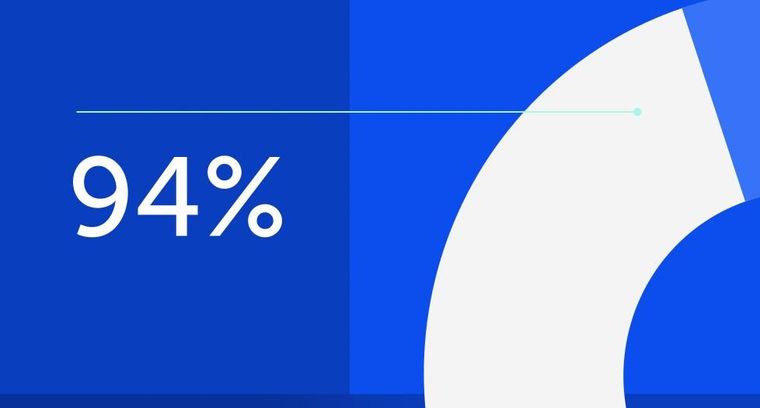
94% of researchers rate our articles as excellent or good
Learn more about the work of our research integrity team to safeguard the quality of each article we publish.
Find out more
ORIGINAL RESEARCH article
Front. Commun., 09 January 2023
Sec. Psychology of Language
Volume 7 - 2022 | https://doi.org/10.3389/fcomm.2022.1088170
This article is part of the Research TopicScience, Technology, and Art in the Spoken Expression of MeaningView all 12 articles
Introduction: Voice has been used to project identity in dubbing, in order to auditory portray appropriate role images in TV dramas. This study investigates the character voices of leading male characters in Empresses in the Palace.
Methods: Different acoustic characteristics of character voices and matching relation between acoustics and role images are explored by comparing F0, CPP, harmonic amplitude differences of speech spectrum.
Results: The voice quality of characters is related to their relative social status. The subordinates usually adopt a higher pitch or breathy voice, while the dominators use a lower pitch or modal/creaky voice. In addition, CPP, F0, and H1-A3 are the key acoustic indicators to distinguish character voices.
Discussion: These results reveal the acoustic characteristics of character voices of certain types, as well as provide guidance for dubbing vividly.
The terms “voice” and “voice quality” have not been defined with a broad agreement, since the researches in these fields are transdisciplinary. These terms are usually defined in both a broad sense and a narrow sense. Narrowly, “voice” refers to how the vocal folds vibrate, namely, vibratory patterns of the vocal folds; and “voice quality” represents the voicing produced at the glottis, which is also termed as “phonation type” or “phonation quality” (Esling et al., 2019, p. 2–8). In a broad sense, “voice” is essentially synonymous with “speech”, while “voice quality” refers to the auditory characteristics of the speaker's voice (Abercrombie, 1967, p. 91; Esling et al., 2019, p. 123; Liu, 2021). The relationship between voice and voice quality can be simply explained as follows: “voice” has a physical and physiological base that refers to the acoustic signal, while “voice quality” refers to the perceptual impression that occurs as a result of that signal (Kreiman and Sidtis, 2011, p. 5). American National Standards Institute also defines “voice quality” as the attribute of auditory sensation (ANSI et al., 1960, p. 45). In the following part, “voice” refers to sound produced by the vocal folds. In contrast, “voice quality” indicates the perceptual ramifications caused by different vocal fold configurations.
Voice quality is one of the primary means by which speakers project their identity, i.e., their physical, psychological, and social characteristics (Laver, 1980, p. 2; Kreiman and Sidtis, 2011, p. 1). Information such as gender, age, and mood can be easily perceived by the listener, even without seeing the speaker. Voice allows us to recognize individuals and emotional states, also called “auditory face” (Belin et al., 2004). The listener can form an impression of the speaker based on the voice. It specifically includes body type, lifestyle, mental state (Kreiman et al., 2005), and even morality (Teshigawara, 2003; Podesva and Callier, 2015). Although voice identification is not always a certainty (Bonastre et al., 2003), we can still recognize a person instantly through voice individuality (Dolar, 2006, p. 22), especially for the recognition of familiar voices (Van Lancker et al., 1985; Eriksson, 2005; Kreiman and Sidtis, 2011; Hansen and Hasan, 2015). For example, even the greeting “hello” can convey information about the speaker his/herself to the listener, allowing the listener to form a judgement of the speaker's personality; based on this, a two-dimensional model on personality judgement was constructed (Wu et al., 2021).
As an essential factor influencing a speaker's identity perception, behavioral research has found that pitch is related to the listener's perception of dominance. Morton (1977) found that birds and mammals use harsh and relatively low-frequency sounds when hostile, while higher-frequency, more pure tone-like sounds are adopted when frightened, appeasing, or approaching in a friendly manner. The association between particular images and certain sounds across many languages is also known as sound symbolism (e.g., Sapir, 1929; Hinton et al., 1994; Fónagy, 2001; among many others). Ohala (1984, 1994) proposed the frequency code hypothesis to represent this sound pattern, indicating that these sound symbolic patterns have phonetic bases. To be specific, for both humans and animals, compared to a voice with higher fundamental frequency (F0), a voice with lower F0 is commonly perceived as having a larger vocalizer and larger body, and are thus perceived more dominant, aggressive, and threatening. Cao and Kong (2016) demonstrated that the length and volume of the human pharyngeal cavity, as well as the length and volume of the vocal tract, are all significantly positively correlated with body height. In recent years, the frequency code hypothesis and sound symbolism have also been used to investigate how human voices match perceptual impression of femininity, vulnerability, submissiveness, politeness, friendliness, insecurity, uncertainty or charisma, and so on (e.g., Grawunder and Winter, 2010; Noble and Xu, 2011; Signorello et al., 2012; Mixdorff et al., 2018; Cartei et al., 2019; Yang et al., 2020; Rallabandi et al., 2021; Weiss et al., 2021), as well as the naming of animation characters when dubbing in Walt Disney cartoons (Lippi-Green, 2012) or Pokémon names (Kawahara et al., 2018). Since high vowels tend to have a higher intrinsic F0 than low vowels (Chen et al., 2021), the names of characters with initial high vowels tend to be smaller and lighter in Pokémon (Kawahara et al., 2018). In addition, Puts et al. (2006) suggested that the pitch of the male voice may reflect his perceptions of his dominance. Specifically, men who believe they are physically dominant to their competitors lower their voice pitch, whereas men who believe they are less dominant raise their pitch. Stern et al. (2021) also indicated a significant negative correlation between voice pitch and self-reported sociosexuality, dominance, and extraversion; moreover, lower voice pitch is perceived as being more attractive in men. All the studies mentioned above demonstrate the importance of pitch in the construction of a speaker's identity.
The definition of dubbing also includes both broad and narrow perspectives. Broadly, dubbing includes all sound elements in a film or TV drama. In the narrow sense, dubbing refers to the creative activity of adding voice to characters by voice actors, adding extra voices and narration, or replacing the dialogue in the original film with another language (Liu, 1994, p. 4). In order to convey identity and to portray a character actively, dubbing requires the conscious use of his/her voice's ability to convey the speaker's message, and to add to films or TV dramas a voice that fits the character's image. In Animation Sound Design, Zhao et al. (2015) mentioned that “sound can also have the same narrative effects as images, and is even, to some extent, precedent over visual expression”. Dubbing can make characters more vivid, bringing new vitality to the film and animation industry.
