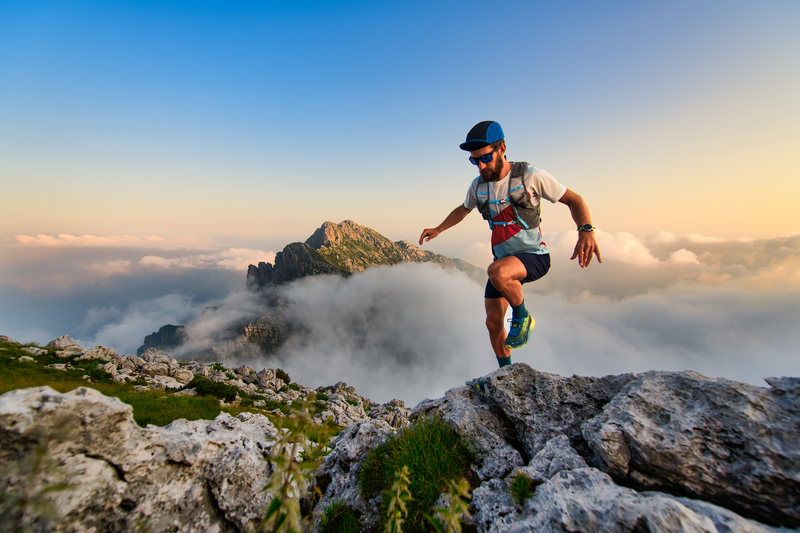
95% of researchers rate our articles as excellent or good
Learn more about the work of our research integrity team to safeguard the quality of each article we publish.
Find out more
ORIGINAL RESEARCH article
Front. Commun. , 16 December 2022
Sec. Health Communication
Volume 7 - 2022 | https://doi.org/10.3389/fcomm.2022.1080948
This article is part of the Research Topic Rethinking Global Health and Communication View all 7 articles
The incorporation of widespread, high-quality prevention campaigns and health communication is an integral part of universally accessible healthcare systems. Importantly, in the context of COVID-19, effective public health communication has proven a key mitigating factor. Considering the global differences in countries' universal health coverage, the scope of this study is to formally compare how governments around the globe communicated at the onset of the pandemic. Health communication research has traditionally focused mainly on practices within particular systems, whereas the global scale of the pandemic provides the opportunity to widen the analysis to differences between systems. In this study, 66,167 tweets from 324 government leaders, health ministers and ministries from 139 countries were analyzed using computational content analysis (i.e., topic modeling). The results show that as the pandemic initially intensified, countries with lower degrees of access to universal healthcare were inclined to communicate differently than countries with widely accessible and strongly equipped health care systems. More specifically, the former compensated their structural vulnerabilities and lack of tradition in health communication by highlighting individual and community responsibilities over government measures. In contrast, the latter countries emphasized the aptness of their healthcare systems and infrastructures.
During the first half of 2020, the novel coronavirus spread globally. Practically every nation around the globe was confronted with this challenge, regardless of their structural aptness to deal with the situation in terms of medical resources and the capacity and performance of their health care systems. Some countries were better equipped than others to handle the situation. A common factor though, was that in dealing with this health crisis, authorities had to rely on their persuasive capacities to inform and sensitize the public. After all, in the absence of unified, agreed upon prevention and treatment strategies (McAteer et al., 2020), governments had to rely on traditional, yet drastic and widely unpopular public health response practices to control their outbreaks. These included physical distancing, quarantine and isolation, as well as the widespread use of protective equipment such as facemasks that, however, were not always sufficiently available (Bai et al., 2020; Khurana et al., 2020; Wu and McGoogan, 2020) and were often resisted (Hornik et al., 2021).
Considering communication with the public to be a key in mitigating the pandemic, the scope of this study is to formally compare and further explore how governments around the globe communicated at the onset of the pandemic. While the literature leaves no doubt that effective and efficient communication is crucial in healthcare, it mostly focuses on communication within a given healthcare system (Nutbeam and Lloyd, 2021). It has a micro or meso-level focus on interactions between health organizations, medical professionals and patients, assessing communicative practices and (in)efficiencies. In this study, we widen the scope to a macro level through a global comparison between healthcare systems as operationalized through the degree of universal healthcare coverage. Particular to the situation of the pandemic is the infusion of politics and governmental communication. COVID-19 forced political actors to engage in public health communication, which is characterized by its own practices and is affected by social, economic, and political factors at the national and regional levels (Schiavo, 2013). There is no doubt that political power and social structures influence the health care system (Gore and Parker, 2019) or that public health is always political (McCartney et al., 2019). Surprisingly, this macro level approach appears to be a relative gap in the literature and the global scale of the pandemic presents itself as an opportunity for comparative empirical exploration and subsequent theory building.
The study's guiding hypothesis is that countries with lower degrees of access to universal health care will be inclined to communicate differently than countries with widely accessible and strongly equipped health care systems. More specifically, we test the presumption that governments of countries with relatively lower access to universal health care compensated their structural vulnerabilities and lack of tradition in health communication by highlighting individual and community responsibilities over government measures and the aptness of the health care system. This overarching hypothesis is tested through the analysis of the official Twitter communication of government leaders and key health officials around the world (i.e., 139 countries). During the onset of the pandemic, from January to May 2020, a corpus of over 65,000 tweets was gathered. Automated topic modeling is used to distinguish between the key themes in those tweets. The evolution of those themes is subsequently analyzed using conditional longitudinal growth models, testing differences for varying degrees of universal health care access on the country level, while controlling for political and economic differences (i.e., countries' gross domestic product and democratic performance respectively).
