- 1School of Education, University of Leeds, Leeds, United Kingdom
- 2School of Medicine, Keele University, Keele, United Kingdom
Revision is a fundamental part of the writing process and is particularly important in the production of high-quality academic writing. This study is an exploratory examination of changes in revision behavior, as measured by keystroke logging software, at the beginning (T1) and end (T2) of a one-month intensive English for Academic Purposes (EAP) course on n = 39 undergraduate and postgraduate students. Bayes Factors (BFs) are utilized as measures of strength of evidence for changes in behavior. In this paper, we examine the application of a Bayesian Hypothesis Testing (BHT) approach and its implications specifically for exploratory studies, i.e., studies with relatively small samples intended to search data for emergent patterns. The results show that, in most cases, we have moderate evidence against any change in behavior over time. Based on this evidence, we conclude that the experimental parameters of further exploratory work into the development of revisions should be modified to maximize the chance of finding patterns in the data from which to generate any confirmatory hypotheses.
1. Introduction
This paper examines the impact of a short-term intensive English for Academic Purposes (EAP) program on revision processes in L2 writing. Revision is a fundamental part of the writing process and plays a particularly important role in academic contexts (Breuer, 2017). While studies investigating on-line (i.e., captured moment-to-moment) revision processes exist (e.g., Barkaoui, 2016; Révész et al., 2017), no previous studies, to the authors' knowledge, have analyzed changes in revision behavior over time via on-line measures. Given the novelty of the area of investigation, i.e., changes in revision over time, no specific hypotheses about the effects of revision are posited as none exist in the literature. Thus, the analyses in this paper are exploratory rather than confirmatory in nature, i.e., this study was not powered to address a particular hypothesis and give strong confirmatory evidence about the likelihood of it being true. The most frequently used approach to deal with this kind of data is null hypothesis significance testing (NHST). However, numerous sources over the past two decades have reported shortcomings of this approach (see e.g., Kline, 2004; Oswald and Plonsky, 2010; Sun and Fan, 2010; Wei et al., 2019). We suggest that there are three problems with this approach when applied to exploratory studies, specifically: (1) p-values tell us nothing about the likelihood of the null hypothesis being true, and thus any follow up a particular hypothesis with further study would probably be futile, (2) p-values only give a binary all-or nothing outcome in a situation where more nuance in interpretation may be needed because of a low sample size and power to detect differences, (3) the information gathered in an exploratory study is usually discarded when moving on to a confirmatory study which is an inefficient use of data that is often expensive and burdensome to collect. We suggest that using Bayes Factors (BFs) to analyze exploratory studies will address these shortcomings. While many previous studies promote, describe, and explain Bayesian Hypothesis-Testing in general (BHT) to the L2 research community (e.g., Norouzian et al., 2018a,b), to our knowledge, this is the first paper to implement and discuss BHT in L2 research explicitly in an exploratory study context.
2. Literature review
2.1. Revisions as a component of theoretical models of writing
Writing is a recursive rather than purely linear process. Zamel (1982) states that to move forward with the task of producing a finished text, writers must often move backward in their text and make revisions. Revisions refer to any kind of change, major or minor, to the already written text at any point in the writing process (Fitzgerald, 1987), not only changes to the final produced text.
Writing revision forms a core component of various theoretical models of the writing process (Hayes and Flower, 1980; Bereiter and Scardamalia, 1987; Hayes, 1996, 2012; Chenoweth and Hayes, 2001). Hayes and Flower (1980), in one of the first and best-known conceptualizations of the writing process, considered reviewing as a recurring intentional process that occurs at all stages of text production. Hayes (1996) in an updated iteration of the 1980 model posits that revision involves basic cognitive processes such as text production, text interpretation, and reflection. As revision involves numerous complex concurrent processes, it is considered to be burdensome on cognitive resources and working memory. As they are producing text, writers continually verify that the text they are writing matches their writing goals, and if there is a mismatch, they revise the text to make it better meet their specific goals. These changes may be linguistic, stylistic or conceptual in nature. A revision occurs as a result of the writer reviewing their text and realizing that a mistake has been made or they are unsatisfied with some aspect of what they have written (Hayes and Flower, 1980). In summary, reviewing is an internal process which involves the evaluation of the extent to which planned writing goals are being achieved, whereas a revision is a physical process which modifies text to be more in line with the planned writing goals.
2.2. Revision behaviors in second language writing
For writers producing text in a second language, factors such as level of writing expertise, level of proficiency in the L2, task type, mode of writing, and the existence of time constraints have been posited to affect the type and number of revisions made (Barkaoui, 2007, 2016). More-skilled writers than less-skilled ones differ in terms of (i) what they revise, (ii) when in the text production process they revise, (iii) how many revisions they make, and iv) the reasons for making revisions (see e.g., Faigley and Witte, 1981; Zamel, 1983; Roca de Larios et al., 2008; Manchón et al., 2009). More-skilled writers tend to make a larger variety of types of revisions, with a focus on those related to text organization and meaning, which usually involve major changes to the content of a text. Conversely, less-skilled writers tend to concentrate more on surface-level revisions to aspects of the text such as spelling and punctuation rather than revising the content of their writing (Faigley and Witte, 1981; Zamel, 1983; Bereiter and Scardamalia, 1987). The concept of working memory capacity (Wen et al., 2015), i.e., the cognitive system that allows us to hold and work with a limited amount of information, is often used to explain the differences in revision behaviors we see between more- and less-skilled writers (Alamargot and Chanquoy, 2001; Stevenson et al., 2006; Chanquoy, 2009; Barkaoui, 2016). Specifically, it is suggested that less-skilled readers' writing processes are not as well automatized as those of more-skilled readers, and thus, they do not have access to sufficient free working memory capacity to enable complex revision processes to be undertaken. More-skilled writers, whose writing processes are more automatized, have free working memory capacity to make revisions which go deeper than the surface of the text (Chanquoy, 2009; Barkaoui, 2016).
