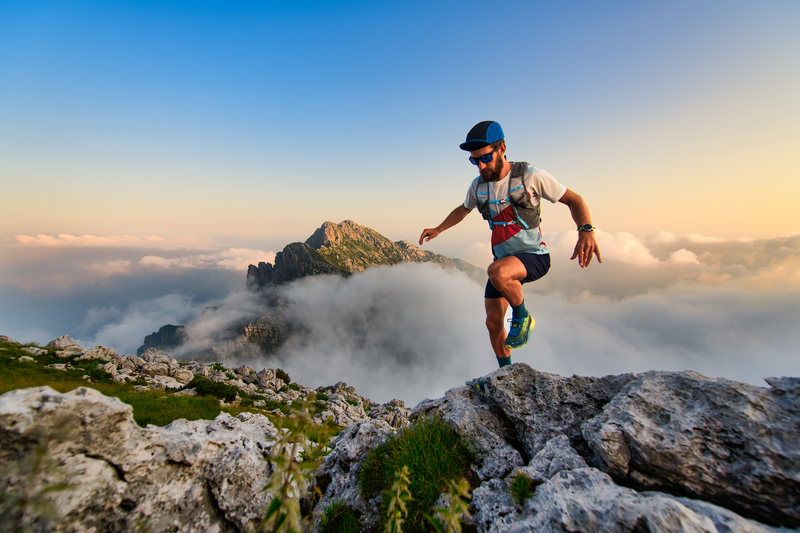
94% of researchers rate our articles as excellent or good
Learn more about the work of our research integrity team to safeguard the quality of each article we publish.
Find out more
ORIGINAL RESEARCH article
Front. Commun. , 01 December 2022
Sec. Disaster Communications
Volume 7 - 2022 | https://doi.org/10.3389/fcomm.2022.1020935
This article is part of the Research Topic Resilience in the Face of Environmental Disasters in the Age of COVID-19 View all 5 articles
Introduction: As knowledge in the scientific community increases regarding how anthropogenic CO2 loading of the atmosphere will impact future hurricane activity, the need for effective and accurate communication of hurricane risk in coastal communities—specifically to non-scientific stakeholders—also increases.
Methods: To better inform hurricane risk communication, this study employs a survey of U.S. Gulf Coast residents immediately following the active 2017 hurricane season to consider how message framing (as established by communicator word choice) and stakeholders' experience may affect receptivity to hurricane hazard information. Specifically, it tests how respondents' perceptions of hurricane risk varied with prior experience and following exposure to randomly assigned, infographic-based depictions of trends in major hurricane (≥ category 3, Saffir Simpson Scale) frequency. The set of randomly assigned infographics varied in both temporal framing (past-to-present trends based on paleo data versus present-to-future trends based on climate projections) and causal framing (‘climate change' versus ‘ocean and atmospheric change').
Results: Damaging hurricane experience may be associated with a tendency towards increasing hurricane risk perceptions after viewing the infographics. For framing effects, temporal framing of hurricane risk messages matters, with forward-looking framings (future projections) causing increased perceptions of risk and storm frequency; effects of causal framing were not significant. The results also show that Gulf Coast residents tend to be more optimistic about the future frequency of major hurricanes than most scientists and, consistent with confirmation and optimism biases, are somewhat resistant to changing their prior risk beliefs based on infographic exposure.
Discussion: These results suggest that future projections – rather than paelo analogs – may be best for quickly communicating expected increases in hurricane risk. Communicators should consider these factors when translating scientific hurricane risk information to the public.
Hurricanes have claimed over 470,000 lives and caused over $700USD billion in damages during the last century, with much of these losses likely caused by continued coastal urbanization (Pielke et al., 2008; Emanuel, 2017). Climatological research is now examining how hurricane activity will change in the twenty-first century, and, alarmingly, most modeling efforts are forecasting an increase in the frequency of intense storms (≥category 3, Saffir Simpson Scale) due to anthropogenic warming (Bender et al., 2010; Knutson et al., 2013; Kossin et al., 2020). Because accurate awareness of hurricane hazard risk is a prerequisite to preparedness actions (Grothmann and Reusswig, 2006; Kellens et al., 2012; Wachinger et al., 2013), there is a need to improve hurricane risk communication to non-scientific coastal stakeholders, specifically with regards to changes in hurricane frequency trends that are rooted in anthropogenic, vs. climate, causes.
This study considers perceptions of trends in hurricane frequency and risk among residents of coastal counties in the United States Gulf Coast region in the months immediately following the active hurricane season of 2017. Specifically, the study considers how residents' expectations regarding future trends in hurricane frequency may be affected by exposure to information about past or future trends in hurricane activity. The Gulf Coast region routinely experiences intense hurricane events, with coastal counties experiencing a landfall event ~1.5 times per century over a 160-year period (1851–2011) (HURDAT, Landsea et al., 2004). However, the short instrumental and historical records (~150 years) are insufficient to resolve how the prehistoric ocean-atmospheric system (older than 1,850 CE) has controlled hurricane activity in the Gulf Coast and, therefore, limit the current power of model projections for future hurricane activity. To resolve these issues, geologic-based approaches in the field of paleotempestology have extended our knowledge of hurricane activity back thousands of years (termed “paleo reconstructions” in this study). Annual records of hurricane passage are preserved in coastal sinkholes and blue holes on limestone landscapes (e.g., Lane et al., 2011; Denommee et al., 2014; van Hengstum et al., 2016; Wallace et al., 2019). Using such information, a study of prehistoric hurricane strikes in northwestern Florida indicates multiple, century-long intervals in the last 5,000 years during which the hurricane frequency exceed 3 intense hurricane strikes per century (Lane et al., 2011). Looking forward, modeling results (termed “future projections” in this study) forecast an increase in the frequency of dangerous, intense hurricane events in the coming decades (Bender et al., 2010; Knutson et al., 2013; Kossin et al., 2020). These results suggest that intense Gulf Coast hurricanes were not only more frequent in geologically-recent times, but will also likely again become more frequent in the near future, due in large part to anthropogenically-forced changes to the climate system.
In the last 10 years, paleo reconstructions and future projections, as complementary sources of information, have transformed knowledge in the scientific community about hurricane risk in the twenty-first century from anthropogenically-forced climate change. The central finding is cautionary—active paleo records serve as a potential analog for future hurricane activity. However, non-scientific coastal residents, stakeholders, and policy makers in the Gulf of Mexico region areas may not be aware of these recent developments in scientific knowledge; given the relative quiescence of the past century (Lane et al., 2011; Donnelly et al., 2015), these groups may significantly underestimate future hurricane risk. To improve the understanding among coastal residents and decision makers of how the risk of intense hurricane activity is changing (and prepare accordingly), it is imperative to consider how best to communicate the latest science regarding trends in Gulf-Coast hurricane frequency.
This study considers two dimensions on which risk communicators may tailor messages about change in hurricane frequency over time: (1) timeframe of trends (i.e., backward-looking trend from paleo reconstructions to present vs. forward-looking trend from present to future projections); and (2) causal attribution of trends (i.e., no attribution, attributed to climate change, or attributed to ocean and atmospheric change). Through an embedded survey experiment, varying combinations of these two dimensions were presented in an infographics format. The objective of this study is to assess how these different ways of presenting information about changes in the frequency of intense hurricanes shape Gulf Coast residents' perceptions of hurricane risk. In addition to timeframe and cause of hurricane trend change, the effect of hurricane experience is tested and other individual characteristics on perceived hurricane risk are considered as controls. The findings indicate that both the severity of recent hurricane experience and the timeframe of trend information are likely to have large and significant effects on risk beliefs for future Gulf Coast hurricanes, and that the trend-timeframe effects may vary based on prior risk beliefs. This has critical implications for the way hurricane risk is communicated to non-scientific audiences.
Using research on graphical risk communication, confirmation and optimism biases, and the role of availability and affect heuristics in risk messaging, this study seeks to isolate the influence of timeframe and causal attribution of hurricane frequency on perceived hazard risk. Risk has multiple definitions, ranging from technical approaches that focus on the frequency or probability of a hazard to social and decision sciences approaches that treat risk as a social, value-laden, and even cultural construction of potential harm (Rohrmann, 1998). As social constructions, perceived risk is subject to cognitive biases and inaccuracies (Slovic, 1991). However, all approximations of risk, whether a modeled probability or an expression of perceived harm, inherently involve uncertainty—uncertainty of the likelihood of an event, uncertainty related to the efficacy of actions taken to prepare or respond to an event, and uncertainty of the value or magnitude of the consequences of an event (Eiser et al., 2012). This uncertainty leads people to depend on others for information; therefore, the important question for studies of hazard risk is “why different individuals and groups hold the opinions that they do and how they are developed, enacted, sustained, and changed” (Eiser et al., 2012, 7).
