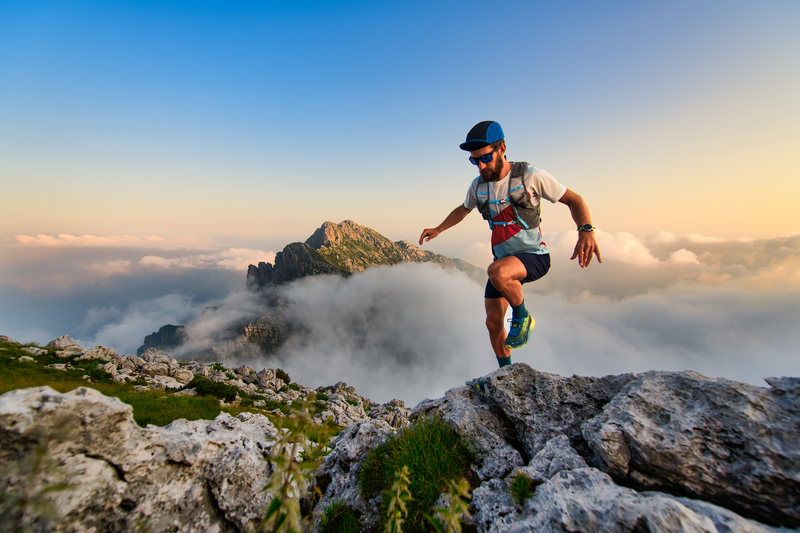
94% of researchers rate our articles as excellent or good
Learn more about the work of our research integrity team to safeguard the quality of each article we publish.
Find out more
ORIGINAL RESEARCH article
Front. Commun. , 20 January 2023
Sec. Advertising and Marketing Communication
Volume 7 - 2022 | https://doi.org/10.3389/fcomm.2022.1022139
Introduction: Pre-launch advertising communications are critical for the early adoption of experiential products. Often, companies release a variety of advertising messages for the same product, which results in a lack of information consistency. Research on the effect of advertising communications with different message content is scarce. Further, most studies on information consistency rely on experimental methods, leaving the actual effect of consumer response on product adoption unknown.
Methods: Treating online comments to movie trailers as consumer response to advertising communication, we propose a natural language processing methodology to measure information consistency. We validate our measurement through an online experiment and test it on 1.3 million YouTube comments.
Results: Our empirical results provide evidence that information consistency driven by trailer-viewing is a key driver of opening box office success.
Discussion: Insights deriving from this study are important to marketing communications research, especially in contexts where early product adoption is critical.
Early adoption is critical for products with short life cycles. This is particularly true in the case of experiential products, where an experience might be available only for a few days or weeks (e.g., music festivals, plays movies). Judging the quality of experiential goods before they are released in the market is a challenging task. To attract consumer interest about an upcoming experience, marketers invest heavily in pre-launch advertising campaigns (Song et al., 2016). At times, these marketing communication campaigns entail a number of different promotional messages, aimed at attracting different segments of the target market. To illustrate this with an example, the trailer campaign of the latest Planet of the Apes movie featured nine different official trailers, which followed different characters. While this strategy might help minimize quality uncertainty, being exposed to different information might cause confusion and harm product adoption.
Consumer response directly driven from advertising is visible online in the form of word-of-mouth (WOM) communication. Consumers' online communications on the various social media platforms provide marketers with the opportunity to gain access into consumer response. For instance, the release of a new trailer can receive thousands of views in 1 day and can further spark consumer engagement in the form of likes, dislikes, comments etc.,. Monitoring and analyzing these forms of communications helps marketers anticipate future product sales. Social media response to advertising communications has lately engaged researchers (Mafael et al., 2021). Yet, studies have not focused entirely on the effect of pre-launch advertising, when little other information about a product is available. WOM research has identified popular metrics which drive product adoption (e.g., volume, valence) (Dellarocas et al., 2007), yet current methodological advances, such as text mining and linguistic analysis, allow for richer insights (Verma and Yadav, 2021).
Further, although word-of-mouth communications research has examined pre-release WOM to predict sales (Divakaran et al., 2017; Houston et al., 2018), it does not focus on consumer response driven specifically by advertising. Additionally, advertising research has explored the effect of repetitive advertising on consumer attitudes, brand recall and purchase decision, but most studies examine the repetition of the same advertising message (Pechmann and Stewart, 1998). Yet, there is evidence that persuasion is achieved through message uniqueness, but also consistency with prior information (Mafael et al., 2021). As a result, the effect of a firm's strategy to present consumers with different information about an upcoming product requires further research.
We explore consumer response to trailer advertising on YouTube and empirically test the relationship between information consistency and opening weekend box office (BO) performance. Treating WOM as response to online advertising communications, we develop a methodology to measure information consistency through behavioral data. Drawing from social psychology theory on individuals' preference for consistent information (Festinger, 1962; Chapanis and Chapanis, 1964), we expect that pre-launch trailer campaigns which manage to maintain consistent opinions will be more effective at driving early movie adoption.
To set the boundaries of this research, we are not interested in measuring content consistency among different ads of the same product (e.g., Becker and Gijsenberg, 2022). Our focus is on the extent to which consistency is reflected in consumers' online communications as a response to different ads of the same product. We propose a Natural Language Processing (NLP) methodology by analyzing language variability in a dataset of 1.3 million YouTube comments on movie trailers. We rely on term frequency-inverse document frequency (tf-idf), to capture the extent to which conversations on trailers of the same movie remain consistent throughout the pre-launch campaign. We validate our proposed methodology through an online experiment and examine the effect of information consistency on opening weekend performance.
Controlling for the volume of comments and for product-related parameters (e.g., sequel, budget), we find support that pre-release information consistency–regardless of ad content–leads to higher product adoption. While recent advertising research has examined the role of content consistency on consumer attitudes (Mafael et al., 2021) and sales (Becker and Gijsenberg, 2022), insight on consumers' information consistency is limited to knowledge building and communication adoption (Vandenbosch and Higgins, 1996; Zhang and Watts, 2003). We contribute to this line of research by linking pre-release information consistency to early product adoption. Our research has methodological implications for those interested in measuring information consistency through behavioral data. As research on information consistency relies on experimental methods and self-report scales, marketers do not have a way to measure or infer consumers' information consistency, unless they collect primary data. In line with the latest marketing research utilizing text data from consumers' online communications (Humphreys and Wang, 2018; Berger, et al., 2022), we propose a novel methodology for the measurement of consistency.