The method for dubbing to portray characters in film and TV drama is mainly to match the perceptual impression produced by the dubbed voice to the character's image. Apart from characters who use the contrast between appearance and voice to reach a comedy effect (e.g., Lina Lamont in Singin'in the Rain, whose beautiful, gentle appearance contrasts sharply with her shrill, harsh voice, Donen et al., 1952), dubbing, in general, needs to match and highlight the uniqueness of the character auditorily, thereby enhancing the audience's recognition of the character's image. For example, in the film My Fair Lady, despite being a flower girl, the leading female is considered an actual princess because of speaking a fluent upper-class style accent (Shaw and Fisher, 1963; Cukor, 1964). In Japanese animations, the epilaryngeal settings, which played a major role in distinguishing four heroic and villainous voice types, were identified as the auditorily critical vocal components that differentiate good and evil characters (Teshigawara, 2003). Moreover, to portray unique and distinctive characters, voice actors often use several stereotypical phonation types or actively raise pitch significantly to affect the listener's perception of the character's voice quality, further reinforcing these stereotypes. For example, popular American media usually use harsh voice and exaggerated emotional states associated with yelling/shouting modes of expression to portray the racial stereotype of black people (Moisik, 2012). Moreover, voice with a Yiddish accent once used to make the stereotype of wolf complete in Disney cartoon (Lippi-Green, 2012, p. 107). Stereotypes on homosexuality also influence dubbing. When acting for homosexual males, voice actors often choose a higher F0, falsetto, or higher formant frequencies to present effeminacy (Podesva, 2007; Cartei and Reby, 2012). Similarly, using creaky voice in Chinese films and TV dramas may be considered a sign of promiscuity (Callier, 2010).
According to the broader definition of voice quality, the perceptual impressions produced by dubbed voices are their voice quality, and the perceptual differences are caused by physiological and acoustic changes reaching a certain level. Thus, there is an acoustic basis for voice quality changes. Sweet voice is often used in Japanese animation for mature and traditional female characters, such as mothers, elder sisters, and teachers. Moreover, they have a lower pitch than the voice of the leading females. As described by Klatt and Klatt (1990) and Pépiot (2014), breathy voice is considered to be feminine; similarly, sweet voice also shows breathy voice (Starr, 2015). In addition, in order to discriminate between protagonists and antagonists acoustically, Teshigawara (2003) investigated the acoustic characteristics of voice quality using the voice of heroes and villains in Japanese animation, indicating that voices of villains usually have a lower second formant (F2) and more high-frequency energy. Tong and Moisik (2021) analyzed the voice of protagonists and antagonists in American cartoons using long-term average spectrum (LTAS), and found that the protagonists and antagonists exhibit high- and low-frequency dominance in their spectral profiles, respectively. Meanwhile, harsh voice is also often used to portray lazy and brutal villains in Chinese films and TV dramas (Callier, 2012).
With the great demand for voice acting in film and TV dramas, the academic community has also paid more attention to acoustic research on voice acting. The commonly used methods include harmonic analysis, LTAS, principal component analysis, etc. By reviewing theoretical and empirical studies on voice acting in the literature, it is not difficult to find that most existing studies try to explore the acoustic characteristics that distinguish the voices of characters of different types through single-parameter acoustic analysis. However, the nature of the voice quality is multi-dimensional. Therefore, it is only by introducing more acoustic parameters into the study of voice acting that we can discuss in-depth which acoustic parameters are the key indicators that distinguish different characters. In other words, the distinctive features across characters need to be sought by means of acoustic analysis to characterize different styles. Furthermore, with the increase in the quality of film and TV dramas, the demand for personalized dubbing continues to grow, placing greater demands on voice actors' expertise. Unfortunately, the existing dubbing guidance is usually subjective and lacks practicality and operability. For example, maintaining a “sense of drama” and “elasticity of voice”, achieving a “richness of both voice and emotion” and improving “characterization” (Yang L., 2021; Yang Z. S., 2021) are too general, and remain at the level of subjective descriptions, not providing a scientific explanation for acoustic characteristics of personalized dubbing, making it difficult to grasp in practice.
Considering these problems mentioned above, this study focuses on the acoustic characteristics of the dubbed voices and their matching with the characters' image. The following two major questions need to be addressed. One is whether there are acoustic differences between different characters' dubbings, as well as the matching between acoustic parameters and the character's image. The other is what kind of reference we can provide to voice actors for improving the matching between the characters and their dubbing based on the acoustic analysis results.
The speech data used in this study was taken from the entire TV series Empresses in the Palace (). The reason for choosing this TV series is that this TV series has a high reputation and is more prototypical among Chinese costume dramas. As of August 2022, it has accumulated 12.81 billion views on LeTV alone, which is a much wider audience. In addition, the TV series has generated a vibrant secondary creation, attracting a larger audience.
In this study, five major male characters were selected from Empresses in the Palace, and their “character status” was determined according to their character image (see Table 1).
Adobe Premiere Pro 2020 was used to extract audio files from MP4-format video files. The sampling rate of speech signals is 44 kHz, with 16-bit sampling resolution, and the recording is monophonic. The speech material consists of monosyllabic and sentence files.
The obtained audio files were divided by character, with 480 monosyllabic tokens for each character, with a total of 2,400 tokens for all five characters. The final (rhyme) part of each token was then annotated in Praat 6.1.37 (Boersma and Weenink, 2021), with the initials, finals, and tones annotated in the first tier, and the finals also separately annotated in the second layer, as shown in Figure 1.
Figure 1. An example of annotated monosyllabic tokens “快kuài请qǐng太tài后hòu (Go and invite the Empress Dowager!)”.
To make sure that the duration of sentence samples for each character was long enough and similar, sentences of varying lengths were joined into a total of 30 sentence samples of around 40 s in Praat software, with a total duration of 1,256 s, while avoiding strong emotions, noise, background music, and sound effects segments as much as possible. Details of sentence samples are given in Table 2.
Based on previous studies discussed in the introduction section (e.g., Ohala, 1984; Starr, 2015; Stern et al., 2021; Tong and Moisik, 2021), this study mainly focuses on acoustic parameters that are closely related to voice quality, and that are mainly used to represent pitch, harmonic-to-noise ratio, and spectral energy intensity.
One measure is F0. It refers to the frequency at which the vocal folds vibrate, i.e., the first harmonic (H1) in the spectrum, which is a physical quantity in acoustics. Pitch, on the other hand, is the perception of the height of a sound, and is a psychological concept (Kong, 2015). The primary physical quantity that carries pitch is F0 (Liu, 1924). F0 plays a vital role in voice quality perception, and can determine the pitch of the voice quality. Generally speaking, the higher the F0, the higher the perceived pitch.
The other measure is cepstral peak prominence (CPP), which is defined as the amplitude of the cepstral peak, measured based on normalized overall amplitude in the spectrum (Hillenbrand et al., 1994). And a speech signal whose spectrum shows a well-defined harmonic structure will show a very prominent cepstral peak (Hillenbrand and Houde, 1996; Miramont et al., 2020). Thus, CPP can be used to quantify the periodicity and harmonic-to-noise ratio of the speech signal. In general, the more periodic the speech signal, the weaker the noise, and the greater value of the CPP. On the contrary, the smaller the CPP, the stronger the noise. Therefore, CPP is also often used to quantify differences among phonation types, and is considered a reliable acoustic parameter for discriminating breathy voice from non-breathy voice (Blankenship, 2002). Hartl et al. (2003) also indicated a negative correlation between breathy voice and CPP.