Risk communication products have been shown to increase perceived risk of natural hazards when those receiving the information lack direct hazard experience and the information is presented graphically and generates an emotional response (Xie et al., 2011; Wachinger et al., 2013). Recent studies suggest that infographic “fact boxes” are more effective than text alone for risk communication and issue engagement (Brick et al., 2020) and that bar graphs can effectively communicate changes in the rate of hazardous events under different conditions (Okan et al., 2018). Studies also indicate that when information about trends in the frequency of natural hazards is presented using graphs that show change over time, the information garners more attention than simple numeric presentations (Visschers et al., 2009). Some research suggests that most people who view such graphs are able to use them to correctly identify trends (Correll and Heer, 2017).
In this study, the graphs for future projections show an increasing risk of intense hurricanes when one interprets the present to future trend directly. In contrast, the graphs for paleo reconstructions suggest a decreasing risk of future intense hurricanes if one projects the paleo to present trend forward in time. Although such extrapolation of past trends to future conditions based on only two observations (i.e., paleo and present-day) may be unwarranted, prevalence of the belief in the “law of small numbers” (Tversky and Kahneman, 1971) may lead to overinference. Such reliance on limited data to form beliefs about trends may be particularly common for unfamiliar topics—such as long-term trends in hurricane risk—about which research subjects lack well-formed beliefs about underlying base rates (Rabin, 2002). Accordingly, it is hypothesized that:
H1: Graphical presentation of paleo or future trends in hurricane risk via infographics will have significantly larger effects on hurricane risk perceptions than the present-only infographic control.
H2: Message recipients will be more likely to interpret trend information as suggesting higher hazard risk when presented with future projections while paleo reconstructions will tend to be interpreted as suggesting lower hazard risk.
People tend to exhibit confirmation bias by interpreting evidence to confirm or support their existing beliefs or expectations (Nickerson, 1998). Although confirmation bias may help maintain prior beliefs of positive or negative emotional valence, optimism bias (Sharot, 2011) suggests that these prior beliefs about the world are more likely to be overly optimistic (i.e., more optimistic than justifiable based on observed outcomes). Because it is difficult to directly measure individuals' actual risk level (especially for future events, for which outcomes have not yet been observed), optimism bias is generally operationalized as the difference between individuals' perceptions of their own risk and the risk to others (which is assumed to be less biased) for the same hazard (Weinstein, 1980). Such an optimism bias has been observed for many hazards, including for hurricane events (e.g., Lyu et al., 2022). Research has recently described how such overly optimistic beliefs are maintained via positive attention bias, which is the process of selectively attending to positive (vs. negative) information about the future. This results in a tendency to update beliefs more based on positive information than on negative information (Sharot, 2011; Singh et al., 2020).
In this study, optimism bias may contribute to a tendency for prior risk beliefs about hurricane frequency to be more optimistic than can be supported by the scientific consensus. To gauge the scientific consensus, we consider the latest physical science report of the Intergovernmental Panel on Climate Change (IPCC), which states that it is “likely” (66–100% chance, the probability range assigned to “likely” outcomes by the IPCC) that the proportion of major tropical cyclones has increased over the last 40 years and expresses “high confidence” that this increase will continue with future warming (Masson-Delmotte et al., 2021). Confirmation bias may contribute to a tendency to leave prior risk beliefs unchanged from before to after viewing the infographic. Positive attention bias may contribute to a tendency to interpret the infographic in a way that minimizes one's perceived risk, strengthening confirmation bias among message recipients who have more positive prior beliefs about hurricane risk while also increasing the odds that recipients of messages suggesting lower risk (as opposed to higher risk) will change their risk beliefs. It is therefore expected that:
H3: Consistent with optimism bias, respondents' pretest hurricane risk beliefs will be more optimistic than experts' beliefs regarding changes in hurricane frequency (i.e., <66% of respondents will expect the frequency of major hurricanes to increase).
H4: Consistent with confirmation bias, respondents will be more likely to hold a given risk belief at posttest when it matches their risk belief at pretest.
H5: Consistent with positive attention bias, the effects of confirmation bias will be asymmetrical: respondents' probability of retaining their prior beliefs from pre to post (as in H4) will be more resistant to changes associated with timeframe treatment (as in H2) when these prior beliefs are positive (i.e., belief in decreasing hurricane frequency) than when they are negative (i.e., belief in increasing hurricane frequency).
H6: Also consistent with positive attention bias, message recipients will be more likely to change their risk beliefs (as in H2) when presented with an infographic most readily interpreted as suggesting lower risk (i.e., a paleo reconstruction) than when presented with an infographic communicating a higher risk (i.e., a future projection).
Research has found that the manner in which risks are presented influences the level of perceived risk; specifically, risk messages that invoke a strong affective response are often easier to recall and may therefore lead message recipients to give more weight to such risks (Keller et al., 2006). When the ease to which one can bring to mind examples of hazard informs risk estimates, individuals are relying on the availability heuristic (Kahneman and Tversky, 1984). When people use feelings associated with a hazard to inform their risk perception, they are relying on the affect heuristic (Slovic et al., 2004). Slovic et al. (2004) and Keller et al. (2006) connect such affective assessments of risk with the availability heuristic (more intense emotions are more readily available to inform such assessments); together, affect and availability have been shown to shape risk perceptions for both climate change (Brosch, 2021) and hurricanes (Rickard et al., 2017). Studies have shown that risk messaging focused on climate change, in particular, is associated with negative affect (e.g., fear) as mainstream media has associated “climate change” with alarmist images and lexicons intended to induce strong emotions (O'Neill and Nicholson-Cole, 2009) and the politicization of climate change has contributed to affectively charged reactions to climate change framings (Feldman and Hart, 2018).
In this study, the affect heuristic is considered by varying attributions for hurricane frequency trends, including attributions to “changes in the climate system” or “ocean and atmospheric change.” This compares the effects of negative affect associated with climate change messaging with the effects of more neutral language. Accordingly, it is expected that:
H7: Message recipients will be more likely to interpret “climate change” attribution, in comparison to “ocean and atmospheric change” or no attribution, as suggesting higher hazard risk.
The most important determinant of perceived risk for natural hazards is hazard experience (Eiser et al., 2012; Kellens et al., 2012; Wachinger et al., 2013). A study of hurricane risk among residents of the U.S. Gulf Coast found that county-level, 15- and 19-year trends in hurricane frequency were positively related with perceptions of increased hurricane frequency (Shao and Goidel, 2016). Another study found that perceptions of increased hurricane strength were positively related to the strength of the most recent landfalling hurricane in the county (Shao et al., 2017).
Trumbo et al. (2011) confirmed the importance of direct experience on risk perceptions, finding that survey respondents who reported being directly impacted by hurricanes Katrina or Rita (both a category 3 at landfall) had significantly higher hurricane risk perceptions than non-impacted respondents. However, lack of direct loss may dampen risk perceptions among persons exposed to (but not harmed by) hurricane hazards. Prior to Hurricane Sandy, for example, Baker et al. (2012) found that Mid-Atlantic coast residents with the lowest risk perceptions were those who had experienced Hurricane Irene (2011, category 1 at landfall) one year earlier but had suffered no damage. Memories of hurricane experience also appear to fade over time, becoming less available for risk appraisal. For example, Trumbo et al. (2014) found lower risk perceptions among respondents in areas affected by Hurricanes Katrina and Rita 2 years after the storms (as compared to in their immediate aftermath). Similarly, Demuth et al. (2016) showed that, among Miami-Dade County residents, risk perceptions for a hypothetical future storm varied in complex ways depending on prior experience, with severe emotional or physical impacts from a past storm increasing risk perceptions. Taken together, these results suggest a positive association between hurricane experience and risk perceptions, particularly if such experience was recent and caused personal loss. Consistent with this literature, it is hypothesized that:
H8: Recent hurricane experience will be associated with higher hazard risk perceptions, particularly if this experience caused severe personal damages or losses.
To test the hypotheses examining the effect of timeframe and causal attribution on hazard risk perceptions an online survey presenting hurricane frequency infographics was administered in December 2017 (for full survey text, see Supplementary material). The survey used a pre-post design where risk was measured before and after respondents were shown hurricane frequency infographics. The infographics varied in the presentation of timeframe—paleo, present, and future trends—as well as causal attribution for hurricane frequency trends—climate change or ocean and atmospheric change.