Finally, our findings offer contextual implications for movie marketing theory and practice. Extending prior BO prediction models which rely on online buzz metrics (e.g., search, volume, likes) (Houston et al., 2018; Kim, 2020), we establish an important parameter that predicts early BO performance. Our research provides directions to marketing managers on the importance of maintaining consistent perceptions throughout the pre-launch phase of the movie. Our proposed methodology may be adopted as a social listening tool to analyze early audience response to online advertising communications and reshape strategic decisions accordingly.
While persuasive communications research has examined the role of repeated advertising in shaping consumer attitudes (Haugtvedt et al., 1994; Kronrod and Huber, 2019), the majority of studies are conducted with the repetition of identical ads (Cacioppo and Petty, 1985). Few studies have examined the role advertising consistency on consumer behavior. Advertising messages which are consistent to prior messages by the same brand are more easily assimilated (Kilgour and Koslow, 2009), generate positive WOM (Mafael et al., 2021), and drive sales especially for smaller brands (Becker and Gijsenberg, 2022). These findings, however, focus on ad message content and do not offer insight on consumers' perceived information consistency as a response to advertising.
Our conceptualization of information consistency is borrowed from information systems research and refers to “the extent to which new information is consistent with prior knowledge” (Zhang and Watts, 2003, p.102). The concept of consistency is based on Festinger's (1962) theory of cognitive dissonance, which conceptualizes the psychological discomfort we experience when we are exposed to new information that contradicts our prior knowledge. In order to avoid cognitive dissonance, individuals strive for consistency (Chapanis and Chapanis, 1964). Consistency leads to more favorable attitudes (Tormala and Clarkson, 2007), while information that is inconsistent with prior knowledge is met with resistance (Zhang and Watts, 2003) and is considered less credible (Cheung et al., 2009).
According to theories of learning through assimilation, information consistency aids in mental model maintenance (Piaget, 1954; Vandenbosch and Higgins, 1996). Information systems research has examined the role of information consistency on learning (Vandenbosch and Higgins, 1996) and knowledge adoption (Zhang and Watts, 2003) as a response to communications. Yet, current marketing communications research (Mafael et al., 2021; Becker and Gijsenberg, 2022) has not explored the role of perceived information consistency as a response to different advertising messages, in driving product adoption. Our research addresses this gap by examining consumer response to trailer advertising on YouTube, in an attempt to measure whether conversations on different trailers of the same movie are consistent and to test the effect of consistency on actual movie sales.
WOM is considered the most effective form of marketing communications. Representing a form of earned communications, it is more credible and trustworthy (Gu et al., 2012). Research on the effect of WOM on product adoption or product sales is exhaustive (Verma and Yadav, 2021). Researchers have utilized WOM reviews on a variety of product categories to gain insight on how different metrics can predict product sales. WOM volume and valence are by far the most popular metrics in traditional WOM research (Godes and Mayzlin, 2004; Hartmann, et al., 2022). Aside from that, researchers have explored other metrics such as review variance (Yin et al., 2022), credibility (Wang et al., 2022) helpfulness (Chou et al., 2022), narrativity (Van Laer et al., 2019) and relevance (Biswas, et al., 2022).
While most WOM research focuses on reviews shared after the release and use of a product, some products heavily rely on pre-release WOM. As such, researchers have recognized the importance of pre-release WOM and have shifted their interest toward communications shared prior to the release of a product. Consumers' speculations about an upcoming product are evident online in the form of pre-release buzz (Houston et al., 2018). Pre-release buzz, which reflects consumers' anticipation (Hennig-Thurau et al., 2012) is critical for the success of products with short life cycles, especially experiential ones, such as movies or video games (Marchand et al., 2017; Bogaert, et al., 2021).
WOM communications research has contributed a lot of insight into how marketers can leverage and analyze pre and post-release WOM to draw insight on consumer response and anticipate product sales. Yet, the majority of studies look at WOM as an emerging phenomenon and do not take into account the potential effect that advertising might have on WOM communications. Indeed, advertising and WOM are considered by some as opposing marketing techniques and researchers have examined the two forms of marketing communications in an attempt to identify the superiority of the one over the other in driving sales (e.g., Feng and Papatla, 2011). However, advertising and WOM can also be treated as complementary marketing activities. Recognizing that advertising communications can spark online WOM, some researchers study advertising as an antecedent of WOM (Thorbjørnsen et al., 2015; Joshi and Musalem, 2020), or examine their joint effect on product adoption (Bruce et al., 2012). Notably, these studies explore post-release WOM communications, but advertising has also been found to shape early opinions (Fay et al., 2019).
In practice, prior to a product's release, consumers may draw information from advertising and may express their anticipation through pre-release buzz. We thus examine WOM communications driven by pre-release advertising in an attempt to extract information consistency and test its effect on product adoption.
The movie industry is selected as an appropriate research context, since product success heavily relies on pre-launch advertising and on pre-release WOM. Extant movie research indicates that advertising influences consumer expectations and consumer behavior (Moul, 2007) and drives both short and long-term performance (Lee et al., 2021). Out of all the sources that consumers consult before making a movie-going decision, the trailer is the most popular one (Boksem and Smidts, 2015). Trailers are considered to be the most effective form of movie advertising (Kernan, 2004), as they minimize quality uncertainty in the pre-release phase of a movie (Schroll and Grohs, 2019). Studies focusing specifically on trailer campaigns identify a positive influence on movie preference (Boksem and Smidts, 2015), purchase intentions (Archer-Brown, et al., 2017) and movie revenue (Hennig-Thurau et al., 2007; Gong et al., 2011).