In addition, the commonly used harmonic amplitude parameters (H1-H2, H2-H4, etc.) and long-term average spectrum (LTAS) are also selected to investigate the energy distribution in different frequency ranges in the spectrum. The selection of harmonic amplitude parameters is based on Kreiman et al. (2014), who proposed the following four harmonic components to be particularly important in the simulation of voice source spectrum, namely, H1-H2 (the amplitude difference between the first harmonic and the second harmonic), H2-H4 (the amplitude difference between the second harmonic and the fourth harmonic), H4-H2k (the amplitude difference between the fourth harmonic and the harmonic nearest 2,000 Hz), H2k-H5k (the amplitude difference between the harmonic nearest 2,000 Hz and the harmonic nearest 5,000 Hz). In addition, H1-A3 (the amplitude difference between the first harmonic and the harmonic nearest to the third formant) is also a useful parameter in studying phonation types (Iseli et al., 2007). The amplitude difference reflects the strength of the spectral energy attenuation among different frequency ranges. The larger the amplitude difference, the stronger the spectral energy attenuation in that range, and the greater the spectral tilt. Specifically, H1-H2 is proportional to the open quotient (OQ), which reflects the duration of the open phase of the vocal folds within a glottal cycle. The larger the OQ, the less tightly closed the vocal folds, the more the airflow leak, the stronger the spectral energy attenuation, and the more prominent the breathy voice (Ladefoged, 2003, p. 178–181; DiCanio, 2009). H2-H4 is the auxiliary measuring parameter to determine phonation types, and has been used among cross-linguistic studies to compare voicing between different phonetic systems (Esposito, 2006). H4-H2k, H1-A3, and H2k-H5k represent the degree of spectral energy attenuation and spectral tilt at low, low-mid, and mid-high frequency ranges respectively. On the other hand, LTAS is described by Leino (2009) as “a means of viewing the average frequency distribution of the sound energy in a continuous speech sample”, reflecting the distribution of spectral energy across frequency ranges. Note that the prerequisite for using LTAS is that the duration of the speech sample is long enough so that the linguistic content of the speech sample can be ignored, and the interference of non-speech components can be avoided, to focus on the personal characteristics of the speaker's voice (Pittam, 1987; Mendoza et al., 1996). On the basis, Li et al. (1969) stated that the duration of the speech sample should be at least 30 s, while Fritzell et al. (1974) stated that LTAS results are more stable and reliable when the speech signal is ~40 s.
In this study, F0, CPP, and harmonic amplitude parameters were extracted using VoiceSauce (Shue et al., 2009), and LTAS was extracted using Wavesurfer (Sjölander and Beskow, 2000). On this basis, Z-scores were calculated using SPSS Statistics 26.0, and data with Z-scores >2 or < -2 (~5%) were considered outliers and removed. The data were then tested for normality using Origin 2021. A t-test was conducted for data that follow a normal distribution, otherwise a Kruskal-Wallis non-parametric test was conducted. Finally, multi-dimensional scaling analysis (MDS) was carried out using SPSS Statistics 26.0.
The pitch measurements for different character voices are analyzed first. Figure 2 shows a boxplot of the F0 data, where data beyond two standard deviations are set as outliers. The value of mean F0 demonstrates that: M02 (Prince) > M05 (Minister) > M04 (Eunuch) > M01 (Monarch) > M03 (Physician). Specifically, M03 (Physician), and M01 (Monarch) have lower mean F0, which are 96.83 and 107.78 Hz, respectively. M02 (Prince) and M05 (Minister) have higher mean F0, 128.57 and 127.02 Hz respectively. M04 (Eunuch) has an intermediate mean F0, which is 113.51 Hz. M02 (Prince), M04 (Eunuch), and M05 (Minister) have larger F0 variation range (outliers included), and larger standard deviations, which are 48–278 Hz (31.01), 46–221 Hz (20.25), and 47–244 Hz (25.87), respectively. However, the F0 variation and standard deviation of M01 (Monarch) and M03 (Physician) are relatively lower, which are 46–191 Hz (15.50) and 71–154 Hz (9.66), respectively.
The following two conclusions can be drawn from the F0 mentioned above. First, based on the relationship between F0 and pitch, it is not difficult to find that M01 (Monarch) and M03 (Physician) have lower mean F0, which suggests that they have a lower pitch, with a deep voice. On the contrary, M02 (Prince) and M05 (Minister) have larger mean F0, higher pitch, and relatively brighter voice quality. Combining Figure 2 with Table 1, we found a clear difference in the social status of the five characters, creating an identity dichotomy between dominators and subordinates. M01 (Monarch), the highest social status dominant of them all, has a lower pitch. The other characters are in the position of subordinate relative to M01 (Monarch). M02 (Prince), M04 (Eunuch), and M05 (Minister) have a relatively higher pitch, but M03 (Physician) has a lower pitch, which will be discussed in detail in section 4. Second, the range of F0 variation, i.e., the pitch range, affects the intonation of the dubbed characters. Chao (1968, p. 39) used the analogy of “large waves” and “small ripples” to illustrate the relationship between intonation and tone in Chinese, in which the tone is superimposed on intonation. “Intonation is the pattern of the pitch movement of an utterance”, and pitch is one of its constituent elements (Cao, 2002; Ding, 2005). Among the male characters, M02 (Prince) and M05 (Minister) have the most extensive F0 range and standard deviation, indicating that they have large F0 fluctuations during phonation. Their voices are with a lilt, with a sense of rhythm and rhyme, and full of emotion. Compared to the voices of the other characters, M01 (Monarch) and M03 (Physician) have a small range of F0 variation, and their voices lack fluctuation. The flatness of the intonation makes the voice sound more calm, or suppressed in emotion.
CPP can be used to measure the periodicity and harmonic-to-noise ratio of the speech signal in the previous literature, which is an essential acoustic parameter for quantifying phonation types (e.g., Hillenbrand et al., 1994; Hillenbrand and Houde, 1996; Blankenship, 2002; Hartl et al., 2003; Miramont et al., 2020). Figure 3 shows a boxplot based on the CPP for each character's dubbed voice. The results for mean CPP indicate that: M01 (Monarch) > M03 (Physician) > M02 (Prince) > M05 (Minister) > M04 (Eunuch). To be specific, M01 (Monarch) has the highest CPP (20.12 dB). M04 (Eunuch) has the lowest CPP (18.06 dB). CPP of the other characters are in between. CPP of the five characters have small standard deviations, which are between 1.7 and 2.2, showing a concentrated distribution pattern. On the whole, M01 (Monarch) has the largest CPP, indicating that this voice has the least noise component in its speech signal. The CPP of M04 (Eunuch) is significantly lower than that of the other characters, indicating a significant noise component in his speech signal. So the turbulent noise can be perceived in his voice.
As discussed in section 2.3, harmonic parameters can be used to analyze spectral energy distribution in the speech signal. These measures represent the attenuation of spectral energy, in which higher values indicate a more substantial energy attenuation in that frequency range. The five harmonic parameters are shown in Table 3 and Figure 4 for each character. Figure 5 is the LTAS of each character.
H1-H2 and H2-H4 indicate the strength of energy attenuation in low frequency, which are important indicators for measuring breathy voice. The larger their values, the more serious the airflow leak through the glottis. Table 3 and Figure 4 show that M04 (Eunuch) has the largest H1-H2 and a relatively large H2-H4, suggesting a strong attenuation of its spectral energy in low frequency. M02 (Prince) has a relatively small H1-H2 and the smallest H2-H4, indicating a weak attenuation of its spectral energy in low frequency. The remaining three characters have an overall intermediate range of energy attenuation in low frequency. H4-H2k and H1-A3 indicate the strength of energy attenuation in low-mid frequency. M03 (Physician) and M04 (Eunuch) both have a larger or the largest H4-H2k and H1-A3, both of which have strong spectral energy attenuation in low-mid frequency. M02 (Prince) and M05 (Minister) have the smallest values, with weak spectral energy attenuation in low-mid frequency. M01 (Monarch) has a smaller H4-H2k and a larger H1-A3, with intermediate energy attenuation in low-mid frequency. H2k-H5k indicates the strength of energy attenuation in mid-high frequency. M02 (Prince) has the largest H2k-H5k, and its spectral energy attenuation is the strongest in mid-high frequency. M04 (Eunuch) has the smallest H2k-H5k, and its spectral energy attenuation is the weakest in mid-high frequency. The remaining three have intermediate energy decay in mid-high frequency.