The level of skill possessed in L2 writing is considered to influence the way in which cognitive capacity is allocated to various activities during the process of text composition. More-skilled writers have been found to show evidence of engaging more with a wider range of writing processes than less skilled writers (Roca de Larios et al., 2008). Manchón et al. (2009) suggest that more-skilled writers in a second language are more flexible in directing their attentional resources during writing. In line with the consensus in the literature, the less-skilled writers in this study focused more on surface level linguistic revisions while the more-skilled writers were able to make both surface level and conceptual revisions. The more skilled the writer, the more they exhibited higher-level cognitive processing in revisions by revising for discourse-level issues like organization, writing style and meaning.
2.3. Keystroke logging in L2 writing and revision research
Over the past two decades, keystroke logging has become an increasingly popular method for capturing data on revision processes for both first language (L1) and second language (L2) writing research (Leijten and Van Waes, 2006; Strömqvist et al., 2006; Van Waes et al., 2009). Keystroke logging records all keystrokes, mouse movements and clicks made during a writing session, allowing researchers to reconstruct the writing process and analyse detailed output. Furthermore, keystroke logging has good ecological validity (Van Waes et al., 2009), compared to methods like think-aloud protocols, as it is relatively unobtrusive and does not interfere with the writing process.
Various studies have looked at revision behaviors extracted from keystroke logged data with reference to the skill level of writers in both L1 and L2 (Thorson, 2000; Lindgren and Sullivan, 2006; Stevenson et al., 2006; Choi, 2007; see e.g., Barkaoui, 2007, 2016). Significantly more revisions were made by writers when writing in their L2 than their L1 (Thorson, 2000; Lindgren and Sullivan, 2006; Stevenson et al., 2006). Furthermore, when writing in an L2, language-related revisions tend to be more frequent than revisions related to content (Choi, 2007). With regards to differences in skill levels, both Barkaoui (2016) and Stevenson et al. (2006) found that lower-skilled writers made more linguistically- oriented typographic revisions. They argue this lack of automaticity in orthographic processing may have overburdened the writers' working memories diverting capacity from higher-level conceptual revisions for style and meaning to the lower-level typographic revisions (Stevenson et al., 2006). Regarding the nature of typographic revisions, Choi (2007) found that more-skilled writers were more apt to make revisions further away from the leading-edge (the point at which the writer is typing), and Barkaoui (2016) found that more proficient writers tended to correct typography at the end of the writing process whereas there was more of a tendency for less-skilled writers to correct as they wrote. Given these results, it might be concluded that the revision processes of higher-skilled writers are more recursive than those of lower-skilled writers, i.e., the higher-skilled writers are more likely to move around the page more when making revisions.
2.4. The advantages of Bayesian hypothesis testing for exploratory studies
As already mentioned, this paper reports on an exploratory rather than a confirmatory study. Wagenmakers et al. (2012) suggest that only studies which pre-specify (and publish) their method of analysis, hypotheses and required sample sizes to test those hypotheses, in advance of data collection, and then follow through on that plan can be called confirmatory and be considered to supply strong evidence. Indeed, L2 research is increasingly moving toward the registration and publication of such confirmatory study protocols (see, e.g., Marsden et al., 2018). While we do not hold such strong beliefs about what studies can be considered confirmatory, we do feel that any outcome without an a priori clearly defined hypothesis, and some consideration of statistical power cannot be considered as confirmatory. Thus, clearly, the outcomes in this study are exploratory in nature. Wagenmakers et al. (2012) by no means dismiss exploratory research, stating “exploration is an essential component of science and is key to new discoveries and scientific progress; without exploratory studies, the scientific landscape is sterile and uninspiring” (p. 635). They further propose that the foci of exploratory work should be to (i) report interesting “patterns” in the data, (ii) evaluate relevant tentative results, and (iii) establish a path for confirmatory studies. Analyzing exploratory data using BFs, a measure of evidence which provides an alternative to p-values, offers some unique advantages.
The first advantage is the fact that they can provide evidence in favor of the null hypothesis (Wagenmakers et al., 2018b). A BF can tell us three things: (1) if the data are more likely to have been generated under the null hypothesis (usually no difference/effect), (2) if the data we have are inconclusive in terms of showing the lack of an effect, and (3) whether the data are more likely to have been generated under the alternative hypothesis (usually some difference/effect). This is in contrast with p-values, which can only tell us two things: (1) if the data are inconclusive in showing the existence or lack of an effect (i.e., p > 0.05), or (2) if we can reject (i.e., p < 0.05) the null hypothesis. Practically, for exploratory studies, this means that BHT allows us to actively close down avenues for further exploration as well as open up new ones. In other words, finding support for a null hypothesis (H0) explicitly implies that an effect is likely not worth following up on (given our specified prior distribution), whereas a p-value > 0.05 only implies that either the effect does not exist or that the experiment did not have sufficient power (i.e., a big enough sample size) to find one. These results in favor of the null hypothesis could be published to stop other researchers going down the same blind alleys. In a confirmatory study, showing evidence for the null is less important as the study will have been designed to provide evidence for the minimally important effect size specified in the power calculation.
A second advantage of BFs is that they allow us to express a graded level of certainty we have in the hypothesis in question (see Table 1) (Wagenmakers et al., 2018b). In contrast, the decision on the analytical framework using p-values is strictly binary, i.e., one can either reject or not reject the null hypothesis, and a p-value of 1 × 10−10 leads to no stronger conclusion than one of 0.049. Practically, for exploratory studies, this means that researchers do not have to follow an all-or-nothing decision-making framework, as they would in a confirmatory study, and can be more tentative in the conclusions they draw from their data about what patterns exist and what might be beneficial to follow up on. Researchers may choose to follow up and collect more data in cases of “anecdotal” or even “no” evidence (see Table 1) as no firm answer has been arrived upon, whereas they would not follow up on a non-significant p-value > 0.05.
A third advantage of BHT is the fact that we can incorporate prior knowledge (Wagenmakers et al., 2018b). While this is not useful for an exploratory study itself, as it is unlikely that we have much prior knowledge given we are exploring a given set of hypotheses, it is useful for any lead-on confirmatory studies. Specifically, the information about an effect, which is found in the exploratory sample, can be encoded beforehand for a confirmatory study, and it does not go to waste as it would in NHST. In other words, the data gathered in the exploratory study can be used in the confirmatory study, reducing the required sample size and thus cost and burden on participants. In frequentist approaches, unless part of a pre-planned internal pilot study, this is not permissible.