Studios typically release multiple trailers for the same movie. These aim to spark audience anticipation, which is often expressed in the form of pre-release WOM. While studies on pre-release WOM have explored the effect of volume and valence on box office performance (Bogaert, et al., 2021), the majority of movie research looks at WOM communications as an emerging, rather than ad-driven, phenomenon (e.g., Castillo et al., 2021). As such, trailer response has rarely been examined through WOM data. The few studies that do analyze trailer response are either concerned with unveiling the intention to see a movie (Craig et al., 2015) or with detecting spikes of activity in pre-release WOM (Gelper et al., 2018). As such, there have been recent calls for research to understand how YouTube data can be used in BO prediction (Ahmad et al., 2020; Bogaert, et al., 2021).
The literature review revealed four important research gaps. First, there is an evident lack of research on pre-launch advertising, especially for products with short life-cycles whose success heavily relies on early adoption. Second, while there have been a few studies that explore the effect of ad content consistency on consumer behavior, to our knowledge, there is no research examining the effect of perceived information consistency as a result of advertising. Third, persuasive communications research on the effect of repeated advertising has not focused on the effect of ads with different message content. Fourth, we identified a context specific gap in the area of movie WOM research, which focuses on pre-release WOM volume to predict early BO performance, overlooking other potentially important WOM metrics. To address these gaps we aim to uncover the effect of perceived information consistency driven by pre-launch advertising on early product adoption.
We gain insight into consumers' information consistency by treating online WOM as advertising response. In line with social psychology theory on cognitive dissonance (Festinger, 1962) and with information systems research on the positive role of information consistency (Vandenbosch and Higgins, 1996; Zhang and Watts, 2003), we expect that, regardless of trailer content, pre-launch advertising campaigns which maintain consistent opinions will be more effective. Driven by findings on the positive effect of trailer advertising on movie performance (Hennig-Thurau et al., 2007; Gong et al., 2011), we anticipate that ad-driven information consistency observed in consumers' pre-release WOM communications will positively influence opening weekend BO performance.
We propose a NLP methodology for the measurement of information consistency. NLP has recently gained attention in communication and marketing research as an appropriate methodology to analyze the abundance of online text data. It offers the opportunity to explore public opinion (Zhang et al., 2021) or to observe the evolution of topics in consumers' conversations overtime (Yang et al., 2021). We focus on the variability of language in comments regarding different trailers of the same movie. We assume that campaigns that feature high (low) variability in their conversations reflect low (high) consistency. Term frequency–inverse document frequency (tf-idf) is a measure which identifies how relevant a term is to a document across a number of different documents. Its main use is to investigate the similarity among different text documents (Adar and Adamic, 2005) and to serve as a statistical comparison measure (Humphreys and Wang, 2018).
Tf-idf is measured by multiplying the term frequency with the inverse document frequency of a term across a set of documents (Sparck Jones, 1972). For a term i in document j, tf-idf is calculated as follows:
Where tfi, j = number of occurrences of term i in document j
N = total number of documents
dfi = number of documents containing i
This way, a term's popularity is offset by the number of documents that include that term. As such, terms that are common in every document rank low, while terms that are common only in one document rank high.
We compile a separate corpus for each different movie. Each corpus N consists of a number of documents j which reflect the comments associated with each YouTube trailer in a movie's campaign. Movies that feature only one trailer in their pre-release campaign will only have one document. Each document j consists of a number of different terms (words) i, which may also appear in the rest of the documents across the corpus. To illustrate this with an example, the movie Power Rangers featured three trailers (documents) in its pre-release campaign. Each document had a list of terms that were used to describe the corresponding trailer, and naturally some terms overlapped. The term “Becky” was mentioned 406 times in total across all documents (374 times on trailer 1, 3 times on trailer 2, and 29 on trailer 3). This term had a low tf-idf. Conversely, the term “Halsey” which was also a popular term in the corpus of Power Rangers, since it occurred 389 times, was only present in the conversation about trailer 1. This term had a high tf-idf.
Since tf-idf reflects the variability of terms, we assume that high average tf-idf signifies a relatively low consistency and vice versa. To reflect this inverse relationship between the tf-idf metric and consistency, we calculate information consistency for each corpus (movie) as follows:
Where wi, j = tf-idf for each term i across all documents j
N = total number of terms in corpus.
We next perform our proposed methodology on our YouTube dataset and validate it through an online experiment.
Our dataset consists of 1.3 million YouTube comments collected during a two-year period (November 2015–December 2017). We focus on this time-period to eliminate any potential bias caused by the closure of theaters due to the pandemic. To ensure the best possible consistency between our dataset and the current industry setting, we selected a time-period where trailers were the main form of movie advertising (Karray and Debernitz, 2017) and YouTube–our platform of choice–was the most popular trailer-viewing platform (Oh et al., 2017). Both these points still apply today (Duo, 2022; Epting, 2022). We collected comments for all wide-release movies (n = 143) whose pre-release campaign started and ended within the data collection period. We initiated a data collection query for every new teaser or trailer (n = 413), from the day that it was released on YouTube until the movie's release date. We mined YouTube comments through YouTube Data Tools (Rieder, 2015), for all official videos that received over ten thousand views by the release dated of the movie.
We cleaned the data by removing stop-words, HTML signs and non-English words. We then performed bigram detection and kept the bigrams that occurred at least twice in our dataset. A bigram is defined as a sequence of two words. The lead researcher manually scanned the remaining bigrams and accepted those that are typically used as one word or phrase in the context of movies (e.g., term a: “Brad,” term b: “Pitt,” bigram: “Brad_Pitt”). This was more often actors' names or movie titles. The final dataset consisted of 52,541 unique terms, including the bigrams.
Information consistency was measured through NLP analysis. We also noted the number of documents involved in the calculation of each movie's tf-idf, which equals the number of trailers for each campaign. After removing three outliers, the number of trailers followed a normal distribution. Naturally, movies with just one trailer returned a tf-idf value of 0. Excluding those movies from our dataset would create a misrepresentative sample. For this reason, we set consistency for those movies at the sample mean. Information consistency was independent from the number of trailers (r = 0.011, p = 0.896).