According to the WHO (2019) about half of the world population lacks access to essential health services without falling into financial hardship, provided that the necessary care would even be available. Figure 1 shows a world map of Universal Health Coverage (UHC) based on data sourced from the World Health Organization (WHO, 2020). The scores are numeric operationalisations with a range from 0 to 100, reflecting the average coverage of essential health services in a country, including “reproductive, maternal, newborn and child health, infectious diseases, non-communicable diseases and service capacity and access, among the general and the most disadvantaged population”. These essential services cover the entire spectrum from prevention to active treatment and rehabilitation. There are clear global divions in UHC as it is generally more limited in the Sub-Saharan and South-East Asia regions, while Western-Europe and North-America generally enjoy much greater healthcare resources. A lack of access to public healthcare has been identified as a key driver of excess mortality rates across various conditions, including cardiovascular diseases and communicable disorders (Kruk et al., 2018). Conversely, there is evidence that shows that broadening health coverage generally, leading to improved access to health care, in turn improves population health (Moreno-Serra and Smith, 2012).
Taking steps toward the implementation of universal health coverage obviously requires significant government expenditure, accounting for at least five percent of the gross domestic product (McIntyre et al., 2017). Such level of investment is particularly challenging to attain for low and middle-income countries, explaining why its implementation goes hand in hand with economic development (Savedoff et al., 2012; Dieleman et al., 2018). In spite of international pressure to foreground universal health coverage as a development priority, the political path toward investing in and implementing accessible public health care remains cumbersome. Besides the availability of and the willingness to allocate funds, it needs an adequate mindset. Beyond political turmoil, the path to universal health coverage is possibly obstructed by pushback by medical professionals (Vian et al., 2015) or even opposition by (parts of) the public (Gollust and Lynch, 2011; Blendon and Benson, 2017). And, related, it requires stable political coalitions that ideologically align with the idea of considering equity in healthcare as a public good or even as a human right (Yamin and Frisancho, 2015), rather than an individual responsibility (Atun et al., 2013, 2015; Chemouni, 2018; Lavers, 2019). Even in developed countries with seemingly established universal health care access, the magnitude of spending is a recurrent topic of debate especially in times of budgetary austerity (Reeves et al., 2015).
It should be emphasized that universal health coverage entails more than mere access to medical services, but also comprises the accessibility and quality of prevention campaigns and health communication (WHO, 2019). As Royston et al. (2020) argue, wide diffusion and access to proper, actionable health information empowers the public to protect and manage their own health and that of significant others. They especially highlight the value of governments, policymakers and health professionals in providing the necessary resources. It has been widely argued that effective health communication and promotion increases health literacy, which is in turn associated with healthier behaviors and healthcare utilization (Berkman et al., 2011; Sørensen et al., 2015).
For most countries, COVID-19 evolved from a distant threat to an immediate, unpredictable risk. The scale of the pandemic became a harsh reality for practically all countries around the globe, regardless of their structural preparedness. COVID-19 stressed health care systems' capacities to test for cases and to treat affected patients. Prior analyses have shown that particularly wealthy countries have been hit by the virus (Cash and Patel, 2020), suggesting that they account for the highest case fatality rates (Dongarwar and Salihu, 2020). A superficial conclusion might be that there is no, or even a negative relationship with universal health coverage and the outcomes of an active outbreak. However, as Cash and Patel (2020) argue, low and middle-income countries have different demographic pyramids and have far fewer seniors who live in retirement homes – a demographic that is particularly at risk. And, it is likely that there is less mobility that allows the virus to spread rapidly.
In spite of those findings, it has been shown that within countries, COVID-19 has disproportionally hit vulnerable populations, in particular ethnic minorities and socially disadvantaged seniors (Blumenthal et al., 2020; Calderón-Larrañaga et al., 2020; Fortuna et al., 2020; Viswanath et al., 2020). Beyond this social stratification within countries, the limited internationally-focused evidence suggests severe inequalities between countries as well (Shadmi et al., 2020). This amplified the call for the need to invest in strong, generally accessible and properly equipped healthcare infrastructures during the height of the early outbreaks (Armocida et al., 2020; Galvani et al., 2020; Iyengar et al., 2020; Tediosi et al., 2020).
It is fair to argue that for any country involved, the pandemic was an unknown, realistic threat. Governments were forced to respond quickly, devise measures, and communicate with the public. This has proven a considerable challenge, leading to unclear, mixed messaging and even misinformation that confused and divided publics (Noar and Austin, 2020; Mohd Hanafiah et al., 2021). Governments had to carefully weigh national interests and the global dimensions of the crisis, public health and the economic implications of health measures, and citizens' and institutions' responsibilities in mitigating the crisis. Authorities have been historically criticized for their slow initial responses in public health crises (Tirkkonen and Luoma-aho, 2011; Li et al., 2014; Guidry et al., 2017, 2020). Added to that, political communication is susceptible to spin, especially as it spreads online (Shin et al., 2018; Tucker et al., 2018). And although in this case, the responsibility to focus on public health governance and leadership likely took primacy (Guest et al., 2020), it is unrealistic that typical influences such as ideology and nationalist sentiments (Karwowski et al., 2020), as well as populist tendencies to appease the electorate (Cowper, 2020) entirely disappeared.