2.5. Research question
As this is the first study to explore changes in on-line revision behavior over time, we do not have detailed a priori expectations about these changes. Rather, in general, we expect that at T2 the writers will be, to some degree, more skilled after the EAP program (T2) than they were before it (T1) and that their revision behaviors will reflect the fact that they have more cognitive capacity for higher-level revision behaviors, as outlined above. The following research question was addressed in the study:
RQ: How does revision behavior, as measured by keystroke-logging, change over the course of a one-month intensive EAP program?
3. Methodology
3.1. Research context
The study was conducted on an intensive four-week pre-sessional EAP summer program at a British university. The aims of the EAP program were to (i) develop students' academic reading and writing skills, (ii) to improve their critical thinking ability, and (ii) to raise learners' awareness of the skills and strategies they might require while studying in the UK. The program was aimed at students with the International English Language Testing System (IELTS) scores of 5.5–6.5 or B1 to B2 on the Common European Framework of Reference (CEFR) (Council of Europe, 2001) and who received a conditional offer from their university because their level of English language proficiency did not meet the minimum requirements. Students received 15 h of teaching per week and were expected to study independently ~ 15 h a week and complete written assignments. Assignments took the form of argumentative essays. The program adopted a task-based approach and comprised three modules: (1) Academic Reading and Writing, (2) Listening, Reading and Discussion, and (3) Oral Presentations. Week by week, the following aspects were covered:
Week 1: Understanding the writing process, organizing information, reporting others' words, and writing introductions,
Week 2: Identifying and evaluating main points in reading, taking notes for argumentative essays, assessing reliability of academic sources, learning to paraphrase and summarize, sequencing paragraphs, recognizing cohesive features in writing, and writing conclusions,
Week 3: Reading and writing critically, taking a position and arguing a case, and integrating multiple sources,
Week 4: Academic writing style, the use of connectives and linking words for cohesion, avoiding sexist writing, and a review of paraphrasing and referencing activities.
Students did not receive any explicit language instruction; however, linguistic errors, such as grammar, vocabulary, and spelling errors, were generally highlighted in feedback and discussed in tutorials. It should be noted that effective revision behavior was not explicitly taught in the course.
3.2. Participants
Participants were a convenience sample of student volunteers (both undergraduate and postgraduate) who received no renumeration for their participation. The data presented were collected as part of a larger multifaceted study of writing behavior development [see e.g., Mazgutova and Kormos (2015) for analysis of the syntactic and lexical development in this group of students and Mazgutova (2020) for an analysis of differential revision behaviors between undergraduate and postgraduate students]. Background information on all participants is given in Table 2. Most participants had a Chinese L1 background—reflective of the population of the EAP course. All students had studied English at school in their home countries but had only limited experience of academic writing in English. None of the students had prior experience of living in English-speaking countries.
3.3. Design and analytical software
The study follows a pretest-posttest quasi-experimental design with the treatment being the EAP course. There was no control group as it was impossible to sample students who would have required an EAP course but were not taking such a course at that time. Inputlog 8 (Leijten and Van Waes, 2013) was used for extraction of the keystroke data, and R version 3.5.1 (Development Core Team, 2017) for data preparation and manipulation. For the BHT, Jeffreys's Amazing Statistics Program (JASP) (JASP-team, 2018) was used because it is open source, free to use, and has a clean GUI1. Vignettes explaining advantages of BHT in general (Wagenmakers et al., 2018b) and how to use JASP (Wagenmakers et al., 2018a) have recently been published.
3.4. Instruments
Participants completed two argumentative writing tasks, one at the beginning (T1) and the other at the end (T2) of the program. Both writing sessions were conducted in a computer lab where students were required to write an essay of between 300 and 400 words. The order of the tasks was counter-balanced (i.e., half the students did topic A at T1 and half did topic B. At T2, students did the topic they did not do at T1). It was assumed that the students might be interested in these topics and would be able to bring in a range of examples from their school life. Both topics require argumentation; this genre was chosen for the present study because it is the main type of writing required in written assignments and exams in a large number of disciplines studied at university level. Therefore, it constitutes the particular focus of the EAP program; all written assignments that students are asked to produce in the course involve argumentation and critical thinking. The instructions and essay prompts chosen to be used in our study were as follows:
“Please read the prompt below carefully and type a 300–400 word essay. You will be given a maximum of 45 minutes to complete this task. Please avoid referring to dictionaries or any other reference books while writing the essay.
Topic A: Exams cause unnecessary stress for students. How far do you agree?
Topic B: Any student caught cheating in school or college exams should be automatically dismissed. How far do you agree?”
3.5. Measures
The following categorization of revisions was adapted from Barkaoui's (2016) scheme by: (i) location relative to previous textual output (Type), (ii) function (orientation), (iii) high/low-textual-level (domain), (iv) method used to make (action) and v) time in the writing process at which they were made (location) was adapted from Barkaoui's (2016) scheme. Figure 1 explains this coding scheme in greater detail. To streamline the process of coding, the Inputlog output was extracted to a spreadsheet. The locations of all revisions were flagged, and revisions made at the leading edge were distinguished from those that were not (see Figure 2). One rater coded all the essays, and a second rater coded five of those essays. The Kappa agreement coefficients were: orientation (κ = 0.53, 95%-CI = 0.44–0.62), domain (κ = 0.85, 95%–CI = 0.78–0.92), action (κ = 0.73, 95%–CI = 0.64–0.82); note that the type and location codes were computed directly from the Inputlog data. The essays were divided into three terciles, by total time taken, and the number of contextual revisions per 100 words was tallied and expressed in the variables “Location: Tercile_1, 2, and 3”. Blind assessment of the essays was done by one author using the IELTS Academic Writing scoring rubric2. Blind second marking was done on a randomly chosen 10% of the essays (ICC3 = 0.73). The overall score was added to the variables to be analyzed and termed, “Score: Total”.
