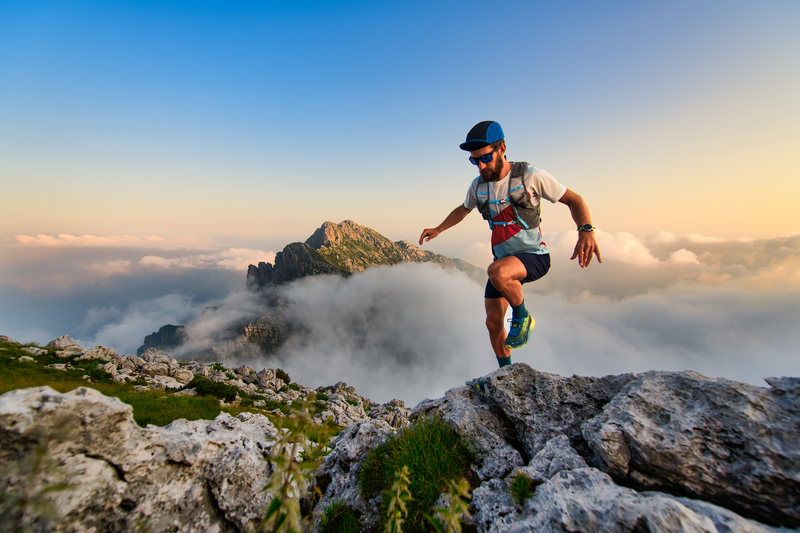
94% of researchers rate our articles as excellent or good
Learn more about the work of our research integrity team to safeguard the quality of each article we publish.
Find out more
ORIGINAL RESEARCH article
Front. Commun. , 25 March 2022
Sec. Psychology of Language
Volume 7 - 2022 | https://doi.org/10.3389/fcomm.2022.839415
This article is part of the Research Topic Fuzzy Boundaries: Ambiguity in Speech Production and Comprehension View all 12 articles
Inappropriate gaps between syllables are one of the core diagnostic features of both childhood apraxia of speech and acquired apraxia of speech. However, little is known about how listeners perceive and identify inappropriate pauses between syllables (gap detection). Only one previous study has investigated the perception of inappropriate pauses between syllables in typical adult speakers and no investigations of gap detection in children's speech have been undertaken. The purpose of this research was to explore the boundaries of listener gap detection to determine at which gap length (duration) a listener can perceive that an inappropriate pause is present in child speech. Listener perception of between-syllable gaps was explored in an experimental design study using the online survey platform Qualtrics. Speech samples were collected from two typically developing children and digitally manipulated to insert gaps between syllables. Adult listeners (n = 84) were recruited and could accurately detect segregation on 80% of presentations at a duration between 100 and 125 ms and could accurately detect segregation on 90% of presentations at a duration between 125 and 150 ms. Listener musical training, gender and age were not correlated with accuracy of detection, but speech pathology training was, albeit weakly. Male speaker gender, and strong onset syllable stress were correlated with increased accuracy compared to female speaker gender and weak onset syllable stress in some gap conditions. The results contribute to our understanding of speech acceptability in CAS and other prosodic disorders and moves towards developing standardised criteria for rating syllable segregation. There may also be implications for computer and artificial intelligence understanding of child speech and automatic detection of disordered speech based on between syllable segregation.
Childhood Apraxia of Speech (CAS) is “a neurological childhood speech sound disorder in which the precision and consistency of movements underlying speech are impaired in the absence of neuromuscular deficits” (American Speech-Language-Hearing Association, 2007). These difficulties in planning and sequencing speech movements result in decreases in the precision, consistency, and intelligibility of speech.
This core deficit of motor planning can be identified by observable speech behaviours, including ‘inconsistent errors on consonants and vowels on repeated productions of syllables and words, lengthened and disrupted coarticulatory transitions between sounds and syllables, and inappropriate prosody particularly in lexical or phrasal stress' (American Speech-Language-Hearing Association, 2007, Definitions of CAS section, para 2.). CAS is thought to have a genetic origin (e.g., Fedorenko et al., 2016), and many single genes have been implicated as causal (Hildebrand et al., 2020) however to date, idiopathic cases predominate. The gold standard of CAS diagnosis in clinical practise is the judgement of perceptual speech features including inappropriate pauses or gaps on transitions between sounds or syllables judged by expert listeners (Murray et al., 2015).
Syllable segregation occurs within a word when the movement from one syllable to the next is disrupted by an inappropriate pause (Brown et al., 2018). Syllable segregation is a hallmark diagnostic feature of CAS representing the reported difficulty transitioning between syllables. Syllable segregation was identified by Murray et al. (2015) as a key symptom of CAS diagnosis, along with poor lexical stress matches, reduced percentage phonemes correct in polysyllabic words, and reduced articulatory accuracy on repetition of a diadochokinetic speech task. Syllable segregation is therefore both a key identifying feature in CAS, and important in differential diagnosis of CAS from other speech disorders (Murray et al., 2015).
Despite the significance of syllable segregation as a diagnostic feature of CAS, there has been little examination of the perceptual characteristics of between-syllable segregation in the speech of children. There are currently no accepted criteria against which to rate segregation (Brown et al., 2018), and there is little research literature regarding how between-syllable segregation in children's speech is perceived by listeners. One study (Shriberg et al., 2017) investigated between-word segregation, however within-word segregation may be a more valuable diagnostic tool, particularly in minimally verbal children. Reporting of segregated speech currently relies on perceptual judgement and there is no existing standard value for the duration of between-syllable segregation which would be considered disordered. In order to know what is perceived to be distorted or disordered, we must first know what is typical. It is therefore important to understand the perception of syllable segregation in the speech of typically developing children as a potential standard from which we can determine disordered production.