Our dependent variable was measured by the opening weekend BO performance in $ million. We controlled for ad awareness reflected in WOM volume which is a strong predictor of early movie adoption (Craig et al., 2015; Houston et al., 2018). We also collected data on a number of movie-related parameters which have been found to influence opening BO performance (e.g., Karniouchina, 2011; Gelper et al., 2018; Houston et al., 2018). Table 1 presents the operationalization of variables.
Before testing our model, we ran a preliminary analysis to validate our proposed measurement methodology through an online experiment.
To validate our proposed measurement of consistency we selected two movies from our dataset which featured high and low consistency values. We took steps to eliminate bias and potential confounding factors by selecting two movies that featured the same pre-release signals and the same number of trailers. Kong Skull Island (Kong) had a high information consistency, while Jack Reacher: Never Go Back (Reacher) had a low consistency.
We recruited 420 workers from Amazon Mechanical Turk who were exposed to one of the two different pre-release trailer campaigns consisting of three different trailers. We followed a between-subjects design to avoid contamination of data due to boredom or reduced attention, consistent with similar prior research (Rethans et al., 1986; Haugtvedt et al., 1994). Participants were randomly assigned to one of the two conditions. Participants were aged between 25 and 39 years old, consistent with the most frequent movie-going age group (MPAA, 2020). To ensure that participants could confidently process information on the trailers, we filtered out those that were not fluent in the English language. To ensure that participants were not familiar with the actual movie and to simulate a pre-release setting as best as we could, we filtered out those who had seen the movie they were assigned to. To simulate the advertising campaign, trailers were shown in their actual order of release. Participants were then asked to answer a set of questions after watching each trailer.
To assess the validity of our proposed measure, we collected respondents' comments on each trailer through a thought-listing task. Thought-listing is a standard process in studies on cognitive response to advertising and persuasive communications (Rethans et al., 1986). Since our aim was to simulate the process of commenting on YouTube, we asked respondents to list whatever thoughts, ideas, or reactions they experienced while watching the trailer. This process was repeated for all three trailers in each condition. We then treated the collection of thoughts for each trailer as one document, and the collection of documents (n =3) for each movie as one corpus. We derived two separate corpora for the two separate conditions and extracted the information consistency for each corpus–movie in a similar fashion to our analysis of the YouTube comments.
To assess whether the actual attitude toward the movie changed as a result of trailer viewing, we measured Product Attitude on a 3-item 7-point semantic differential scale (very bad–very good, like–dislike, negative–positive), consistent with prior research on ad repetition (Rethans et al., 1986). The first item was reverse-coded. We repeated this after each trailer. The scale was reliable (Cronbach's a: 0.916 for Trailer 1; 0.907 for Trailer 2; 0.946 for Trailer 3).
To measure the Perceived Consistency across the three trailers, we adapted the information consistency scale from relevant prior research (Zhang and Watts, 2003) (see Appendix A). Respondents were asked to report the extent to which the second and third trailer was consistent with their initial assumptions, on an 8-item 7-point scale (to no extent–to a great extent). Items 4 to 8 were reverse coded. The scale was reliable (Cronbach's a: 0.796).
Eighteen workers failed the attention checks and their responses were excluded from the dataset. The remaining 402 cases were equally split between each condition (n = 201).
The text from the thought-listing task was cleaned and analyzed, similar to the analysis of the YouTube dataset. We derived a codebook of a total of 1,351 unique terms and bigrams and applied it to all text documents. Although comments on Kong exhibited higher Consistency (0.9917) compared to Reacher (0.9892), the difference was not as prominent as our YouTube dataset (ConsistencyKONG = 0.9934; ConsistencyREACHER = 0.6784). This might be attributed to the general excitement of trailer-watching experimental tasks or to the fact that the volume of comments in the experiment was controlled and was therefore identical in the two conditions.
Results revealed that the mean Product Attitude extracted after each trailer demonstrated higher fluctuations for Reacher (low consistency condition), compared to Kong (Figure 1).
To confirm that attitudes toward Reacher exhibited higher variation than Kong as a result of trailer exposure, we performed paired-samples t-tests between the three trailers of each movie. Tables 1, 2 present the results. Indeed, significant mean differences were observed between all pairs of Reacher's trailers. Conversely, mean differences in product attitudes after each trailer exposure for Kong were not significant. These findings suggest that perceptions formed throughout Kong's campaign remained fairly consistent compared to Reacher.
Additionally, participants exposed to the Kong campaign, exhibited significantly higher perceived information consistency (M = 5.31, SD = 0.95) than those in the low consistency condition (M = 5.08, SD = 1.11), t(400) = 2.28, p < 0.05.
Findings from the online experiment were in line with our expectations on the two conditions and provided appropriate validation of our proposed measurement.
We proceed with our analysis of behavioral data to test the effect of information consistency on early adoption. Our sample consisted of 413 trailers, advertising 143 movies. Each campaign featured between 1 and 8 trailers, with an average of 3 trailers. After removing three outliers due to extreme number of trailers in the campaign or extreme WOM volume, the number of trailers followed a normal distribution.1 The remaining 140 movies opened to $28 million on average. On average, each movie received 9.6 thousand comments. Consistency ranged between 0.584 and 0.998. The average budget was $68 million. Twenty-nine percent (29%) of the movies were sequels and 42% included a star featured on IMDb's StarMeter. Sixty-six percent (66%) had an age rating of PG or PG-13. Seven of the movies were not reviewed by professional critics prior to their release. The average rating of the rest of the sample was 56 out of 100. On average, movies opened to 3,189 screens. Descriptive statistics and exploratory correlations are reported in Table 3.
We performed OLS regressions in a three-step process to observe the incremental effect of consistency. We first entered the movie-related control variables in a benchmark model and then added WOM volume, followed by information consistency. The histogram of standardized residuals and the P-P plot indicated that the data contained normally distributed errors. The scatterplot of standardized residuals showed that the data met the assumptions of linearity and homoscedasticity (see Appendix B). We also tested Models 2 and 3 including valence, but the parameter was not significant and did not improve the model's fit (see Appendix C). Results are reported in Table 4.
