analysis. It could be the case that children with DLD compensate for less efficient statistical learning mechanisms by depending more on, for example, declarative learning, which might explain why we did not find a significant relationship between cross-situational word learning and lexical-semantic knowledge in our group of children with DLD. Unfortunately, we were not able to compare the contribution of cross-situational word learning to vocabulary between children with and without DLD, as the TD children in our experiment were not tested on lexical-semantic skills.
5.3. RQ3: Does high contextual diversity enhance cross-situational word learning?
We manipulated contextual diversity between subjects to investigate whether variability in the learning environment would affect cross-situational word learning in children with and without DLD. Although performance was higher in the condition with higher contextual diversity on average, there was no significant effect of condition, nor a significant interaction between condition and group, and thus we cannot answer the question whether variability in the learning environment influences cross-situational word learning in children with and without DLD. The eye-tracking data also did not reveal evidence for a difference in on-line learning for the two conditions.
5.4. Concluding remarks and future research
Our study shows that children with DLD are less proficient when learning word meanings based on 721 cross-situational statistics in an implicit task. If utilizing contexts with different amounts of referential uncertainty by implicitly tracking co-occurrences between words and visual referents works less efficiently in children with DLD, this could hamper the acquisition of vocabulary. Although the relationship between cross-situational word learning and existing lexical knowledge requires more investigation, our study contributes to our knowledge of different types of statistical learning in children with DLD. The cross-situational word learning paradigm aims to mimic real-life situations with referential uncertainty. However, it is far from realistic. Zhang et al. (2021) investigated naturalistic cross-situational word learning in children who are playing with toys. Future research could compare this naturalistic cross-situational word learning between children with and without DLD.
Data availability statement
The datasets presented in this study can be found in online repositories. The names of the repository/repositories and accession number(s) can be found at: https://doi.org/10.21942/uva.c.6152406.
Ethics statement
The studies involving human participants were reviewed and approved by Ethics Committee of the Faculty of Humanities, University of Amsterdam. Written informed consent to participate in this study was provided by the participants' legal guardian/next of kin.
Author contributions
IB, JR, and PB designed the experiment. IB recruited the participants, collected the data, and wrote the first draft of the manuscript. IB and PB analyzed the data. JR and PB provided feedback and contributed to the manuscript. All authors contributed to the article and approved the submitted version.
Funding
This work was supported by NWO (Netherlands Organization for Scientific Research) under Grant 322-89-014.
Acknowledgments
We would like to sincerely thank all children who participated and their caretakers. Also many thanks to the speech therapists and teachers from VierTaal, The Royal Auris Group and Pento for their extensive help on facilitating children's participation, as well as the staff members from the primary schools that participated in our research. Finally, we would like to thank our test-assistant Maayke Sterk and our lab technician Dirk Jan Vet.
Conflict of interest
The authors declare that the research was conducted in the absence of any commercial or financial relationships that could be construed as a potential conflict of interest.
Publisher's note
All claims expressed in this article are solely those of the authors and do not necessarily represent those of their affiliated organizations, or those of the publisher, the editors and the reviewers. Any product that may be evaluated in this article, or claim that may be made by its manufacturer, is not guaranteed or endorsed by the publisher.
Supplementary material
The Supplementary Material for this article can be found online at: https://www.frontiersin.org/articles/10.3389/fcomm.2023.1021654/full#supplementary-material
Footnotes
1. ^Since we posit multiple research questions, we adjust the significance criterion to p = 0.01 as opposed to the conventional p = 0.05.
2. ^We had planned to test an age-matched group of TD children. Unfortunately, we were unable to administer the tests as all primary schools in the Netherlands were closed from March to June 2020 due to the outbreak of COVID-19. After the reopening of the schools many restrictions still applied, making it impossible to enter schools for testing participants. We therefore decided to use a subset of an already collected pilot data as control data. No articles based on this data have been published. As a result of this, the control group, unlike the DLD group, was not tested on the background tasks measuring vocabulary, morphosyntactic skills, phonological processing and non-verbal intelligence. This means the control group could unfortunately not be matched on vocabulary skills to the DLD group.