COVID-19 forced political actors to engage in public health communication, regardless of whether they were accustomed to that or not. As argued, wider access to universal health coverage goes hand in hand with a mature culture of professional, reliable health communication. As such, the scope and quality of health communication is inevitably a function of social, economic, and political factors (Schiavo, 2013). The situation vastly differs per country, not in the least for the public health resources that are available, their public accessibility, and the overall rate of health literacy. In this study, we particularly hypothesize that the nature of the initial COVID-19 response, and how governments globally communicated about it, is explained by the degree to which health resources were available to the public. This is expressed in the varying degrees in universal health coverage. More specifically, we predict that countries with limited access and fewer resources handled that lack of resources by emphasizing individual and the public's responsibility in mitigating the pandemic risk. Conversely, we expect that countries with a strong degree of universal health coverage relied on that strength and highlighted their preparedness and availability of medical resources.
This study aims to (a) explore the nature of governments' public communication at the onset of COVID-19 and (b) to test predictions on national differences in that communication based on varying degrees of structural universality of health coverage in those countries. This requires sampling comparable government communication that is indicative of the nature of a nation's response. This study relies on Twitter data posted by government leaders and health officials around the world. Early research on individual countries has indicated how the pandemic widely sparked conversation on social media (Park et al., 2020; Poirier et al., 2020). On Twitter, COVID-19 was the most trending and talked about issue online in early 2020. Both politicians and health officials have weighed in on these discussions and used the platform to communicate with journalists, opinion leaders, and the general public. This renders it a particularly suitable resource to probe governments' responses and the nature of the communication on these responses.
A list of affected countries was sourced from the World Health Organization. For each country, we manually searched Google for the names of the country's leadership (i.e., president, vice-president, prime minister when applicable), the name of its health minister and the official health department name. Next, for each actor, a Google Search query was run with a search string that combined the country, the name, and “Twitter official” (e.g., “Australia + Scott + Morrison + twitter + official”). The top results were closely inspected to verify whether they redirected to a Twitter account that legitimately belonged to that person or health department. If that was the case, the Twitter handle was added to a database. A total of 472 of Twitter handles were collected, dispersed over 185 countries worldwide.
Subsequently, on May 2nd, all tweets that were posted by these accounts from the first of January 2020 until April 30th, 2020 were exhaustively sourced and collected from Twitter. In total, 94,461 unique tweets were collected.
The collected tweets were then translated using the Google Translate Application Programming Interface (API). This API automatically detects the source language of a text string that is sent with a request and returns a translation to a language of choice. In this case, the tweets were translated to English. The tweets that were already posted in English remained untouched. The language of the translated tweets was cross-checked, using the “langdetect” Python module. Only tweet translations that were post hoc identified as English were retained (N = 78,536).
Next, all tweets were cleaned with the prospect of automated text analysis. The actual analysis, with the aim to find distinct topics within the bulk of tweets, relied on Latent Dirichlet Allocation (LDA) topic modeling (Blei et al., 2003; Jelodar et al., 2019). This is an unsupervised machine learning approach to discover abstract topics that occur in unstructured text documents. It groups together keywords that co-occur with high frequency, which together are expected to reflect a distinct topic. A single document can be covered by multiple topics to a varying extent.
A first step in cleaning was to remove all distracting components from the tweets. Using regular expressions – which is a commonly used tool for pattern detection in strings – all punctuation, numbers, @ mentions, hashtags (#), URLs, and indications of retweets were removed from the tweet texts. Next, words that occurred more than a hundred times in the corpus were visually inspected by the researchers. This led to a compact list of non-sensical letter combinations and words that failed to translate. Items from this list were either removed from the tweets or replaced by corrected translations. This was also the case for all words that indicate a certain time as the timing of the tweet itself is later on incorporated as an explanatory variable. Finally, all of the tweets were scanned for references to countries. If the named country was self-referential, the substring was automatically replaced by “self-reference” in the tweet. When it referred to another country, it was replaced by “other-reference”. This was done for all countries except China, as this country was expected to be central in varying topics.
Subsequently, all stop words, were automatically identified and removed using the “NLTK” English stop word repository. Stop words are common words that do not carry much (or any) distinctive meaning. This was followed by a lemmatisation of each tweet to group inflective word forms. This was accomplished using the “SpaCy” Python module. As a result, we produced a cleaner, more homogenous, and easier to interpret corpus.
Finally, the tweets were tokenized and written into a vocabulary. This is the collection of words present in the corpus. To get to this vocabulary, each tweet was transformed into a list of its lemmatised words (i.e., tokens). Bigrams and trigrams were included as well, which are the combinations of respectively two or three adjacent words. Only words, bigrams and trigrams with an absolute count of at least 50 and an occurrence in no more than ten per cent of the tweets were retained. This ensures that both all too obscure and too common, indistinctive elements are ignored. In total, the vocabulary consisted of 38,378 unique tokens.
Universal health coverage index is a measure obtained from the World Health Organization (WHO, 2019) for 174 countries (Figures 2, 3). It reflects the average coverage of essential health services in a country, including “reproductive, maternal, newborn and child health, infectious diseases, non-communicable diseases and service capacity and access, among the general and the most disadvantaged population”. The index is scaled from 0 to 100.
The following variables were added with the purpose to serve as control variables. As argued, the path to the implementation of universal health care includes the necessary financial means and a political platform that is supported by the public (Savedoff et al., 2012). To account for the effect of a country's wealth and a democratic openness, the following measures – alongside the progression of the pandemic – are factored in.
Gross Domestic Product in billions of US dollars was sourced from the World Bank, with data available for the year 2018 (IBRD-IDA, 2020).
Democracy Index is a compound measure developed by the Economist Intelligence Unit (EIU, 2020), available for 167 countries. The measure is based on 60 indicators that are grouped in five categories: electoral process and pluralism, functioning of government, political participation, political culture, and civil liberties. The index is scaled 0 to 10. The 2019 version was used in the analysis (EIU, 2020).