The survey used a pre-post, 3 × 3 fractional factorial design (see Table 1), with six treatment groups and one control. For hurricane frequency, the control group received information about only the present-day frequency; in the treatment groups, one half were shown information about changes in frequency from the past to the present (paleo scenarios) and the other half were shown information about changes in frequency from the present to the future (future scenarios). The treatment groups also varied in the information supplied regarding the causes of the reported changes in frequency, with one third of paleo and future scenario respondents given no causal information, another third given information attributing changes to climate change, and the final third given information attributing changes to ocean and atmospheric change.
Table 1. 3 × 3 Fractional factorial design for infographic presented in survey experiment (six treatment groups and one control).
The survey sample included adult residents of Gulf Coast counties designated as part of the National Oceanic and Atmospheric Agency's Coastal Zone Management Program (CZMP). In Texas, Louisiana, Mississippi, and Alabama, respondents were recruited from all CZMP counties; in Florida, respondents were recruited from panhandle-area CMPZ counties within 50 km of the coast and from CMPZ counties in peninsular Florida that bordered the Gulf Coast.
The survey sample included 2,873 respondents, with analyses performed using 2,762 attentive respondents (111 inattentive eliminated for failing one or more attention checks). Participants were recruited by Qualtrics to fill quotas that match the demographics of the population in the targeted survey region on sex, age, and race/ethnicity. Qualtrics draws from a number of panels to recruit participants. Incentives, ranging from discounts and gift cards to entries into sweepstake drawings, are provided by Qualtrics for participation. Quotas were also specified to match the survey sample to state population proportions. Quota sampling techniques are found to produce accurate, valid samples of a population because the sample is drawn from a large panel, based on known criteria from the population (Ansolabehere and Schaffner, 2014; Miller et al., 2020). The sample, compared to the population parameters noted parenthetically, includes: sex-−48.14% males (48.64%) and 51.86% females (51.36%); age-−12.11% adults aged 18–24 years (12.48%), 38.01% adults aged 25–44 years (34.73%), 24.78% adults aged 45–64 years, and 25.10% (18.49%) adults aged 65 years and older; race/ethnicity-−55.13% (55.61%) white adults, 23.46% (23.27%) Hispanic adults, 16.60% (16.39%) African-American adults, and 4.80% (4.73%) adults of other races; and state-−3.69% (3.85%) Alabama residents, 39.33% (39.98%) Florida residents, 13.99% (13.65%) Louisiana residents, 2.19% (2.40%) Mississippi residents, and 40.79% (40.12%) Texas residents. The sample pool, compared to the targeted region, includes 1 of 2 Alabama counties, 17 of 30 Florida counties, 17 of 19 Louisiana parishes, 2 of 3 Mississippi counties, and 18 of 19 Texas counties.
To adjust the sample for the discrepancies in quotas, a weight was constructed using an iterative proportional fitting or raking method by Bergmann (2011). While the weight does not correct for all bias in the sample, it does offer a method for addressing sample skews. The results, therefore, should be more generalizable to the targeted population. Additionally, attention check questions were included throughout to ensure respondents were appropriately engaged with the survey material. Respondents with one or more failed attention checks were eliminated from the sample.
The survey provided two measures of hazard risk: (1) perceived risk from major hurricanes and (2) beliefs about changes in the frequency of major hurricanes in the Gulf Coast region. The risk perception question asked respondents: “How much risk or danger do you feel you are at from major hurricanes? Slide the marker to indicate your feeling on a scale of 0 (none at all) to 100 (extreme).” This question was modeled on Kahan et al. (2014) and Retchless (2018), who found that similar question formats provided a robust, single-item assessment of perceived risk for climate change and sea level rise, respectively. The 101-level variable for post-treatment risk perceptions (0–100 scale) was treated as continuous (Johnson and Creech, 1983). Respondents were also asked: “Think about how often major hurricanes occur in the Gulf Coast region. Which of the following comes closest to your view? Increase, decrease, no change, or random fluctuations?” Response options were modeled on frequency changes considered in previous studies of perceptions of Gulf Coast hurricane risk (Shao and Goidel, 2016) and models of climate system change, more generally (Leiserowitz et al., 2010). The questions on perceived risk and changes in hurricane frequency were asked before and after to the infographic treatment, thereby providing pre and post data to test for the effects of the infographic messaging in terms of timeframe and causal attribution of hurricane frequency. The randomly assigned infographic and associated pre-post dependent variable measures were placed at the beginning of the survey (immediately after screening questions) to avoid potentially biasing responses through exposure to other questions (especially those regarding climate change).
The primary independent variables of interest included in the model of hazard risk are timeframe of the hurricane frequency infographic and causal attribution of the hurricane trends. Hurricane frequency information was presented both textually and via bar graphs; see examples in Figure 1. Timeframes presented in the infographics included a paleo frame (showing paleo to present trends in hurricane frequency), a present frame (showing present-only hurricane frequency), and a future frame (showing present to future trends in hurricane frequency). The infographics also included a textual description of the hurricane frequency trends. This text included a causal attribution for hurricane frequency trends, varying from climate change, ocean and atmospheric change, to no cause given. Respondents were randomly assigned to infographic treatment or control (i.e., present frame, no causal attribution) groups.
Figure 1. Examples of hurricane frequency infographics randomly assigned to respondents: (A) Paleo frequency and climate change; (B) future frequency and ocean/atmospheric change; (C) control.
Frequency information for landfalling major hurricanes in the Gulf Coast region was developed based on NOAA hurricane tracks for the 1851–2011 time period (HURDAT, e.g. Landsea et al., 2004). The average frequency of major hurricane landfalls across all study area counties for this time period was 2.34, equivalent to 1.46 (~1.5) storms per county per century. Paleo frequency scenarios (analysis of the prehistoric record) were developed from the geologic-based results of Lane et al. (2011), which found that major hurricanes in the northeastern Gulf were up to four times more frequent around 2,500 years ago than in the post-1851 A.D. portion of the sediment record. Future scenarios were developed based on Bender et al. (2010), which found support for near doubling (+90%) in frequency of category 4 and 5 storms in Atlantic by 2100. Based on this data, present frequencies were shown as 1.5 major hurricane landfalls in each county per century, while past and future major hurricane landfalls were shown as twice as frequent, at 3 per county per century. Given the uncertainties inherent in developing basin-wide paleo frequencies from a single paleo record and in developing future frequencies from climate models, these estimated changes in frequency can be considered broadly consistent with the paleo record and climate model projections.
In addition to timeframe and causal attribution of hurricane frequency, hazard experience is included as a key independent variable in the model. To measure hazard experience, respondents who agreed that “a hurricane this year in the Gulf Coast region” had “threaten[ed] you or your community” completed an additional item ranking the severity of their hurricane experience in 2017 on a 0–100 scale [“What impact did the hurricane this year have on your home and personal property? Slide the marker to indicate damages from 0 (none at all) to 100 (extreme devastation)”]; respondents who did not agree that they had been threatened by a hurricane in 2017 were assigned a score of 0 on this scale.
Perceptions of trends in hurricane strength and frequency have been found to be significantly affected by political party identification and belief in climate change (Shao and Goidel, 2016; Shao et al., 2017). Accordingly, the model includes measures of climate change belief and concern as well as political ideology, more generally. To measure climate change belief, respondents were asked: “Which of these statements about the earth's temperature comes closest to your view? The earth is getting warmer mostly because of human activity such as burning fossil fuels. The earth is getting warmer mostly because of natural patterns in the earth's environment. Or the earth is not getting warmer.” This was recoded into two binary predictors: belief that the earth is getting warmer and belief that this warming is human-caused. To measure concern for the issue of climate change, respondents were asked to indicate their agreement (from strongly disagree to strongly agree) with the statement: “I am worried about the consequences that climate change may have for myself and my family.” The measure of political ideology included in the survey is the widely accepted self-placement of the ideology scale, ranging from extremely liberal (scale point of 1) to moderate (scale point of 4) to extremely conservative (scale point of 7).
Individuals' level of trust in authorities and experts is also strongly associated with risk perceptions and frequency beliefs for natural hazards and receptivity to related risk communications (Eiser et al., 2012; Kellens et al., 2012; Wachinger et al., 2013). To measure trust in authorities, the survey asked respondents a series of questions pertaining to each level of government: “How much trust do you have in [federal/state/local] government to protect you in a disaster event?” The average of responses, ranging from none to a lot, is included in the model.
Additionally, higher hurricane and flood risk perceptions have been found among persons who are older, female, less educated, lower income, ethnic minority, or homeowners (Peacock et al., 2005; Kellens et al., 2012). Shao and Goidel (2016) also found that Gulf Coast residents who were older or Hispanic were more likely to have perceived decreases in major hurricane frequency over the preceding 1–2 decades; women were also found to be more likely to perceive hurricanes as becoming stronger over this time period (Shao et al., 2017). To capture the effect of these characteristics on hurricane risk perception, the model includes measures of: sex (male or female), age (18–24; 25–44; 45–64; and 65 years and older), minority ethnicity (non-white self-identification, including Hispanic or Latino, African American, or other race or ethnic group), education (high school, some college, associate's degree, bachelor's degree, or post-graduate degree), and home ownership (rent or own).