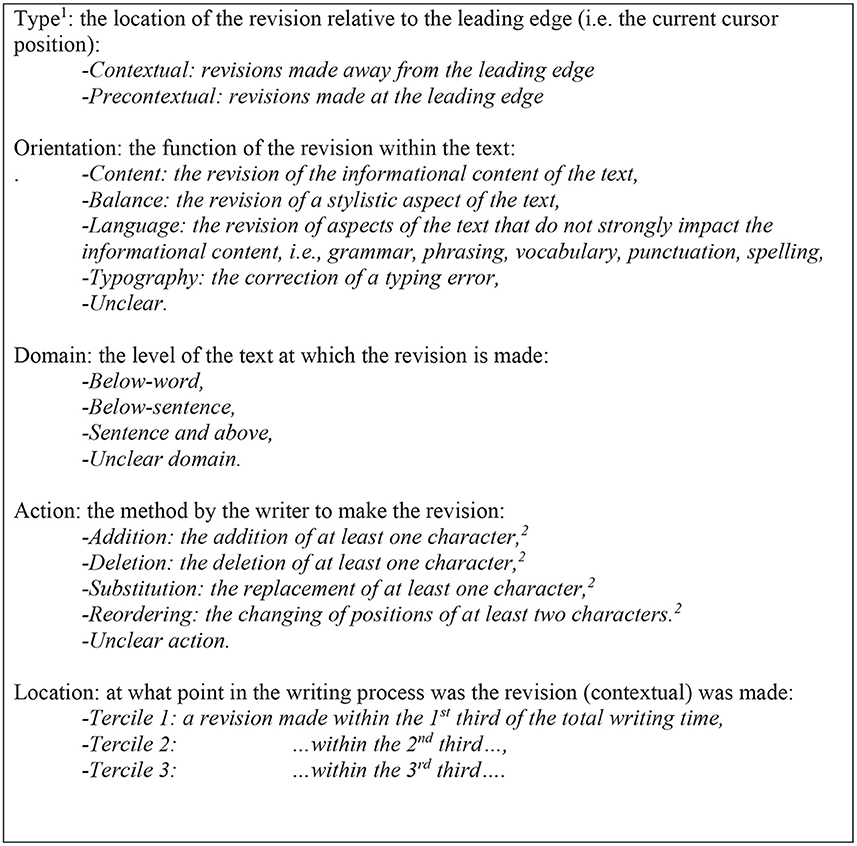
Figure 1. Taxonomy of revisions—adapted from Barkaoui (2016). 1Barkaoui (2016) has an additional coding scheme for contextual revisions. We did not categorize and analyze contextual revisions as our focus was on changes in higher-level revision behaviors.2 In Barkaoui's (2016) scheme the action class refers to changes made at the whole word level and above. In our scheme any addition, deletion, substitution or reordering is considered an action as it was more logically consistent that each contextual revision has a prescribed function (orientation), level of text (domain) and method (action).
3.6. Bayesian hypothesis testing
Bayes Factors are calculated by comparing the capability of two competing hypotheses, H0 and the Alternative (H1), to describe some observed data (Wagenmakers et al., 2018b). The BF is expressed on a positive continuous scale on which a value above one (usually) represents evidence in favor of H1 and values below one (usually) represent evidence in favor of H0. A BF of 10 indicates that data are 10 times more likely to have occurred under H1 than under H0, while a value of 0.1 (note: 1/0.1 = 10) indicates that the data are 10 times more likely to have occurred under H0 than under H1 (Lee and Wagenmakers, 2013). Table 1 gives a common benchmark interpretation for BFs. Two key points to infer from this table are: (i) BFs provide a measure of strength of evidence, and (ii) BFs can give evidence for H0 (Wagenmakers et al., 2018b). Readers who require more background on the subject are directed to recent work which more fully explores the theoretical underpinnings, advantages and practical implications of Bayesian methods in general, i.e., not specific to exploratory studies (see e.g., Norouzian et al., 2018a,b; Wagenmakers et al., 2018a).
3.7. Bayesian paired T-test
As already mentioned, this analysis is exploratory as we do not have detailed a priori expectations about changes over time on our measures, rather we are exploring the data using a BHT testing framework to uncover patterns. Given the often low-stakes nature of exploratory analysis and the relatively low sample size, we do not want to increase the complexity of the analysis beyond necessity, we want to enhance the communicability of our results. Therefore, we have chosen to apply Bayesian paired T-tests to each outcome. Note, however, if this were a higher-stakes confirmatory study with a larger sample size, we would be using a more complex approach such as Bayesian mixed-effects modeling for the analysis (see e.g., Van Waes et al., 2021).
Bayesian methods in general and BHT in particular involve the combination of a prior distribution with a likelihood to give a posterior distribution. The prior distribution represents pre-existing beliefs about the hypothesis. In the case of Bayesian paired t-tests in JAPS, this prior distribution is expressed on the effect size (Cohen's D) scale. Figure 3 gives a visualization of a Cauchy (0, 0.707)3 prior, the default in JASP4, which represents a belief that we are 50% sure that the effect size of the difference in question lies between ±0.707. Compared with a normal distribution in the same plot, the Cauchy prior has comparatively fat tails, which makes it more permissive of a large effect size, e.g., >±2.5, than a standard normal prior would be. The BF can be strongly influenced by this prior, and JASP provides diagnostic plots for a sensitivity-analysis on the effect of the prior on the BF. The likelihood which we combine with the prior comes from what the data we have collected tells us about the effect size. All other things being equal, a large difference in means and a small standard deviation (SD) within groups will indicate that there is a large effect. We use the posterior distribution to make statistical inferences about the effect size. JASP computes and outputs the BFs, the 95% credible intervals (an interval analogous to frequentist confidence intervals), proportion wheels visually representing the support for H0 Vs. H1, and plots of the prior and posterior distributions. The Bayesian (BFs), Frequentist (P-values and t-statistic) and effect size (Cohen's D) statistics were extracted using JASP (JASP-team, 2018). Given this is an exploratory study, and there is little in the way of established belief we chose a default prior, Cauchy (0, 0.707), and do not specify a direction for the test.