The distinctive features of the voice of the five characters can be obtained when combining the acoustic characteristics of all parameters in each character's voice (see Table 4).
F0 shows the pitch level of the character's voice, while CPP and the strength of spectral energy attenuation (i.e., spectral tilt) together show whether the character's voice has breathy voice. Usually, the lower the CPP, and the stronger the overall attenuation of the spectral energy, the higher the degree of breathy voice. The voice quality of the five characters in Table 4 has the following characteristics. To be specific, in terms of F0, the dominant M01 (Monarch), who has the highest social status, has a lower F0 and a lower voice pitch, while the subordinate M05 (Minister) and M02 (Prince), who have a relatively low social status, both have a higher F0 and a higher voice pitch. Regarding phonation types, M01 (Monarch) has a neutral level of all harmonic parameters and the largest CPP, with no obvious non-modal phonation characteristics, which can be considered a modal voice among the five characters. Within the other characters, the higher social status dominators M02 (Prince) and M05 (Minister) have a weaker energy attenuation than the other characters, a weaker noise component in the speech signal, and no apparent breathy voice. The lower social status subordinate characters M03 (Physician) and M04 (Eunuch) have an overall stronger attenuation in spectral energy, a stronger noise component in the speech signal, and obvious breathy voice. It can be seen that, when dubbing for a subordinate character, the voice actors tend to choose to raise pitch or use breathy voice; however, when dubbing for a dominant character, the actor tends to lower pitch and does not adopt breathy voice.
The five characters can be easily distinguished from the perspective of subjective auditory perception. In terms of acoustic performance, the voices of the five characters also differ acoustically by comparing F0, CPP, and harmonic parameters. These phenomena make us wonder whether voices that differ significantly in speech perception are also acoustically significantly different. Which acoustic parameters are the key acoustic indicators that distinguish character voices? Which characters have more similar voices than others, and which have more different voices? Based on the questions mentioned above, we combine the five characters in pairs to form a total of 10 pairs. According to the normality test results, parametric or non-parametric tests are conducted on the F0, CPP, and harmonic parameters of the voices. For each parameter, the ratio of significant differences in the 10 pairs is calculated as the discrimination rate of that parameter. The results show only significant differences among some parameters for some characters (see Table 5).
As can be seen from Table 5, CPP shows significant or highly significant differences between all 10 pairs (p < 0.05 or p < 0.01), suggesting CPP is significantly different among all five characters. F0 shows highly significant differences (p < 0.01 or p < 0.001) for each pair, except between M02 (Prince) and M05 (Minister). That is, with the exception of M02 (Prince)-M05 (Minister), the rest of the character pairs can be discriminated from each other by F0. For H1-A3, with the exception of M02 (Prince)-M05 (Minister), there are significant or highly significant differences between all pairs (p < 0.05 or p < 0.001). For H2-H4, with the exception of the pairs M01 (Monarch)-M04 (Eunuch) and M02 (Prince)-M03 (Physician), there are significant or highly significant differences between all pairs (p < 0.05 or p < 0.01). There is no significant difference for H1-H2 between the pairs M01 (Monarch)-M03 (Physician), M01 (Monarch)-M05 (Minister), and M02 (Prince)-M05 (Minister), and no significant difference for H4-H2k between the pairs M01 (Monarch)-M02 (Prince), M01 (Monarch)-M05 (Minister), and M02 (Prince)-M05 (Minister). For H2k-H5k, only six of 10 pairs shows significant difference (p < 0.05 or p < 0.01), while the differences between other pairs are not statistically significant.
The statistical tests reveal whether the voices of the five characters differ significantly on each of the seven acoustic parameters. The multi-dimensional scaling (MDS) analysis is adopted to show the distribution of the voices of the five characters in low dimensions more clearly. MDS is used to reduce the high-dimensional space of the distance between the characters' voices, measuring seven parameters including F0, CPP, H1-H2, etc., into a lower-dimensional space. The degree of dissimilarity of the five character voices in two dimensions is explored using the distance among characters (Torgerson, 1952; Cox and Cox, 2008). The results are shown in Figure 6.
Figure 6 demonstrates the distribution of the five voices in a two-dimensional space, with distances that reflect their mutual dissimilarity. According to the statistical result (Stress = 0.17, RSQ = 0.69), the goodness-of-fit indexes are fairly good. In terms of the degree of dissimilarity among the character voices, an intuitive interpretation of Figure 6 is that, the further apart the character voices in space, the more significant the difference in voice quality among the character voices; and conversely, the closer the distance, the more similar the voice qualities of the character voices. According to Figure 6, M02 (Prince) and M05 (Minister) are closer in the space, indicating that they are acoustically similar. Moreover, Table 5 also shows that in 57% of cases, the acoustic parameters do not discriminate between these two voices. M01 (Monarch) is also closer to M05 (Minister), and there is no significant difference between their acoustic parameters in 43% of the cases. The distances between M01 (Monarch), M02 (Prince), M03 (Physician), and M04 (Eunuch) are all farther apart from each other, indicating that they are acoustically different. Table 5 shows a significant difference between the acoustic parameters of all these four voices in 86% of cases. In terms of phonation types, this space can be divided into three parts depending on the degree of breathy voice, namely, obvious breathy voice (M03 Physician and M04 Eunuch), modal voice (M01 Monarch), and no obvious breathy voice (M02 Prince and M05 Minister). Dimension 1 can form a continuum from no breathy voice (right) to breathy voice (left). In terms of the relative social status of the characters, Dimension 2 can similarly divide the character voices into two parts, that is, higher relative social status (M01 Monarch) and lower relative social status (M02 Prince, M03 Physician, M04 Eunuch, and M05 Minister), forming a continuum concerning the relative social status of the characters.
The result of MDS in Figure 6 is constructed based on seven acoustic measures, while we need to further explore the extent to which each of these parameters is related to these two dimensions. Therefore, a correlation analysis was conducted between each acoustic parameter (averaged measurement for each character) and the coordinates of these characters in each MDS dimension. The results are shown in Table 6. The correlation coefficient between each acoustic parameter and each dimension represents the extent to which the parameter can explain the variation of the corresponding dimension. Specifically, the larger the absolute value of the correlation coefficient, the better this acoustic parameter reflects the corresponding dimension. In Table 6, H4-H2k, H1-H2, H1-A3, and F0 are strongly correlated with Dimension 1, suggesting that these four acoustic parameters can better explain the “degree of breathy voice”. The harmonic parameters are negatively correlated with Dimension 1, and F0 is positively correlated with Dimension 1, which is in line with the cross-linguistic studies indicating that “breathy voice may lead to a reduction in F0” (Liu et al., 2020; Liu, 2021). CPP and F0 correlate strongly with Dimension 2, suggesting that these two acoustic parameters better explain the “relative social status of the characters”. CPP is positively correlated with Dimension 2, and F0 is negatively correlated with Dimension 2, which is in line with previous research indicating that “dominant characters tend to use creaky voice” (e.g., Puts et al., 2006; Stern et al., 2021). As the “dominant” and “subordinate” roles in this study are analyzed in two dimensions: “degree of breathy voice” and “relative social status of the character”, the interpretation and prediction of role types using acoustic parameters also requires the two separate dimensions.