Previous research exploring perception of between-syllable pauses has primarily focused on “gap detection,” which refers to a listener's ability to detect a noiseless temporal gap between two stimuli (e.g., Mishra et al., 2014). Research has typically focused on either “within-channel” gap detection where the non-speech sounds on the boundary of the gap are spectrally symmetrical, or “between-channel,” where the non-speech sounds bordering the gap are spectrally asymmetrical and therefore more closely resemble speech signals. Gap detection thresholds within the literature vary with stimuli and listener. For example, Heldner (2011) reported that the gap detection threshold varied from 58 to 204 ms.
One study has investigated adult perception of syllable segregation per se. Brown et al. (2018) investigated perception of syllable segregation in adult speech and found that the perceptual limen of syllable segregation for adult listeners when listening to words with inserted gaps created from ambient noise was 80 ms at an 80% accuracy threshold. In Brown's study, a fixed anchor method was used in which an anchor stimulus with no manipulated gap and a stimulus with the artificial gap were presented in series. Participants judged the second stimulus as to whether they could hear a gap within the word. This was a type of modified just noticeable difference (JND). Full JND was not used in Brown's study for pragmatic reasons, that is, to reduce the number of presentations. Full JND would have taken 475 presentations, significantly greater than the 80 presentations used. Such a JND approach was therefore not used to answer the fundamental question of the research which was to establish the level at which any segregation is perceived by the majority of listeners. This level is known as the perceptual limen of syllable segregation.
Importantly for CAS diagnosis, the perceptual limen of syllable segregation in typically developing children's speech has not yet been studied. There are known suprasegmental differences between adult and child speech (e.g., Lee et al., 1999), which may result in a higher limen of perception for syllable segregation. Child and adult speech differ significantly in the following ways: children's speech is characterised by higher fundamental and secondary formant frequencies, increased duration of fricative consonant length, higher consonant-vowel duration ratios, and more similar spectral characteristics of different phones than adult productions of the same sounds (Gerosa et al., 2006). Children's speech is also slower, with the movement of articulators less coordinated than in adult speech (e.g., Cychosz et al., 2019), and children produce more consonant distortions as part of typical development (e.g., Storkel, 2019). Similarly, durational variability for children's speech is greater than adult's speech, converging to adult levels around age 13 years (Gerosa et al., 2006). These features may contribute to a lengthened perceptual limen for between-syllable segregation compared to perception of the same phenomena in adult speakers.
Musical training, speech pathology training, age and gender have been identified as factors which may impact perception of auditory features (Pichora-Fuller et al., 2006; Giannela-Samelli and Schochat, 2008; Mishra et al., 2014; Brown et al., 2018). Musicians have significantly lower between-channel gap detection thresholds compared to non-musicians (Mishra et al., 2014; Elangovan et al., 2016), with one study finding that between channel gap detection thresholds in musicians were on average half those in non-musicians (Mishra et al., 2014). However, it is important to note that within-channel gap detection stimuli do not fully represent the complexity of speech sound signals and therefore cannot be readily generalised to the perception of between-syllable segregation (Brown et al., 2018). Only one study has examined differences in accuracy of gap detection resulting from speech pathology training. This study compared accuracy between untrained listeners and experienced speech pathologists rating the presence of syllable segregation and found a difference in accuracy of identification at the 90% accuracy threshold (Brown et al., 2018). Younger age is also correlated with increased accuracy of gap detection (Pichora-Fuller et al., 2006). Gap detection thresholds have been found to be greater for older listeners (67–82 years old, mean 75 years) than for younger listeners (21–35 years old, mean 24 years) (Pichora-Fuller et al., 2006). Few studies have examined the relationship between listener gender and accuracy of gap detection. One study reported males performed slightly better in the gaps-in-noise test, which uses white noise as a stimulus (Giannela-Samelli and Schochat, 2008) and is therefore of limited utility to between-syllable gap detection.
There is also limited previous research regarding stimuli factors which may influence perception of auditory features. Speaker gender has been identified as a factor which may influence perception. Existing research suggests that female speakers may be overall more intelligible than male speakers (Markham and Hazan, 2004; Yoho et al., 2018) in both subjective and objective measures, however no existing research has investigated the interaction of gender and perception of syllable segregation. Similarly, stress pattern of spoken stimuli may influence perception, although there is limited research investigating syllable stress pattern and perception of syllable segregation. One previous study (Brown et al., 2018) found that syllable stress pattern was weakly correlated with accuracy of gap detection. These factors therefore warrant further investigation.
Despite the known differences in production between adult and child speakers, no comparison between what is acceptable in adult and child speech has been undertaken regarding within word pauses. That is, it is unknown whether the limen of perception in child speech is similar to that reported for adult speakers or not. Such a comparison may provide valuable information for speech pathologists in working with children with CAS including assisting in identifying the need to train listeners to what is typical in child speech for therapy accuracy. Additionally, it may assist in the design of speech recognition systems, which are largely trained on adult speech (Shahin et al., 2020). These systems have shown a substantial degradation in performance when tested on child speech, due to the linguistic and acoustic mismatches outlined above (Shahin et al., 2020). There is therefore a gap in the existing literature regarding the differences in the perceptual limen of adult speech compared to child speech.
Non-words may be most appropriate to investigate listeners' perception of syllable segregation for multiple reasons, including that a listeners' pre-existing idea of words' pronunciation may cause potential confounds with their perception of the word (Gierut et al., 2010) and non-words separate perception from any semantic context. Importantly, previous research in detection of syllable segregation used non-words to investigate listener perception (Brown et al., 2018).
Despite syllable segregation being a diagnostic feature of CAS, understanding the duration of the gap between syllables is an emerging field. If a value for the perceptual limen of between-syllable gaps is identified, this may be used to contribute to the development of standardised training and rating tools which could be used in both diagnosis and treatment of CAS. The purpose of this study was therefore to explore the perceptual boundaries of adult listeners when judging artificial syllable segregation in the speech of typically developing children.
1a. What is the threshold for accurate detection of a between-channel gap within non-words?