3. ^The eyetrackingR package uses the formula Logit = log [Prop/(1 – Prop)], where Prop represents the proportion of looks towards the target image. When the value of Prop equals 0 or 1, a small value (0.1) is added: Logit = log[(Prop + 0.1)/(1 – Prop + 0.1)].
4. ^This means that for the three predictors, we will not be able to generalize from our eight specific items to a hypothetical infinite population of possible items. This means that discarding the by-item random slopes yields new interpretations for p-values for the between-participants effects (group, condition, and age); note, though, that the false positive rate for generalizing to a hypothetical infinite population of possible participants is not affected.
References
Aguilar, J. M., and Plante, E. (2014). Learning of grammar-like visual sequences by adults with and without language-learning disabilities. J. Speech Lang. Hear. Res. 57, 1394–1404. doi: 10.1044/2014_JSLHR-L-13-0124
Aguilar, J. M., Plante, E., and Sandoval, M. (2017). Exemplar variability facilitates retention of word learning by children with specific language impairment. Lang. Speech Hear. Serv. Sch. 49, 72–84. doi: 10.1044/2017_LSHSS-17-0031
Ahufinger, N., Guerra, E., Ferinu, L., Andreu, L., and Sanz-Torrent, M. (2021). Cross-situational statistical learning in children with developmental language disorder. Lang. Cogn. Neurosci. 36, 1180–1200. doi: 10.1080/23273798.2021.1922723
Alt, M., and Plante, E. (2006). Factors that influence lexical and semantic fast mapping of young children with specific language impairment. J. Speech Lang. Hear. Res. 49, 941–954. doi: 10.1044/1092-4388(2006/068)
Alt, M., Plante, E., and Creusere, M. (2004). Semantic features in fast-mapping: performance of preschoolers with specific language impairment versus preschoolers with normal language. J. Speech Lang. Hear. Res. 47, 407–420. doi: 10.1044/1092-4388(2004/033)
Baldwin, D. A. (1991). Infants' contribution to the achievement of joint reference. Child Dev. 62, 875–890. doi: 10.1111/j.1467-8624.1991.tb01577.x
Bates, D., Mächler, M., Bolker, B. M., and Walker, S. C. (2015). Fitting linear mixed-effects models using lme4. J. Stat. Softw. 67, 1–48. doi: 10.18637/jss.v067.i01
Blythe, R. A., Smith, K., and Smith, A. D. M. (2010). Learning times for large lexicons through cross-situational learning. Cogn. Sci. 34, 620–642. doi: 10.1111/j.1551-6709.2009.01089.x
Boersma, P., and Weenink, D. (2019). Praat: Doing Phonetics by Computer (6.1.05). Available online at: https://www.praat.org/ (accessed September, 2019).
Bogaerts, L., Siegelman, N., and Frost, R. (2021). Statistical learning and language impairments: toward more precise theoretical accounts. Perspect. Psychol. Sci. 16, 319–337. doi: 10.1177/1745691620953082
Brackenbury, T., and Pye, C. (2005). Semantic deficits in children with language impairments: issues for clinical assessment. Lang. Speech Hear. Serv. Sch. 36, 5–16. doi: 10.1044/0161-1461(2005/002)
Broedelet, I., Boersma, P., and Rispens, J. (2023). Distributional learning of novel visual object categories in children with and without developmental language disorder. Lang. Develop. Res. 2, 1–43. doi: 10.34842/2023.0528
Carey, S. (1978). “The child as word learner,” in Linguistic Theory and Psychological Reality, eds M. Halle, J. Bresnan, and A. Miller (Cambridge, MA: MIT Press), 264–293.