Progression of the pandemic consists of three measures, obtained through humdata.org (2020), original sourced from the John Hopkins Institute. It consists of the absolute progression of number of (a) confirmed cases, (b) deaths, and recoveries registered COVID-19 related deaths per country, per day from 22 January 2020 until May 1st, 2020.
Because these additional measures are not available for all countries, the complete dataset was ultimately reduced to 66,167 tweets nested in 324 Twitter handles from 139 countries.
Before we can test our predictions on the differences in the nature of government communication tied to differences in universal health coverage, it is necessary to exhaustively map out the nature of that communication.
In order to find meaningful patterns in this large corpus of texts, we used the Mallet integration of “GENSIM”, which is a natural language processing package for Python. The algorithm iteratively runs through the documents, which in this case are lemmatized tweets. It looks for words present in the used vocabulary, which is a repository of single words (and in this case also bigrams and trigrams). Based on empirical co-occurrence, the algorithm assigns related words to a predefined number of k topics, and iteratively refines that allocation. A common approach to identify a suitable number of topics, is to repeat the analysis with an increasing number of k topics. For each model with k topics, measures of coherence are calculated. In this analysis, the C_v coherence statistic was considered (Kapadia, 2019).
The plot in Figure 4 shows the progression of model coherence measures when the topics increase. Each model was run with 2,000 iterations. A tried approach is to preferably select a parsimonious model with a sufficiently high coherence score. In this case, coherences increase with a higher number of topics, peaking at ten topics (C_v = 0.52), which is a relatively substantial score, especially taking into account the limited length of the documents that it is based on (i.e., short tweets).
Still, despite availability of a mathematical benchmark, the decision to adopt the ten-topic solution depends on the extent to which it makes interpretative sense. The following paragraphs sketch out the contours of these topics (Table 1). At the same time, they are analyzed longitudinally, considering a key set of structural, explanatory variables. More specifically, hierarchical regression models explain the representation of each topic in the body of tweets (Run in R, using the LME package). The key explanatory variables are universal healthcare coverage and time, whereas source of the tweet, democracy indices, and gross domestic product are included as control variables. The statistically significant effects in Table 2 are integrated in the discussion of the topics below. Figures 5–7 visualize the significant interaction effects of region, universal health coverage, gross domestic product, and democratic performance with time.
Figure 6. Interaction plots showing time lines per topic, for varying levels of universal health coverage and GDP.
Topic 1: Government preventive measures is characterized by keywords that highlight the role of the government in taking measures and inciting action to control the pandemic. During the 1st months of the pandemic, this topic generally increased in prominence, and further went up with growing numbers of confirmed cases and deaths. Countries that score lower on universal health coverage and have a higher democracy index showed a steeper increase in this topic throughout time. This type of communication predominantly originates from the government leadership, rather than health ministers/bodies. Whereas Eastern European countries show a steeper increasing slope, Western European countries tend to show a less pronounced increase.
Topic 2: Availability of the health department highlights the efforts of health ministries in being available for the public. There are no differences between countries that are explained by the degree universal health care coverage. Overall, countries in the Middle East, North Africa, and the Sub-Saharan region display a stronger overall increase in tweeting on this topic. This communication usually originates from the health ministers and the health ministries.
Topic 3: Economic support is made up by dominant lemma's that focus on economic activity and government measures to support it, predominantly tweeted by government leaders. The results also show additional decline for this topic in countries with a stronger universal health coverage, and also a higher GDP and democratic index. In general, this kind of discourse decreases during the pandemic. A noticeable exception is North America, where the topic of economic support increases, while it declines at a higher rate in Australasian and Sub-Saharan countries.
Topic 4: National press briefings and media referrals represents language that informs on situational updates with regards to the nation, which is more often communicated by health ministers and ministries. This presence of this topic gradually declined, especially in the Australasian countries that were affected first. Surprisingly, we find a decreasing rate in North American countries, which might be in large explained by the particular political situation in the US. The overall decline in the topic is less pronounced as confirmed cases go up, and for countries with higher GDP and a lower democracy index. Finally, there is no apparent relation with universal health coverage.
Topic 5: Availability of medical resources, usually uttered by health ministers and ministries, combines language on the status of the healthcare system, highlighting the availability and support of medical staff. Overall, this topic increases more strongly in tweets from countries with higher universal health coverage, as well as a higher GPD and a higher democracy index. Mentions of available medical resources generally go up over time, especially in Australasian, Eastern European and Sub-Saharan countries. However, there is again a noticeable decrease for North American countries.
Topic 6: Confirmed cases and death toll provides statistics on the status of the pandemic. The data show a strong increase for Sub-Saharan countries, while there is a noticeable strong increase over time for countries with lower GDP and lower democracy indices. There is no relation with universal health coverage. This topic is more pronounced in the tweets of health departments and ministers, and it obviously positively associated with the number of confirmed cases and deaths.
Topic 7: Support and solidarity contains language that emphasizes love and solidarity, explicitly referencing the people (as fellow citizen, as members of a family). It increases over time for countries with lower universal healthcare coverage and is also more pronounced for countries with lower GDP and a higher democracy index. This kind of discourse is more dominant in the tweets of government leaders. It increases over time in North American countries (where it is overall more present) and declines for the Sub-Saharan region.
Topic 8: Individual preventive measures is made up by lemmas that reference preventing the spread of the virus (e.g., staying at home, hand hygiene, and wearing face masks), mostly by health ministers and ministries. It increases over time in countries with lesser universal health coverage and countries with higher democracy indices. These recommendations increase over time especially when confirmed cases and deaths go up. The rate of increase is slightly more pronounced in Western Europe and Australasia.