1b. What is the strength of relationship between gap duration and between-channel gap detection accuracy?
2. Do stimulus factors impact the listener perceptual limen of between-syllable segregation?
a. Do non-words with a strong onset syllable stress pattern have a shorter perceptual limen than weak onset syllable stress patterns?
b. Does speaker gender affect the perceptual limen of syllable segregation?
3. Do listener factors impact the listener perceptual limen of between-syllable segregation?
a. Do listeners with musical training have a shorter perceptual limen compared to listeners without musical training?
b. Do listeners with speech pathology training have a shorter perceptual limen compared to listeners without speech pathology training?
c. Do younger listeners have a shorter perceptual limen compared to older listeners?
d. Does listener gender affect the perceptual limen of syllable segregation?
This study used a cross sectional experimental design using the online platform Qualtrics (Qualtrics, 2021). The research was approved by The University of Sydney Human Research Ethics Committee (2021/753). There were two groups of participants involved in this study—child speaker participants (hereafter referred to as “speakers”) and adult listener participants (hereafter “listeners”). All participants gave informed consent to participate.
To be considered eligible for this study, children were required to speak English with an Australian accent, have hearing within the normal range, have typically developing speech and language, and no structural or neuromuscular deficits as determined by an oral-musculature assessment completed by an experienced qualified speech-language pathologist (the second author). Two children were recruited and parents provided written consent. Speakers were therefore 1 male child and 1 female child, aged 10 and 8 respectively.
Following Brown et al. (2018), a set of 4 non-words from the Syllable Repetition Task (Shriberg et al., 2009) (ma'da, 'maba, da'ba, 'bada) were selected as target productions. These words were chosen as they were two syllable words suitable for acoustic manipulation which contained a variety of stress patterns including two strong onset words ('bada, 'maba) and two weak onset words (da'ba, ma'da) to examine any differences in listener perception as a result of stress pattern.
Single word utterances were used in preference to connected speech to reduce the influence of other words in the utterance on the listener's perception of a word. Two syllable non-words were used to ensure comparability of results with previous research (Brown et al., 2018).
Speakers were asked to imitate an adult female (second author) saying the stimuli. Samples were recorded using Audacity 2.4.2® (Audacity Team, 2021) in a quiet space using a head mounted AKG microphone at a mouth to microphone distance of 5 cm and a Roland Quad Capture sound card attached to a laptop computer. No audible distortions were found in the stimuli when reviewed.
Sample preparation followed Brown et al. (2018). Samples were edited using Audacity 2.4.2® software (Audacity Team, 2021). All samples were normalised to −1.0 dB to ensure volume was consistent across samples and the “noise reduction” feature in Audacity was applied to remove background noises or distortions in the clip that could interfere with a listener's perception of the recording. The inserted recorded gap was copied from periods of ambient sound in the clip instead of pure silence which, if used, may have resulted in detectable sound distortions. Gaps of the selected ambient sound, ranging in duration from 25 to 200 ms, were then inserted into the single word samples. Gaps were inserted at the pre-voice onset pause between the first and second syllable of the four non-words.
Two small pilot studies (total n = 7) were initially conducted with gaps of 50 ms increments (50–200 ms) based on previously reported gap detection research (Brown et al., 2018). All listeners were able to detect segregation at 200 ms. The pilot results suggested that the 80% accuracy threshold was at least 100 ms and no higher than 150 ms, and the 90% accuracy threshold at least 150 ms and no higher than 200 ms, indicating the need for smaller increments to reliably determine the limen of perception as well as the need for a gap condition of 175 ms. These findings combined with prior research regarding gap detection (Brown et al., 2018) indicated 25 ms was the most appropriate gap increment. An upper limit of 200 ms was therefore chosen as a gap all listeners should be able to detect reliably. A total of nine gap conditions were therefore used (1) no gap, (2) 25 ms gap, (3) 50 ms, (4) 75 ms, (5) 100 ms, (6) 125 ms, (7) 150 ms, (8) 175 ms, and (9) 200 ms. Pilot participants did not participate in the primary study.
Listeners were then recruited to judge the stimuli. Listeners were required to be between 18 and 59 years of age. This age range was selected to reduce the impact of presbycusis and age-related cognitive decline. Listeners were required to have no current or previous history of hearing loss, no self-reported current ear infection, no self-reported current or history of cognitive impairment, and to be an Australian English speaker. All listeners were asked to undertake a hearing screen using Hearing Australia Online Hearing Assessment (Hearing Australia, 2021) and self-report a result within the normal range. Listeners were recruited via social media, word of mouth, and advertising within The University of Sydney.
A total of 140 listeners aged 18–59 consented to participate in the study. No identifying information was collected about listeners.
Some 49 listeners started the survey but did not complete any listening tasks. These listeners were removed from the data set. Three (3) listeners who answered either “yes, segregated,” or “no, not segregated” to all questions were removed from the data set. Three (3) listeners who only answered one question were removed. One listener achieved a mean score of 29.1% compared to the mean of all listeners, which was 69.5%. The apparent difficulty this listener had with the task suggested they may not actually meet the inclusion criteria, and so they were removed from the data set. A total of 56 listeners were therefore removed from the data set without analysis. Five (5) listeners partially completed the listening tasks but failed to complete the entire study. These listener responses were included in the data analysis and consequently some analyses have varying participant numbers.
A total 84 listeners (61 women, 22 men, 1 other) were therefore included in the data analysis. The mean age was 28.4 years (SD 11.3; range 18–59). Nineteen (19) listeners indicated that they had received musical training, which was defined as either having received musical training within the previous 5 years or practising as a professional musician, and 46 had received speech pathology training. Speech pathology training was defined as a listener being either a qualified speech pathologist or a speech pathology student. Of these, 42 were speech pathology students and 4 were qualified speech pathologists. Demographic data regarding age, speech pathology training, musical training, listener gender was collected and is reported in Table 1.