The final model (Model 3) was significant [F(8, 124) = 31.459, p < 0.01]. Multicollinearity was below critical thresholds; all variance inflation factors were below 3, with the exception of the number of screens (3.08). Model fit was good and explained an extra 5% of variance compared to the benchmark model (Model 1), bringing the R2 (Adj. R2) from 0.621 (0.603) to 0.670 (0.649). The effect of information consistency was significant (b = 0.251, p < 0.01), and along with the number of screens (b = 0.414, p < 0.01), information consistency was the strongest BO predictor. Adding consistency to the model, made WOM volume non-significant (b = 0.031, p = 0.710).
In conclusion, movie campaigns which achieved a high level of consistency were more likely to do well at the BO.
This research examined the role of information consistency driven by pre-launch online advertising communications in predicting early adoption. We proposed a novel methodology for the measurement of consistency through online WOM communications, based on NLP and, in particular, on the tf-idf metric. We observed the language similarity or variability in consumers' pre-release conversations relating to different trailers of the same movie. Our analysis of 1.3 million YouTube comments on movie trailers revealed that consistency was an important BO predictor. Our findings are consistent with prior social psychology and advertising research on the positive effects of consistency (Tormala and Clarkson, 2007; Kilgour and Koslow, 2009; Mafael et al., 2021). Notably, the effect of information consistency on product adoption even eliminated the effect of an established WOM metric–volume.
This research offers a number of important theoretical and research contributions. We position our work within the fields of marketing communications, social psychology and information systems. Recent advertising research uncovered the positive effect of ad content consistency (Mafael et al., 2021; Becker and Gijsenberg, 2022) and market consistency (Pauwels et al., 2022) on consumer attitudes. We extend this line of research by adopting an information systems lens and focusing on information consistency instead. Our findings confirm and extend prior research findings on the positive effect of content consistency (Mafael et al., 2021; Becker and Gijsenberg, 2022) to perceived information consistency, found in consumers' response to advertising (WOM). To our knowledge, this is the first study to measure information consistency through WOM and to link it to actuals sales. By doing so, our research adds new knowledge to traditional theories of persuasive communications on the repetition of identical advertising messages (e.g., Pechmann and Stewart, 1998; Kronrod and Huber, 2019). Our findings suggest that repetition of different advertising messages for the same product can also have a positive effect on consumer response as long as they maintain consistent consumer opinions.
Our finding that information consistency predicts opening weekend BO performance better than WOM volume also has important implications for movie research. Extending prior BO prediction models which rely on online advertising (Lee et al., 2021), social media engagement (Castillo et al., 2021; Liao and Huang, 2021) and particularly pre-release WOM (Houston et al., 2018), we point to a novel metric that can be used to predict early movie success.
Addressing research calls to use text mining tools in marketing research (Berger, et al., 2022), we propose a novel methodology for measuring information consistency through the analysis of behavioral data. By doing so, we overcome potential prior research limitations and add to two lines of research relying on self-report scales: (a) general advertising research measuring ad response (Haugtvedt et al., 1994; Till and Baack, 2005) and (b) information systems research measuring information consistency (Vandenbosch and Higgins, 1996; Zhang and Watts, 2003). Our research has important implications for marketers, and studio executives alike. Financing a movie is a high-risk investment (Squire, 2016). In fact, our data shows that the average production budget amounts to $68 million with an average opening weekend of only $28 million. To minimize risk, studios invest on heavy promotional campaigns. Yet, there is still evidence that movie marketers struggle to understand consumer response (Rainey, 2016). This is partly due to the fact that advertisers' reports to movie distributors include basic metrics and do not provide meaningful insight (Johnson and Nicola, 2017). Our research adds an alternative metric to the mix, which can predict opening weekend BO.
Additionally, our work offers useful implications for the design of pre-release campaigns. Although the number of different trailers was not related to information consistency, the content and the effect of those trailers on audience response could make or break a movie. Striving for consistency through a well-designed pre-release campaign that builds on any prior information, should be a priority for trailer editors and marketers. Finally, the proposed methodology can be adopted by movie marketers interested in extracting consumer response metrics to predict the performance of their movie and of competitive movie campaigns.
While this study contributes significant new knowledge in the areas of advertising and WOM communications, it is not free of limitations. We highlight the most important ones and hope that they will be addressed in future research. Analyzing the content of trailers was outside the scope of this research, but trailer content has been found to influence investors' decisions (Karray and Debernitz, 2017) and it would be worth investigating its effect on consumer decisions and especially on the online ad response parameters examined in this research.
We did not take into account the frequency of a user's comments or the order of trailers. Thus, we have not controlled for the potential effect of prior knowledge from exposure to earlier trailers of the same movie. Additionally, earlier trailers are present online for a longer period and their contribution toward pre-release perceptions might be stronger or weaker than later trailers. While we used the release of a new trailer to collect data on YouTube, conversations on an older trailer do not simply stop with the release of a new trailer. As such, WOM on the first trailer often overlaps with WOM on the second and third trailer. We acknowledge potential limitations of predictive validity by not testing our model using a hold-out sample, such as the second year of data in our two-year dataset. We hope that future rigorous big data analysis combined with experimental methods will address these issues.
Notably, our dataset only included wide-release, blockbuster movies (e.g., Star Wars, Justice League) aimed at a mass market. However, limited-release movies, appealing to a heterogeneous audience might actually benefit from an inconsistent ad campaign, targeting different audience segments with different trailers (e.g., different angles of the movie). Thus, it would be worth exploring under which circumstances an inconsistent campaign might be beneficial in order to provide marketing managers with recommendations on how they can ensure that each audience segment is exposed to the desired advertising message.
We acknowledge that our data was collected a few years ago and that technological advances might have changed the way that audiences interact with online video content. Notably, the pandemic has instigated significant changes in the movie industry. The closure of theaters and the rise of streamers might have encouraged audiences to shift from movie-going to streaming. For this reason, we call future researchers to explore the role of information consistency with more current data and to focus on movie success metrics other than the box office.