3.8. Assumption checking
Bayesian hypothesis testing still requires that we check assumptions with rigor. A three-step procedure was employed to check the assumptions of the paired t-test. In step 1, approximate normality was examined via the Shapiro-Wilk test. If non-normality was found, a Box-Cox power transformation was made (see Fox and Weisberg, 2011). Note the variables Orientation: Balance, Domain: Sentence_or_Above, and Action: Reordering were found to contain many 0 s, and we were unable to transform them to the approximate normal. The analysis of these variables comes with a warning. Visual inspection of the qqplots (a plot that allows diagnosis deviation from a distribution) and the Shapiro Wilk test showed that the variables, Location: Tercile 2 (SW = 0.950, p = 0.004), and Action: Substitution (SW = 0.957, p = 0.010) did not transform particularly well to the approximate normal. In step 2, all variables were checked for outliers > ±3 on the z scale. Only one outlier was found in the variable Location: Tercile_2 and this outlier was reduced to have a value of 2SDs from the mean. In step 3, all variables were checked for homogeneity of variance. The only variable showing some heterogeneity of variance was Domain: Below_Word [Levene's–F−4.480 (df = 1), p = 0.038].
4. Results
Table 3 gives the untransformed means and SDs for each of the outcome variables, transformed to be per 100 words, where appropriate. Notice there are clear differences within revision behavior categories, e.g., before the course, Type: Contextual = 4.20 (3.01), Type: Precontextual = 28.82 (19.43). However, visual inspection indicates few differences over time, which is the focus of this paper. Figure 4 gives example results plots and diagnostic output from JASP. The top panels refer to the hypothesis that there is a change from T1 to T2 on the variable Action: Addition. The top-left panel gives various pieces of information. The BF10 is in favor of H1 (note: BF01 is in favor of H0, and 1/5.361 = 0.187). The proportion-wheel (pie-chart) next to the BF values gives us a visual representation of the support for each hypothesis, dark gray in favor of H1 and white in favor of H0. While there is clearly more support for H0 there is still some support for H1, hence, we have only moderate evidence for H0, according to the benchmark in Table 1. The two distributions plotted represent the prior (dotted line) and posterior (solid line) probabilities and the 95% credible interval is marked as a horizontal whisker plot at the plot above them. The center of the posterior is close to the middle of the prior telling us that the data indicate there is only a small effect. Furthermore, the 95% credible intervals (analogous to frequentist confidence intervals) cross zero, again indicating no significant difference or effect. The top-right panel is a sensitivity analysis of the choice of prior. The line represents the value of the BF (y-axis) when the prior (x-axis) varies. This plot shows that even if our prior were narrower e.g., a Cauchy prior (0, 0.350), representing a significantly stronger belief in a smaller effect size, there would still be moderate evidence for H0. The bottom two panels reveal the same patterns for the Score: Total variable. There is strong support for H1 in the proportion wheel and the distance between the prior and the posterior distributions in the bottom-left panel. Additionally, as the bottom-left panel indicates, there would still be strong evidence for a difference between T1 and T2, unless we had a very narrow prior.
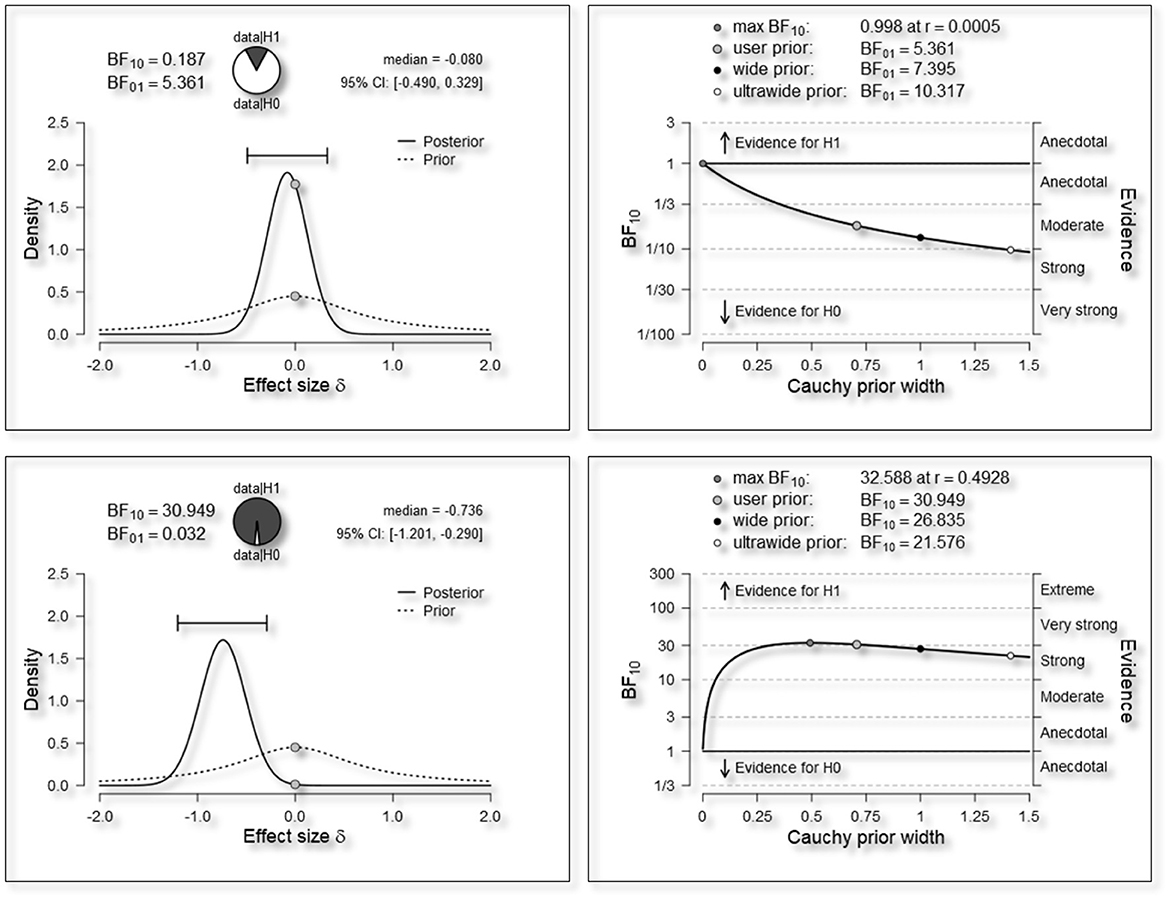
Figure 4. Example test information and robustness analysis plots. Top-left (A)—Prior and posterior mass plots Action: addition, Top-right (B)—Robustness analysis Action: addition, Bottom-left (C)—Prior and posterior mass plots Score: total, Bottom-right (D)—Robustness analysis Score: total.