A set of 80 (4 stimuli x 9 gap conditions x 2 speaker genders + 10% repeats) were played in two randomised orders. All modified words spoken by the male child were placed in a random order block and all modified words spoken by the female child were similarly blocked. The order of the two blocks was switched halfway through the data collection period to reduce any order effect associated with the gender of the speaker. Participants were asked to respond to “Indicate if you did hear segregation or did not hear segregation.” Binary choice answer options were “yes, segregated” and “no, not segregated.” Binary choice has been found to reduce bias in ratings (Harvey, 2016). No feedback was provided.
To answer research question 1a, the percentage of stimuli detected accurately for each gap condition was calculated across all listeners and graphed, to indicate trends by gap condition. The limen of perception for listeners was marked at both 80 and 90% accuracy thresholds, to include both accuracy thresholds used in syllable segregation research previously (Brown et al., 2018).
A second measure of accuracy was used to answer research questions 1b, 2a, and 2b. Because participants made eight responses for each gap condition (four words by two speaker genders), it was possible to calculate a proportion of correct responses at each gap condition. The 0 ms gap (control) condition was excluded in statistical analyses, to investigate only perception of inserted gaps. Kolmogorov-Smirnov tests of normality (Chakravarti et al., 1967) showed distributions were heavily skewed for some gap durations. The design was repeated measures because participants had accuracy scores for all gap conditions. The non-parametric Friedman test (Friedman, 1937) tested equality of median accuracy across gap durations. Strength of relationship between accuracy and gap condition was calculated by converting the Friedman p value to a correlation r value. The conversion was done by finding the z score on the standard normal distribution which corresponded to the Friedman p value, then applying the formula r = |z|/√n (Ratner, 2009).
To address research questions 2a and 2b, the relationships between the stimulus factors (word stress pattern and speaker gender) and accuracy, accuracy was first graphed to show gap durations with separations in accuracy for strong/weak onset words vs. weak/strong onset words, and between female and male speakers. Differences at these gap durations were then analysed using Wilcoxon signed-rank tests (Wilcoxon, 1945). The test statistics were converted to correlation r values, as an effect size index, using the formula r = |z|/√n.
To address the listener factor research questions 3a to 3d, accuracy across all 8 gap conditions was averaged for each listener and then correlated with the dichotomous listener factors using parametric point biserial correlations (rpb) (Cureton, 1956). Kolmogarov-Smirnov tests indicated average accuracy was normally distributed, meaning parametric tests could be used. Listener age was grouped into (1) younger listeners (aged 18–32; 77.4% of listeners) and (2) older listeners (aged 37–59, 22.6% of listeners). These age bands were selected as this was where the data showed a natural break in age distribution.
Supplementary analysis of inter-rater reliability used intraclass correlation coefficients, two-way random with absolute agreement (ICC 2,1) across the average accuracy scores of all 83 raters who rated all gap conditions (one rater did not rate all gap conditions) (Bartko, 1966). ICC values between 0.5 and 0.75 indicated moderate reliability, values between 0.75 and 0.9 indicated good reliability and values >0.9 indicated excellent reliability (Koo and Li, 2016).
Intra-rater reliability of responses was analysed using Cohen's Kappa (Cohen, 1960) due to the binary data collected. A result of >0.8 indicated very good agreement; 0.61–0.8 good agreement; 0.41–0.60 moderate agreement; 0.21–0.40 fair agreement and <0.20 poor agreement (Landis and Koch, 1977; Altman, 1991).
A post hoc analysis was conducted to compare the limen of perception in child speech and the limen of perception reported in adult speech (Brown et al., 2018). An individual participant data meta-analysis was conducted to determine the accuracy of listener detection of syllable segregation at each gap duration using raw data obtained from Brown et al. (2018) and the data included in this study. Wilcoxon Rank Sum tests were used to compare accuracy of listeners when listening to adult speech, as in data collected by Brown and colleagues, and when listening to child speech, as collected by the present study. Non-parametric point biserial correlations were used to measure the strength of these differences.
Analyses were conducted using SPSS Version 27.0 (IBM Corp, 2020) and R version 3.1.1 (R Core Team, 2021). For all correlation effect sizes, a small effect was indicated by an r between 0.1 and 0.3, a medium effect between 0.3 and 0.5 and a large effect by >0.5 (Fritz et al., 2011).
Across all listening tasks listeners achieved 60.1% “accurate yes”; 9.5% “accurate no,” 0.6% “inaccurate yes”; and 29.7% “inaccurate no.” Figure 1 shows the mean 80 and 90% accuracy thresholds across all listener groups.
The listener limen of perception at 80% accuracy was at least 100 ms and no higher than 125 ms. At 90% accuracy, the limen of perception was at least 125 ms and no higher than 150 ms. Within all sub-groups of listeners, the limen of perception at 80% accuracy was also at least 100 ms and no higher than 125 ms. At the 90% accuracy thresholds, sub-groups differed in their limen of perception. Table 2 outlines the 80 and 90% accuracy thresholds for each sub-group of listeners. Figure 2 shows the proportion of accurate gap detections by gap duration.
A Friedman's test with follow up pairwise comparisons showed that there was an increase in accuracy up to 150 ms. After this, there was no statistically significant increase in accuracy of detection. There was a strong relationship between increased gap duration and accuracy of detection ( = 446.56, p < 0.01). This converts to an r effect size measurement of 0.79. The mean and median scores, standard deviation and interquartile range of each gap condition are shown in Supplementary Material 2.
There was a positive correlation between increased length of inserted gap and increased accuracy of gap detection, which is shown in Supplementary Material 3.