Topic 9: International relations refers to relations between the countries' officials. It is only mildly related to the pandemic, as only 26% of the tweets that predominantly fit this topic explicitly references the pandemic. Therefore, it could be considered as a continuation of “business as usual”. Overall, this topic declines in prominence, especially for countries with lower degrees of universal health coverage and a lower GDP. It also declines at a slower rate in Australasian and Latin American countries.
Topic 10: (Inter)national celebration is made up by language that focuses on congratulating people, both with a national and international focus. This effect relatively decreases stronger over time in countries with lower universal health coverage, GDP, and a higher democracy index. For example, we can see a spike around the 8th of March, which was international women's day. This generally positive and subjectively phrased topic declines during the pandemic, which is increasingly so when confirmed cases and death toll rise. Similar to the previous one, this topic is likely an indication of communication as it would occur before the pandemic.
Drawing on a computational content analysis, ten distinct themes were identified within the corpus of tweets gathered from government Twitter accounts around the globe during the first months of the pandemic. This computational approach allowed to cope with the magnitude and linguistic diversity of the data that would otherwise not be feasible to code manually. The extracted themes are indicative for the breadth and depth of the discourse. This discourse divided mainly into communication with a focus on public health, as opposed to more general themes in political communication, such as economic and international relations.
As the pandemic spread, typical political issues, and arguments communicated by government leaders made way for health communication. This shift is clearly indicated by the increasing dominance of topics related to managing the pandemic: the preventive measures issued by the government, the emphasis on the availability of the health department, the availability of medical resources, the pressure to adopt individual preventive measures, national press briefings, and statistics on confirmed cases and deaths. Most of these topics are slightly more present in the communication by health ministers and ministries, rather than the government leadership (except for preventive measures by the government). Still, it is clear that government leaders did not shy away from the responsibility to inform the public on the COVID response.
The central hypothesis was that countries with varying degrees of universal healthcare coverage communicated differently throughout the onset of the pandemic. The results generally support this prediction. Countries with a strong healthcare coverage highlighted their availability of medical resources. As argued, strong public healthcare systems fit a culture of the government carrying responsibility for the health of the nation (Savedoff et al., 2012). They have the resources to cater for public health and apparently leaned into that capacity to reassure the public. These countries were relatively quick to communicate government action, which was less the case for countries with lower degrees of universal health coverage. Their initial response in terms of government measures took longer, but gradually intensified over time to eventually exceed these of countries with a stronger capability. A possible explanation, consistent with literature, is that taking action to communicate openly and intervene in the interest of public health was not an usual practice for the governments. This further ties in with the finding that countries with lower universal health coverage's public health communication was relatively more focused over time on highlighting individual preventive measures. That is urging citizens to stay at home, practice hygiene, and wear masks.
Equally important is the finding that countries with a lower degree of healthcare coverage emphasized the importance of support and solidarity. These messages are phrased abstractly and rarely mention the pandemic explicitly. There are multiple ways to interpret this finding. On the one hand, it could be seen as a deflection, which is hardly productive in mitigating the spread. It supports the prediction that countries that without strong publicly accessible health infrastructure compensate that lack by shifting responsibility to individuals and the community in lieu of governments organizing adequate responses. On the other hand, it could be argued that in the response to a highly infectious decease, any mitigation strategy needs to appeal to everyone's responsibility and stimulate the willingness to exemplify protective behavior not only to safeguard oneself, but the wider community. After all, it has been repeatedly demonstrated that for example the community adoption of facemasks was an effective strategy to limit the spread (Cheng et al., 2020; Zeng et al., 2020). Although it is at this point too early to draw definite conclusions, a possible lesson from these findings could be that it could be a risk to favor to highlighting structural capacity. Although this can prevent panic and offer reassurance, it might also instill too much confidence in the healthcare infrastructure. If this takes primacy over prevention at the individual level, there is a risk that citizens feel overly comfortable and take more risks. This possibly increases the spread, eventually stressing the health care capacity anyway – as was the case (Cash and Patel, 2020; Dongarwar and Salihu, 2020; Iyengar et al., 2020).
All considered, this study provides a high-level vantage point that has proven sufficiently sensitive to tease out the diversity in the kind of language uttered by governments on Twitter during the onset of the pandemic. Moreover, it clearly points to (inter)national differences, and health care systems in particular. The macro perspective is relatively uncommon in health communication, and yet, a global pandemic requires particularly that kind of insights. The outcomes of this study invite future research to further probe and explain these differences and relate them to (continuing) epidemiological data, and to draw lessons from them to improve future health crisis communication. The key will be to consider global differences in the impact of COVID-19 and the effectiveness of measures and, importantly, the communication of those measures. The findings of this study should be instrumental in compiling that holistic picture.
Yet, several limitations should be taken into account. Importantly, there is the underlying assumption that communication on Twitter is to a considerable extent indicative for the overall communication strategy by the included actors in the various countries. Our study does indicate that Twitter is more than a collage of information bits; it represents a broader flow of communication and discourse, where various themes and nuances will influence post-COVID-19 communication in the future. Here, we recommend consecutive studies including other health discourses to move beyond a traditional understanding of health communication as information transfer toward a more useful definition of dialogue, information exchange and discourse (Lee and Garvin, 2003).
Another limitation is the translation procedure. Although the performance of Google Translate is considerable and continually improves, it is likely that is unable to capture all linguistic nuance. However, as topic modeling is a technique that seeks out larger, aggregate patterns, it is fair to say that these overall patterns are most likely reflective of the actual width of themes in COVID-19 communication. Therefore, we recommend these findings as guidance to dig deeper into international differences in communication patterns surrounding COVID-19.