Table 6. Correlation coefficient between acoustic parameters and MDS coordinates (three decimal places).
The following two conclusions can be drawn from these results. First, only one of the seven parameters (i.e., CPP) can 100% discriminate the characters' voices, suggesting that it is difficult to completely distinguish the voices using a single acoustic parameter. Nonetheless, some consistent patterns can also be found in the data. For example, CPP, F0, and H1-A3 are the key acoustic indicators for distinguishing character voices. CPP has the highest discrimination rate (100%), F0 and H1-A3 have a higher discrimination rate (90%), followed by H2-H4 (80%), H1-H2 and H4-H2k (70%), and H2k-H5k the lowest (60%). Second, in the two-dimensional space, Dimension 1 can be interpreted as the degree of breathy voice, and Dimension 2 can be interpreted as the characters' social status level. In terms of similarity among character voices, the pair M02 (Prince)-M05 (Minister) and the pair M01 (Monarch)-M05 (Minister) are closer in space distance, and the discrimination rate between the acoustic parameters of each pair is mostly at a lower level, with relatively similar acoustic performances. M01 (Monarch), M02 (Prince), M03 (Physician), and M04 (Eunuch) are distant from each other in this space, and there are significant differences between a vast majority of their acoustic parameters, with the acoustic performance of the four being more distinctly different. Thus, the statistical test results for the acoustic parameters are consistent with the result of the MDS for acoustic distance, namely, character voices with significant differences between acoustic parameters being more acoustically distant, and conversely, character voices with smaller differences between acoustic parameters being less acoustically distant. Finally, for the interpretation and prediction of role types using acoustic parameters, these two separate dimensions are both required. H4-H2k, H1-H2, H1-A3, and F0 can better explain Dimension 1—“degree of breathy voice”; and CPP and F0 can better explain Dimension 2—“relative social status of the characters”.
It is well-known that voice acting aims to increase the audience's recognition of the character's image auditorily, with the ultimate goal of making a perfect combination of the re-creation using audible speech and the original character (Li, 2007). Based on the acoustic experiment, this section discusses the matching between acoustic parameters and character images, and the implications for guiding dubbing practice.
Firstly, in terms of pitch, adult males' larynx and pharyngeal cavities grow fast due to gender differentiation at puberty, causing an increase in the length and thickness of the vocal folds, leading to a significantly lower frequency of vocal fold vibration than in females. F0 has already been proven to be a reliable cue for distinguishing the voices of adult males and females in many previous studies (e.g., Murry and Singh, 1980; Honorof and Whalen, 2010). In general, low pitch is considered a distinctive feature of the adult male voice. In addition, cross-linguistic studies have found that the pitch range for normal adult male speech is between 80 and 180 Hz, and the range usually does not exceed 100 Hz, with a median of ca. 140 Hz (e.g., Baken and Orlikoff, 2000; Keating and Kuo, 2012; Kuang, 2013; Liu, 2019). According to section 3.1, the average F0s of all characters have a maximum of 128.57 Hz and a minimum of 96.83 Hz, all below 140 Hz, which is at the lower level of normal male pitch. Thus, the voices of all five characters are typically of heterosexual masculine temperament in pitch (Podesva, 2007; Cartei and Reby, 2012). Since the low pitch is somewhat in accord with the male voice's stereotype, it helps to further cast the character's image, making use of the audiences' perception of the lower pitch to position the character quickly. In terms of pitch range, except for M03 (Physician), the pitch range of all other characters' voices reached at least 145 Hz, with M02 (Prince) even reaching 230 Hz, making the pitch range much larger than normal male speech (80–180 Hz as mentioned above). As the F0 reflects not only the tonal information but also the intonational information, it is an important acoustic indicator for the expression of emotional intonation (Zhang et al., 2008). Therefore, intonation is generally changed through changing frequency (Zhang et al., 2021). Usually, there is a greater range of F0 variation for intense emotions such as cheerfulness and anger, and a smaller range of F0 variation for inhibited emotions such as calmness or sadness (Gao et al., 2005; Jia, 2017). Regarding the five characters, M02 (Prince) and M05 (Minister) have a higher pitch range with more dramatic change, making voices with a lilt, creating the image of gentle and affectionate literati who likes poetry, and a loyal minister who is impassioned and forthright in his advice, respectively. Thus, voice actors can exaggerate the pitch variation when dubbing a character, which helps to emphasize the character's feelings in the scene, and to enhance the actors' expressiveness and the audience's sense of immersion. At the same time, voice actors can also make use of the effect of pitch on perception, creating prototypical heterosexual male figures by lowering F0, as well as intensifying or suppressing emotions by increasing or decreasing F0 variation, in order to influence the audiences' perception of the plots and the character images, facilitating a better auditory portrayal of the characters.
Secondly, similar to the sound pattern of “frequency code”, numerous previous studies have shown that creaky voice is often associated with higher social status or greater dominance, whereas whispery voice and harsh voice are often associated with lower social status or role of subordinate (Esling, 1978; Ohala, 1984; Yuasa, 2010; Hornibrook et al., 2018; Tavi et al., 2019). This viewpoint is consistent with the results in section 3.4. The dominant characters, i.e., M01 (Monarch), M02 (Prince), and M05 (Minister), have no significant breathy voice compared to the other characters. On the contrary, the subordinate characters, i.e., M03 (Physician) and M04 (Eunuch), significantly use breathy voice. On this basis, in voice training or practice, voice actors can use modal or creaky voice to cast the dominant roles. The subordinate roles should be built using breathy voice. Based on the relationship between physiology, acoustics, and perception, a relationship between a type of character image and a specific voice quality can be established, so that the dubbed voice can be in accord with the character image, to match the audience's perception, and to enhance the credibility and impact of the dubbed characters. Moreover, cross-linguistic studies have shown that breathy voice is usually accompanied by a lower F0 compared to modal voice (Liu et al., 2020; Liu, 2021). M03 (Physician) and M04 (Eunuch) have stronger energy attenuation in low and low-mid frequencies, and there is a significant glottal leak during phonation, which causes lower F0, and pitch is lower than expected. Therefore, when dubbing for subordinate characters who require the use of breathy voice, the voice actor may choose to appropriately lower the pitch, in order to facilitate a smoother and sustained production of breathy voice, thus reducing the difficulty of the voicing.
Finally, voice plasticity is the prerequisite for voice actors to dub for different roles; however, since dubbing needs to fit the personal character, age, and other factors, the possible range of the voice actor's performance is limited by the quality of his/her voice quality, which is determined by the physiological conditions of the voice actor (Kreiman and Sidtis, 2011; Gao, 2013). The reconcilement of the character's image with the physical condition of the voice actor, and bringing out the uniqueness of the actor's voice, are vital considerations. For example, M04 (Eunuch) is a low-ranked and hard-working character, and the choice of an older voice actor (60-year-old, see Table 1) to dub his voice improved the perceived suitability between the voice and the character. From a physiological point of view, aging leads to atrophy of the vocal folds, which are part of the thyroarytenoid muscle, and a significant increase in glottal width, resulting in severe airflow leak through the vocal folds during phonation. This leads to a stronger attenuation in the voice's overall energy, and the appearance of obvious breathy voice (Fischer-Jørgensen, 1967; Dave, 1968; Winkler and Sendlmeier, 2006; Kreiman and Sidtis, 2011, p. 117; Gregory et al., 2012). In terms of acoustics, M04 (Eunuch) has a lower or the lowest spectral energy in most of the frequency ranges, and a strong energy attenuation. As a result, the dubbed voice of M04 (Eunuch) has a sense of fatigue due to its low energy, and a sense of humbleness due to the breathy voice. This voice quality also fits the character's image of being usually unable to speak loudly due to his low social status, which facilitates the portrayal of this character perfectly. Due to the physical limitations, all voice actors' voices are limited to some extent, so measuring the range of roles suitable for the physiological conditions of an actor can contribute, both to the producers' selection of voice actors, and to the personal career planning of a voice actor.