An independent samples t-test was used to compare the overall accuracy of the listener groups at each duration. This revealed no significant differences between overall accuracy of perception of syllable segregation between male (mean = 69.50%, SD = 12.55) and female listeners (mean = 69.66%, SD = 10.50, rpb = 0.01); listeners with musical training (mean = 68.66%, SD = 11.61, rpb = 0.05) and listeners without musical training (mean = 69.99%, SD =10.82, rpb = 0.05); younger listeners (mean = 69.81%, SD =11.47), and older listeners (mean = 69.22%, SD = 8.91, rpb = 0.02). Listeners with speech pathology training (mean = 71.81%, SD = 10.34) were more accurate than listeners without speech pathology training (mean= 66.99%, SD = 11.26, rpb = 0.22).
Graphical screening was used to identify the gap duration with the largest differences between strong and weak onset words, which were then analysed for statistical significance using Wilcoxon signed-ranks test. Figure 3 was used to visually determine gap conditions for further analysis.
Based on Figure 3, 50, 100, and 125 ms were selected for further analysis using the Wilcoxon signed ranks test. This revealed a statistically significant difference in accuracy of detection between strong and weak onset words at these gap conditions. Listeners were more accurate when listening to strong onset words with 100 ms gaps (r = 0.33, p < 0.01) and 125 ms gaps (r = 0.25, p = 0.01). Listeners may be more accurate when listening to weak onset words with 50 ms gaps (r = 0.18, p = 0.05). This is inconclusive.
Graphical screening was used to identify the gap durations with the largest differences between female and male speakers, which were then analysed for statistical significance using the Wilcoxon Signed Ranks test. Figure 4 was created to determine these points of interest.
Based on graphical screening shown in Figure 4, gap durations with the largest differences between female and male speakers were 25, 75 and 100 ms. The Wilcoxon Signed Ranks Test revealed a difference in accuracy of listener detection between male and female speakers at these gap conditions. Listeners were more accurate when listening to female speech at 75 ms (r = 0.29, p = 0.005), and to male speech at 100 ms (r = 0.22, p =0.024) and 150 ms (r = 0.34, p = 0.001).
Listeners had an average intra-rater reliability of K = 0.709 (95% CI 0.65–0.77) suggesting listeners had a good level of agreement within their own judgements. Listeners had an average inter-rater reliability of ICC = 0.727 (95% CI 0.545–0.908), suggesting they also had moderate reliability with each other.
The impact of musical training, speech pathology training, listener age and listener gender on inter-rater reliability was investigated. There was no statistically significant difference between these groups. Table 3 outlines the inter-rater reliability of each group.
The effect of different stimuli factors on listener inter-rater reliability was also investigated. Table 4 outlines the inter-rater reliability of listeners when listening to different stimulus factors.
Table 5 outlines the comparison between listener accuracy when rating children's or adult's speech. The gap conditions with the greatest differences in listener accuracy were 75 and 100 ms, where listeners were more accurate when rating adult speech. For all conditions where a significant finding is reported, listeners were more accurate with adult than child samples.
The limen of perception of syllable segregation and the listener and speaker features which impact accurate detection of such segregation were variables of interest.
The first question was: what is the perceptual limen of adult listeners for syllable segregation, and how strong is the relationship between syllable segregation (gap) duration and the accuracy of its detection? As expected, the limen of perception of syllable segregation in children's speech was higher than that reported for adult speech (Brown et al., 2018). The threshold of accurate detection of syllable segregation in children's speech at the 80% accuracy threshold was at least 100 ms and no higher than 125 ms. At 90% accuracy, this limen was at least 125 ms and no higher than 150 ms. These values are higher than those reported in Brown et al. (2018) where the 80% threshold was 80 ms and the 90% threshold was 90 ms. The post-hoc analysis showed that there were statistically significant differences in listener accuracy when rating adult vs. child speech at gap lengths 75, 100, 125 and 200 ms. The statistically significant difference in accuracy found at 200 ms may be a statistical artefact of a greater proportion of listeners in Brown and colleagues' study correctly identifying a gap than in the present study, or may be due to listener fatigue, as this present study included a greater number of presentations (n = 80) than Brown and colleagues' study (n = 32).
The impact of stimulus factors on the listener perceptual limen of syllable segregation was investigated as the second research question. Research questions regarding which stimulus factors would impact detection were: Do strong onset words have a shorter perceptual limen than weak onset words; and does speaker gender affect the point at which listeners can identify segregation? These findings suggest that adults may be more accurate when detecting gaps in adult speech than in child speech. This potentially higher perceptual limen may be due in part to the different perceptual characteristics of children's speech described previously. When judging syllable segregation in children's speech a different standard may be required when compared with the same judgement for adult speech. That is, compared to adult speech, children's speech may need to have a greater pause duration between syllables to be considered segregated.
Unlike Brown et al. (2018), this study used child speech with both male and female speakers as well as a greater number of listeners and a larger number of samples per listener. Thus, these results may contribute more information regarding the speaker and listener factors which influence accurate detection of syllable segregation.
The third question sought to answer: do listener factors impact the listener perceptual limen of between—syllable segregation? These listener factor research questions were: Do listeners with musical training have a shorter perceptual limen compared to listeners without musical training; Do listeners with speech pathology training have a shorter perceptual limen compared to listeners without speech pathology training; Do younger listeners have a shorter perceptual limen compared to older listeners; and, does listener gender affect limen of perception in children's speech?
Musical training, age and gender did not contribute to an individual's overall perceptual accuracy while a weak correlation was found between speech pathology training and accuracy of gap detection. This is in contrast to Brown et al., who found that speech pathology training did not result in a significant difference in perceptual accuracy of syllable segregation (Brown et al., 2018). This may be due to the larger listener group (n = 84) used in this study compared to Brown's study (n = 30). That is, Brown and colleagues' sample size may not have been sufficiently large to detect a correlation between speech pathology training and accuracy of gap detection.