Finally, we acknowledge limitations of simple NLP-based analysis compared to deep learning models, especially when it comes to language used for specific product categories. Pre-release WOM on movies features a very particular language, and trailer advertising is unique in the sense that it provides a free sample of the actual product. For this reason, we call future researchers to explore the role of online ad response in other product categories, particularly those that rely on early product adoption.
The dataset supporting the conclusions of this article will be made available by the authors, without undue reservation. The raw data from the preliminary analysis is not readily available because the experimental subjects have agreed to a particular informed consent. Requests to access this dataset should be directed to JK.
Ethical review and approval was not required for the study on human participants in accordance with the local legislation and institutional requirements. The patients/participants provided their written informed consent to participate in this study.
Material preparation, data collection, and analysis were performed by JK. The first draft of the manuscript was written by JK. All authors contributed to the study conception and design, commented on previous versions of the manuscript, read, and approved the final manuscript.
The authors are grateful to the University of Cyprus for their support through an internal grant. JK has received funding from the European Regional Development Fund and the Republic of Cyprus through the Research and Innovation Foundation RESTART 2016-2020 Program, under the OPPORTUNITY/0916/MSCA/0020 grant agreement.
The authors kindly acknowledge Antonis Photiou for his kind contribution toward setting up the online experiment.
The authors declare that the research was conducted in the absence of any commercial or financial relationships that could be construed as a potential conflict of interest.
All claims expressed in this article are solely those of the authors and do not necessarily represent those of their affiliated organizations, or those of the publisher, the editors and the reviewers. Any product that may be evaluated in this article, or claim that may be made by its manufacturer, is not guaranteed or endorsed by the publisher.
The Supplementary Material for this article can be found online at: https://www.frontiersin.org/articles/10.3389/fcomm.2022.1022139/full#supplementary-material
1. ^The number of trailers was completely independent from consistency (r = 0.011, p = 0.896), confirming our decision to exclude it from our calculation of consistency and from our final model.
Adar, E., and Adamic, L. A. (2005). “Tracking information epidemics in blogspace,” in Proceedings of the 2005 IEEE/WIC/ACM International Conference on Web Intelligence (Compiegne), 325–334.
Ahmad, I. S., Bakar, A. A., and Yaakub, M. R. (2020). Movie revenue prediction based on purchase intention mining using youtube trailer reviews. Inf. Proc. Manag. 57, 102278. doi: 10.1016/j.ipm.2020.102278
Archer-Brown, C., Kampani, J., Marder, B., Bal, A. S., and Kietzmann, J. (2017). Conditions in prerelease movie trailers for stimulating positive word of mouth. J. Advert. Res. 57, 159–172. doi: 10.2501/JAR-2017-023
Becker, M., and Gijsenberg, M. J. (2022). Consistency and commonality in advertising content: helping or hurting? Int. J. Res. Mark. doi: 10.1016/j.ijresmar.2022.05.004. [Epub ahead of print].
Berger, J., Packard, G., Boghrati, R., Hsu, M., Humphreys, A., Luangrath, A., et al. (2022). Wisdom from words : marketing insights from text. Mark. Lett. 33, 365–377. doi: 10.1007/s11002-022-09635-6
Biswas, B., Sengupta, P., Kumar, A., Delen, S., and Gupta, S. (2022). A critical assessment of consumer reviews: a hybrid NLP-based methodology. Decis. Support Syst. 159:113799. doi: 10.1016/j.dss.2022.113799
Bogaert, M., Ballings, M., Van den Poel, D., and Oztekin, A. (2021). Box office sales and social media: a cross-platform comparison of predictive ability and mechanisms. Decis. Support Syst. 147:113517. doi: 10.1016/j.dss.2021.113517
Boksem, M. A. S., and Smidts, A. (2015). Brain responses to movie tailers predict individual preferences for movies and their population-wide commercial success. J. Mark. Res. 52, 482–492. doi: 10.1509/jmr.13.0572
Bruce, N. I., Foutz, N. Z., and Kolsarici, C. (2012). Dynamic effectiveness of advertising and word of mouth in sequential distribution of new products. J. Mark. Res. 49, 469–486. doi: 10.1509/jmr.07.0441
Cacioppo, J., and Petty, R. (1985). “Central and peripheral routes to persuasion: the role of message repetition,” in Psychological Processes and Advertising Effects, eds Mitchell, A. and Alwitt, L. Hillside, NJ: Lawrence Erbaum Associates, pp. 91–112.
Castillo, A., Benitez, J., Llorenz, J., and Luo, X. (2021). Social media-driven customer engagement and movie performance: theory and empirical evidence. Decis. Support Syst. 145, 113516. doi: 10.1016/j.dss.2021.113516
Chapanis, N. P., and Chapanis, A. (1964). Cognitive dissonance: five years later. Psychol. Bull. 61, 1–22. doi: 10.1037/h0043457
Cheung, M. Y., Luo, C., Sia, C. L., and Chen, H. (2009). Credibility of electronic word-of-mouth: informational and normative determinants of on-line consumer recommendations. Int. J. Elect. Commer. 13, 9–38. doi: 10.2753/JEC1086-4415130402
Chou, Y., Chuang, H. H., and Liang, T. (2022). Elaboration likelihood model, endogenous quality indicators, and online review helpfulness. Decis. Support Syst. 153, 113683. doi: 10.1016/j.dss.2021.113683
Craig, S. C., Greene, W., and Versaci, A. (2015). E-word of mouth: early predictor of audience engagement: how pre-release “E-WOM” drives box-office outcomes of movies. J. Advert. Res. 55, 62–72. doi: 10.2501/JAR-55-1-062-072
Dellarocas, C., Zhang, X., and Awad, N. (2007). Exploring the value of online product reviews in forecasting sales: the case of motion pictures. J. Interact. Mark. 21, 23–45. doi: 10.1002/dir.20087
Divakaran, P. K. P., Palmer, A., Søndergaard, H. A., and Matkovskyy, R. (2017). Pre-launch prediction of market performance for short lifecycle products using online community data. J. Interact. Mark. 38, 12–28. doi: 10.1016/j.intmar.2016.10.004
Duo, M. (2022). 10 Best Video Hosting Solutions to Consider in 2022 (Free vs Paid). Kinsta. Available online at: https://kinsta.com/blog/ (Accessed 28 November, 2022).