This study came to the following three conclusions utilizing acoustic analysis based on the dubbed voice of male characters from Empresses in the Palace.
First, the voice quality of the five characters has the following characteristics. Regarding F0, characters with higher social status use a low pitch, while those with relatively lower status adopt a high pitch. In terms of phonation type, the voice of male characters with higher social status do not use breathy voice notably, compared to the characters with lower status, who use breathy voice frequently. Thus, when dubbing for subordinate characters in Empresses in the Palace, voice actors tend to raise their pitch or to use breathy voice. On the contrary, low pitch and modal voice or creaky voice are applied to dominating characters in this TV series.
Second, although the dubbed voice of the five characters can be well-discriminated in auditory sensation, CPP is the only single acoustic parameter that can discriminate all five characters, followed by F0 and H1-A3. The three parameters mentioned above are the key acoustic indicators for discriminating character voices. Thus, multiple parameters are of great importance in discriminating character voices. Furthermore, existing parameters may not be enough for this purpose, so more fine-grained acoustic parameters shall be found to effectively discriminate character voices in the further study. Moreover, the results of statistical tests are consistent with the MDS. Characters with significant acoustic differences have greater distances in MDS, while those with fewer acoustic differences have shorter distances in MDS. M02 (Prince) and M05 (Minister) have similar character voices acoustically, and so do M01 (Monarch) and M05 (Minister). Character voices of M01 (Monarch), M02 (Prince), M03 (Physician), and M04 (Eunuch) have apparent differences between each pair.
Third, the findings of this study can also provide some guidance for the practice of voice acting. When dubbing, the voice actors need to quickly get in the scene, and to become one with their characters, which places high demands on the voice actors. In addition to experiencing the emotions of the characters and dubbing immersively, voice actors can improve their extent of fitting with the character in three ways. Firstly, they can exaggerate and typify some acoustic characteristics, such as pitch, to emphasize the character's image. Secondly, they can establish a relationship between a type of character image and a specific type of voice quality, linking character types to voice quality, in order to match the audience's expectations in perception, and to improve the expressiveness of the dubbed voices. Thirdly, they may judge the range of roles suitable for their physiological conditions, in order to improve the match between voice acting and character image, and also to reduce the difficulty of dubbing.
The raw data supporting the conclusions of this article will be made available by the authors, without undue reservation.
WL, XZ, and CL conceived and designed the study, participated in the statistical analysis, interpreted the data, and wrote the first draft of the manuscript. XZ collected the data. All authors contributed to the article and approved the submitted version.
This work was supported by National Social Science Foundation (No. 22CYY022) and Future Plan for Young Scholars of Shandong University.
The authors declare that the research was conducted in the absence of any commercial or financial relationships that could be construed as a potential conflict of interest.
All claims expressed in this article are solely those of the authors and do not necessarily represent those of their affiliated organizations, or those of the publisher, the editors and the reviewers. Any product that may be evaluated in this article, or claim that may be made by its manufacturer, is not guaranteed or endorsed by the publisher.
ANSI United States of America Standards Institute, and Acoustical Society of America (eds.).., (1960). Acoustical Terminology: ANSI S1.1-1960. New York, NY: American National Standards Institute.
Baken, R. J., and Orlikoff, R. F. (2000). Clinical Measurement of Speech and Voice, 2nd Edn. San Diego, CA: Singular Thomson Learning.
Belin, P., Fecteau, S., and Bedard, C. (2004). Thinking the voice: neural correlates of voice perception. Trends Cogn. Sci. 8, 129–135. doi: 10.1016/j.tics.2004.01.008
Blankenship, B. (2002). The timing of nonmodal phonation in vowels. J. Phon. 30, 163–191. doi: 10.1006/jpho.2001.0155
Boersma, P., and Weenink, D. (2021). Praat: Doing Phonetics by Computer. Version 6.1.37. Available online at: http://www.praat.org/ (accessed January 23, 2022).
Bonastre, J. F., Bimbot, F., Boë, L. J., Campbell, J. P., Reynolds, D. A., and Magrin-Chagnolleau, I. (2003). “Person authentication by voice: a need for caution,” in 8th European Conference on Speech Communication and Technology (Geneva), 33–36.
Callier, P. (2010). “Voice quality, rhythm and valorized femininities,” in Poster Session Presented at Sociolinguistics Symposium (Southampton), 18.
Callier, P. (2012). “Variation in phonation type. Distributions and meanings in a mass-mediated context,” in Poster Session Presented at New Ways of Analyzing Variation (Bloomington), 41.
Cao, H. L., and Kong, J. P. (2016). Correlations between vocal tract parameters and body heights in adult humans. J. Tsinghua Univ. 56, 1184–1189. doi: 10.16511/j.cnki.qhdxxb.2016.26.009
Cao, J. F. (2002). The relationship between tone and intonation in Mandarin Chinese. Stud. Chin. Lang. 3, 195–202+286.
Cartei, V., Garnham, A., Oakhill, J., Banerjee, R., Roberts, L., and Reby, D. (2019). Children can control the expression of masculinity and femininity through the voice. R. Soc. Open Sci. 6, 190656. doi: 10.1098/rsos.190656
Cartei, V., and Reby, D. (2012). Acting gay: male actors shift the frequency components of their voices towards female values when playing homosexual characters. J. Nonverbal Behav. 36, 79–93. doi: 10.1007/s10919-011-0123-4
Chen, W. R., Whalen, D. H., and Tiede, M. K. (2021). A dual mechanism for intrinsic F0. J. Phon. 87, 101063. doi: 10.1016/j.wocn.2021.101063
Cox, M. A., and Cox, T. F. (2008). “Multidimensional scaling,” in Handbook of Data Visualization, eds C. H. Chen, W. K. Härdle, and A. Unwin (Berlin, Heidelberg: Springer), 315–347.
Dave, R. (1968). A formant analysis of the clear, nasalized, and murmured vowels in Gujarati. Ann. Rep. Inst. Phonet. Univ. Copenh. 2, 119–132. doi: 10.7146/aripuc.v2i.130678
DiCanio, C. T. (2009). The phonetics of register in Takhian Thong Chong. J. Int. Phon. Assoc. 39, 162–188. doi: 10.1017/S0025100309003879
Ding, L. (2005). The composition and function of intonation in Putonghau. J. Shaanxi Univ. Technol. 3, 59–63.
Donen, S., Kelly, G., and Freed, A. (1952). Singing in the Rain. New York, NY: MGM/Pathe Home Video.
Eriksson, A. (2005). “Tutorial on forensic speech science,” in Proceedings of the 9th European Conference on Speech Communication and Technology (Lisbon), 4–8.
Esling, J. H. (1978). The identification of features of voice quality in social groups. J. Int. Phon. Assoc. 8, 18–23. doi: 10.1017/S0025100300001699
Esling, J. H., Moisik, S. R., Benner, A., and Crevier-Buchman, L. (2019). Voice Quality The Laryngeal Articulator Model. London: Cambridge University Press.