Chung, H., and Yim, D. (2020). Quick incidental learning of words by children with and without specific language impairment: an eye-tracking study. Commun. Sci. Disord. 25, 499–516. doi: 10.12963/csd.20715
Conway, C. M., Bauernschmidt, A., Huang, S. S., and Pisoni, D. B. (2010). Implicit statistical learning in language processing: word predictability is the key. Cognition 114, 356–371. doi: 10.1016/j.cognition.2009.10.009
Desmottes, L., Maillart, C., and Meulemans, T. (2017). Mirror-drawing skill in children with specific language impairment: improving generalization by incorporating variability into the practice session. Child Neuropsychol. 23, 463–482. doi: 10.1080/09297049.2016.1170797
Dink, J., and Fergusson, B. (2015). eyetrackingR: an R Library for Eye-Tracking Data Analysis. Available online at: http://www.eyetrackingr.com (accessed August, 2022).
Dockrell, J. E., Messer, D., and George, R. (2001). Patterns of naming objects and actions in children with word finding difficulties. Lang. Cogn. Process. 16, 261–286. doi: 10.1080/01690960042000030
Dockrell, J. E., Messer, D., George, R., and Ralli, A. (2003). Beyond naming patterns in children with WFDs-definitions for nouns and verbs. J. Neurolinguistics 16, 191–211. doi: 10.1016/S0911-6044(02)00012-X
Drljan, B., and Vuković, M. (2019). Comparison of lexical-semantic processing in children with developmental language disorder and typically developing peers. GOVOR 36, 119–138. doi: 10.22210/govor.2019.36.07
Ellis, E. M., Gonzalez, M. R., and Deák, G. O. (2014). Visual prediction in infancy: what is the association with later vocabulary? Lang. Learn. Dev. 10, 36–50. doi: 10.1080/15475441.2013.799988
Evans, J. L., Saffran, J. R., and Robe-Torres, K. (2009). Statistical learning in children with specific language impairment. J. Speech Lang. Hear. Res. 52, 321–335. doi: 10.1044/1092-4388(2009/07-0189)
Fitneva, S. A., and Christiansen, M. H. (2011). Looking in the wrong direction correlates with more accurate word learning. Cogn. Sci. 35, 367–380. doi: 10.1111/j.1551-6709.2010.01156.x
Gleitman, L. R., Cassidy, K., Nappa, R., Papafragou John, A., and Trueswell, C. C. (2005). Hard words. Lang. Learn. Dev. 1, 23–64. doi: 10.1207/s15473341lld0101_4
Gómez, R. L. (2002). Variability and detection of inviariant structure. Psychol. Sci. 13, 431–436. doi: 10.1111/1467-9280.00476
Gordon, J., Schumm, J. S., Coffland, C., and Doucette, M. (1992). Effects of inconsiderate versus considerate text on elementary students' vocabulary learning. Read. Psychol. 13, 157–169. doi: 10.1080/027027192130204
Graf Estes, K. (2009). From tracking statistics to learning words: statistical learning and lexical acquisition. Lang. Linguist. Compass 3, 1379–1389. doi: 10.1111/j.1749-818X.2009.00164.x
Gray, S. (2004). Word learning by preschoolers with specific language impairment: predictors and poor learners. J. Speech Lang. Hear. Res. 47, 1117–1132. doi: 10.1044/1092-4388(2004/083)
Grunow, H., Spaulding, T. J., Gómez, R. L., and Plante, E. (2006). The effects of variation on learning word order rules by adults with and without language-based learning disabilities. J. Commun. Disord. 39, 158–170. doi: 10.1016/j.jcomdis.2005.11.004
Haebig, E., Saffran, J. R., and Ellis Weismer, S. (2017). Statistical word learning in children with autism spectrum disorder and specific language impairment. J. Child Psychol. Psychiatry 58, 1251–1263. doi: 10.1111/jcpp.12734