Epting, C. (2022). The 10 Coolest Viral Movie Marketing Campaigns. Screen Crush. Available online at: https://screencrush.com/best-viral-movie-marketing (Accessed 28 November, 2022).
Fay, B., Keller, E., and Larkin, R. (2019). How measuring consumer conversations can reveal advertising performance. J. Advert. Res. 59, 433–439. doi: 10.2501/JAR-2019-043
Feng, J., and Papatla, P. (2011). Advertising: stimulant or suppressant of online word of mouth? J. Interact. Mark. 25, 75–84. doi: 10.1016/j.intmar.2010.11.002
Gelper, S., Peres, R., and Eliashberg, J. (2018). Talk bursts: the role of spikes in prerelease word-of-mouth dynamics. J. Mark. Res. 55, 801–817. doi: 10.1177/0022243718817007
Godes, D., and Mayzlin, D. (2004). Using online conversations to study word-of-mouth communication. Mark. Sci. 23, 545–560. doi: 10.1287/mksc.1040.0071
Gong, J. J., Van der Stede, W. A., and Young, M. (2011). Real options in the motion picture industry: evidence from film marketing and sequels. Contemp. Account. Res. 28, 1438–1466. doi: 10.1111/j.1911-3846.2011.01086.x
Gu, B., Park, J., and Konana, P. (2012). The impact of external word-of-mouth sources on retailer sales of high-involvement products. Inf. Syst. Res. 23, 182–196. doi: 10.1287/isre.1100.0343
Hartmann, J., Heitmann, M., Siebert, C., and Schamp, C. (2022). More than a feeling: accuracy and application of sentiment analysis. Int. J. Res. Mark. doi: 10.1016/j.ijresmar.2022.05.005. [Epub ahead of print].
Haugtvedt, C. P., Schumann, D. W., Schneier, W. L., and Warren, W. L. (1994). Advertising repetition and variation strategies: implications for understanding attitude strength. J. Consum. Res. 21, 176. doi: 10.1086/209391
Hennig-Thurau, T., Houston, M. B., and Walsh, G. (2007). Determinants of motion picture box office and profitability: an interrelationship approach. Rev. Manag. Sci. 1, 65–92. doi: 10.1007/s11846-007-0003-9
Hennig-Thurau, T., Marchand, A., and Hiller, B. (2012). The relationship between reviewer judgments and motion picture success: re-analysis and extension. J. Cult. Econ. 36, 249–283. doi: 10.1007/s10824-012-9172-8
Hennig-Thurau, T., Wiertz, C., and Feldhaus, F. (2015). Does Twitter matter? The impact of microblogging word of mouth on consumers' adoption of new movies. J. Acad. Mark. Sci. 43, 375–394. doi: 10.1007/s11747-014-0388-3
Houston, M., Kupfer, A., Hennig-Thurau, T., and Spann, M. (2018). Pre-release consumer buzz. J. Acad. Mark. Sci. 46, 338–360. doi: 10.1007/s11747-017-0572-3
Humphreys, A., and Wang, R. J. H. (2018). Automated text analysis for consumer research. J. Consum. Res. 44, 1274–1306. doi: 10.1093/jcr/ucx104
Johnson, B., and Nicola, M. (2017). Winning Your Audiences: Marketing Movies in the Connected World. gruvi. Available online at: https://www.gruvi.tv/ebook (accessed April 15, 2022).
Joshi, Y., and Musalem, A. (2020). When consumers learn, money burns: signaling quality via advertising with observational learning and word of mouth. Mark. Sci. 40, 168–188. doi: 10.1287/mksc.2020.1246
Karniouchina, E. (2011). Impact of star and movie buzz on motion picture distribution and box office revenue. Int. J. Res. Marke. 28, 62–74. doi: 10.1016/j.ijresmar.2010.10.001
Karray, S., and Debernitz, L. (2017). The effectiveness of movie trailer advertising. Int. J. Advert. 36, 368–392. doi: 10.1080/02650487.2015.1090521
Kernan, L. (2004). Coming Attractions: Reading American Movie Trailers. Austin: University of Texas Press.
Kilgour, M., and Koslow, S. (2009). When and why do creative thinking techniques work? Trading off originality and appropriateness to make more creative advertising. J. Acad. Mark. Sci. 37, 298–309. doi: 10.1007/s11747-009-0133-5
Kim, H. (2020). Do online searches influence sales or merely predict them? The case of motion pictures. Eur. J. Mark. 55, 337–362. doi: 10.1108/EJM-08-2019-0655
Kronrod, A., and Huber, J. (2019). Ad wearout: How time can reverse the negative effect of frequent advertising repetition on brand preference. Int. J. Res. Mark. 36, 306–324. doi: 10.1016/j.ijresmar.2018.11.008
Lee, Y., Kim, S. H., and Cha, K. C. (2021). Impact of online information on the diffusion of movies: Focusing on cultural differences. J. Bus. Res. 130, 603–609. doi: 10.1016/j.jbusres.2019.08.044
Liao, L., and Huang, T. (2021). The effect of different social media marketing channels and events on movie box office: An elaboration likelihood model perspective. Inf. Manag. 58:103481. doi: 10.1016/j.im.2021.103481
Mafael, A., Raithel, S., Taylor, C. R., and Stewart, D. W. (2021). Measuring the role of uniqueness and consistency to develop effective advertising. J. Advert. 50, 494–504. doi: 10.1080/00913367.2021.1883488
Marchand, A., Hennig-Thurau, T., and Wiertz, C. (2017). Not all digital word of mouth is created equal: understanding the respective impact of consumer reviews and microblogs on new product success. Int. J. Res. Mark. 34, 336–354. doi: 10.1016/j.ijresmar.2016.09.003
Moul, C. C. (2007). Measuring word of mouth's impact on theatrical movie admissions. J. Econo. Manag. Strateg. 16, 859–892. doi: 10.1111/j.1530-9134.2007.00160.x
MPAA (2020). Theme Report 2019. Available online at: https://www.motionpictures.org/wp-content/uploads/2020/03/MPA-THEME-2019.pdf (accessed April 15, 2022).