Esposito, C. M. (2006). The Effects of Linguistic Experience on the Perception of Phonation (Dissertation's thesis). University of California, Los Angeles, CA, United States.
Fischer-Jørgensen, E. (1967). Phonetic analysis of breathy (murmured) vowels in Gujarati. Ann. Rep. Inst. Phonet. Univ. Copenh. 2, 35–85. doi: 10.7146/aripuc.v2i.130674
Fónagy, I. (2001). Languages within Language. An Evolutive Approach. Amsterdam; Philadelphia, PA: John Benjamins.
Fritzell, B., Hallén, O., and Sundberg, J. (1974). Evaluation of Teflon injection procedures for paralytic dysphonia. Folia Phoniatr. Logopaed. 26, 414–421. doi: 10.1159/000263803
Gao, H., Su, G. C., and Chen, S. G. (2005). Acoustic features analysis of mandarin speech under various emotional status. Space Med. Med. Eng. 5, 350–354.
Gao, P. (2013). Study of Emotion in Film and Television Dubbing (Master's thesis). Henan University, Kaifeng, China.
Grawunder, S., and Winter, B. (2010). “Acoustic correlates of politeness: prosodic and voice quality measures in polite and informal speech of Korean and German speakers,” in International Conference for Speech Prosody 5 (Chicago, IL), 10–14.
Gregory, N. D., Chandran, S., Lurie, D., and Sataloff, R. T. (2012). Voice disorders in the elderly. J. Voice 26, 254–258. doi: 10.1016/j.jvoice.2010.10.024
Hansen, J. H., and Hasan, T. (2015). Speaker recognition by machines and humans: a tutorial review. Inst. Elect. Electron. Eng. Signal Process. Mag. 32, 74–99. doi: 10.1109/MSP.2015.2462851
Hartl, D. M., Hans, S., Vaissière, J., and Brasnu, D. F. (2003). Objective acoustic and aerodynamic measures of breathiness in paralytic dysphonia. Eur. Arch. Otorhinolaryngol. 260, 175–182. doi: 10.1007/s00405-002-0542-2
Hillenbrand, J., Cleveland, R. A., and Erickson, R. L. (1994). Acoustic correlates of breathy vocal quality. J. Speech Lang. Hear. Res. 37, 769–778. doi: 10.1044/jshr.3704.769
Hillenbrand, J., and Houde, R. A. (1996). Acoustic correlates of breathy vocal quality: Dysphonic voices and continuous speech. J. Speech Lang. Hear. Res. 39, 311–321. doi: 10.1044/jshr.3902.311
Hinton, L., Nichols, J., and Ohala, J. J., (eds.). (1994). Sound Symbolism. New York, NY: Cambridge University Press.
Honorof, D. N., and Whalen, D. H. (2010). Identification of speaker sex from one vowel across a range of fundamental frequencies. J. Acoust. Soc. Am. 128, 3095–3104. doi: 10.1121/1.3488347
Hornibrook, J., Ormond, T., and Maclagan, M. (2018). Creaky voice or extreme vocal fry in young women. N. Zeal. Med. J. 131, 36–40.
Iseli, M., Shue, Y. L., and Alwan, A. (2007). Age, sex, and vowel dependencies of acoustic measures related to the voice source. J. Acoust. Soc. Am. 121, 2283–2295. doi: 10.1121/1.2697522
Jia, H. M. (2017). [[Inline Image]][[Inline Image]][[Inline Image]]The Analysis of Chinese Emotional Intonation Based on the Discourse of Chinese Movies and Televisions (Master's thesis). Jinan University, Guangzhou, China.
Kawahara, S., Noto, A., and Kumagai, G. (2018). Sound symbolic patterns in Pokémon names. Phonetica 75, 219–244. doi: 10.1159/000484938
Keating, P., and Kuo, G. (2012). Comparison of speaking fundamental frequency in English and Mandarin. J. Acoust. Soc. Am. 132, 1050–1060. doi: 10.1121/1.4730893
Klatt, D. H., and Klatt, L. C. (1990). Analysis, synthesis, and perception of voice quality variations among female and male talkers. J. Acoust. Soc. Am. 87, 820–857. doi: 10.1121/1.398894
Kreiman, J., Gerratt, B. R., Garellek, M., Samlan, R., and Zhang, Z. (2014). Toward a unified theory of voice production and perception. Loquens 1, e009. doi: 10.3989/loquens.2014.009
Kreiman, J., and Sidtis, D. (2011). Foundations of Voice Studies: An Interdisciplinary Approach to Voice Production and Perception. Oxford: Wiley-Blackwell.
Kreiman, J., Vanlancker-Sidtis, D., and Gerratt, B. R. (2005). “Perception of voice quality,” in The Handbook of Speech Perception, eds D. B. Pisoni and R. E. Remez (Oxford: Blackwell Publishing), 338-361.
Kuang, J. (2013). The tonal space of contrastive five level tones. Phonetica 70, 1–23. doi: 10.1159/000353853
Ladefoged, P. (2003). Phonetic Data Analysis: An Introduction to Fieldwork and Instrumental Techniques. Oxford: Wiley-Blackwell.
Laver, J. (1980). The phonetic description of voice quality. Camb. Stud. Linguist. London 31, 1–186.
Leino, T. (2009). Long-term average spectrum in screening of voice quality in speech: untrained male university students. J. Voice 23, 671–676. doi: 10.1016/j.jvoice.2008.03.008
Li, K. P., Hughes, G.W., and House, A. S. (1969). Correlation characteristics and dimensionality of speech spectra. J. Acoust. Soc. Am. 46, 1019–1025. doi: 10.1121/1.1911794
Lippi-Green, R. (2012). English with an Accent: Language, Ideology, and Discrimination in the United States. London: Routledge.
Liu, W. (2019). An acoustic and perceptual study of the five level tones in Hmu (Xinzhai Variety). Chin. J. 1, 79–87. doi: 10.21437/Interspeech.2020-0056
Liu, W. (2021). Physiological and physical basis of phonation types and its linguistic value. Essays Linguist. 1, 204–233.
Liu, W., Lin, Y. J., Yang, Z., and Kong, J. P. (2020). Hmu (Xinzhai variety). J. Int. Phon. Assoc. 50, 240–257. doi: 10.1017/S0025100318000336
Mendoza, E., Valencia, N., Muñoz, J., and Trujillo, H. (1996). Differences in voice quality between men and women: use of the long-term average spectrum (LTAS). J. Voice 10, 59–66. doi: 10.1016/S0892-1997(96)80019-1
Miramont, J. M., Restrepo, J. F., Codino, J., Jackson-Menaldi, C., and Schlotthauer, G. (2020). Voice signal typing using a pattern recognition approach. J. Voice 36, 34–42. doi: 10.1016/j.jvoice.2020.03.006
Mixdorff, H., Niebuhr, O., and Hönemann, A. (2018). “Model-based prosodic analysis of charismatic speech,” in Proceedings of 9th International Conference of Speech Prosody (Poznan), 814–818.
Moisik, S. R. (2012). Harsh voice quality and its association with blackness in popular American media. Phonetica 69, 193–215. doi: 10.1159/000351059
Morton, E. S. (1977). On the occurrence and significance of motivation-structural rules in some bird and mammal sounds. Am. Nat. 111, 855–869. doi: 10.1086/283219
Murry, T., and Singh, S. (1980). Multidimensional analysis of male and female voices. J. Acoust. Soc. Am. 68, 1294–1300. doi: 10.1121/1.385122
Noble, L., and Xu, Y. (2011). “Friendly speech and happy speech-are they the same?,” in 17th International Congress of Phonetic Sciences (Hong Kong), 1502–1505.