Hamrick, P., Lum, J. A. G., and Ullman, M. T. (2018). Child first language and adult second language are both tied to general-purpose learning systems. Proc. Natl. Acad. Sci. U. S. A. 115, 1487–1492. doi: 10.1073/pnas.1713975115
Hedenius, M., Persson, J., Tremblay, A., Adi-Japha, E., Veríssimo, J., Dye, C. D., et al. (2011). Grammar predicts procedural learning and consolidation deficits in children with specific language impairment. Res. Dev. Disabil. 32, 2362–2375. doi: 10.1016/j.ridd.2011.07.026
Horst, J. S., and Samuelson, L. K. (2008). Fast mapping but poor retention by 24-month-old infants. Infancy 13, 128–157. doi: 10.1080/15250000701795598
Hsu, H. J., and Bishop, D. V. (2014). Sequence-specific procedural learning deficits in children with specific language impairment. Dev. Sci. 17, 352–365. doi: 10.1111/desc.12125
Hsu, H. J., Tomblin, J. B., and Christiansen, M. H. (2014). Impaired statistical learning of non-adjacent dependencies in adolescents with specific language impairment. Front. Psychol. 5, 175. doi: 10.3389/fpsyg.2014.00175
Jackson, E., Leitao, S., Claessen, M., and Boyes, M. (2019a). Fast mapping short and long words: examining the influence of phonological short-term memory and receptive vocabulary in children with developmental language disorder. J. Commun. Disord. 79, 11–23. doi: 10.1016/j.jcomdis.2019.02.001
Jackson, E., Leitão, S., Claessen, M., and Boyes, M. (2019b). The evaluation of word-learning abilities in people with developmental language disorder: a scoping review. Int. J. Lang. Commun. Disord. 54, 742–755. doi: 10.1111/1460-6984.12490
Kachergis, G., Yu, C., and Shiffrin, R. M. (2014). Cross-situational word learning is both implicit and strategic. Front. Psychol. 5, 588. doi: 10.3389/fpsyg.2014.00588
Kan, P. F., and Windsor, J. (2010). Word learning in children with primary language impairment: a meta-analysis. J. Speech Lang. Hear. Res. 53, 739–756. doi: 10.1044/1092-4388(2009/08-0248)
Kapa, L. L., and Erikson, J. A. (2020). The relationship between word learning and executive function in preschoolers with and without developmental language disorder. J. Speech Lang. Hear. Res. 63, 2293–2307. doi: 10.1044/2020_JSLHR-19-00342
Kaufman, S. B., DeYoung, C. G., Gray, J. R., Jiménez, L., Brown, J., Mackintosh, N., et al. (2010). Implicit learning as an ability. Cognition 116, 321–340. doi: 10.1016/j.cognition.2010.05.011
Kemény, F., and Lukács, Á. (2021). The role of statistical learning and verbal short-term memory in impaired and typical lexical development. Front. Commun. 6, 452. doi: 10.3389/fcomm.2021.700452
Kidd, E. (2012). Implicit statistical learning is directly associated with the acquisition of syntax. Dev. Psychol. 48, 171–184. doi: 10.1037/a0025405
Kidd, E., and Arciuli, J. (2016). Individual differences in statistical learning predict children's comprehension of syntax. Child Dev. 87, 184–193. doi: 10.1111/cdev.12461
Lahey, M., and Edwards, J. (1999). Naming errors of children with specific language impairment. J. Speech Lang. Hear. Res. 42, 195–205. doi: 10.1044/jslhr.4201.195
Lammertink, I., Boersma, P., Rispens, J., and Wijnen, F. (2020a). Visual statistical learning in children with and without DLD and its relation to literacy in children with DLD. Read. Writ. 33, 1557–1589. doi: 10.1007/s11145-020-10018-4