Listener age and gender were not also correlated with increased accuracy of perception overall. This is in contrast to existing literature regarding perceptual accuracy and age, which suggests that accuracy of detection of perceptual features declines with increased age (Snell and Frisina, 2000). The current finding on age may be due to the listener age restriction in study design and the requirement for listeners to pass a hearing screen prior to beginning the listening tasks, which may have mitigated the effect of any presbycusis present in other studies. Other possible sources of age variation were not examined in this study. The current literature is divided regarding the effect of gender on accuracy of detection of perceptual features. A larger sample may be required to confirm the current finding of no difference.
Accuracy of detection overall was not correlated with either speaker gender or onset stress pattern across all listener responses. This may be of clinical relevance and of importance to the development of computer and artificial intelligence tools, as this suggests that the speech of both male and female children may be held to the same standard when judging syllable segregation although the small sample size should be acknowledged. Accuracy of detection was correlated with speaker gender and stress onset pattern at some gap durations.
There was a statistically significant difference in accuracy of detection at the 100 ms gap condition for both factors. As the limen of perception at 90% accuracy was between 125 and 150 ms, this difference in detection occurred at a gap length lower than the limen. This suggests that listeners may be more accurate when detecting syllable segregation in strong-weak stress pattern words (compared to weak-strong stress pattern words), and in male speakers, at least in the present sample, when the inserted gap is shorter.
This study recruited two typically developing children as speakers, resulting in the need to artificially insert gaps to mimic natural segregation. However, it is possible that these artificial gaps do not truly reflect the natural syllable segregation that occurs in CAS, as other speech features (such as inappropriate lexical stress and speech sound errors) may be involved in listeners' judgments of the presence of syllable segregation (Murray et al., 2015). It must also be considered that the stimuli used were two syllable non-words. This potentially limits our ability to readily generalise these results to naturally occurring syllable segregation in a range of speakers across a range of words.
Inclusion criteria for this study differed from previous studies which examined listener factors and gap detection. This study collected information on musical training, which was defined here as a listener who had received music lessons within the previous five years, or who practised as a professional musician. Other studies which have examined musicians have used more specific selection criteria, including having commenced musical training in childhood and receiving specific academic training (e.g. Mishra et al., 2014; Elangovan et al., 2016). Similarly, of the 84 listeners who were included in the data analysis here, only 19 of these were musicians by the current definition. These factors increase the risk of a type II error as does the limited number of older adults were recruited for the study regarding the age variable. Similarly, most of the listeners with speech pathology training included in this study were students, who have more limited experience in detecting auditory features compared to qualified practising speech pathologists. This may have contributed to the weak differences in accuracy of perception between these groups.
Whilst the reported accuracy thresholds of 80 and 90% for the limen of perception are appropriate for use in a research context, there remains the question of whether these are sufficiently sensitive or specific for a clinical context. Judgments of syllable segregation are most likely to occur in real time in clinical settings, without an anchor stimulus for comparison, and in combination with other speech errors. Additionally, various distractors are present in a clinical setting including background noise and child behaviour. Clinical practise often requires a clinician to rate multiple speech features simultaneously. Perhaps the accuracy threshold for a limen in clinical contexts, and in children with actual CAS, would be higher than reported here.
While it was beyond the scope of this paper, future research should investigate perception of syllable segregation using a wider range of speakers and stimuli. This includes testing non-words with a greater range of phonemes, testing real words, testing polysyllabic real and non-words with a range of lengths and stress patterns, and testing in languages other than English. Future research should also explore listeners' perception of natural syllable segregation occurring in the speech of children with CAS. Such research could provide valuable information regarding listener perception of this feature which could be applied to the development of standardised diagnostic tools, computer and artificial intelligence use in treatment and diagnosis of CAS. Future research should also consider examining the threshold of gap detection using smaller gap increments, for example 5 ms, within the ranges identified as significant here.
This research has a number of clinical implications relevant to the diagnosis and treatment of CAS. Firstly, it provides data on the pause duration at which listeners can perceive segregation in child speech. This data could be used to determine what level of segregation may constitute a significant therapy goal and be used to train clinicians to rate these features more accurately and reliably. For example, Rapid Syllable Transition (ReST) treatment (McCabe et al., 2017) is one of a limited set of evidence-based treatments for CAS. This treatment relies on a clinician's real time perception of syllable segregation. Training clinicians using real and modified samples around the limen could increase the accuracy and speed of such decisions and potentially the efficacy of the intervention. A refined limen of perception of syllable segregation in children with CAS could also be used to develop computer-aided tools which could be used for diagnosis and treatment of CAS.
This research may also aid the development of an AI tool for diagnosis of CAS and other prosodic disorders. Given the limited accessibility of Speech-Language Pathologists (McGill et al., 2020) children may benefit from computer-aided speech therapy tools as a means to reduce waiting lists and increase access generally (Shahin et al., 2020). However, accuracy of automated disordered speech analysis tools is not yet reliable enough to be used clinically (Shahin et al., 2020). Identifying the threshold of accurate gap detection could be used to improve computer-aided tools for the diagnosis and treatment of CAS and other prosodic disorders. Such results may also have implications for further development of computer and artificial intelligence recognition of children's speech more broadly. Current speech recognition systems trained on adult speech show a degradation in performance when used for child speech, due to linguistic and acoustic mismatches between adult and child speech (Shahin et al., 2020). These findings may therefore be useful in improving artificial intelligence in the treatment and diagnosis of children's speech disorders and in understanding child speech in general.
Listener factors of musical training, age and gender were not significantly correlated with accuracy of detection of syllable segregation while speech pathology training was weakly related to increased accuracy of gap detection. This has clinical implications for speech pathology practise and suggests that specific training may be required for clinicians treating CAS or other prosodic disorders which feature syllable segregation. The weak relationship between speech pathology training and average accuracy does however suggest that members of the community may be able to identify syllable segregation in the speech of children with CAS with accuracy not far below clinicians. As increased therapy dosage is related to generalisation of skills (Edeal and Gildersleeve-Neumann, 2011), this finding has implications for service delivery models and utilisation of family members as therapists into speech-language therapies.