Ohala, J. J. (1984). An ethological perspective on common cross-language utilization of F0 of voice. Phonetica 41, 1–16. doi: 10.1159/000261706
Ohala, J. J. (1994). “The frequency code underlies the sound-symbolic use of voice pitch,” in Sound Symbolism, eds L. Hinton, J. Nichols, and J. J. Ohala (New York, NY: Cambridge University Press), 325–347.
Pépiot, E. (2014). Male and female speech: a study of mean F0, F0 range, phonation type and speech rate in Parisian French and American English speakers. In Speech Prosody 7, 305–309. doi: 10.21437/SpeechProsody.2014-49
Pittam, J. (1987). The long-term spectral measurement of voice quality as a social and personality marker: a review. Lang. Speech 30, 1–12. doi: 10.1177/002383098703000101
Podesva, R. J. (2007). Phonation type as a stylistic variable: the use of falsetto in constructing a persona. J. Sociolinguist. 11, 478–504. doi: 10.1111/j.1467-9841.2007.00334.x
Podesva, R. J., and Callier, P. (2015). Voice quality and identity. Annu. Rev. Appl. Linguist. 35, 173–194. doi: 10.1017/S0267190514000270
Puts, D. A., Gaulin, S. J. C., and Verdolini, K. (2006). Dominance and the evolution of sexual dimorphism in human voice pitch. Evol. Hum. Behav. 27, 283–296. doi: 10.1016/j.evolhumbehav.2005.11.003
Rallabandi, S. S., Naderi, B., and Möller, S. (2021). “Identifying the vocal cues of likeability, friendliness and skilfulness in synthetic speech,” in Proceedings of 11th International Speech Communication Association Speech Synthesis Workshop (Budapest), 1–6.
Sapir, E. (1929). A study in phonetic symbolism. J. Exp. Psychol. 12, 225–239. doi: 10.1037/h0070931
Shaw, B., and Fisher, J. (1963). Pygmalion. Melbourne, VIC: Royal Victorian Institute for the Blind Educational Centre.
Shue, Y. L., Keating, P., Vicenik, C., and Yu, K. (2009). VoiceSauce: a program for voice analysis. J. Acoust. Soc. Am. 126, 2221. doi: 10.1121/1.3248865
Signorello, R., Derrico, F., Poggi, I., and Demolin, D. (2012). “How charisma is perceived from speech: a multidimensional approach,” in 2012 International Conference on Privacy, Security, Risk and Trust and 2012 International Conference on Social Computing (Amsterdam), 435–440.
Sjölander, K., and Beskow, J. (2000). “Wavesurfer-An open source speech tool,” in 6th International Conference on Spoken Language Processing (Beijing), 464–467.
Starr, R. L. (2015). Sweet voice: the role of voice quality in a Japanese feminine style. Lang. Soc. 44, 1–34. doi: 10.1017/S0047404514000724
Stern, J., Schild, C., Jones, B. C., DeBruine, L. M., Hahn, A., Puts, D. A., et al. (2021). Do voices carry valid information about a speaker's personality? J. Res. Pers. 92, 104092. doi: 10.1016/j.jrp.2021.104092
Tavi, L., Alumäe, T., and Werner, S. (2019). “Recognition of creaky voice from emergency calls,” in 20th Interspeech Conference (Graz), 1990-1994.
Teshigawara, M. (2003). Voices in Japanese Animation: A Phonetic Study of Vocal Stereotypes of Heroes and Villains in Japanese Culture (Dissertation's thesis). University of Victoria, Victoria, BC, Canada.
Tong, K. H., and Moisik, S. R. (2021). Detecting protagonists and antagonists in the voice quality of American cartoon characters: a quantitative LTAS-based analysis. Phonetica 78, 345–384. doi: 10.1515/phon-2021-2009
Torgerson, W. S. (1952). Multidimensional scaling: I. Theory and method. Psychometrika 17, 401–419. doi: 10.1007/BF02288916
Van Lancker, D., Kreiman, J., and Emmorey, K. (1985). Familiar voice recognition: patterns and parameters part I: recognition of backward voices. J. Phon. 13, 19–38. doi: 10.1016/S0095-4470(19)30723-5
Weiss, B., Trouvain, J., Barkat-Defradas, M., and Ohala, J. J. (2021). Voice Attractiveness: Studies on Sexy, Likable, and Charismatic Speakers. Singapore: Springer.
Winkler, R., and Sendlmeier, W. (2006). EGG open quotient in aging voices—Changes with increasing chronological age and its perception. Logoped. Phoniatr. Vocol. 31, 51–56. doi: 10.1080/14015430500445534
Wu, Q., Liu, Y., Li, D., Leng, H., Iqbal, Z., and Jiang, Z. (2021). Understanding one's character through the voice: dimensions of personality perception from Chinese greeting word “Ni Hao”. J. Soc. Psychol. 161, 653–663. doi: 10.1080/00224545.2020.1856026
Yang, L. (2021). Artistic creation and diversified expression of film and television dubbing. Media Forum 4, 113–114.
Yang, Z., Huynh, J., Tabata, R., Cestero, N., Aharoni, T., and Hirschberg, J. (2020). “What makes a speaker charismatic? Producing and perceiving charismatic speech,” in Proceedings of 10th International Conference on Speech Prosody (Tokyo), 685–689.
Yang, Z. S. (2021). On the ability of artistic creation in film and television dubbing in the era of intelligent media. J. Commun. 22, 101–102.
Yuasa, I. P. (2010). Creaky voice: a new feminine voice quality for young urban-oriented upwardly mobile American women?. Am. Speech 85, 315–337. doi: 10.1215/00031283-2010-018
Zhang, F., Huang, L., Chao, X., Shi, Y., and Qu, C. Y. (2021). Acoustic characteristics of vocal emotion sound of hearing-impaired children and normal hearing children aged 3~5. J. Audiol. Speech Pathol. 29, 146–150.
Zhang, P., Wang, L. H., and Liu, S. (2008). “On fundamental frequency contour synthesis and control method for Chinese speech synthesis,” in Proceedings of the 27th Chinese Control Conference (Kunming), 3211–3214.
Keywords: character voice, male character, social status, voice quality, acoustic analysis
Citation: Liu W, Zhang X and Liang C (2023) An acoustic study on character voices of dominators and subordinates: A case study on male characters in Empresses in the Palace. Front. Commun. 7:1088170. doi: 10.3389/fcomm.2022.1088170
Received: 03 November 2022; Accepted: 07 December 2022;
Published: 09 January 2023.
Edited by:
Plinio Almeida Barbosa, State University of Campinas, BrazilReviewed by:
Sandra Madureira, PUCSP, BrazilCopyright © 2023 Liu, Zhang and Liang. This is an open-access article distributed under the terms of the Creative Commons Attribution License (CC BY). The use, distribution or reproduction in other forums is permitted, provided the original author(s) and the copyright owner(s) are credited and that the original publication in this journal is cited, in accordance with accepted academic practice. No use, distribution or reproduction is permitted which does not comply with these terms.
*Correspondence: Changwei Liang, bGlhbmdjaGFuZ3dlaUBzZHUuZWR1LmNu
†These authors have contributed equally to this work and share first authorship
Disclaimer: All claims expressed in this article are solely those of the authors and do not necessarily represent those of their affiliated organizations, or those of the publisher, the editors and the reviewers. Any product that may be evaluated in this article or claim that may be made by its manufacturer is not guaranteed or endorsed by the publisher.
Research integrity at Frontiers
Learn more about the work of our research integrity team to safeguard the quality of each article we publish.