Lammertink, I., Boersma, P., Wijnen, F., and Rispens, J. (2017). Statistical learning in specific language impairment: a meta-analysis. J. Speech Lang. Hear. Res. 1–13. doi: 10.1044/2017_JSLHR-L-16-0439
Lammertink, I., Boersma, P., Wijnen, F., and Rispens, J. (2019). Children with developmental language disorder have an auditory verbal statistical learning deficit: evidence from an online measure. Lang. Learn. 70, 137–148. doi: 10.1111/lang.12373
Lammertink, I., Boersma, P., Wijnen, F., and Rispens, J. (2020b). Statistical learning in the visuomotor domain and its relation to grammatical proficiency in children with and without developmental language disorder: a conceptual replication and meta-analysis. Lang. Learn. Dev. 16, 426–450. doi: 10.1080/15475441.2020.1820340
Leonard, L. B., Nippold, M. A., Kail, R., and Hale, C. A. (1983). Picture naming in language-impaired children. J. Speech Hear. Res. 26, 609–615. doi: 10.1044/jshr.2604.609
Lukács, Á., and Kemény, F. (2014). Domain-general sequence learning deficit in specific language impairment. Neuropsychology 28, 472–483. doi: 10.1037/neu0000052
Lum, J. A. G., Conti-Ramsden, G., Morgan, A. T., and Ullman, M. T. (2014). Procedural learning deficits in specific language impairment (SLI): a meta-analysis of serial reaction time task performance. Cortex 51, 1–10. doi: 10.1016/j.cortex.2013.10.011
MacNamara, J. (1972). Cognitive basis of language learning in infants. Psychol. Rev. 79, 1–13. doi: 10.1037/h0031901
Mainela-Arnold, E., and Evans, J. L. (2014). Do statistical segmentation abilities predict lexical-phonological and lexical-semantic abilities in children with and without SLI? J. Child Lang. 41, 327–351. doi: 10.1017/S0305000912000736
Mainela-Arnold, E., Evans, J. L., and Coady, J. A. (2010). Explaining lexical-semantic deficits in specific language impairment: the role of phonological similarity, phonological working memory, and lexical competition. J. Speech Lang. Hear. Res. 53, 1742–1756. doi: 10.1044/1092-4388(2010/08-0198)
Marinellie, S. A., and Johnson, C. J. (2002). Definitional skill in school-age children with specific language impairment. J. Commun. Disord. 35, 241–259. doi: 10.1016/S0021-9924(02)00056-4
Markman, E. M., and Wachtel, G. F. (1988). Children's use of mutual exclusivity to constrain the meanings of words. Cogn. Psychol. 20, 121–157. doi: 10.1016/0010-0285(88)90017-5
McGregor, K. K. (1997). The nature of word-finding errors of preschoolers with and without word- finding deficits. J. Speech Lang. Hear. Res. 40, 1232–1244. doi: 10.1044/jslhr.4006.1232
McGregor, K. K., Arbisi-Kelm, T., and Eden, N. (2017). The encoding of word forms into memory may be challenging for college students with developmental language impairment. Int. J. Speech Lang. Pathol. 19, 43–57. doi: 10.3109/17549507.2016.1159337
McGregor, K. K., Berns, A. J., Owen, A. J., Michels, S. A., Duff, D., Bahnsen, A. J., et al. (2012). Associations between syntax and the lexicon among children with or without ASD and language impairment. J. Autism Dev. Disord. 42, 35–47. doi: 10.1007/s10803-011-1210-4
McGregor, K. K., Newman, R. M., Reilly, R. M., and Capone, N. C. (2002). Semantic representation and naming in children with specific language impairment. J. Speech Lang. Hear. Res. 45, 998–1014. doi: 10.1044/1092-4388(2002/081)