Table 4 gives the consolidated output from both Bayesian and frequentist procedures. Interpreting the p-values, we have no statistically significant findings, aside from the difference in Scores: Total between T1 and T2 (p < 0.001). Interpreting the BFs gives more information. There is very strong evidence for H1 on Score: Total, inconclusive evidence for either H1 or H0 on Domain: Sentence_or_Above, and, importantly moderate evidence for H0 on all other variables. In general, on the keystroke-logged variables, we see moderate evidence that there is no difference between T1 and T2.
5. Discussion and conclusion
In this study, we explored changes in revision behavior, as measured by keystroke logging over the course of an EAP program, using BHT. Our research question “How does revision behavior, as measured by keystroke-logging, change over the course of a one-month intensive EAP program?” was related to substantive changes in revision behavior. Our general expectation was that, given the participants had undertaken an intensive four-week course on academic writing, they would be more skilled writers at T2 than at T1, and we would see differences between the more- and less-skilled writers, in line with those we see in the literature (e.g., Faigley and Witte, 1981; Zamel, 1983; Roca de Larios et al., 2008; Manchón et al., 2009) thought to be due to differences in working memory capacity and automatization (Alamargot and Chanquoy, 2001; Stevenson et al., 2006; Chanquoy, 2009; Barkaoui, 2016). We found moderate evidence against there being differences from T1-T2 on all measures but one (sentence or above level revisions) and, on that measure, the evidence was inconclusive. In contrast with the findings of Roca de Larios et al. (2008), we found anecdotal evidence of writers at T2 not engaging more with revisions in the final stages (third tercile of writing time) than they did at T1. We found anecdotal evidence of no difference in the proportions of higher-level (i.e., content, balance) and lower-level (i.e., language, typography) revisions as suggested by Manchón et al. (2009), Barkaoui (2016) and Stevenson et al. (2006). We found anecdotal evidence of participants not differing in the numbers of overall revisions made before and after the EAP course in contrast to Choi (2007). In summary, we mostly found evidence against the differences expounded between higher- and lower-skilled writing from the literature.
It is curious that very strong evidence is seen of improvement in IELTS rating between T1 and T2, showing a probable increase in writing skill. It is important to remember that revision strategies were not explicitly taught on the course. It seems likely that the improvements in the participants' written language were unrelated to their revision behavior. For example, on the EAP course, participants were taught to appropriately address the task, using academic vocabulary, cohesive devices, etc. Tentatively, it could be concluded that 4 weeks may not have been enough time to have developed significant levels of automaticity in language production and released additional working memory capacity to be used in revision.
The use of BHT in this exploratory study has allowed us to make more of our data and provide more detailed recommendations for follow up studies that we would have been able to do under the frequentist paradigm. Our first stated advantage of BHT, the fact that it can provide evidence for the null hypothesis means that we can recommend that follow up studies are unlikely to be successful using the same experimental parameters as ours. In other words, researchers looking at changes in revision behavior in the future should, for example, use a different set of outcome measures and/or increase the time between data collections to allow time for more writing development. Replication of our study would likely be futile. Had we relied on p-values alone, we would have only been able to conclude that our sample size was not sufficient to capture differences in revision behavior. Our second stated advantage of BHT, nuanced levels of strength of evidence rather than a binary outcome, also allows us to accept the anecdotal levels of evidence that lead to that conclusion that following up on this exploratory study with the same design, over the same timeframe, in a similar context and with a comparable population, would be unlikely to show changes in revision behavior. In a frequentist framework, anecdotal evidence, most likely to be supplied from small sample size exploratory studies, is not usually considered sufficient or acted upon. Our final stated advantage of BHT, the possibility to carry data over from an exploratory study to a confirmatory study, encodes as a prior, does not really apply to this study as we found no differences in revision behaviors between T1 and T2, and we are not planning to take any of the results forward to a confirmatory study. In summary, our exploration led to the conclusion that looking for differences in revision behavior in periods of only a few weeks—where revision skills have not been explicitly taught—is likely to be futile, and other researchers should probably not try to do this in the future.
Recent work has been undertaken on the construction and validation of automatic extraction of revision tag sets from keystroke logging data (Conijn et al., 2020, 2021, 2022). The analyses reported above were done before this exciting methodology was available, and this should be viewed as a limitation of this paper. Future work on this dataset will involve re-analyzing the data using the methods of Conijn et al. (2020, 2021, 2022) and reexamining the results compared to those from the Barkaoui (2016) revision taxonomy.
To conclude, we found evidence against there being changes in revision behaviors over the four weeks of the EAP course, as reported on in this study. These results may indicate that salient changes in revision behaviors may take more than 1 month to manifest, and future studies exploring these changes should use a substantially longer time frame, and perhaps more modern methods of categorizing revision behaviors (Conijn et al., 2020, 2021, 2022). The use of BHT to analyze and communicate the results of exploratory studies allowed us to infer substantially more from of our data and make more nuanced recommendations than we could do with frequentist methods.
Data availability statement
The raw data supporting the conclusions of this article will be made available by the authors, without undue reservation.
Ethics statement
The studies involving human participants were reviewed and approved by FASS-LUMS Research Ethics Committee, Lancaster University. The patients/participants provided their written informed consent to participate in this study.