The raw data supporting the conclusions of this article will be made available by the authors, without undue reservation.
FW was responsible for setting up the theoretical framework. CC was responsible for the methodological set up of the study. The whole project was a collaborative research endeavor. All authors contributed to the article and approved the submitted version.
The authors declare that the research was conducted in the absence of any commercial or financial relationships that could be construed as a potential conflict of interest.
All claims expressed in this article are solely those of the authors and do not necessarily represent those of their affiliated organizations, or those of the publisher, the editors and the reviewers. Any product that may be evaluated in this article, or claim that may be made by its manufacturer, is not guaranteed or endorsed by the publisher.
Armocida, B., Formenti, B., Palestra, F., Ussai, S., and Missoni, E. (2020). COVID-19: Universal health coverage now more than ever. J. Global Health 10, 010350–010350. doi: 10.7189/jogh.10.010350
Atun, R., Aydin, S., Chakraborty, S., Sümer, S., Aran, M., Gürol, I., et al. (2013). Universal health coverage in Turkey: enhancement of equity. Lancet. 382, 65–99. doi: 10.1016/S0140-6736(13)61051-X
Atun, R., de Andrade, L. O. M., Almeida, G., Cotlear, D., Dmytraczenko, T., Frenz, P., et al. (2015). Health-system reform and universal health coverage in Latin America. Lancet. 385, 1230–1247. doi: 10.1016/S0140-6736(14)61646-9
Bai, Y., Yao, L., Wei, T., Tian, F., Jin, D.-Y., Chen, L., et al. (2020). Presumed asymptomatic carrier transmission of COVID-19. JAMA 323, 1406–1407. doi: 10.1001/jama.2020.2565
Berkman, N. D., Sheridan, S. L., Donahue, K. E., Halpern, D. J., Viera, A., Crotty, K., et al. (2011). Health literacy interventions and outcomes: an updated systematic review. Evid. Rep. Technol. Assess. 199, 1–941. doi: 10.7326/0003-4819-155-2-201107190-00005
Blei, D. M., Ng, A. Y., and Jordan, M. I. (2003). Latent dirichlet allocation. J. Mach. Learn. Res. 3, 993–1022. doi: 10.1162/2fjmlr.2003.3.4-5.993
Blendon, R. J., and Benson, J. M. (2017). Public opinion about the future of the affordable care act. New Engl. J. Med. 377, e12. doi: 10.1056/NEJMsr1710032
Blumenthal, D., Fowler, E. J., Abrams, M., and Collins, S. R. (2020). Covid-19 — Implications for the Health Care System. New Engl. J. Med. 383, 1483–1488. doi: 10.1056/NEJMsb2021088
Calderón-Larrañaga, A., Dekhtyar, S., Vetrano, D. L., Bellander, T., and Fratiglioni, L. (2020). COVID-19: risk accumulation among biologically and socially vulnerable older populations. Age. Res. Rev. 63, 101149. doi: 10.1016/j.arr.2020.101149
Cash, R., and Patel, V. (2020). Has COVID-19 subverted global health? Lancet 395, 1687–1688. doi: 10.1016/S0140-6736(20)31089-8
Chemouni, B. (2018). The political path to universal health coverage: Power, ideas and community-based health insurance in Rwanda. World Develop. 106, 87–98. doi: 10.1016/j.worlddev.2018.01.023
Cheng, V. C.-C., Wong, S.-C., Chuang, V. W.-M., So, S. Y.-C., Chen, J. H.-K., Sridhar, S., et al. (2020). The role of community-wide wearing of face mask for control of coronavirus disease 2019 (COVID-19) epidemic due to SARS-CoV-2. J. Infection. 81, 107–114. doi: 10.1016/j.jinf.2020.04.024
Cowper, A. (2020). Covid-19: are we getting the communications right? BMJ 368, m919. doi: 10.1136/bmj.m919
Dieleman, J. L., Sadat, N., Chang, A. Y., Fullman, N., Abbafati, C., Acharya, P., et al. (2018). Trends in future health financing and coverage: future health spending and universal health coverage in 188 countries, 2016–40. Lancet. 391, 1783–1798. doi: 10.1016/S0140-6736(18)30697-4
Dongarwar, D., and Salihu, H. M. (2020). COVID-19 Pandemic: marked global disparities in fatalities according to geographic location and universal health care. Int. J. MCH AIDS 9, 213–216. doi: 10.21106/ijma.389
EIU (2020). Democracy Index 2019. Available online at: https://www.eiu.com/topic/democracy-index (accessed May 5, 2022).