This study suggests that the limen of perception of syllable segregation in children's speech is at least 125 ms and no higher than 150 ms. This study also suggests that the limen of perception of children's speech is higher than that of adult speech, and that adult listeners are less accurate when detecting syllable segregation at gap lengths of 75, 100, 125 and 200 ms in children's speech compared to adult speech although the latter finding needs confirmation. There is no evidence that there is any difference in listener accuracy or reliability related to musical training, age or gender in their perception of syllable segregation in typical children's speech. There is some evidence which suggests that speech pathology training may result in improved accuracy of gap detection. Overall, the findings provide useful information that may contribute to the development of a standardised rating tool for syllable segregation to be used in the assessment, diagnosis and management of CAS as well as contribute to the further development of computer and artificial intelligence for use in treatment and diagnosis of speech disorders.
The original contributions presented in the study are included in the article/Supplementary Material, further inquiries can be directed to the corresponding author.
The studies involving human participants were reviewed and approved by The University of Sydney Human Research Ethics Committee. Written informed consent to participate in this study was provided by the participants' legal guardian/next of kin.
CO'F designed the study with support from PM and AP. CO'F and PM collected the data with support from AP. CO'F cleaned and prepared the data. CO'F and RH analysed the data. CO'F wrote the manuscript with support from PM, AP, and RH. All authors approved the final version of the manuscript. CO'F, PM, and RH contributed to the revisions.
The authors declare that the research was conducted in the absence of any commercial or financial relationships that could be construed as a potential conflict of interest.
All claims expressed in this article are solely those of the authors and do not necessarily represent those of their affiliated organizations, or those of the publisher, the editors and the reviewers. Any product that may be evaluated in this article, or claim that may be made by its manufacturer, is not guaranteed or endorsed by the publisher.
The authors would like to thank Tayla Brown for permission to re-analyse the data in Brown et al. (2018). We would also like to thank the participants and Callie Swayze for help during her 2018 internship.
The Supplementary Material for this article can be found online at: https://www.frontiersin.org/articles/10.3389/fcomm.2022.839415/full#supplementary-material
Altman, D.. (1991). Practical Statistics for Medical Research. London: Chapman and Hall. doi: 10.1201/9780429258589
American Speech-Language-Hearing Association. (2007). Childhood Apraxia of Speech [Technical report]. Available online at: http://www.asha.org/policy (accessed September 2, 2021)
Audacity Team (2021). Audacity(R): Free audio editor and recorder. Version 2.4.2. Available online at: https://audacityteam.org/ (accessed November 30, 2021)
Bartko, J.. (1966). The intraclass correlation coefficient as a measure of reliability. Psychol. Rep. 19, 3–11. doi: 10.2466/pr0.1966.19.1.3
Brown, T., Murray, E., and McCabe, P. (2018). The boundaries of auditory perception for within-word syllable segregation in untrained and trained adult listeners. Clin. Linguist. Phon. 32, 979–996. doi: 10.1080/02699206.2018.1463395
Chakravarti, M., Laha, R., and Roy, J. (1967). Handbook of Methods of Applied Statistics, Volume I. Hoboken, NJ: John Wiley and Sons. 392–394.
Cohen, J.. (1960). A coefficient of agreement for nominal scales. Educ. Psychol. Meas. 20, 37–46. doi: 10.1177/001316446002000104
Cychosz, M., Edwards, J., Munson, B., and Johnson, K. (2019). Spectral and temporal measures of coarticulation in child speech. J. Accoust. Soc. 146, 516–522. doi: 10.1121/1.5139201
Edeal, M., and Gildersleeve-Neumann, C. (2011). The importance of production frequency in therapy for childhood apraxia of speech. Am. J. Speech Lang. Pathol. 20, 95–110. doi: 10.1044/1058-0360(2011/09-0005)
Elangovan, S., Payne, N., Smurzynski, J., and Fagelson, A. (2016). Musical training influences auditory temporal processing. J. Hear. Sci. 6, 37–44. doi: 10.17430/901913
Fedorenko, E., Morgan, A., Murray, E., Cardinaux, A., Mei, C., Tager-Flusberg, H., et al. (2016). A highly penetrant form of childhood apraxia of speech due to deletion of 16p11.2. Eur. J. Hum. Genet. 24, 302–306. doi: 10.1038/ejhg.2015.149
Friedman, M.. (1937). The use of ranks to avoid the assumption of normality implicit in the analysis of variance. J. Am. Stat. Assoc. 32, 675–701. doi: 10.1080/01621459.1937.10503522
Fritz, C., Morris, P., and Richler, J. (2011). Effect size estimates: current use, calculations, and interpretation. Exp. Psychol. Gen. 141, 2–18. doi: 10.1037/a0024338
Gerosa, M., Lee, S., Giuliani, D., and Narayanan, S. (2006). “Analyzing children's speech: an acoustic study of consonants and consonant-vowel transition [Conference presentation],” in 2006 IEEE International Conference on Acoustics Speech and Signal Processing Proceedings (Toulouse).