Author contributions
DM contributed to the conceptualization, data collection and writing of this study. GM Contributed to the analysis and writing of this study. Both authors contributed to the article and approved the submitted version.
Funding
The funding for data collection was provided by Lancaster University and the funding for writing up the paper by the University of Leeds.
Conflict of interest
The authors declare that the research was conducted in the absence of any commercial or financial relationships that could be construed as a potential conflict of interest.
Publisher's note
All claims expressed in this article are solely those of the authors and do not necessarily represent those of their affiliated organizations, or those of the publisher, the editors and the reviewers. Any product that may be evaluated in this article, or claim that may be made by its manufacturer, is not guaranteed or endorsed by the publisher.
Footnotes
1. ^Norouzian et al. (2018b) online app: (https://rnorouzian.shinyapps.io/bayesian-t-tests/) could also have been used for our purposes.
2. ^https://takeielts.britishcouncil.org/sites/default/files/2018-01/IELTS_task_2_Writing_band_descriptors.pdf (accessed April 2022).
3. ^By convention many statistical distributions are expressed in the format: name (location, scale), where name is the name of the distribution, location is an indication of where it sits on a scale (e.g., for the normal distribution the Mean), and scale is some measure of spread around the location (e.g., for the normal distribution SD).
4. ^See Norouzian et al. (2018b) and Wagenmakers et al. (2018a) for justifications and further explanation of this prior.
References
Alamargot, D., and Chanquoy, L. (2001). Through the Models of Writing. Dordrecht-Boston-London: Kluwer Academic Publishers. doi: 10.1007/978-94-010-0804-4
Barkaoui, K. (2007). Revision in second language writing: what teachers need to know. TESL Canada J. 25, 81–92. doi: 10.18806/tesl.v25i1.109
Barkaoui, K. (2016). What and when second-language learners revise when responding to timed writing tasks on the computer : the roles of task type, second language proficiency, and keyboarding skills. The Modern Language J. 100, 320–340. doi: 10.1111/modl.12316
Bereiter, C., and Scardamalia, M. (1987). The Psychology of Written Composition. Hillsdale, NJ: Lawrence Erlbaum.
Breuer, E. O. (2017). Revision processes in first language and foreign language writing: differences and similarities in the success of revision processes. J. Acad. Writing. 7, 27–42. doi: 10.18552/joaw.v7i1.214
Chanquoy, L. (2009). “Revision processes,” in The SAGE Handbook of Writing Development (Los Angeles, CA: SAGE). p. 89–97. doi: 10.4135/9780857021069.n6
Chenoweth, N. A., and Hayes, J. R. (2001). Fluency in writing: generating text in L1 and L2. Written Commun. 18, 80–98. doi: 10.1177/0741088301018001004
Choi, Y. H. (2007). On-line revision behaviors in EFL writing process. English Teach. 62, 69–93. doi: 10.15858/engtea.62.4.200712.69
Conijn, R., Dux Speltz, E., and Chukharev-Hudilainen, E. (2021). Automated extraction of revision events from keystroke data. Read. Writ. 1–26. doi: 10.1007/s11145-021-10222-w
Conijn, R., Dux Speltz, E., Van Zaanen, M., Van Waes, L., and Chukharev-Hudilainen, E. (2020). “A process-oriented dataset of revisions during writing,” in Proceedings of the 12th Language Resources and Evaluation Conference (pp. 363–368). doi: 10.31234/osf.io/h25ak
Conijn, R., Dux Speltz, E., Zaanen, M. V., Waes, L. V., and Chukharev-Hudilainen, E. (2022). A product-and process-oriented tagset for revisions in writing. Written Commun. 39, 97–128. doi: 10.1177/07410883211052104
Council of Europe. (2001). The Common European Framework of Reference for Languages: Learning, Teaching, Assessment. Cambridge: Cambridge University Press.
Faigley, L., and Witte, S. (1981). Analyzing revision. College Compos. Commun. 32, 400–407. doi: 10.2307/356602
Fitzgerald, J. (1987). Research on revision in writing. Rev. Edu. Res. 57, 481–506. doi: 10.3102/00346543057004481
Fox, J., and Weisberg, S. (2011). An R Companion to Applied Regression Second Edition. Thousand Oaks CA: Sage.
Hayes, J. R. (1996). “A new framework for understanding cognition and affect in writing,” in C. M. Levy and S. Ransdell, eds The Science of Writing: Theories, Methods, Individual Differences, and Application (Mahwah, NJ: Lawrence Erlbaum Associates). p. 1–55.
Hayes, J. R. (2012). Modeling and remodeling writing. Written Commun. 29, 369–388. doi: 10.1177/0741088312451260
Hayes, J. R., and Flower, L. S. (1980). “Identifying the organization of writing processes,” in Cognitive Processes in Writing (Hillsdale, NJ: Lawrence Erlbaum). p. 3–30.
Kline, R. B. (2004). Beyond significance testing: Reforming Data Analysis Methods in Behavioral Research 1st ed. Washington, DC.: American Psychological Association. doi: 10.1037/10693-000
Lee, M. D., and Wagenmakers, E. J. (2013). Bayesian Cognitive Modeling: A Practical Course. Cambridge: Cambridge University Press. doi: 10.1017/CBO9781139087759
Leijten, M., and Van Waes, L. (2006). “Inputlog: new perspectives on the logging of on-line writing,” in K. P. H. Sullivan and E. Lindgren, eds Computer Key-stroke Logging and Writing: Methods and Applications (Oxford, UK: Elsevier). p. 1873–94.