The research questions were evaluated using regression models in R statistical software version 3.6.3 (R Core Team, 2020), with observation weights applied to correct for sampling biases (section Survey sample, Bergmann, 2011). The 101-level variable for post-treatment risk perceptions (0–100 scale) was treated as continuous (Johnson and Creech, 1983); effects on post-treatment risk from treatment and hazard experience were therefore evaluated using standard multiple linear regression for survey data (svyglm function from R Survey package, version 4.1-1, Lumley, 2004). Effects on post-treatment beliefs about change in hurricane frequency were evaluated using multinomial logistic regression with reference category as hurricanes constant through time (multinom function from the nnet package, version 7.3-12, Ripley et al., 2016). Variables in regression models were as described in sections Dependent variable measures, Independent variable measures, and Control variable measures. For the dependent variables, post-test values were modeled with pre-test values included as a covariate (Dimitrov and Rumrill, 2003; Senn, 2006). To address possible threats to external validity, interactions between pretests and treatments were tested and retained if significant (p < 0.05, Dimitrov and Rumrill, 2003). This controls for any significant pretest-treatment interactions and allows identification of groups that responded differently to treatments (Brankovic et al., 2019).
Models were validated via standard measures (Bowerman and O'connell, 1990; Menard, 2000). Independent and control variables for both linear and multinomial logistic models were evaluated for multicollinearity using generalized variable inflation factor (GVIF) testing (Fox and Monette, 1992), which adjusts traditional VIF testing to account for the multiple comparisons performed when testing effects of categorical variables using dummy variables. The square of the GVIF1/(2·df) value is considered comparable to the traditional VIF (Fox and Monette, 1992), for which values < 5 are ideal and < 10 are considered acceptable (Menard, 1995; O'Brien, 2007). Both models were also evaluated for goodness of fit using omnibus tests comparing intercept-only, intercept-and-baseline, main-effects, and main-and-interaction-effects models (Rao-Scott chi-square test for linear regression, chi-square test for multinomial logistic regression) and estimates of explained variance (R2 for linear regression and McFadden R2 for multinomial logistic regression) (McFadden, 1977; Menard, 2000). For the linear model, Huber-White robust standard errors were used to address deviations from assumptions regarding heteroskedasticity of residuals. Tabular results are presented for both linear (coefficients, standard errors, and significance levels) and multinomial logistic (beta coefficients, standard errors, and significance levels) regression models. Post-hoc testing of interaction effects is performed using estimated marginal means, which predict average responses across the levels of all model factors and the means of all model covariates (R emmeans library, Lenth, 2021), with p-values adjusted for multiple comparisons as appropriate (using Tukey, Šidák, or false discovery rate [FDR] methods). Interaction effects are explored graphically using plots of estimated marginal means of dependent variables (predicted risk perception scores or predicted probabilities of hurricane frequency beliefs) for relevant combinations of independent variables (R ggeffects library, Lüdecke, 2018).
Table 2 reports changes in the dependent variables—hurricane risk perceptions and perceived trends in hurricane frequency—due to temporal framing of hurricane frequency (paleo or future) and formatting changes to the cause of trends in hurricane frequency (climate system change or ocean and atmospheric change). The results draw on a sample of 2,762 valid responses (111 invalid responses because of failed attention checks). Among this sample, hurricane risk perceptions slightly decreased from a mean of 64.88 (on a scale 0 “no danger” to 100 “extreme danger”) with the pre-treatment measure to a mean of 62.17 with the post-treatment measure. Post-treatment risk perceptions were lower with paleo treatment (vs. future treatment) and with assignment to ocean and atmospheric change treatment (vs. climate system change treatment). For perceived trends in hurricane frequency, among those assigned the paleo timeframe treatment there was a higher percentage (28.17%) reporting belief that the number of hurricanes is decreasing. In contrast to the paleo treatment group, among those assigned the future timeframe treatment there was a higher prevalence (nearly 63%) of the perception that hurricanes were increasing through time.
Table 2. Descriptive statistics of hurricane risk, frequency, and cause by temporal framing and cause treatments.
Beyond the treatments' effects, there are notable trends between hurricane experience and perceptions of hurricane risk, frequency, and cause. Perceptions of hurricane risk increased with reported hurricane damages to personal property. The mean risk perception was 57.44 for those with no damage (either did not experience a hurricane in 2017 or reported no damage associated with a hurricane in 2017), 67.51 for those rating damage at 50, and 83.99 for those rating damage at 100. Furthermore, perceptions of increasing hurricane frequency were more prevalent with higher levels of reported hurricane damage. Among those with no damage, 40.49% said the number of hurricanes is increasing while 47.46% of those with damage at 50 and 52.78% of those with damages at 100 said the same.
Omnibus chi square tests showed that fit for both risk perceptions and frequency belief models improved significantly (p < 0.0001 for all tests) for intercept-and-baseline models (as compared to intercept-only) and for main-effects models (as compared to intercept-and-baseline). For risk perceptions, the model containing main and interaction effects did not improve fit over the main-effects model (p = 0.89). For frequency beliefs, the model with an interaction between timeframe treatment and pre-treatment beliefs significantly improved fit relative to the main-effects model (p < 0.0001); however, the model with an interaction between causal treatment and pre-treatment beliefs did not improve fit over main-effects (p = 0.60).
Based on these omnibus goodness-of-fit results, risk perceptions were modeled using a main-effects-only model (Table 3) and frequency beliefs were modeled using main-effects with an interaction between timeframe treatment and pre-treatment beliefs (Table 4). For the risk perceptions model, Model R2 of 0.66 suggests moderate to substantial model fit (Mooi and Sarstedt, 2011). For the frequency beliefs model, McFadden pseudo-R2 of 0.33 is suggestive of excellent model fit (McFadden, 1977). Multicollinearity was considered low for the risk perception model (square of the GVIF1/(2·df) value <5 for all variables) and moderate but acceptable for the frequency belief model (square of the GVIF1/(2·df) value <8 for pre-treatment risk beliefs and timeframe treatment and <5 for all other variables).
A plot of predicted post-treatment risk perceptions by pretreatment risk perceptions and assigned infographic treatments shows that higher post treatment values were associated with higher pretreatment values and with future treatments (Figure 2). Consistent with no significant interaction between timeframe treatment and pre-treatment risk perceptions, the slopes of the lines showing effects of pre-treatment risk perceptions by present, paleo, of future treatment groups are parallel. A plot of post-treatment probabilities for beliefs about change in hurricane frequency (constant, decrease, increase, or random) by pretreatment frequency beliefs and assigned infographic treatments shows that respondents tended to maintain frequency beliefs from pre to post and that future treatments were associated with higher probabilities of expecting increasing hurricane frequency while paleo treatments were associated with higher probabilities of expecting decreasing hurricane frequency (Figure 3). Consistent with the significant interaction found between timeframe treatment and pre-treatment beliefs, the plot shows that effects of assignment to present, paleo, of future treatment on probability varied with pre-treatment frequency beliefs.
Figure 2. Plot of predicted post-treatment risk perception scores by pretreatment risk perceptions and assigned infographic treatments.
Figure 3. Plot of post-treatment probabilities for beliefs about change in hurricane frequency by pretreatment frequency beliefs and assigned infographic treatments.
H1: Graphical presentation of paleo or future trends in hurricane risk via infographics will have significantly larger effects on hurricane risk perceptions than the present-only infographic control.
H1 was partially supported for risk perceptions and fully supported for frequency beliefs. For risk perceptions, future treatment resulted in an average increase in risk score of 7.82 points relative to present-only control (p < 0.001); however, the effect of paleo treatment was not significantly different from the effect of the control (p > 0.1). For frequency beliefs, future and paleo formats were found to have larger effects (i.e., larger deviations from the mean probability of each response) than the present-only format (F ratios of 320.21 [future], 253.40 [paleo], and 187.83 [present]; all significantly different from the mean response, p < 0.0001). Contrasts for present—future (F = 9.35, p < 0.0001) and present—paleo (F = 12.45, p < 0.0001) confirmed that these differences between treatment and control effects were significant.
H2: Message recipients will be more likely to interpret trend information as suggesting higher hazard risk when presented with future projections while paleo reconstructions will tend to be interpreted as suggesting lower hazard risk.