McGregor, K. K., Oleson, J., Bahnsen, A., and Duff, D. (2013). Children with developmental language impairment have vocabulary deficits characterized by limited breadth and depth. Int. J. Lang. Commun. Disord. 48, 307–319. doi: 10.1111/1460-6984.12008
McGregor, K. K., Smolak, E., Jones, M., Oleson, J., Eden, N., Arbisi-Kelm, T., et al. (2022). What children with developmental language disorder teach us about cross-situational word learning. Cogn. Sci. 46. doi: 10.1111/cogs.13094
McMurray, B., Horst, J. S., and Samuelson, L. K. (2012). Word learning emerges from the interaction of online referent selection and slow associative learning. Psychol. Rev. 119, 831–877. doi: 10.1037/a0029872
Melby-Lervåg, M., Lervåg, A., Lyster, S. A. H., Klem, M., Hagtvet, B., Hulme, C., et al. (2012). Nonword-repetition ability does not appear to be a causal influence on children's vocabulary development. Psychol. Sci. 23, 1092–1098. doi: 10.1177/0956797612443833
Misyak, J. B., Christiansen, M. H., and Tomblin, J. B. (2010). On-line individual differences in statistical learning predict language processing. Front. Psychol. 1, 31. doi: 10.3389/fpsyg.2010.00031
Montgomery, J. W., Magimairaj, B. M., and Finney, M. C. (2010). Working memory and specific language impairment: an update on the relation and perspectives on assessment and treatment. Am. J. Speech Lang. Pathol. 19, 78–94. doi: 10.1044/1058-0360(2009/09-0028)
Nash, M., and Donaldson, M. L. (2005). Word learning in children with vocabulary deficits. J. Speech Lang. Hear. Res. 48, 439–458. doi: 10.1044/1092-4388(2005/030)
Nation, K. (2014). Lexical learning and lexical processing in children with developmental language impairments. Philos. Trans. R. Soc. Lond. B. Biol. Sci. 369, 20120387. doi: 10.1098/rstb.2012.0387
Newman, R., Ratner, N. B., Jusczyk, A. M., Jusczyk, P. W., and Dow, K. A. (2006). Infants' early ability to segment the conversational speech signal predicts later language development: a retrospective analysis. Dev. Psychol. 42, 643–655. doi: 10.1037/0012-1649.42.4.643
Noonan, N. B. (2018). Exploring the Process of Statistical Language Learning. London, ON: The University of Western Ontario.
Obeid, R., Brooks, P. J., Powers, K. L., Gillespie-Lynch, K., and Lum, J. A. G. (2016). Statistical learning in specific language impairment and autism spectrum disorder: a meta-analysis. Front. Psychol. 7, 1245. doi: 10.3389/fpsyg.2016.01245
Perry, L. K., Samuelson, L. K., Malloy, L. M., and Schiffer, R. N. (2010). Learn locally, think globally: exemplar variability supports higher-order generalization. Psychol. Sci. 21, 1894–1902. doi: 10.1177/0956797610389189
Plante, E., Ogilvie, T., Vance, R., Aguilar, J. M., Dailey, N. S., Meyers, C., et al. (2014). Variability in the language input to children enhances learning in a treatment context. Am. J. Speech Lang. Pathol. 23, 530–545. doi: 10.1044/2014_AJSLP-13-0038
Psychology Software Tools Inc (2016). E-Prime (3.0). Available online at: https://support.pstnet.com/ (accessed October, 2019).
Quam, C., Cardinal, H., Gallegos, C., and Bodner, T. (2020). Sound discrimination and explicit mapping of sounds to meanings in preschoolers with and without developmental language disorder. Int. J. Speech Lang. Pathol. 23, 26–37. doi: 10.1080/17549507.2020.1750701
R Core Team (2020). R: A Language and Environment for Statistical Computing. R Foundation for Statistical Computing. Available online at: https://www.R-project.org/ (accessed August, 2022).