Giannela-Samelli, A., and Schochat, E. (2008). The gaps-in-noise test: gap detection thresholds in normal-hearing young adults. Int. J. Audiol. 47, 238–245. doi: 10.1080/14992020801908244
Gierut, J., Morrisette, M., and Ziemer, S. (2010). Nonwords and generalisation in children with phonological disorders. Am. J. Speech Lang. Pathol. 19, 167–177. doi: 10.1044/1058-0360(2009/09-0020)
Harvey, C.. (2016). Binary choice vs ratings scales: a behavioural science perspective. Int. J. Mark. Res. 58, 647–648. doi: 10.2501/IJMR-2016-041
Hearing Australia (2021). Hearing Australia: Online Hearing Assessment. Available online at: https://www.hearing.com.au/onlineassessment (accessed October 31, 2021)
Heldner, M.. (2011). Detection thresholds for gaps, overlaps, and no-gap-no-overlaps. J. Acoust. Soc. 130, 508–513. doi: 10.1121/1.3598457
Hildebrand, M., Jackson, V., Scerri, T., Reyk, O., Coleman, M., Braden, R., et al. (2020). Severe childhood speech disorder: gene discovery highlights transcriptional dysregulation. Neurology 94, 2148–2167. doi: 10.1212/WNL.0000000000009441
Koo, T., and Li, M. (2016). A guideline of selecting and reporting intraclass correlation coefficients for reliability research. J. Chiropr. Med. 15, 155–163. doi: 10.1016/j.jcm.2016.02.012
Landis, J., and Koch, D. (1977). The measurement of observer agreement for categorical data. Biometrica 33,159–164. doi: 10.2307/2529310
Lee, S., Potamianos, A., and Narayanan, S. (1999). Acoustics of children's speech: developmental changes of temporal and spectral parameters. J. Acoust. Soc. Am. 105, 1455–1468. doi: 10.1121/1.426686
Markham, D., and Hazan, V. (2004). The effect of talker- and listener-related factors on intelligibility for a real-word, open-set perception test. J. Speech Lang. Hear Res. 47, 725–737. doi: 10.1044/1092-4388(2004/055)
McCabe, P., Thomas, D., Murray, E., Crocco, L., and Madill, C. (2017). Rapid syllable transition treatment-ReST. Sydney, NSW: The University of Sydney.
McGill, N., Crowe, K., and Mcleod, S. (2020). “Many wasted months”: Stakeholders' perspectives about waiting for speech-language pathology services. Int. J. Speech. Lang. Pathol. 22, 313–326. doi: 10.1080/17549507.2020.1747541
Mishra, S., Panda, M., and Herbert, C. (2014). Enhanced auditory temporal gap detection in listeners with musical training. J. Acoust. Soc. Am. 136, 173–178. doi: 10.1121/1.4890207
Murray, E., McCabe, P., Heard, R., and Ballard, K. (2015). Differential diagnosis of children with suspected childhood apraxia of speech. J. Speech Lang. Hear. Res. 58, 43–60. doi: 10.1044/2014_JSLHR-S-12-0358
Pichora-Fuller, M., Schneider, B., Benson, N., Hamstra, S., and Storzer, E. (2006). Effect of age on detection of gaps in speech and nonspeech markers varying in duration and spectral symmetry. J. Accoust. Soc. Am. 119, 1143–1155. doi: 10.1121/1.2149837
R Core Team (2021). R: A Language and Environment for Statistical Computing. Vienna: R Foundation for Statistical Computing. Available online at: https://www.R-project.org/ (accessed February 16, 2022)
Ratner, B.. (2009). The correlation coefficient: its values range between +1/−1, or do they? J. Target. Meas. Anal. Mark. 17, 139–142. doi: 10.1057/jt.2009.5
Shahin, M., Zafar, U., and Ahmed, B. (2020). The automatic detection of speech disorders in children: challenges, opportunities, and preliminary results. IEEE J. Sel. Top. Signal Process. 14, 400–412. doi: 10.1109/JSTSP.2019.2959393
Shriberg, L., Lohmeier, H., Campbell, T., Dollaghan, C., Green, J., and Moore, C. (2009). A nonword repetition task for speakers with misarticulations: the syllable repetition task (SRT). J. Speech Lang. Hear, Res. 52, 1189–1212. doi: 10.1044/1092-4388(2009/08-0047)
Shriberg, L., Strand, E., Fourakis, M., Jakielski, K., Hall, S., Karlsson, H., et al. (2017). A diagnostic marker to discriminate childhood apraxia of speech from speech delay: I. development and description of the pause marker. J. Speech Lang. Hear. Res. 60, 1096–1117. doi: 10.1044/2016_JSLHR-S-16-0148
Snell, K., and Frisina, D. (2000). Relationships among age-related differences in gap detection and word recognition. J. Acoust. Soc. Am. 107, 1615–1626. doi: 10.1121/1.428446
Storkel, H.. (2019). Using developmental norms for speech sounds as a means of determining treatment eligibility in schools. Perspect. ASHA Special Interest Groups. 4, 67–75. doi: 10.1044/2018_PERS-SIG1-2018-0014
Wilcoxon, F.. (1945). Individual comparisons by ranking methods. Biometr. Bull. 1, 80–83. doi: 10.2307/3001968
Keywords: speech disorder, auditory perception, child, apraxia, artificial intelligence
Citation: O'Farrell C, McCabe P, Purcell A and Heard R (2022) The Adult Perceptual Limen of Syllable Segregation in Typically Developing Paediatric Speech. Front. Commun. 7:839415. doi: 10.3389/fcomm.2022.839415
Received: 19 December 2021; Accepted: 25 February 2022;
Published: 25 March 2022.
Edited by:
Christopher Carignan, University College London, United KingdomReviewed by:
Min Ney Wong, Hong Kong Polytechnic University, Hong Kong SAR, ChinaCopyright © 2022 O'Farrell, McCabe, Purcell and Heard. This is an open-access article distributed under the terms of the Creative Commons Attribution License (CC BY). The use, distribution or reproduction in other forums is permitted, provided the original author(s) and the copyright owner(s) are credited and that the original publication in this journal is cited, in accordance with accepted academic practice. No use, distribution or reproduction is permitted which does not comply with these terms.
*Correspondence: Patricia McCabe, dHJpY2lhLm1jY2FiZUBzeWRuZXkuZWR1LmF1
Disclaimer: All claims expressed in this article are solely those of the authors and do not necessarily represent those of their affiliated organizations, or those of the publisher, the editors and the reviewers. Any product that may be evaluated in this article or claim that may be made by its manufacturer is not guaranteed or endorsed by the publisher.
Research integrity at Frontiers
Learn more about the work of our research integrity team to safeguard the quality of each article we publish.