H2 was also partially supported for risk perceptions and fully supported for frequency beliefs. For risk perceptions, relative to the control, future treatment resulted in the expected significant increase in risk perceptions, but paleo treatment showed no significant effect (as described for H1). For frequency beliefs, post-hoc pairwise comparisons of the probabilities of holding each frequency belief (constant, decrease, increase, or random), regardless of pretreatment beliefs, showed significantly higher probabilities of believing that frequency will increase (decrease) in groups assigned the future (paleo) format as compared to the other three formats. Specifically, among respondents assigned the future timeframe, the probabilities of expecting an increase were on average 40.32% higher than expecting a constant rate, 46.05% higher than expecting a decrease, and 38.57% higher than expecting random fluctuations (p < 0.0001 for all comparisons). Among respondents assigned the paleo timeframe, the probabilities of expecting a decrease were on average 25.94% higher than expecting a constant rate (p < 0.0001), 17.52% higher than expecting an increase (p < 0.01), and 17.59% higher than expecting random fluctuations (p < 0.01).
H3: Consistent with optimism bias, respondents' pretest hurricane risk beliefs will be more optimistic than expert's beliefs regarding changes in hurricane frequency (i.e., <66% of respondents will expect the frequency of major hurricanes to increase).
H3 was supported. At pretest, 1,193 of 2,762 respondents expected major hurricane frequency to increase, suggesting an ~43.19% chance that any given respondent would expect an increase. A 1-sample proportions test confirmed that this was significantly lower (p < 0.0001) than the lower bound of scientific consensus for the probability for increasing major hurricane frequency (66%, as assessed by the IPCC, Masson-Delmotte et al., 2021). Among respondents who did not expect an increasing frequency, 14.01% expected a constant frequency, 2.35% expected a decreasing frequency, and 40.44% expected random fluctuations.
H4: Consistent with confirmation bias, respondents will be more likely to hold a given risk belief at posttest when it matches their risk belief at pretest.
H4 was fully supported for both risk perceptions and frequency beliefs. For risk perceptions, a one unit increase on the pretreatment risk perception score was associated with an average increase of 0.77 units at posttreatment (p < 0.001, Table 3). For frequency beliefs, pretreatment frequency belief was a significant predictor of posttreatment values for all posttreatment frequency belief categories (joint F test for overall effect of pretreatment beliefs by posttreatment beliefs, p < 0.0001 for effect of pretreatment beliefs in each of four posttreatment belief categories). To identify which pretreatment beliefs were responsible for this effect, pairwise contrasts were considered for the effects of different pretreatment frequency beliefs on the probability of holding each belief at posttreatment. Results showed that probabilities of holding each belief at posttreatment were significantly higher (p <0.0001 and 35–50% higher probabilities for all contrasts) for matching pretreatment beliefs as compared to any of the other, non-matched pretreatment beliefs (e.g., respondents who expected an increased frequency at pretreatment were more likely than those who initially expected decreased, random, or constant frequencies to expect an increased frequency at posttreatment). No other pairwise comparisons of pretreatment groups were significant (p > 0.05), suggesting that the tendency to retain pretreatment frequency beliefs at posttreatment accounted for the entire effect observed for pretreatment beliefs.
H5: Consistent with positive attention bias, the effects of confirmation bias will be asymmetrical: respondents' probability of retaining their prior beliefs from pre to post (as in H4) will be more resistant to changes associated with timeframe treatment (as in H2) when these prior beliefs are positive (i.e., belief in decreasing hurricane frequency) than when they are negative (i.e., belief in increasing hurricane frequency).
H6: Also consistent with positive attention bias, message recipients will be more likely to change their risk beliefs (as in H2) when presented with an infographic most readily interpreted as suggesting lower risk (i.e., a paleo reconstruction) than when presented with an infographic communicating a higher risk (i.e., a future projection).
H5 and H6 were not supported for risk perceptions but were partially supported for frequency beliefs. Because these hypotheses consider how the effects of timeframe treatment vary with respondents' pretreatment risk perceptions or frequency beliefs, the significance of interaction terms for pretreatment perceptions/beliefs and timeframe treatment was evaluated first. For risk perceptions, the interaction term for pretreatment risk perception scores and timeframe treatment did not significantly improve model fit (Rao-Scott likelihood ratio test for risk model with interaction terms considered in section Model selection and validation, p = 0.68), suggesting that timeframe treatment effects were independent of pretreatment risk perceptions. For frequency beliefs, pretreatment beliefs had a significant interaction with timeframe treatment (analysis of deviance chi square test, p < 0.001; Table 4). This interaction was significant (joint F test, p < 0.01) in each posttreatment frequency belief category except for “decrease.” For respondents expecting increased frequency at posttreatment, post-hoc tests showed that this interaction was driven by significant differences (p < 0.05) in the effects of future or paleo treatment (relative to the present-only control) for respondents expecting increased frequency at pretreatment as compared to those with constant or random pretreatment frequency beliefs. Examination of these significant contrasts of contrasts within the posttreatment “increase” group (and inspection of the associated quadrant of the plot of post-treatment probabilities, lower left of Figure 3) showed that these differences were associated with a smaller (larger) control-relative effect of future (paleo) treatment in respondents who initially expected frequency to increase (as compared to those who initially expected constant or random frequency). This suggests that respondents initially expecting an increased frequency tended to interpret the more neutral control in a manner consistent with their prior beliefs (consistent with confirmation bias) while also remaining somewhat open to changing their beliefs when presented with the more optimistic paleo treatment (consistent with positive attention bias).
Additional post-hoc tests were used to consider whether effects of timeframe treatment for matching or different pre-post frequency beliefs were consistent with H5 and H6, respectively. For H5, these post-hoc contrasts considered whether the probability of maintaining frequency beliefs of positive or negative emotional valence (i.e., continuing to expect a decreased or increased frequency, respectively) varied with timeframe treatment. There was no significant difference in the probability of maintaining an expectation of decreased frequency from pre to post for paleo (p = 0.59) or future (p = 0.66) treatments (as compared to the present-only control). However, there was a significant difference in the probability of maintaining an expectation of increased frequency from pre to post for paleo (31.75% lower probability, p < 0.0001) and future (10.36% higher probability, p = 0.03) treatments (as compared to the present-only control). The large and highly significant reduction in probability of maintaining an expectation of increased frequency for respondents exposed to the paelo treatment is consistent with positive attention bias as described in H5. The lack of a significant difference in the probability of maintaining an expectation of decreased frequency when exposed to the future treatment could be due in part to positive attention bias (dampening the effect of future treatment as in H5) but may also have resulted at least in part from the comparably smaller sample sizes (and larger standard errors) for respondents in the decrease-decrease group (see the discussion of H3 in this section and section Descriptive statistics).
For H6, these post-hoc tests considered whether the probability of changing to frequency beliefs with an opposite emotional valence from pre to post (e.g., switching from expecting decreased frequency to expecting increased frequency) varied with timeframe treatment. The paleo treatment group was estimated to be 31.33% more likely to change from expecting increased frequency to expecting decreased frequency than the present-only control group (p < 0.0001); the probability of switching from expecting increased frequency to decreased frequency was not significantly different between the future treatment group and the control group (p = 0.75). There was no significant difference in the probability of changing from expecting decreased frequency to expecting increased frequency from pre to post for paleo (p = 0.49) or future (p = 0.92) treatments (as compared to the present-only control). The large and highly significant increase in probability of switching from increased to decreased frequency beliefs for the paelo treatment group is consistent with positive attention bias as described in H6 (Notably, paleo treatment was also associated with a similarly large, control-relative increase in the probability of switching from expecting constant or random frequency to expecting decreased frequency; this similar effect of paleo treatment across groups with different pretreatment frequency beliefs is consistent with the finding of no significant interaction between pretreatment and treatment timeframe for the posttreatment: decrease group). The lack of a significant difference in the probability of changing from decreased to increased frequency beliefs when exposed to the future treatment could be due in part to positive attention bias (dampening the effect of future treatment as in H6) but may also have resulted at least in part from the comparably smaller sample sizes (and larger standard errors) for respondents in the decrease-increase group (see the discussion of H3 in this section and section Descriptive statistics).
H7: Message recipients will be more likely to interpret “climate change” attribution, in comparison to “ocean and atmospheric change” or no attribution, as suggesting higher hazard risk.
H7 was not supported for risk perception or frequency belief dependent variables. There was no significant effect of causal treatment on posttreatment risk perception scores (Rao-Scott likelihood ratio test for main effects only model, p = 0.78; Table 3) or frequency beliefs (analysis of deviance chi square test, p = 0.93; Table 4).
H8: Recent hurricane experience will be associated with higher hazard risk perceptions, particularly if this experience caused severe personal damages or losses.