Ralli, A. M., Dockrell, J., and Dockrell, J. E. (2010). Real world word learning: exploring the development of children's lexical representations. Psychology, 17, 1–24. doi: 10.12681/psy_hps.23750
Raven, J., Raven, J., and Court, J. (2003). Manual for Raven's Progressive Matrices and Vocabulary Scales. San Antonio, TX: Harcourt Assessment. doi: 10.53841/bpstest.2003.rvs
Rice, M. L., Buhr, J. C., and Nemeth, M. (1990). Fast mapping word-learning abilities of language-delayed preschoolers. J. Speech Hear. Disord. 55, 33–42. doi: 10.1044/jshd.5501.33
Rice, M. L., Oetting, J. B., Marquis, J., Bode, J., and Pae, S. (1994). Frequency of input effects on word comprehension of children with specific language impairment. J. Speech Hear. Res. 37, 106–122. doi: 10.1044/jshr.3701.106
Rispens, J., and Baker, A. (2012). Nonword repetition: the relative contributions of phonological short-term memory and phonological representations in children with language and reading impairment. J. Speech Lang. Hear. Res. 55, 683–694. doi: 10.1044/1092-4388(2011/10-0263)
Saffran, J. R., Aslin, R. N., and Newport, E. L. (1996). Statistical learning by 8-month-old infants. Science 274, 1926–1928. doi: 10.1126/science.274.5294.1926
Saffran, J. R., Newport, E. L., Aslin, R. N., Tunick, R. A., and Barrueco, S. (1997). Incidental language learning: listening (and learning) out of the corner of your ear. Psychol. Sci. 8, 101–105. doi: 10.1111/j.1467-9280.1997.tb00690.x
Sandgren, O., Salameh, E. K., Nettelbladt, U., Dahlgren-Sandberg, A., and Andersson, K. (2021). Using a word association task to investigate semantic depth in Swedish-speaking children with developmental language disorder. Logoped. Phoniatr. Vocol. 46, 134–140. doi: 10.1080/14015439.2020.1785001
Semel, E., Wiig, E., and Secord, W. (2010). Clinical Evalutation of Language Fundamentals: Dutch Version, Eds W. Kort, E. Compaan, M. Schittekatte, and P. Dekker, 3rd ed. London: Pearson.
Shafto, C. L., Conway, C. M., Field, S. L., and Houston, D. M. (2012). Visual sequence learning in infancy: domain-general and domain-specific associations with language. Infancy 17, 247–271. doi: 10.1111/j.1532-7078.2011.00085.x
Sheng, L., and McGregor, K. K. (2010). Lexical-semantic organization in children with specific language impairment. J. Speech Lang. Hear. Res. 53, 146–159. doi: 10.1044/1092-4388(2009/08-0160)
Siegelman, N., Bogaerts, L., Kronenfeld, O., and Frost, R. (2017). Redefining “learning” in statistical learning: what does an online measure reveal about the assimilation of visual regularities? Cogn. Sci. 142, 692–727. doi: 10.1111/cogs.12556
Singh, L., Steven Reznick, J., and Xuehua, L. (2012). Infant word segmentation and childhood vocabulary development: a longitudinal analysis. Dev. Sci. 15, 482–495. doi: 10.1111/j.1467-7687.2012.01141.x
Smith, K., Smith, A. D. M., and Blythe, R. A. (2011). Cross-situational learning: an experimental study of word-learning mechanisms. Cogn. Sci. 35, 480–498. doi: 10.1111/j.1551-6709.2010.01158.x
Smith, L., and Yu, C. (2008). Infants rapidly learn word-referent mappings via cross-situational statistics. Cognition 106, 1558–1568. doi: 10.1016/j.cognition.2007.06.010
Smolak, E., McGregor, K. K., Arbisi-Kelm, T., and Eden, N. (2020). Sustained attention in developmental language disorder and its relation to working memory and language. J. Speech Lang. Hear. Res. 63, 4096–4108. doi: 10.1044/2020_JSLHR-20-00265
Spencer, M., Kaschak, M. P., Jones, J. L., and Lonigan, C. J. (2015). Statistical learning is related to early literacy-related skills. Read. Writ. 28, 467–490. doi: 10.1007/s11145-014-9533-0
Suanda, S. H., Mugwanya, N., and Namy, L. L. (2014). Cross-situational statistical word learning in young children. J. Exp. Child Psychol. 126, 395–411. doi: 10.1016/j.jecp.2014.06.003
Suanda, S. H., and Namy, L. L. (2012). Detailed behavioral analysis as a window into cross-situational word learning. Cogn. Sci. 36, 545–559. doi: 10.1111/j.1551-6709.2011.01218.x
Tomblin, J. B., Mainela-Arnold, E., and Zhang, X. (2007). Procedural learning in adolescents with and without specific language impairment. Lang. Learn. Dev. 3, 269–293. doi: 10.1080/15475440701377477
Ullman, M. T., and Pullman, M. Y. (2015). A compensatory role for declarative memory in neurodevelopmental disorders. Neurosci. Biobehav. Rev. 51, 205–222. doi: 10.1016/j.neubiorev.2015.01.008
Venker, C. E. (2019). Cross-situational and ostensive word learning in children with and without autism spectrum disorder. Cognition 183, 181–191. doi: 10.1016/j.cognition.2018.10.025
Viviani, P. (1990). “Eye movement in visual search: cognitive, perceptual and motor control aspects,” in Eye Movements and their Role in Visual Cognitive Processes, ed E. Kowler (Amsterdam: Elsevier Science Publishers BV), 353–393.