Oh, C., Roumani, Y., Nwankpa, J., and Hu, H.-F. (2017). Beyond likes and tweets: Consumer engagement behavior and movie box office in social media. Inf. Manag. 54, 25–37. doi: 10.1016/j.im.2016.03.004
Pauwels, K., Bharat, S., Fisher, S., and Anita, K. (2022). Should you change your ad messaging or execution? It depends on brand age. Appl. Mark. Anal. 8, 43–54. doi: 10.2139/ssrn.3913055
Pechmann, C., and Stewart, D. (1998). Advertising repetition: a critical review of wearin and wearout. Curr. Issues Res. Advert. 11, 285–329. doi: 10.1080/01633392.1988.10504936
Rainey, J. (2016). The Perils of Promotion: Pricey TV Campaigns, Fear of Change Shackles Movie Spending. Variety. Available online at: https://variety.com/2016/film/features/movie-marketing-advertising-tv-campaigns-1201724468 (accessed April 23, 2022).
Rethans, A. J., Swasy, J. L., and Marks, L. J. (1986). Effects of television commercial repetition, receiver knowledge, and commercial length: a test of the two-factor model. J. Mark. Res. 23, 50–61. doi: 10.1177/002224378602300106
Rieder, B. (2015). YouTube Data Tools, Computer software. Vers. 1.0. N.p. Available online at: https://tools.digitalmethods.net/netvizz/youtube/index.php (accessed January 12, 2018).
Schroll, R., and Grohs, R. (2019). Uncertainty in prerelease advertising. J. Advert. 48, 167–180. doi: 10.1080/00913367.2018.1532853
Song, R., Jang, S., and Cai, G. G. (2016). Does advertising indicate product quality? Evidence from prelaunch and postlaunch advertising in the movie industry. Mark. Lett. 27, 791–804. doi: 10.1007/s11002-015-9377-7
Sparck Jones, K. (1972). A statistical interpretation of term specificity and its application in retrieval. J. Doc. 28, 11–21. doi: 10.1108/eb026526
Squire, J. E. (2016). The Movie Business Book. 4th edn. in eds, Squire, J. E. New York: Simon and Schuster.
Thorbjørnsen, H., Ketelaar, P., Van 'T Riet, J., and Dahlén, M. (2015). How do teaser advertisements boost word of mouth about new products? J. Advert. Res. 55, 73–80. doi: 10.2501/JAR-55-1-073-080
Till, B. D., and Baack, D. W. (2005). Does creative advertising matter? J. Advert. 34, 47–57. doi: 10.1080/00913367.2005.10639201
Tormala, Z. L., and Clarkson, J. J. (2007). Assimilation and contrast in persuasion: the effects of source credibility in multiple message situations. Pers. Soc. Psychol. Bull. 33, 559–571. doi: 10.1177/0146167206296955
Van Laer, T., Edson Escalas, J., Ludwig, S., and Van Den Hende, E. A. (2019). What happens in vegas stays on tripadvisor? A theory and technique to understand narrativity in consumer reviews. J. Consum. Res. 46, 267–285. doi: 10.1093/jcr/ucy067
Vandenbosch, B., and Higgins, C. (1996). Information acquisition and mental models: an investigation into the relationship between behaviour and learning. Inf. Syst. Res. 7, 198–214. doi: 10.1287/isre.7.2.198
Verma, S., and Yadav, N. (2021). Past, present, and future of electronic word of mouth (EWOM). J. Interact. Mark. 53, 111–128. doi: 10.1016/j.intmar.2020.07.001
Wang, Q., Zhang, W., Li, J., Mai, F., and Ma, Z. (2022). Effect of online review sentiment on product sales: the moderating role of review credibility perception. Comput. Hum. Behav. 133, 107272. doi: 10.1016/j.chb.2022.107272
Yang, A., Choi, I. M., Abeliuk, A., and Saffer, A. (2021). The influence of interdependence in networked publics spheres: how community-level interactions affect the evolution of topics in online discourse. J. Comput. Mediated Commun. 26, 148–166. doi: 10.1093/jcmc/zmab002
Yin, D., de Vreede, T., Steele, L. M., and de Vreede, G.-J. (2022). Decide now or later: making sense of incoherence across online reviews. Inf. Syst. Res. doi: 10.1287/isre.2022.1150. [Epub ahead of print].
Zhang, W., and Watts, S. (2003). Knowledge Adoption in Online Communities of Practice. in ICIS 2003 Proceedings, 96–109. Available online at: http://aisel.aisnet.org/cgi/viewcontent.cgi?article=1114andamp;context=icis2003 (accessed April 15, 2022).
Keywords: information consistency, prelaunch advertising, WOM communication, natural language processing, YouTube, trailers
Citation: Kampani J and Nicolaides C (2023) Information consistency as response to pre-launch advertising communications: The case of YouTube trailers. Front. Commun. 7:1022139. doi: 10.3389/fcomm.2022.1022139
Received: 18 August 2022; Accepted: 29 December 2022;
Published: 20 January 2023.
Edited by:
Tat-Huei Cham, UCSI University, MalaysiaReviewed by:
Boon-Liat Cheng, Sunway University, MalaysiaCopyright © 2023 Kampani and Nicolaides. This is an open-access article distributed under the terms of the Creative Commons Attribution License (CC BY). The use, distribution or reproduction in other forums is permitted, provided the original author(s) and the copyright owner(s) are credited and that the original publication in this journal is cited, in accordance with accepted academic practice. No use, distribution or reproduction is permitted which does not comply with these terms.
*Correspondence: Julia Kampani, aWthbXBhMDFAdWN5LmFjLmN5
Disclaimer: All claims expressed in this article are solely those of the authors and do not necessarily represent those of their affiliated organizations, or those of the publisher, the editors and the reviewers. Any product that may be evaluated in this article or claim that may be made by its manufacturer is not guaranteed or endorsed by the publisher.
Research integrity at Frontiers
Learn more about the work of our research integrity team to safeguard the quality of each article we publish.