H8 was fully supported for risk perception and partially supported for frequency beliefs. Posttreatment risk perception scores were found to be 0.07 points higher on average for each one-point increase in severity of hurricane experience (p < 0.001, Table 3). Each one-point increase in this severity score was also associated with 1% lower odds at posttest of expecting decreased (p < 0.001, Table 4) or random (p < 0.01, Table 4) hurricane frequency than of expecting constant frequency. However, more severe reported hurricane experience was not associated with higher odds of expecting an increased vs. constant hurricane frequency at posttest (p > 0.05, Table 4). As shown in Figure 4, the probability of expecting an increased hurricane frequency at posttest did increase with more severe reported hurricane experience but a similar positive relationship was also observed between the probability of expecting constant frequency and severity of hurricane experience.
Figure 4. Plot of predicted probabilities of post–treatment frequency beliefs for self-assessed severity of the impact of 2017 hurricane season.
The results suggest that infographics may influence hurricane risk beliefs and that, when updating risk beliefs based on bar graphs from such graphics, infographic users will tend to look for simple linear trends in the data, projecting them forward where necessary. Thus, bar graphs showing more frequent storms for future vs. present will tend to be interpreted as supporting increased hurricane frequency, while bar graphs showing less frequent storms for present vs. past will tend to be interpreted as supporting decreased frequency. The results also show that Gulf Coast residents tend to be more optimistic about the future frequency of major hurricanes than most scientists and, consistent with confirmation bias, are somewhat resistant to changing their prior risk beliefs based on infographic exposure. However, consistent with positive attention bias, coastal residents who initially expect hurricane frequency to increase may be much less likely to expect an increase (and more likely to expect a decrease) when shown the paleo-timeframe infographic.
More recent and damaging hurricane experience also appears to prime coastal residents to interpret risk messages in a manner that suggests higher risk from future hurricanes. Unexpectedly, the results also showed that more damaging recent hurricane experience was not only associated with a higher probability of expecting increased hurricane frequency but was also associated with a higher probability of expecting constant frequency. This unexpected result is in line with research linking the widely-observed positive relationship between hazard experience and subsequent risk perceptions with effects of hazard experience on the perceived controllability of the hazard (Helweg-Larsen and Shepperd, 2001). Persons who have recently suffered significant losses due to a hurricane event may be more likely to adopt a risk-averse strategy of vigilant awareness toward incorporating new information about the hazard into their risk beliefs, preferring to avoid surprises and lower the odds of being caught unprepared. Such a heightened need to feel in control of or well prepared for future hurricane risk could lead persons with recent negative hurricane experience to be more likely to interpret new information in a way that increases the likelihood of expecting constant or increasing hurricane frequency. Unlike decreasing frequency (which may be seen as overly optimistic considering recent hurricane experience) or random frequency (which suggests an element of unpredictability that may frustrate attempts to plan for the next storm), an expectation of constant or increasing frequency may suggest efforts to remain “realistic” given recent experience or to prepare for the possibility of an even more threatening future, respectively. Alternatively, for respondents reporting more damaging recent hurricane experience, a constant frequency of intense hurricanes may have been interpreted as maintaining an already high frequency of damaging storms (possibly at a frequency equivalent to that for respondents who lacked damaging recent hurricane experience but expected the frequency of intense hurricanes to increase).
Also unexpectedly, compared to no attribution or attribution to ocean and atmospheric change, climate change attribution was not associated with higher risk perceptions or odds of expecting increased hurricane frequency. The lack of a significant framing effect for climate change attribution could be explained in part by prior work showing that such framing effects are strongest for political independents and moderates (Benjamin et al., 2017); because the regression analysis was not segmented by political beliefs, an effect only present for less politically polarized respondents may not have been detectable. It is also possible that the specific dependent variables used in the study may not be strongly affected by this framing effect, or that the alternative framings (ocean and atmospheric change and no attribution) were not sufficiently distinct from the climate change framing to create a detectable effect (Benjamin et al., 2017 found an effect of climate change vs. global warming framings for general beliefs but not serious consequences of climate change, while Sapiains et al., 2016 found an association between climate change vs. identity-centered framing for behavioral intentions; however, studies have not shown a specific association between climate change framing vs. “ocean-atmospheric change” framing for risk beliefs about climate-related hazards, like hurricanes).
These results support the following recommendations for communicators of hurricane risk:
• Temporal framing matters. If seeking to increase perceptions of risk and storm frequency (e.g., to ensure that they are in line with expected increases in frequency of strong storms under climate change), forward-looking messages may be most successful. Messages that frame present hazard risk in relation to higher past frequencies (similar to the paleo format here) may decrease expected future frequencies (perhaps due to an assumption that the downward trend presented in the message will continue). By corollary, these results also indicate that scientists will have a greater challenge communicating the significance of paleo hurricane reconstructions to non-scientific coastal stakeholders, namely the role this data plays in delineating long-term drivers, variability, and long-term risk of natural hurricane activity in throughout the North Atlantic Basin. Paleo reconstructions may yet have value in public communications of future hurricane risk: if conditions supporting much more frequent intense North Atlantic hurricanes have happened before then they can happen again. In this way, paleo reconstructions may serve as a useful analog for communicating the risks posed by future hurricane activity. However, our study suggests that communicators will need to provide significant additional context to nudge public audiences toward this interpretation of paleo-to-present trends in intense hurricane frequency.
• Communicators should consider how their messages will likely interact with their audience's prior beliefs. Consistent with prior research, this study confirmed that prior hurricane risk beliefs affect receptivity to new information about this hazard.
• Tailoring messaging about the role of climate change in changes to hurricane frequency to an audience's worldview (see, e.g., Kahan et al., 2014) by highlighting natural (rather than anthropogenic) causes appears unlikely to affect expected storm risk and frequency.
• Where feasible, communicators should give careful consideration to the timing of their risk messages relative to recent disasters. People who have recently experienced a disaster may be more open to increasing their beliefs about hurricane risk based on new information. However, the results suggest that those reporting more personal experience of hurricane damage have a higher probability of expecting both increased and constant hurricane frequency (more research is needed to confirm this and explore possible explanations). Notably, whether recent damaging hurricane experience is more strongly associated with expecting increased or constant frequency of intense hurricanes may matter more in some communication contexts (e.g., multidecadal climate change impacts) than in others (e.g., short term hurricane preparedness).
Future work should consider testing these results for other geographic contexts and hazards. Future studies should also move beyond the simple infographics tested here to consider how hurricane-trend messages that are more detailed and context-rich affect perceptions of the hazard. For example, to better match products that people might see online or on a television newscast, the bar graphs used here could be replaced with line graphs that show a more detailed trend over time. Future work could also address possible question-order effects not accounted for in this survey design (e.g., responses to control variable measures for climate change beliefs may have been influenced by exposure to the infographic earlier in the survey). This future work may also benefit from qualitative methods (e.g., open-ended survey questions or focus groups) that could complement the quantitative, regression-based approach taken here. Ultimately, there is also a need to better understand how such messages may affect not only perceptions of hurricane risk and frequency, but also how these perceptions may drive the mitigative and adaptive behaviors needed to prepare for the likelihood that strong, damaging storms like those observed along the Gulf Coast in 2017 will continue to become more common.
The datasets presented in this study can be found in online repositories. The names of the repository/repositories and accession number(s) can be found below: The dataset generated for this study and the R code used to perform data analyses can both be found in the associated DesignSafe-CI repository: https://doi.org/10.17603/ds2-s7wv-pn32.
The studies involving human participants were reviewed and approved by IRB Administrative Office, Human Research Protection Program, Texas A&M University Division of Research. The patients/participants provided their written informed consent to participate in this study.
DR was responsible for preparing survey materials and planning survey methods, leading data analysis, preparing most manuscript content (including text, figures, and tables), and editing and revising. AR was responsible for preparing survey materials and planning survey methods, leading IRB application, providing summary statistics and associated tables, preparing some manuscript content, and editing and revising. All authors contributed to the article and approved the submitted version.
The research described in this manuscript was supported through a 2017 award from the Texas A&M University at Galveston Comprehensive Research Funds.
The authors thank Dr. Peter Van Hengstum (Texas A&M University at Galveston) for his advice and guidance in locating and interpreting paleo data for U.S. Gulf Coast hurricane activity.
The authors declare that the research was conducted in the absence of any commercial or financial relationships that could be construed as a potential conflict of interest.
All claims expressed in this article are solely those of the authors and do not necessarily represent those of their affiliated organizations, or those of the publisher, the editors and the reviewers. Any product that may be evaluated in this article, or claim that may be made by its manufacturer, is not guaranteed or endorsed by the publisher.