Fortuna, L. R., Tolou-Shams, M., Robles-Ramamurthy, B., and Porche, M. V. (2020). Inequity and the disproportionate impact of COVID-19 on communities of color in the United States: The need for a trauma-informed social justice response. Psychol. Trauma 12, 443. doi: 10.1037/tra0000889
Galvani, A. P., Parpia, A. S., Pandey, A., Zimmer, C., Kahn, J. G., and Fitzpatrick, M. C. (2020). The imperative for universal healthcare to curtail the COVID-19 outbreak in the USA. EClinicalMedicine 23, 100380. doi: 10.1016/j.eclinm.2020.100380
Gollust, S. E., and Lynch, J. (2011). Who deserves health care? The effects of causal attributions and group cues on public attitudes about responsibility for health care costs. J. Health Politics, Policy Law. 36, 1061–1095. doi: 10.1215/03616878-1460578
Gore, R., and Parker, R. (2019). Analysing power and politics in health policies and systems. Global Public Health 14, 481–488. doi: 10.1080/17441692.2019.1575446
Guest, J. L., del Rio, C., and Sanchez, T. (2020). The Three Steps Needed to End the COVID-19 Pandemic: Bold Public Health Leadership, Rapid Innovations, and Courageous Political Will. JMIR Public Health Surveill. 6, e19043. doi: 10.2196/19043
Guidry, J. P. D., Jin, Y., Orr, C. A., Messner, M., and Meganck, S. (2017). Ebola on Instagram and Twitter: How health organizations address the health crisis in their social media engagement. Public Relat. Rev. 43, 477–486. doi: 10.1016/j.pubrev.2017.04.009
Guidry, J. P. D., Meganck, S. L., Perrin, P. B., Messner, M., Lovari, A., and Carlyle, K. E. (2020). #Ebola: Tweeting and Pinning an Epidemic. Atlantic J. Commun. 29, 79–92. doi: 10.1080/15456870.2019.1707202
Hornik, R., Kikut, A., Jesch, E., Woko, C., Siegel, L., and Kim, K. (2021). Association of COVID-19 misinformation with face mask wearing and social distancing in a nationally representative US sample. Health Commun. 36, 6–14. doi: 10.1080/10410236.2020.1847437
IBRD-IDA (2020). GDP (current US$). Available online at: https://data.worldbank.org/indicator/NY.GDP.MKTP.CD (accessed May 5, 2022).
Iyengar, K., Mabrouk, A., Jain, V. K., Venkatesan, A., and Vaishya, R. (2020). Learning opportunities from COVID-19 and future effects on health care system. Diab. Metab. Syndr. 14, 943–946. doi: 10.1016/j.dsx.2020.06.036
Jelodar, H., Wang, Y., Yuan, C., Feng, X., Jiang, X., Li, Y., et al. (2019). Latent Dirichlet allocation (LDA) and topic modeling: models, applications, a survey. Multim. Tools Applic. 78, 15169–15211. doi: 10.1007/s11042-018-6894-4
Kapadia, S. (2019). Evaluate Topic Models: Latent Dirichlet Allocation (LDA). A step-by-step guide to building interpretable topic models. Available online at: https://towardsdatascience.com/evaluate-topic-model-in-python-latent-dirichlet-allocation-lda-7d57484bb5d0 (accessed May 5, 2022).
Karwowski, M., Kowal, M., Groyecka, A., Białek, M., Lebuda, I., Sorokowska, A., et al. (2020). When in danger, turn right: Covid-19 threat promotes social conservatism and right-wing presidential candidates? doi: 10.31234/osf.io/pjfhs
Khurana, S., Singh, P., Sinha, T. P., Bhoi, S., and Mathur, P. (2020). Low-cost production of handrubs and face shields in developing countries fighting the COVID19 pandemic. Am. J. Infec. Control 48, 726–727. doi: 10.1016/j.ajic.2020.03.016
Kruk, M. E., Gage, A. D., Joseph, N. T., Danaei, G., García-Sais,ó, S., and Salomon, J. A. (2018). Mortality due to low-quality health systems in the universal health coverage era: a systematic analysis of amenable deaths in 137 countries. Lancet. 392, 2203–2212. doi: 10.1016/S0140-6736(18)31668-4
Lavers, T. (2019). Towards Universal Health Coverage in Ethiopia's ‘developmental state'? The political drivers of health insurance. Soc. Sci. Med. 228, 60–67. doi: 10.1016/j.socscimed.2019.03.007
Lee, R. G., and Garvin, T. (2003). Moving from information transfer to information exchange in health and health care. Soc. Sci. Med. 56, 449–464. doi: 10.1016/S0277-9536(02)00045-X
Li, J., Vishwanath, A., and Rao, H. R. (2014). Retweeting the Fukushima nuclear radiation disaster. Commun. ACM. 57, 78–85. doi: 10.1145/2500881
McAteer, J., Yildirim, I., and Chahroudi, A. (2020). The vaccines act: deciphering vaccine hesitancy in the time of COVID-19. Clin. Infect. Dis. 71, 703–705. doi: 10.1093/cid/ciaa433
McCartney, G., Popham, F., McMaster, R., and Cumbers, A. (2019). Defining health and health inequalities. Public health 172, 22–30. doi: 10.1016/j.puhe.2019.03.023
McIntyre, D., Meheus, F., and Røttingen, J.-A. (2017). What level of domestic government health expenditure should we aspire to for universal health coverage? Health Econ. Policy Law. 12, 125–137. doi: 10.1017/S1744133116000414
Mohd Hanafiah, K., Ng, C., and Wan, A. M. (2021). Effective communication at different phases of COVID-19 prevention: roles, enablers and barriers. Viruses 13, 1058. doi: 10.3390/v13061058
Moreno-Serra, R., and Smith, P. C. (2012). Does progress towards universal health coverage improve population health? Lancet. 380, 917–923. doi: 10.1016/S0140-6736(12)61039-3
Noar, S. M., and Austin, L. (2020). (Mis)communicating about COVID-19: insights from health and crisis communication. Health Commun. 35, 1735–1739. doi: 10.1080/10410236.2020.1838093
Nutbeam, D., and Lloyd, J. E. (2021). Understanding and responding to health literacy as a social determinant of health. Annu. Rev. Public Health 42, 159–173. doi: 10.1146/annurev-publhealth-090419-102529
Park, H. W., Park, S., and Chong, M. (2020). Conversations and medical news frames on Twitter: infodemiological study on COVID-19 in South Korea. J. Med. Internet Res. 22, e18897. doi: 10.2196/18897
Poirier, W., Ouellet, C., Rancourt, M.-A., Béchard, J., and Dufresne, Y. (2020). (Un)Covering the COVID-19 Pandemic: framing analysis of the crisis in Canada. Canad. J. Polit. Sci. 52, 365–371. doi: 10.1017/S0008423920000372
Reeves, A., McKee, M., and Stuckler, D. (2015). The attack on universal health coverage in Europe: recession, austerity and unmet needs. Eur. J. Public Health 25, 364–365. doi: 10.1093/eurpub/ckv040
Royston, G., Pakenham-Walsh, N., and Zielinski, C. (2020). Universal access to essential health information: accelerating progress towards universal health coverage and other SDG health targets. BMJ Global Health. 5, e002475. doi: 10.1136/bmjgh-2020-002475
Savedoff, W. D., de Ferranti, D., Smith, A. L., and Fan, V. (2012). Political and economic aspects of the transition to universal health coverage. Lancet. 380, 924–932. doi: 10.1016/S0140-6736(12)61083-6
Schiavo, R. (2013). Health Communication: From Theory to Practice. Second Edition. Hoboken, NJ: John Wiley & Sons.