Leijten, M., and Van Waes, L. (2013). Keystroke logging in writing research: using Inputlog to analyze and visualize writing processes. Written Commun. 30, 358–392. doi: 10.1177/0741088313491692
Lindgren, E., and Sullivan, K. P. H. (2006). “Analysing online revision,” in Computer Key-stroke Logging: Methods and Applications (Oxford, UK: Elsevier). p.157–188. doi: 10.1163/9780080460932_010
Manchón, R. M., de Larios, J., and Murphy, R. (2009). “The temporal dimension and problem-solving nature of foreign language composing. Implications for theory,” in R. M. Manchón, ed. Writing in Foreign Language Contexts. Learning, Teaching and Research (Clevedon: Multilingual Matters). p. 102–129. doi: 10.21832/9781847691859-008
Marsden, E., Morgan-Short, K., Trofimovic, P., and Ellis, N. C. (2018). Introducing registered reports at language learning: promoting transparency, replication, and a synthetic ethic in the language sciences. Language Learn. 68, 309–320. doi: 10.1111/lang.12284
Mazgutova, D. (2020). Changes in revision behaviours of L2 writers in an intensive english for academic purposes program. Int. J. Edu. Methodol. 6, 715–727. doi: 10.12973/ijem.6.4.715
Mazgutova, D., and Kormos, J. (2015). Syntactic and lexical development in an intensive english for academic purposes programme. J. Second Language Writ. 29, 3–15. doi: 10.1016/j.jslw.2015.06.004
Norouzian, R., de Miranda, M., and Plonsky, M. A. (2018a). A Bayesian approach to measuring evidence in L2 research: an empirical investigation. Modern Language J. 103, 248–261. doi: 10.1111/modl.12543
Norouzian, R., de Miranda, M., and Plonsky, M. A. (2018b). The Bayesian revolution in L2 research : an applied approach. Language Learn. 68, 1032–1075. doi: 10.1111/lang.12310
Oswald, F. L., and Plonsky, L. (2010). Meta-analysis in second language research: choices and challenges. Anual Rev. Appl. Linguistics 30, 85–110. doi: 10.1017/S0267190510000115
Révész, A., Kourtali, N., and Mazgutova, D. (2017). Effects of task complexity on L2 writing behaviors and linguistic complexity. Language Learn. 67, 208–241. doi: 10.1111/lang.12205
Roca de Larios, J., Manchón, R., Murphy, L., and Marin, J. (2008). The foreign language writer's strategic behaviour in the allocation of time to writing processes. J. Second Language Writ. 17, 30–47. doi: 10.1016/j.jslw.2007.08.005
Stevenson, M., Schoonen, R., and de Glopper, K. (2006). Revising in two languages: A multi-dimensional comparison of online writing revisions in L1 and FL. J. Second Language Writ. 15, 201–233. doi: 10.1016/j.jslw.2006.06.002
Strömqvist, S., Holmqvist, K., Johansson, V., Karlsson, H., and Wengelin, Å. (2006). “What keystroke logging can reveal about writing,” in K.P.H. Sullivan; E. Lindgren, eds Computer Key-stroke Logging and Writing: Methods and Applications (Amsterdam: Elsevier). p. 45–71. doi: 10.1163/9780080460932_005
Sun, S., and Fan, X. (2010). Effect size reporting practices in communication research. Commun. Methods Measures 4, 989–1004. doi: 10.1080/19312458.2010.527875
Thorson, H. (2000). Using the computer to compare foreign and native language writing processes: a statistical and case study approach. Modern Language J. 84, 155–170. doi: 10.1111/0026-7902.00059
Van Waes, L., Leijten, M., Roeser, J., Olive, T., and Grabowski, J. (2021). Measuring and assessing typing skills in writing research. J. Writ. Res. 13, 107–153. doi: 10.17239/jowr-2021.13.01.04
Van Waes, L., Leijten, M., and Van Weijen, D. (2009). Keystroke logging in writing research: observing writing processes with Inputlog. German Foreign Language 2, 41–64.
Wagenmakers, E., Love, J., Marsman, M., Jamil, T., Ly, A., Verhagen, J., et al. (2018a). Bayesian inference for psychology. Part II : Example applications with JASP. Psychonomic Bull. Rev. 25, 58–76. doi: 10.3758/s13423-017-1323-7
Wagenmakers, E., Marsman, M., Jamil, T., Alexander, L., Verhagen, J., Jonathon, L., et al. (2018b). Bayesian inference for psychology. Part I : theoretical advantages and practical ramifications. Psychonomic Bull. Rev. 25,35–57. doi: 10.3758/s13423-017-1343-3
Wagenmakers, E., Wetzels, R., Borsboom, D., Maas, H. L., Van Der, J., and Kievit, R. A. (2012). An Agenda for purely confirmatory research. Perspect. Psychol. Sci. 6, 632–638. doi: 10.1177/1745691612463078
Wei, R., Hu, Y., and Xiong, J. (2019). Effect size reporting practices in applied linguistics research: a study of one major journal. SAGE Open 9, 1–11. doi: 10.1177/2158244019850035
Wen, Z., Mota, M. B., and McNeill, A. (2015). Working Memory in Second Language Acquisition and Processing (Vol. 87). Bristol: Multilingual Matters. doi: 10.21832/9781783093595
Zamel, V. (1982). Writing: the process of discovering meaning. TESOL Q. 16, 195–209. doi: 10.2307/3586792
Keywords: writing, emerging technologies, digital writing, writing development, teaching and learning writing, Bayesian, exploratory studies, study design
Citation: Mazgutova D and McCray G (2023) An exploratory analysis of revision behavior development of L2 writers on an intensive English for academic purposes program using Bayesian methods. Front. Commun. 7:934583. doi: 10.3389/fcomm.2022.934583
Received: 02 May 2022; Accepted: 23 December 2022;
Published: 09 January 2023.
Edited by:
Aysel Saricaoglu, Social Sciences University of Ankara, TürkiyeReviewed by:
Julie Aydinli, Social Sciences University of Ankara, TürkiyeNadezhda Dobrynina, Iowa State University, United States
Copyright © 2023 Mazgutova and McCray. This is an open-access article distributed under the terms of the Creative Commons Attribution License (CC BY). The use, distribution or reproduction in other forums is permitted, provided the original author(s) and the copyright owner(s) are credited and that the original publication in this journal is cited, in accordance with accepted academic practice. No use, distribution or reproduction is permitted which does not comply with these terms.
*Correspondence: Diana Mazgutova, ZC5tYXpndXRvdmEmI3gwMDA0MDtsZWVkcy5hYy51aw==