The Supplementary Material for this article can be found online at: https://www.frontiersin.org/articles/10.3389/fcomm.2022.1020935/full#supplementary-material
Ansolabehere, S., and Schaffner, B. F. (2014). Does survey mode still matter? Findings from a 2010 multi-mode comparison. Pol. Anal. 22, 285–303. doi: 10.1093/pan/mpt025
Baker, E. J., Broad, K., Czajkowski, J., and Orlov, B. (2012). Risk Perceptions and Preparedness among Mid-Atlantic Coastal Residents in Advance of Hurricane Sandy (No. 2012–18). Philadelphia, PA: The Wharton School, University of Pennsylvania.
Bender, M. A., Knutson, T. R., Tuleya, R. E., Sirutis, J. J., Vecchi, G. A., Garner, S. T., et al. (2010). Modeled impact of anthropogenic warming on the frequency of intense Atlantic hurricanes. Science 327, 454–458. doi: 10.1126/science.1180568
Benjamin, D., Por, H.-. H, and Budescu, D. (2017). Climate change vs. global warming: who is susceptible to the framing of climate change? Environ. Behav. 49, 745–770. doi: 10.1177/0013916516664382
Bergmann, M. (2011). IPFWEIGHT: Stata Module to Create Adjustment Weights for Surveys. Available online at: https://ideas.repec.org/c/boc/bocode/s457353.html (accessed June 15, 2022).
Bowerman, B. L., and O'connell, R. T. (1990). Linear Statistical Models: An Applied Approach. Duxbury Press.
Brankovic, M., Kardys, I., Steyerberg, E. W., Lemeshow, S., Markovic, M., Rizopoulos, D., et al. (2019). Understanding of interaction (subgroup) analysis in clinical trials. Eur. J. Clin. Invest. 49, e13145. doi: 10.1111/eci.13145
Brick, C., McDowell, M., and Freeman, A. L. J. (2020). Risk communication in tables vs. text: A registered report randomized trial on ‘fact boxes'. Royal Soc. Open Sci. 7, 190876. doi: 10.1098/rsos.190876
Brosch, T. (2021). Affect and emotions as drivers of climate change perception and action: a review. Curr. Opin. Behav. Sci. 42, 15–21. doi: 10.1016/j.cobeha.2021.02.001
Correll, M., and Heer, J. (2017). “Regression by eye: Estimating trends in bivariate visualizations,” in Proceedings of the 2017 CHI Conference on Human Factors in Computing Systems (New York, NY, United States: Association for Computing Machinery), 1387–1396.
Demuth, J. L., Morss, R. E., Lazo, J. K., and Trumbo, C. (2016). The Effects of Past Hurricane Experiences on Evacuation Intentions through Risk Perception and Efficacy Beliefs: A Mediation Analysis. Weather Clim. Soc. 8, 327–344. doi: 10.1175/WCAS-D-15-0074.1
Denommee, K. C., Bentley, S. J., and Droxler, A. W. (2014). Climatic controls on hurricane patterns: A 1200-y near-annual record from Lighthouse Reef, Belize. Sci. Rep. 4, 3876. doi: 10.1038/srep03876
Dimitrov, D. M., and Rumrill, J. (2003). Pretest-posttest designs and measurement of change. Work 20, 159–165.
Donnelly, J. P., Hawkes, A. D., Lane, P., MacDonald, D., Shuman, B. N., Toomey, M. R., et al. (2015). Climate forcing of unprecedented intense-hurricane activity in the last 2000 years. Earth's Future 3, 49–65. doi: 10.1002/2014EF000274
Eiser, R. J., Bostrom, A., Burton, I., Johnston, D. M., McClure, J., Paton, D., et al. (2012). Risk interpretation and action: A conceptual framework for responses to natural hazards. Int. J. Disas. Risk Reduct. 1, 5–16. doi: 10.1016/j.ijdrr.2012.05.002
Emanuel, K. (2017). Assessing the present and future probability of Hurricane Harvey's rainfall. Proc. Natl. Acad. Sci. 114, 12681–12684. doi: 10.1073/pnas.1716222114
Feldman, L., and Hart, P. S. (2018). Climate change as a polarizing cue: Framing effects on public support for low-carbon energy policies. Global Environ. Change 51, 54–66. doi: 10.1016/j.gloenvcha.2018.05.004
Fox, J., and Monette, G. (1992). Generalized Collinearity Diagnostics. J. Am. Stat. Assoc. 87, 178–183. doi: 10.1080/01621459.1992.10475190
Grothmann, T., and Reusswig, F. (2006). People at Risk of Flooding: Why Some Residents Take Precautionary Action While Others Do Not. Nat. Hazards 38, 101–120. doi: 10.1007/s11069-005-8604-6
Helweg-Larsen, M., and Shepperd, J. A. (2001). Do moderators of the optimistic bias affect personal or target risk estimates? A review of the literature. Person. Soc. Psychol. Rev. 5, 74–95. doi: 10.1207/S15327957PSPR0501_5
Johnson, D. R., and Creech, J. C. (1983). Ordinal measures in multiple indicator models: A simulation study of categorization error. Am. Sociol. Rev. 398–407. doi: 10.2307/2095231
Kahan, D. M., Crow, D., and Boykoff, M. T. (2014). ‘Making climate-science communication evidence-based: All the way down,” in Culture, Politics and Climate Change: How Information Shapes our Common Future (London, UK: Routledge).
Kahneman, D., and Tversky, A. (1984). Choices, values, and frames. Am. Psychol. 39, 341. doi: 10.1037/0003-066X.39.4.341
Kellens, W., Terpstra, T., and De Maeyer, P. (2012). Perception and communication of flood risks: a systematic review of empirical research. Risk Anal. 33, 24–49. doi: 10.1111/j.1539-6924.2012.01844.x
Keller, C., Siegrist, M., and Gutscher, H. (2006). The role of the affect and availability heuristics in risk communication. Risk Anal. 26, 631–639. doi: 10.1111/j.1539-6924.2006.00773.x
Knutson, T. R., Sirutis, J. J., Vecchi, G. A., Garner, S., Zhao, M., Kim, H., et al. (2013). Dynamical downscaling projections of twenty-first-century Atlantic Hurricane Activity: CMIP3 and CMIP5 model-based scenarios. J. Clim. 26, 6591–6617. doi: 10.1175/JCLI-D-12-00539.1
Kossin, J. P., Knapp, K. R., Olander, T. L., and Velden, C. S. (2020). Global increase in major tropical cyclone exceedance probability over the past four decades. Proc. Natl. Acad. Sci. 117, 11975–11980. doi: 10.1073/pnas.1920849117
Landsea, C. W., Anderson, C., Charles, N., Clark, G., Dunion, J., Fernandez-Partagas, J., et al. (2004). “The Atlantic hurricane database re-analysis project: Documentation for the 1851–1910 alterations and additions to the HURDAT database,” in Hurricanes and Typhoons: Past, Present and Future (New York, NY: Columbia University Press), 177–221.
Lane, P., Donnelly, J. P., Woodruff, J. D., and Hawkes, A. D. (2011). A decadally-resolved paleohurricane record archived in the late Holocene sediments of a Florida sinkhole. Mar. Geol. 287, 14–30. doi: 10.1016/j.margeo.2011.07.001
Leiserowitz, A., Maibach, E. W., Roser-Renouf, C., and Smith, N. (2010). Americans' knowledge of climate change (Yale Project on Climate Change Communication) (New Haven, CT: Yale University). Available online at: http://environment.yale.edu/climate/files/ClimateChangeKnowledge2010.pdf
Lüdecke, D. (2018). ggeffects: Tidy data frames of marginal effects from regression models. J. Open Source Softw. 3, 772. doi: 10.21105/joss.00772
Lumley, T. (2004). Analysis of complex survey samples. J. Stat. Softw. 9, 1–19. doi: 10.18637/jss.v009.i08
Lyu, Y.-. P, and Adams, T. (2022). Preparing for real-time weather risk management: The decision models of household evacuation under uncertainty for Taiwanese and US residents. Nat. Haz. 114, 405–425. doi: 10.1007/s11069-022-05395-8
Masson-Delmotte, V., Zhai, P., Pirani, A., Connors, S. L., Péan, C., Berger, S., et al. (2021). “Climate change 2021: The physical science basis,” in Contribution of Working Group I to the Sixth Assessment Report of the Intergovernmental Panel on Climate Change (Geneva, Switzerland: IPCC), 2.
McFadden, D. (1977). Quantitative Methods for Analyzing Travel Behavior of Individuals: Some Recent Developments (Berkeley, CA: Institute of Transportation Studies, University of California Berkeley).
Menard, S. (1995). An introduction to logistic regression diagnostics. Appl. Logist. Regr. Anal. 1, 58–79.