Vlach, H. A., and DeBrock, C. A. (2017). Remember dax? Relations between children's cross-situational word learning, memory, and language abilities. J. Mem. Lang. 93, 217–230. doi: 10.1016/j.jml.2016.10.001
Vlach, H. A., and Johnson, S. P. (2013). Memory constraints on infants' cross-situational statistical learning. Cognition 127, 375–382. doi: 10.1016/j.cognition.2013.02.015
von Koss Torkildsen, J., Dailey, N. S., Aguilar, J. M., Gómez, R., and Plante, E. (2013). Exemplar variability facilitates rapid learning of an otherwise unlearnable grammar by individuals with language-based learning disability. J. Speech Lang. Hear. Res. 56, 618–629. doi: 10.1044/1092-4388(2012/11-0125)
Yang, Y., Yim, D., Kim, S., and Han, J. (2013). The relationship among receptive vocabulary, non-word repetition, and quick incidental learning in preschoolers with and without delay in vocabulary development. Commun. Sci. Disord. 18, 379–391. doi: 10.12963/csd.13079
Yu, C., and Ballard, D. H. (2007). A unified model of early word learning: integrating statistical and social cues. Neurocomputing 70, 2149–2165. doi: 10.1016/j.neucom.2006.01.034
Yu, C., and Smith, L. (2012). Modeling cross-situational word–referent learning: prior questions. Psychol. Rev. 119, 21–39. doi: 10.1037/a0026182
Yu, C., and Smith, L. B. (2007). Rapid word learning under uncertainty via cross-situational statistics. Psychol. Sci. 18, 414–420. doi: 10.1111/j.1467-9280.2007.01915.x
Yu, C., and Smith, L. B. (2011). What you learn is what you see: using eye movements to study infant cross-situational word learning. Dev. Sci. 14, 165–180. doi: 10.1111/j.1467-7687.2010.00958.x
Yu, C., Zhong, Y., and Fricker, D. (2012). Selective attention in cross-situational statistical learning: evidence from eye tracking. Front. Psychol. 3, 148. doi: 10.3389/fpsyg.2012.00148
Yurovsky, D., Fricker, D. C., Yu, C., and Smith, L. B. (2014). The role of partial knowledge in statistical word learning. Psychon. Bull. Rev. 21, 1–22. doi: 10.3758/s13423-013-0443-y
Keywords: developmental language disorder (DLD), statistical learning, cross-situational learning, eye-tracking, lexical-semantic abilities
Citation: Broedelet I, Boersma P and Rispens J (2023) Implicit cross-situational word learning in children with and without developmental language disorder and its relation to lexical-semantic knowledge. Front. Commun. 8:1021654. doi: 10.3389/fcomm.2023.1021654
Received: 17 August 2022; Accepted: 23 January 2023;
Published: 28 February 2023.
Edited by:
Mila Vulchanova, Norwegian University of Science and Technology, NorwayReviewed by:
David Saldaña, Sevilla University, SpainVsevolod Kapatsinski, University of Oregon, United States
Copyright © 2023 Broedelet, Boersma and Rispens. This is an open-access article distributed under the terms of the Creative Commons Attribution License (CC BY). The use, distribution or reproduction in other forums is permitted, provided the original author(s) and the copyright owner(s) are credited and that the original publication in this journal is cited, in accordance with accepted academic practice. No use, distribution or reproduction is permitted which does not comply with these terms.
*Correspondence: Iris Broedelet, iris.broedelet@gmail.com