Shadmi, E., Chen, Y., Dourado, I., Faran-Perach, I., Furler, J., Hangoma, P., et al. (2020). Health equity and COVID-19: global perspectives. Int. J. Equity Health 19, 104. doi: 10.1186/s12939-020-01218-z
Shin, J., Jian, L., Driscoll, K., and Bar, F. (2018). The diffusion of misinformation on social media: Temporal pattern, message, and source. Comput. Human Behav. 83, 278–287. doi: 10.1016/j.chb.2018.02.008
Sørensen, K., Pelikan, J. M., Röthlin, F., Ganahl, K., Slonska, Z., Doyle, G., et al. (2015). Health literacy in Europe: comparative results of the European health literacy survey (HLS-EU). Eur. J. Public Health. 25, 1053–1058. doi: 10.1093/eurpub/ckv043
Tediosi, F., Lönnroth, K., Pablos-Méndez, A., and Raviglione, M. (2020). Build back stronger universal health coverage systems after the COVID-19 pandemic: the need for better governance and linkage with universal social protection. BMJ Global Health 5, e004020. doi: 10.1136/bmjgh-2020-004020
Tirkkonen, P., and Luoma-aho, V. (2011). Online authority communication during an epidemic: A Finnish example. Public Relat. Rev. 37, 172–174. doi: 10.1016/j.pubrev.2011.01.004
Tucker, J. A., Guess, A., Barberá, P., Vaccari, C., Siegel, A., Sanovich, S., et al. (2018). Social media, political polarization, and political disinformation: A review of the scientific literature. (accessed March 19, 2018). doi: 10.2139/ssrn.3144139
Vian, T., Feeley, F. G., Domente, S., Negruta, A., Matei, A., and Habicht, J. (2015). Barriers to universal health coverage in Republic of Moldova: a policy analysis of formal and informal out-of-pocket payments. BMC Health Serv. Res. 15, 319. doi: 10.1186/s12913-015-0984-z
Viswanath, K., Lee, E. W. J., and Pinnamaneni, R. (2020). We need the lens of equity in COVID-19 communication. Health Commun. 35, 1743–1746. doi: 10.1080/10410236.2020.1837445
WHO (2019). Universal health coverage (UHC). Available online at: https://www.who.int/news-room/fact-sheets/detail/universal-health-coverage-(uhc) (accessed May 5, 2022).
WHO (2020). UHC service coverage index. Available online at: https://www.who.int/data/gho/indicator-metadata-registry/imr-details/4834 (accessed May 5, 2022).
Wu, Z., and McGoogan, J. M. (2020). Characteristics of and important lessons from the coronavirus disease 2019 (COVID-19) outbreak in China: Summary of a report of 72 314 cases from the chinese center for disease control and prevention. JAMA 323, 1239–1242. doi: 10.1001/jama.2020.2648
Yamin, A. E., and Frisancho, A. (2015). Human-rights-based approaches to health in Latin America. Lancet. 385, e26–e29. doi: 10.1016/S0140-6736(14)61280-0
Keywords: health coverage, health policy, Twitter, Twitter - content analysis, global changes, COVID-19
Citation: Weder F and Courtois C (2022) Differences in universal health coverage and governments' COVID-19 communication: A global comparative analysis. Front. Commun. 7:1080948. doi: 10.3389/fcomm.2022.1080948
Received: 26 October 2022; Accepted: 02 December 2022;
Published: 16 December 2022.
Edited by:
Yan Su, Peking University, ChinaReviewed by:
Douglas Ashwell, Massey University Business School, New ZealandCopyright © 2022 Weder and Courtois. This is an open-access article distributed under the terms of the Creative Commons Attribution License (CC BY). The use, distribution or reproduction in other forums is permitted, provided the original author(s) and the copyright owner(s) are credited and that the original publication in this journal is cited, in accordance with accepted academic practice. No use, distribution or reproduction is permitted which does not comply with these terms.
*Correspondence: Franzisca Weder, RnJhbnppc2NhLldlZGVyQGdtYWlsLmNvbQ==
†Deceased
Disclaimer: All claims expressed in this article are solely those of the authors and do not necessarily represent those of their affiliated organizations, or those of the publisher, the editors and the reviewers. Any product that may be evaluated in this article or claim that may be made by its manufacturer is not guaranteed or endorsed by the publisher.
Research integrity at Frontiers
Learn more about the work of our research integrity team to safeguard the quality of each article we publish.