Menard, S. (2000). Coefficients of Determination for Multiple Logistic Regression Analysis. Am. Stat. 54, 17–24. doi: 10.1080/00031305.2000.10474502
Miller, C. A., Guidry, J. P., Dahman, B., and Thomson, M. D. (2020). A tale of two diverse Qualtrics samples: Information for online survey researchers (www.google.com/search?biw=1280&bih=593&q=Philadelphia&si=AC1wQDCwN61−ebmuwbQCO5QCrgOvEq5bkWeIzJ5JczItzAKNdTsvrV0OGkyFkKoJdZ2ahbpLStqT020JBLMabymphQrxTQRG−3S−kIdKzoIGUfkv4qqJ−EbvkpxgxHBAEOQ3nyqgd0sfJmiHaF6oexImkQxIhka7raX_RUgwkwsmYJnnzM57meuWlqJRTcNfiOAtWHFzSk06&sa=X&ved=2ahUKEwjI2bbLoL77AhXwEVkFHU−qDRwQmxMoAHoECGUQAg Philadelphia, PA: AACR).
Mooi, E., and Sarstedt, M. (2011). A Concise Guide to Market Research: The Process, Data, and Methods Using IBM SPSS Statistics (Berlin, Germany: Springer-Verlag). Available online at: https://www.springer.com/us/book/9783642125416
Nickerson, R. S. (1998). Confirmation bias: A ubiquitous phenomenon in many guises. Rev. Gener. Psychol. 2, 175–220. doi: 10.1037/1089-2680.2.2.175
O'Brien, R. M. (2007). A caution regarding rules of thumb for variance inflation factors. Qual. Quant. 41, 673–690. doi: 10.1007/s11135-006-9018-6
Okan, Y., Stone, E. R., and Bruin de Bruine, W. (2018). Designing graphs that promote both risk understanding and behavior change. Risk Anal. 38, 929–946. doi: 10.1111/risa.12895
O'Neill, S., and Nicholson-Cole, S. (2009). “Fear won't do it” promoting positive engagement with climate change through visual and iconic representations. Sci. Commun. 30, 355–379. doi: 10.1177/1075547008329201
Peacock, W. G., Brody, S. D., and Highfield, W. (2005). Hurricane risk perceptions among Florida's single family homeowners. Landscape Urban Plann. 73, 120–135. doi: 10.1016/j.landurbplan.2004.11.004
Pielke, R. A., Gratz, J., Landsea, C. W., Collins, D., Saunders, M. A., Musulin, R., et al. (2008). Normalized hurricane damage in the United States: 1900–2005. Nat. Haz. Rev. 9, 29–42. doi: 10.1061/(ASCE)1527-6988(2008)9:1(29)
R Core Team. (2020). R: A Language and Environment for Statistical Computing (Version 3.6.3). Available online at: https://www.r-project.org (accessed July 12, 2022).
Rabin, M. (2002). Inference by believers in the law of small numbers. Q. J. Econ. 117, 775–816. doi: 10.1162/003355302760193896
Retchless, D. P. (2018). Understanding local sea level rise risk perceptions and the power of maps to change them: The effects of distance and doubt. Environ. Behav. 50, 483–511. doi: 10.1177/0013916517709043
Rickard, L. N., Schuldt, J. P., Eosco, G. M., Scherer, C. W., and Daziano, R. A. (2017). The proof is in the picture: the influence of imagery and experience in perceptions of hurricane messaging. Weather Clim. Soc. 9, 471–485. doi: 10.1175/WCAS-D-16-0048.1
Rohrmann, B. (1998). The risk notion: Epistemological and empirical considerations. Integrative Risk Assessment, 39–46.
Sapiains, R., Beeton, R. J., and Walker, I. A. (2016). Individual responses to climate change: Framing effects on pro-environmental behaviors. J. Appl. Soc. Psychol. 46, 483–493. doi: 10.1111/jasp.12378
Senn, S. (2006). Change from baseline and analysis of covariance revisited. Stat. Med. 25, 4334–4344. doi: 10.1002/sim.2682
Shao, W., and Goidel, K. (2016). Seeing is believing? An examination of perceptions of local weather conditions and climate change among residents in the US Gulf Coast. Risk Anal. 36, 2136–2157. doi: 10.1111/risa.12571
Shao, W., Xian, S., Keim, B. D., Goidel, K., and Lin, N. (2017). Understanding perceptions of changing hurricane strength along the US Gulf coast. Int. J. Climatol. 37, 1716–1727. doi: 10.1002/joc.4805
Singh, L., Schüpbach, L., Moser, D. A., Wiest, R., Hermans, E. J., Aue, T., et al. (2020). The effect of optimistic expectancies on attention bias: Neural and behavioral correlates. Sci. Rep. 10, 6495. doi: 10.1038/s41598-020-61440-1
Slovic, P. (1991). Beyond Numbers: A Broader Perspective on Risk Perception. Acceptable Evidence: Science and Values in Risk Management, 48.
Slovic, P., Finucane, M. L., Peters, E., and MacGregor, D. G. (2004). Risk as analysis and risk as feelings: Some thoughts about affect, reason, risk, and rationality. Risk Anal. 24, 311–322. doi: 10.1111/j.0272-4332.2004.00433.x
Trumbo, C., Lueck, M., Marlatt, H., and Peek, L. (2011). The effect of proximity to hurricanes katrina and rita on subsequent hurricane outlook and optimistic bias. Risk Anal. 31, 1907–1918. doi: 10.1111/j.1539-6924.2011.01633.x
Trumbo, C., Meyer, M. A., Marlatt, H., Peek, L., and Morrissey, B. (2014). An assessment of change in risk perception and optimistic bias for hurricanes among gulf coast residents. Risk Anal. 34, 1013–1024. doi: 10.1111/risa.12149
Tversky, A., and Kahneman, D. (1971). Belief in the law of small numbers. Psychol. Bull. 76, 105. doi: 10.1037/h0031322
van Hengstum, P. J., van Donnelly, J. P., Fall, P. L., Toomey, M. R., Albury, N. A., and Kakuk, B. (2016). The intertropical convergence zone modulates intense hurricane strikes on the western North Atlantic margin. Sci. Rep. 6, 1–10. doi: 10.1038/srep21728
Visschers, V. H. M., Meertens, R. M., Passchier, W. W. F., and De Vries, N. N. K. (2009). Probability information in risk communication: a review of the research literature. Risk Anal. 29, 267–287. doi: 10.1111/j.1539-6924.2008.01137.x
Wachinger, G., Renn, O., Begg, C., and Kuhlicke, C. (2013). The risk perception paradox—implications for governance and communication of natural hazards. Risk Anal. 33, 1049–1065. doi: 10.1111/j.1539-6924.2012.01942.x
Wallace, E. J., Donnelly, J. P., Hengstum, P. J., van Wiman, C., Sullivan, R. M., Winkler, T. S., et al. (2019). Intense hurricane activity over the past 1500 years at South Andros Island, The Bahamas. Paleoceanogr. Paleoclimatol. n/a(n/a). doi: 10.1029/2019PA003665
Weinstein, N. D. (1980). Unrealistic optimism about future life events. J. Person. Soc. Psychol. 39, 806. doi: 10.1037/0022-3514.39.5.806
Keywords: hurricane hazards, risk communication, risk perceptions, optimism bias, temporal framing
Citation: Retchless DP and Ross AD (2022) Effects of temporal framing and hazard experience on receptivity to hurricane risk messages: A survey of U.S. Gulf Coast residents. Front. Commun. 7:1020935. doi: 10.3389/fcomm.2022.1020935
Received: 16 August 2022; Accepted: 18 November 2022;
Published: 01 December 2022.
Edited by:
Shabana Khan, Indian Research Academy, IndiaReviewed by:
Adam Rainear, West Chester University, United StatesCopyright © 2022 Retchless and Ross. This is an open-access article distributed under the terms of the Creative Commons Attribution License (CC BY). The use, distribution or reproduction in other forums is permitted, provided the original author(s) and the copyright owner(s) are credited and that the original publication in this journal is cited, in accordance with accepted academic practice. No use, distribution or reproduction is permitted which does not comply with these terms.
*Correspondence: David P. Retchless, cmV0Y2hsZWRAdGFtdWcuZWR1
Disclaimer: All claims expressed in this article are solely those of the authors and do not necessarily represent those of their affiliated organizations, or those of the publisher, the editors and the reviewers. Any product that may be evaluated in this article or claim that may be made by its manufacturer is not guaranteed or endorsed by the publisher.
Research integrity at Frontiers
Learn more about the work of our research integrity team to safeguard the quality of each article we publish.